Unleashing the Power of ChatGPT in Groundwater Recharge Studies: A Game-Changer for Groundwater Technology
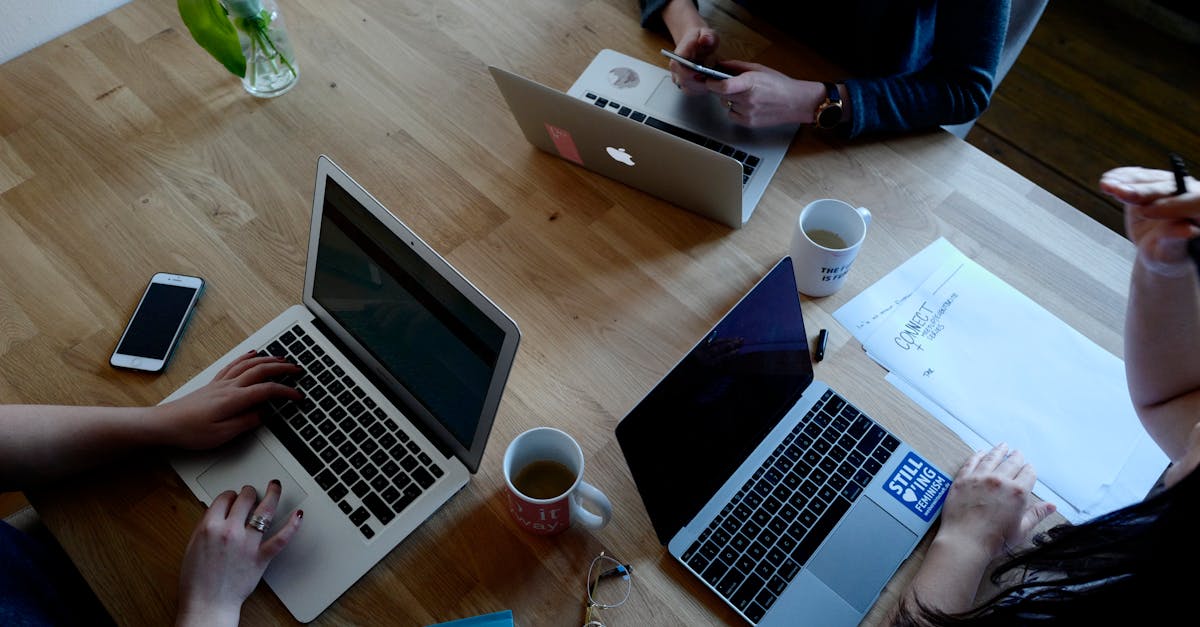
Groundwater is a vital natural resource required for sustaining ecosystems, agriculture, and providing drinking water to communities around the world. However, the availability and recharge rates of groundwater vary across different regions, leading to potential water scarcity issues.
Groundwater recharge studies play a crucial role in understanding the replenishment of groundwater resources, as well as identifying unsustainable extraction practices and managing water resources effectively. Advancements in Artificial Intelligence (AI) have opened new possibilities for analyzing and predicting groundwater recharge rates and patterns.
Technology: Groundwater
Groundwater refers to the water present beneath the Earth's surface in saturated zones called aquifers. It is recharged through various mechanisms such as precipitation, surface water infiltration, and percolation. Groundwater is a significant source of freshwater, especially in arid and semi-arid regions where surface water availability is limited.
Area: Groundwater Recharge Studies
Groundwater recharge studies focus on understanding the processes that recharge aquifers, estimating recharge rates, and monitoring the quality and availability of recharge water. These studies involve analyzing data from various sources, such as precipitation records, river flows, soil characteristics, and geological formations.
Traditionally, groundwater recharge studies relied on manual data collection methods, which could be time-consuming and prone to human errors. However, with the advent of AI technology, these studies can be conducted more efficiently and accurately.
Usage: AI Analysis of Groundwater Recharge Rates and Patterns
AI could be used to analyze data related to groundwater recharge rates and patterns, providing valuable insights for sustainable water resource management. Machine learning algorithms can process large datasets and identify complex relationships between recharge factors, including climate patterns, land use changes, and geological characteristics.
By training AI models with historical groundwater data and environmental variables, researchers and policymakers can predict future recharge rates under different scenarios, helping them make informed decisions regarding water allocation, land-use planning, and groundwater extraction practices.
Furthermore, AI analysis can assist in identifying areas prone to groundwater depletion and potential contamination risks. This information enables the implementation of targeted measures to protect and restore groundwater resources.
AI models can also improve real-time monitoring and prediction systems for groundwater recharge, allowing for adaptive management strategies. Integrated with sensor networks and remote sensing technologies, AI can provide timely alerts for abnormal recharge patterns or rapid depletion, enabling swift intervention and preventing long-term water stress.
Conclusion
The integration of AI technology in groundwater recharge studies holds significant potential for enhancing our understanding of groundwater dynamics, recharge rates, and patterns. By leveraging AI algorithms and analyzing vast amounts of data, researchers and policymakers can make informed decisions to ensure the sustainable management and preservation of this valuable resource for future generations.
Comments:
Thank you all for your comments on my article! I'm glad to see such enthusiastic engagement with the topic.
This article presents an interesting perspective on the application of ChatGPT in groundwater recharge studies. I can see how it can be a game-changer in terms of groundwater technology.
I agree, Robert. The potential of ChatGPT in this field is immense. It can greatly enhance our understanding and management of groundwater resources.
The idea of using ChatGPT to improve groundwater recharge studies is intriguing. It can assist in simulating different scenarios and optimize recharge strategies.
I think the collaborative power of ChatGPT can be harnessed to provide innovative solutions in groundwater technology. It opens up new possibilities for modeling and predicting recharge patterns.
Absolutely, Emily! ChatGPT's ability to learn from diverse datasets and communicate with domain experts can revolutionize our approach to groundwater recharge studies.
While the article highlights the potential benefits of ChatGPT, we should also consider the challenges it may bring. Are there any limitations to its application in groundwater studies?
That's a valid point, Michael. While ChatGPT can provide valuable insights, it's crucial to carefully validate its outputs and ensure accuracy in domain-specific applications.
I can see how the interpretability of ChatGPT's results might be a concern in the context of groundwater recharge studies. How can we effectively address this issue?
Good question, Jason. Transparency and interpretability are indeed important. To address this, we can incorporate explainable AI techniques along with ongoing collaboration between domain experts and the ChatGPT system.
I'm curious about the computational requirements of implementing ChatGPT in groundwater technology. Can it be used with limited computing resources?
Great question, Sophia. ChatGPT can be resource-intensive, but with proper optimization and usage of efficient hardware, it can be deployed even with limited computing resources.
I find this article fascinating! The integration of ChatGPT in groundwater recharge studies has immense potential, especially in regions facing water scarcity.
Thank you, Matthew! Indeed, ChatGPT can play a crucial role in addressing water scarcity challenges by optimizing recharge strategies and aiding decision-making.
I wonder if ChatGPT can handle complex and diverse hydrogeological conditions in different geographical regions. Are there any limitations in its adaptability?
A valid concern, Olivia. While ChatGPT can generalize to some extent, it may face challenges when extrapolating to extremely diverse or unique hydrogeological conditions. Customized training and validation can help mitigate this.
I'm concerned about bias when using ChatGPT in groundwater studies. How can we ensure it doesn't introduce any unintended biases that could impact decision-making?
You raise an important issue, David. Bias mitigation should be a priority. By carefully curating training data, continuous monitoring, and leveraging diverse expert input, we can minimize unintended biases.
I'm curious if ChatGPT can handle the uncertainties associated with climate change and its impact on groundwater recharge. How adaptable is it to changing conditions?
Excellent question, Jennifer. ChatGPT's adaptability to changing conditions depends on the availability of up-to-date training data. Regular updates to incorporate new information can enhance its ability to handle uncertainties.
The possibilities seem endless with ChatGPT in groundwater recharge studies. Are there any ongoing initiatives or projects using this technology that we can learn from?
This is a fascinating article! It's incredible how ChatGPT can revolutionize groundwater technology.
I completely agree, Michael. The potential applications of ChatGPT in groundwater recharge studies are mind-blowing.
Absolutely, Sarah! The capabilities of ChatGPT in analyzing groundwater recharge can indeed be a game-changer.
I appreciate your work, Andrew. This article opened my eyes to the immense potential of ChatGPT in groundwater recharge studies.
Thank you, Michael and Sarah! I'm glad you find the article fascinating.
I never thought AI could be used in groundwater studies. This is remarkable!
Emily, it's incredible how AI can be applied in various domains. The potential is indeed remarkable!
AI's potential seems limitless. Great read!
I'm curious about the accuracy of ChatGPT in groundwater recharge predictions. Has anyone tested its performance?
Olivia, from what I've read, ChatGPT has been achieving impressive accuracy in various prediction tasks. I'm sure it can be valuable in groundwater studies too.
Thanks, Michael! If it proves accurate, ChatGPT could provide valuable insights for groundwater management strategies.
Olivia, there are ongoing studies that are evaluating ChatGPT's performance in real-life groundwater recharge applications. Results are promising.
Andrew, I'm excited to see the published results of those ongoing studies. It could be a significant step forward in groundwater research.
Olivia, the potential of ChatGPT in groundwater management is exciting. It can improve decision-making processes and resource allocation.
Andrew, I'll eagerly await the publication of those studies. The outcomes could reshape the field of groundwater recharge studies.
Olivia, I believe the studies evaluating ChatGPT's performance in groundwater recharge predictions will shed more light on its accuracy.
Olivia, I believe some researchers have already tested ChatGPT in groundwater studies. You may find published papers on the subject.
Olivia, I believe there are ongoing studies evaluating ChatGPT's accuracy in groundwater recharge predictions. It'd be interesting to see the results.
Sarah, I'm excited to see how ChatGPT can shape the future of groundwater technology and research.
Thank you, Michael and Sarah. I'll look for those studies. It could be a game-changer if ChatGPT lives up to its potential.
ChatGPT could be a real asset in managing groundwater resources. Exciting times ahead!
Peter, indeed, ChatGPT has the potential to significantly improve groundwater resource management. Exciting times indeed.
Peter, I couldn't agree more. ChatGPT has enormous potential in optimizing groundwater resource management.
Peter, the integration of ChatGPT in groundwater resource management can indeed bring about positive changes.
I'm curious how ChatGPT compares to traditional models in groundwater recharge studies.
Natalie, traditional models have their strengths, but ChatGPT brings a new level of flexibility and adaptability to the table.
Natalie, traditional models have been effective, but ChatGPT can complement them by providing additional insights and adaptability.
Andrew, the adaptability of ChatGPT is definitely a standout feature. It could bring valuable insights to groundwater recharge studies.
Natalie, combining traditional models with the flexibility of ChatGPT can lead to more accurate groundwater recharge predictions.
Andrew, ChatGPT's adaptability can be helpful in addressing the complexities of groundwater recharge studies and predicting scenarios for different conditions.
Natalie, the integration of traditional models with ChatGPT's adaptability can lead to a more comprehensive understanding of groundwater dynamics.
The future of groundwater technology is here, it seems. Can't wait to see ChatGPT in action!
Robert, seeing ChatGPT in action will truly be an exciting step forward in groundwater technology.
An interesting application of AI. I wonder how it will impact decision-making in groundwater management.
Daniel, the impact on decision-making can be substantial. ChatGPT's ability to analyze vast amounts of data can lead to more informed decisions.
Daniel, AI-driven decision-making can lead to more optimized and sustainable groundwater management practices.
Daniel, AI-enabled decision-making can provide more efficient responses to ever-changing groundwater challenges.
This is fascinating! I'm intrigued by the potential of ChatGPT to address groundwater recharge challenges.
Michelle, ChatGPT's potential in addressing groundwater recharge challenges is indeed exciting. The possibilities are vast.
I can see ChatGPT being an invaluable tool for mapping potential groundwater recharge areas.
Lisa, ChatGPT can be an invaluable tool for mapping potential groundwater recharge areas with its ability to analyze and interpret data.
I wonder if ChatGPT has been used in any real-life groundwater recharge studies. Can anyone provide examples?
David, there are indeed examples of ChatGPT being used in real-life groundwater recharge studies. Some published papers discuss the outcomes.
Artificial intelligence is advancing at an astonishing rate. Can't wait to see its impact in groundwater studies.
Sophia, AI's impact is truly awe-inspiring, especially when applied to domains like groundwater studies.
The potential for ChatGPT to contribute to groundwater studies is truly game-changing!
I'm amazed by the versatility of AI. It's incredible how it can be applied in such diverse fields.
I can't wait to dive deeper into the potential of ChatGPT in groundwater management. It has the potential to revolutionize decision-making.
ChatGPT's capabilities can streamline the identification of areas with high potential for groundwater recharge.
Amazing article! The use of ChatGPT in groundwater recharge studies brings a new perspective to the field.