Unleashing the Power of ChatGPT: Enhancing Sentiment Analysis in Semantic Web Technology
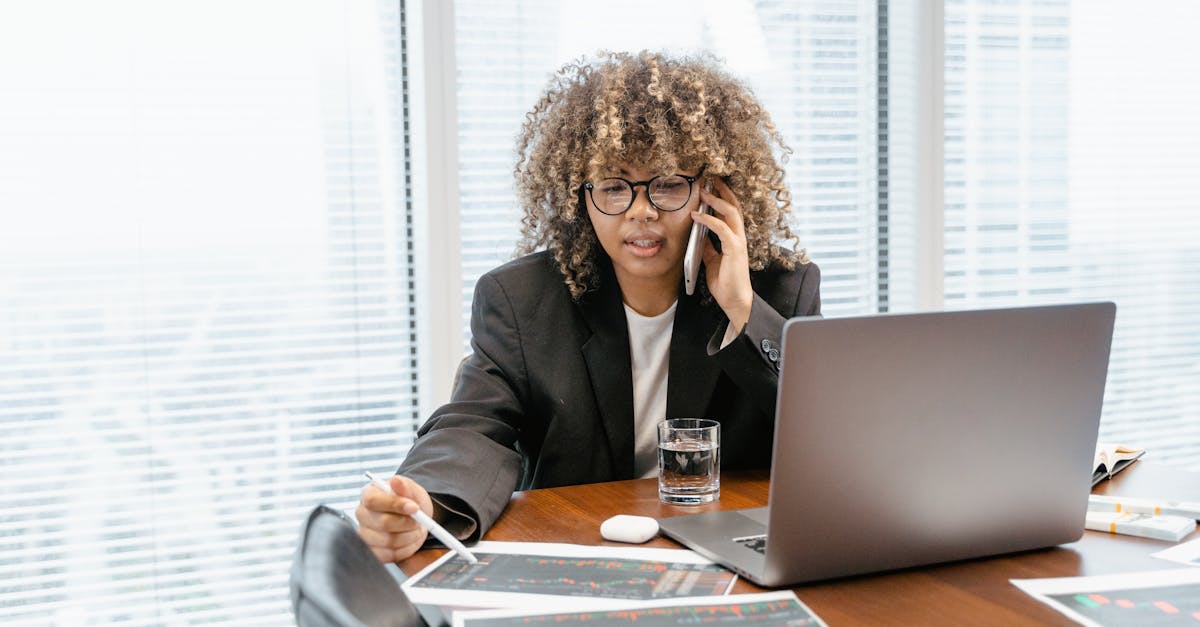
Sentiment Analysis is a field of study that focuses on understanding and classifying the sentiment expressed in textual data, such as social media posts, reviews, and customer feedback. It plays a crucial role in various industries, including marketing, customer service, and product development. However, traditional sentiment analysis approaches often fall short in capturing the true meaning and context of sentiments expressed by users. This is where Semantic Web technology comes into play.
What is Semantic Web?
The Semantic Web is an extension of the World Wide Web that enables machines to understand and interpret the meaning of information. It uses standardized vocabularies, ontologies, and linked data to create a web of interconnected and machine-readable data. The goal of the Semantic Web is to facilitate the exchange and integration of information across different systems and applications.
How Semantic Web Improves Sentiment Analysis
The traditional approach to sentiment analysis typically relies on keyword-based methods, where sentiment is determined based on the presence or absence of certain words. While this approach can provide useful insights, it often fails to capture the complexities and nuances of human language.
Semantic Web technology helps improve sentiment analysis by understanding the semantics and context of the sentiments expressed in textual data. It enables machines to go beyond simple keyword matching and consider the relationships and meanings associated with the words used in the text.
For example, let's consider the following sentence: "The new smartphone is not bad." A keyword-based sentiment analysis might classify this as a negative sentiment due to the presence of the word "bad." However, by considering the semantics and context of the sentence, a system utilizing Semantic Web technology could interpret it as a positive sentiment, since "not bad" is commonly used to express satisfaction or approval.
Utilizing Ontologies and Linked Data
A key component of the Semantic Web is the use of ontologies, which provide a formal representation of concepts and their relationships. Ontologies can be domain-specific and capture the knowledge and terminology relevant to a particular field, such as product reviews or social media conversations. By utilizing ontologies in sentiment analysis, machines can better understand the specific domain and context of the textual data, leading to more accurate sentiment classification.
In addition, Semantic Web technology facilitates the integration of linked data, which involves connecting related information from different sources. By leveraging linked data, sentiment analysis systems can gather additional context about the entities mentioned in the textual data, such as products, brands, or people. This contextual information helps in disambiguating sentiments and understanding their implications.
Benefits and Applications
By incorporating Semantic Web technology into sentiment analysis, several benefits and applications can be realized.
Firstly, it enables a more accurate interpretation of sentiments, leading to improved sentiment classification. Machines can better understand the semantics and context of the sentiments expressed, reducing misclassifications and providing more reliable insights.
Secondly, the use of ontologies and linked data enhances domain-specific sentiment analysis. Different industries and domains have their unique terminologies and context. Custom ontologies can capture this knowledge, enabling sentiment analysis systems to achieve domain-specific understanding and analysis.
Lastly, the integration of Semantic Web technology with sentiment analysis opens up avenues for advanced functionalities, such as opinion mining and sentiment summarization. Machines can uncover patterns, trends, and deeper insights from large volumes of textual data, contributing to better decision-making and understanding customer sentiments.
Conclusion
The Semantic Web holds significant potential in improving sentiment analysis by incorporating deeper semantic understanding and contextual analysis. By going beyond simple keyword matching, sentiment analysis systems can better capture the true meaning and intent behind textual sentiments. As more industries recognize the importance of accurate sentiment analysis, the adoption of Semantic Web technology is expected to grow, leading to more reliable and insightful results.
Comments:
Thank you all for taking the time to read my article. I hope you find the topic interesting!
Great article, Tiffani! I always enjoy reading about advancements in sentiment analysis. It's fascinating how ChatGPT can enhance it for semantic web technology.
I agree, Michael! The potential of ChatGPT in improving sentiment analysis is really promising. It can open up new opportunities for understanding user opinions in the context of semantic web applications.
The ability of ChatGPT to generate responses that align with user sentiment is impressive. I wonder how it performs with complex and nuanced sentiments.
That's a great point, Sophia! ChatGPT does well with basic sentiments, but handling complex and nuanced sentiments is indeed a challenge. It can still generate generic responses in some cases.
I think ChatGPT has the potential to revolutionize sentiment analysis in the semantic web. The ability to generate more context-aware responses will significantly enhance user experiences.
Absolutely, Oliver! Context plays a crucial role in understanding sentiment, and ChatGPT's advancements in leveraging semantic information can greatly improve the accuracy of sentiment analysis systems.
I'm skeptical about fully relying on ChatGPT for sentiment analysis. While it shows promise, we need to ensure it doesn't generate biased or inappropriate responses. Human review is important.
You raise a valid concern, Michelle. ChatGPT is a tool that aids sentiment analysis, but it should always be complemented with human review to mitigate potential biases or inaccuracies.
The article presents a compelling case for integrating ChatGPT in sentiment analysis for the semantic web. I'm excited to see how this technology evolves and is applied in real-world scenarios.
Indeed, Alex! The advancements in ChatGPT's sentiment analysis capabilities can unlock new possibilities in understanding user emotions and tailoring experiences on the semantic web.
I have concerns about privacy and data security when using ChatGPT for sentiment analysis. How can we ensure that sensitive information is not compromised?
Valid point, Tiffany! When using ChatGPT or any similar technology, it's crucial to handle user data responsibly, implement proper security measures, and comply with relevant regulations to protect user privacy.
To build on Tiffany's concerns, what steps can be taken to train ChatGPT on diverse datasets to avoid biases and ensure fair sentiment analysis?
Excellent question, Sophia! Training ChatGPT on diverse datasets and carefully curating those datasets can help minimize biases and ensure fair sentiment analysis. Regular evaluation and feedback loops are key.
While ChatGPT offers enhancements in sentiment analysis, I wonder if there are any limitations or potential shortcomings that should be considered.
That's a valid concern, Robert. ChatGPT can sometimes struggle with ambiguous or sarcastic sentiments, leading to incorrect analysis. It's important to be mindful of its limitations and have fallback mechanisms in place.
I agree, Emily. While ChatGPT has seen significant advancements, it's crucial to use it as a tool rather than relying solely on its analysis. Human judgment and oversight are still essential.
I'm impressed by the potential of ChatGPT in sentiment analysis, but I wonder how it can handle sentiments in different languages and cultural nuances.
That's an important consideration, John. ChatGPT performs best in English, but its performance with other languages and cultural nuances can be limited. Adapting and training it for different languages and cultures is an ongoing area of research.
The potential of ChatGPT in sentiment analysis is remarkable! It can greatly assist businesses in understanding customer feedback and improving their products and services.
Absolutely, Olivia! ChatGPT's capabilities in sentiment analysis have significant applications in areas like customer feedback analysis and brand reputation management.
Tiffani, great job on the article! The examples you provided really helped in understanding the potential impact of ChatGPT in semantic web technology.
Thank you, Daniel! I'm glad the examples resonated with you. ChatGPT's advancements can indeed make sentiment analysis more effective and valuable for the semantic web.
Are there any specific challenges in applying ChatGPT to sentiment analysis in real-time scenarios where responses need to be generated quickly?
Good point, Sophia! Real-time sentiment analysis with ChatGPT can be challenging due to the response generation time. Balancing speed and accuracy is key to enable quick analysis without compromising quality.
I can see ChatGPT being valuable in social media sentiment analysis. It can help businesses gauge public opinions quickly and respond effectively.
Exactly, Alex! Social media platforms generate a vast amount of user sentiment data, and leveraging ChatGPT for sentiment analysis can provide valuable insights to businesses.
What measures can be taken to mitigate potential ethical concerns when using ChatGPT for sentiment analysis?
Ethical considerations are crucial, Liam. Transparency about the use of ChatGPT, ensuring user consent, and addressing biases and privacy concerns are important steps in mitigating ethical risks when using it for sentiment analysis.
I wonder if there are any risks associated with over-reliance on ChatGPT's sentiment analysis in decision-making processes.
That's a valid concern, Oliver. While ChatGPT can provide valuable insights, it should be one of many factors considered in decision-making. Diverse data sources and human judgment are essential for robust decision-making.
How do you foresee the future integration of ChatGPT's sentiment analysis capabilities with other AI technologies?
Great question, Amelia! The integration of ChatGPT's sentiment analysis with other AI technologies can lead to more comprehensive and context-aware systems. Collaborative efforts can harness the strengths of different technologies for enhanced analysis.
Do you think ChatGPT will ever reach a point where it can truly understand and interpret human emotions in a nuanced way?
While it's difficult to predict the future with certainty, John, advancements in sentiment analysis, combined with other AI technologies, can bring us closer to a point where ChatGPT can better understand and interpret nuanced human emotions.
I believe it's an exciting area of research. As our understanding of human emotions evolves and AI technologies advance, we may eventually see ChatGPT reaching a higher level of emotional understanding.
In addition to sentiment analysis, what are some other potential applications for ChatGPT in the semantic web?
Good question, Robert! Beyond sentiment analysis, ChatGPT can also contribute to tasks like natural language understanding, text generation, content recommendation, and context-based interactions in the semantic web.
I'd like to see ChatGPT integrated into chatbots for customer support. It has the potential to enhance the conversational experience and provide more relevant and empathetic responses.
Absolutely, Olivia! ChatGPT's sentiment analysis capabilities can greatly improve customer support chatbots, making them more capable of understanding and addressing user concerns effectively.
It was an excellently written article, Tiffani! I appreciate your insights on the advancements ChatGPT brings to sentiment analysis in the semantic web.
Thank you so much, Daniel! I'm glad you found the article insightful. ChatGPT's advancements have the potential to make sentiment analysis more valuable in various applications.
I wonder how ChatGPT can handle sentiments expressed through emojis and other non-textual cues.
That's an interesting question, Sophia! ChatGPT's current capabilities mainly focus on text-based sentiment analysis. Incorporating non-textual cues like emojis is a research area that holds promise for more accurate sentiment analysis.
Tiffani, your article provided a comprehensive overview of ChatGPT's impact on sentiment analysis. It's exciting to see advancements in AI technologies driving innovation in the semantic web.
Thank you, Michael! I'm glad you found the article comprehensive. Indeed, AI technologies like ChatGPT have the potential to bring about significant advancements and improvements in the semantic web domain.
Thanks, Tiffani, for shedding light on the potential of ChatGPT in sentiment analysis. I'm excited to explore its applications further.
You're welcome, Emily! I'm thrilled that you found the potential of ChatGPT in sentiment analysis intriguing. There's plenty more to explore and discover in this exciting area of research.
Tiffani, your article convinced me of the importance of incorporating ChatGPT in sentiment analysis for the semantic web. Thank you for sharing your insights!
Thank you, Oliver! I appreciate your kind words. Incorporating ChatGPT in sentiment analysis opens up new possibilities for understanding user sentiments in the context of the semantic web.