Unleashing the Power of ChatGPT for Data Analysis in Novell Technology
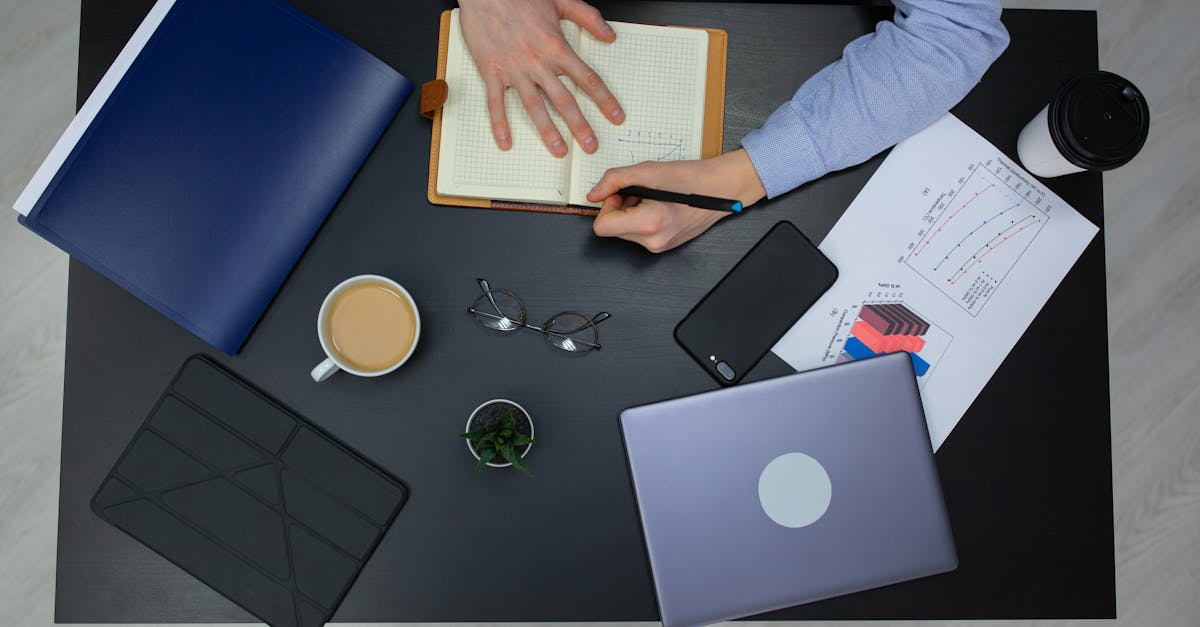
Novell is a technology that provides powerful tools and frameworks for data analysis. It is widely used in various industries, including finance, healthcare, marketing, and research. With its advanced features and capabilities, Novell enables users to efficiently analyze large sets of data and extract valuable insights.
Technology Overview
Novell is a data analysis technology that offers a range of tools and techniques to handle complex data analysis tasks. It provides powerful algorithms, statistical models, and visualizations, making it easier for data analysts and scientists to explore, analyze, and interpret the data. Novell is designed to handle big data and can efficiently process and analyze large volumes of structured and unstructured data.
Areas of Application
Novell finds applications in various domains where data analysis is crucial. In the finance industry, it can be used to analyze financial data, predict market trends, and identify potential investment opportunities. In the healthcare sector, Novell can help analyze patient records, detect patterns in disease outbreaks, and support clinical decision-making. In the marketing field, it can be utilized to analyze customer behavior, optimize campaigns, and enhance targeting strategies. Additionally, Novell is extensively used in research and academia to conduct scientific experiments, analyze survey data, and explore patterns in research data.
Usage and Benefits
Novell offers a wide range of functionalities that can greatly benefit data analysts and scientists. With Novell's intuitive interface, users can easily import and preprocess data from various sources, including databases, spreadsheets, and text files. The technology supports various data formats and can handle structured, semi-structured, and unstructured data. Novell provides a vast collection of statistical functions, machine learning algorithms, and data mining techniques that enable users to perform advanced analysis tasks such as regression analysis, clustering, classification, and text mining.
Novell also offers powerful visualization capabilities, allowing users to create meaningful and interactive visual representations of their data. Visualizations play a crucial role in data analysis as they help analysts identify patterns, trends, and outliers more easily. Novell's visualization tools enable users to create charts, graphs, and dashboards that effectively communicate insights to stakeholders and decision-makers.
Another significant advantage of Novell is its scalability and performance. It is designed to handle large-scale data analysis, enabling users to process and analyze terabytes or even petabytes of data efficiently. Novell utilizes parallel processing and distributed computing techniques to optimize the analysis process and reduce processing time. This scalability makes Novell suitable for big data analysis, where traditional software and tools may struggle to deliver timely results.
Conclusion
Novell is a powerful technology that empowers data analysts and scientists to explore, analyze, and gain insights from large sets of data. With its advanced features, intuitive interface, and scalability, Novell is an invaluable tool for industries and research institutions that heavily rely on data analysis. By leveraging Novell's capabilities, organizations can make more informed decisions, improve operational efficiency, and gain a competitive edge in today's data-driven world.
Overall, Novell contributes significantly to the field of data analysis and plays a vital role in unlocking the potential hidden within vast amounts of data.
Comments:
Thank you for reading my article on 'Unleashing the Power of ChatGPT for Data Analysis in Novell Technology'. I'm excited to hear your thoughts and answer any questions you may have!
Great article, Philip! I find the concept of using ChatGPT for data analysis intriguing. How do you see it being applied in more complex scenarios?
@Alice, I agree! It would be interesting to know how applicable ChatGPT is in complex industries such as healthcare or finance.
@Eve, I've seen applications of ChatGPT in healthcare where it assists in data analysis for personalized treatment plans or studying patient demographics.
@Julia, fascinating! Can ChatGPT aid in identifying trends or anomalies in healthcare data, leading to potential early detection of diseases or adverse events?
@Michael, absolutely! By analyzing a large volume of healthcare data, ChatGPT can assist in identifying patterns that may aid in early detection or prevention.
@Julia, that's amazing! ChatGPT could potentially contribute to improving healthcare outcomes by detecting patterns that may be challenging for human analysts to identify.
@Michael, indeed! ChatGPT can assist in identifying rare events or correlations that might be challenging to find using traditional analysis methods.
@Quincy, couldn't agree more. Early detection of rare events can significantly impact patient outcomes and the effectiveness of healthcare interventions.
@Michael, ChatGPT can also contribute to reducing the workload of healthcare professionals, allowing them to focus on critical tasks while AI assists with analysis.
@Timothy, that's a significant point. By automating portions of the analysis, healthcare professionals can allocate more time to providing quality patient care.
@Victoria, absolutely. Utilizing AI models responsibly can lead to better efficiency and the overall improvement of healthcare services.
@Timothy, @Victoria, very insightful comments! Efficient utilization of AI models in healthcare can lead to optimized resource allocation and improved patient outcomes.
@Eve, absolutely! ChatGPT's ability to understand natural language makes it a valuable tool in extracting insights from complex healthcare data.
@Philip Johnson, in healthcare data analysis, how can ChatGPT adapt to evolving medical knowledge and stay up-to-date with the latest research and findings?
@Olivia, excellent question! ChatGPT can be periodically retrained using the latest medical literature and research findings to ensure it incorporates up-to-date knowledge.
@Olivia, to keep ChatGPT updated with evolving medical knowledge, experts can curate datasets that include recent research publications and clinical guidelines.
@Rachel, that's a valuable approach. By incorporating datasets with the latest medical knowledge, ChatGPT can adapt to changes and maintain its relevance and accuracy.
@Rachel, another aspect is collaboration with domain experts who can review and validate ChatGPT's generated insights, ensuring they align with the latest research.
@Samantha, excellent point. Experts' feedback and continuous evaluation help refine the model and enhance its performance in the medical domain.
@Rachel, continuous evaluation is crucial as medical guidelines evolve rapidly, and new research is published. It ensures ChatGPT remains aligned with the best practices.
@Walter, you're right. The dynamic nature of the medical field demands ongoing assessments of AI models to keep up with the latest standards and procedures.
@Rachel, @Samantha, periodic reevaluation of the model's performance in clinical scenarios helps identify and address potential biases or limitations as well.
@Xavier, absolutely right. Bias detection and mitigation strategies should be incorporated into the evaluation process of AI models used in critical domains like healthcare.
@Alice, @Eve, absolutely! While ChatGPT has shown promise in various contexts, applying it to complex scenarios requires careful training and domain-specific fine-tuning.
Hi Philip, I enjoyed your article! Do you think ChatGPT can outperform traditional data analysis methods in terms of accuracy?
@Bob, I believe ChatGPT has the potential to achieve higher accuracy in certain tasks compared to traditional methods. However, it may still face challenges when handling noisy or incomplete data.
@Frank, I agree. The quality and comprehensiveness of the training data play a significant role in ChatGPT's accuracy. An unbiased and diverse dataset is essential.
@Karen, well said! Bias mitigation techniques can help address the limitations and improve the quality of the generated outputs.
@Karen, I fully agree with you. Diversity and representativeness of the training data are crucial to ensure fair and accurate outcomes in various applications.
@Nadia, absolutely. Transparency and ethical considerations are key factors to address when utilizing powerful AI models like ChatGPT for data analysis.
@Bob, indeed! ChatGPT's accuracy can be exceptional, but it's essential to consider the quality of the training data and potential biases.
Hello Philip, thanks for sharing your insights. I can see how ChatGPT can assist with exploratory data analysis, but does it have any limitations?
@Charlie, while ChatGPT is powerful, it is not without limitations. It may generate plausible-sounding but incorrect answers, especially if the input is ambiguous or there are knowledge gaps.
@Charlie, agreed! Evaluating and verifying the responses generated by ChatGPT during data analysis is crucial to ensure accurate insights.
Interesting read, Philip! Have you had any hands-on experience using ChatGPT for data analysis? If so, could you share a practical example?
@Daniel, I've used ChatGPT for data analysis in marketing research. It helped identify patterns and generate hypotheses for further investigation. It definitely saves time!
@Henry, that sounds fascinating! How do you ensure the reliability of ChatGPT's insights in marketing research?
@Isabella, great question! We verify the insights generated by ChatGPT by cross-referencing them with established methodologies and subject matter experts.
@Henry, it's great to see how ChatGPT complements traditional research methods. But are there any privacy concerns when using AI models for data analysis?
@Ursula, privacy is indeed a critical consideration. Proper anonymization and handling of sensitive data should be ensured when using AI models like ChatGPT.
@Henry, what are the computational requirements for deploying ChatGPT for data analysis? Does it demand extensive computing resources?
@Yara, deploying ChatGPT for data analysis generally requires significant computing resources, especially if large amounts of data need to be processed and analyzed.
@Yara, cloud-based solutions and GPU acceleration are often utilized to handle the computational demands, making it more feasible for various organizations.
@Zane, that's good to know. Accessibility to cloud platforms and advancements in hardware can facilitate the adoption of AI-driven data analysis using ChatGPT.
@Isabella, in marketing research, we emphasize validating ChatGPT's insights by comparing past successful campaigns, A/B testing, and analyzing real-time market data.
@Leo, that makes sense. It's essential to combine the power of ChatGPT with traditional research methods for reliable and actionable insights.
@Isabella, ChatGPT's insights in marketing research should be interpreted cautiously as they may lack contextual understanding and may not account for recent market shifts.
@Peter, you're right. Human expertise should always be combined with AI-generated insights to consider the broader context and make informed decisions.
@Daniel, perfect example. ChatGPT can act as a virtual assistant, automating repetitive analysis tasks and providing valuable insights to analysts.