Unleashing the Power of ChatGPT: Revolutionizing Content Recommendation in Language Services
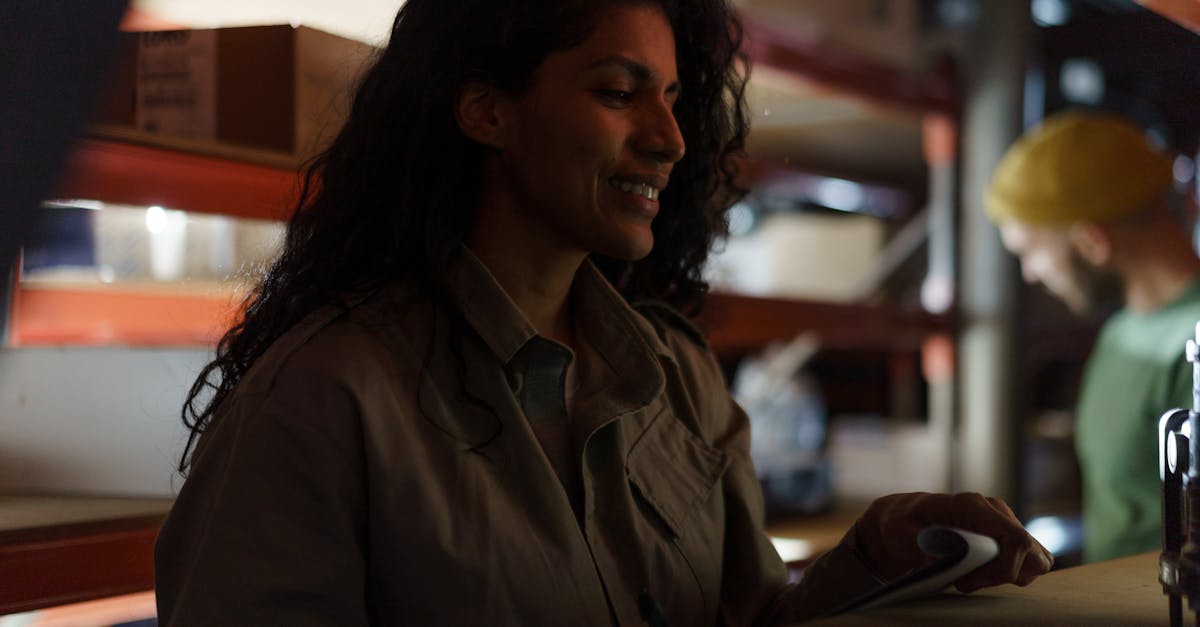
Language services have revolutionized the way we consume content online. With the vast amount of information readily available, it can be overwhelming to find relevant and interesting content that aligns with our interests. That's where content recommendation systems come into play. By leveraging machine learning algorithms, these systems analyze our prior user behavior to provide personalized content recommendations tailored to our preferences.
Understanding Content Recommendation Systems
Content recommendation systems use various techniques to understand user preferences and provide relevant recommendations. At the core, these systems gather data about user behavior, such as browsing history, search queries, and content interactions. This data is then analyzed using machine learning algorithms to identify patterns and make predictions about the user's interests.
One common approach used in content recommendation systems is collaborative filtering. This technique analyzes user interactions with content and identifies patterns of similarity between users. Based on these patterns, the system can recommend content that users with similar interests have found engaging. For example, if a user has shown a preference for technology-related articles, the system can recommend similar articles from a wide range of sources.
Another approach is content-based filtering, which leverages the characteristics of the content itself. By analyzing the textual content, metadata, or other features, the system can identify similarities between different items. For example, if a user has shown an interest in articles related to web development, the system can recommend similar articles based on the keywords, tags, or topics associated with the content.
Benefits of Personalized Content Recommendations
Personalized content recommendations offer several benefits to both users and content providers. For users, these recommendations save time and effort by presenting them with content that aligns with their interests. Instead of manually searching for relevant articles or videos, users can rely on the system to curate a tailored list of recommendations.
Content providers also benefit from personalized recommendations as they can increase user engagement and satisfaction. By delivering content that resonates with users, providers are more likely to retain users and encourage them to spend more time on their platforms. Furthermore, personalized recommendations enhance the user experience by providing a sense of personalization and customization.
Challenges and Future Developments
While content recommendation systems have proven to be effective, there are still challenges to overcome. One significant challenge is the issue of privacy and data protection. As these systems rely on collecting user data, ensuring the privacy and security of this data is crucial. Striking a balance between providing personalized recommendations and respecting user privacy is an ongoing challenge in this field.
Another challenge is the potential for bias in the recommendations. Content recommendation algorithms are designed to prioritize content based on user preferences, which can inadvertently reinforce echo chambers or filter bubbles. As users are presented with content similar to their interests, they may be less exposed to diverse viewpoints or new ideas. Addressing this issue requires careful algorithm design and continuous evaluation.
In the future, we can expect further advancements in content recommendation systems. With the advent of newer machine learning techniques, such as deep learning and natural language processing, these systems are becoming more accurate and capable of understanding complex user preferences. Furthermore, the integration of real-time data and contextual information will enable even more personalized recommendations.
Conclusion
Language services have transformed the way we discover and consume content online. Content recommendation systems, powered by machine learning algorithms, provide personalized recommendations based on prior user behavior. These systems save time for users, increase engagement for content providers, and enhance the overall user experience. However, addressing challenges related to privacy and bias will be crucial for the future development and ethical use of these systems. As technology advances, we can expect more sophisticated content recommendation systems that cater to individual preferences and offer a truly personalized content discovery experience.
Comments:
Thank you all for taking the time to read my article on ChatGPT and its potential for content recommendation in language services. I'm excited to hear your thoughts and engage in a discussion!
Great article, Je'quan! ChatGPT definitely seems like a game-changer in content recommendation. I can see how it would improve user experience and help deliver more personalized suggestions.
I agree, Bethany. The power of AI in language services is rapidly evolving. ChatGPT's ability to understand and recommend content based on natural language input can greatly enhance the effectiveness of content delivery.
Absolutely, Rahul! As someone who works in the language services industry, I can see how ChatGPT could revolutionize the way we provide recommendations to users. It has the potential to save time and improve overall satisfaction.
I'm curious about the accuracy of ChatGPT's recommendations. Can it consistently understand and recommend relevant content? AI-powered recommendations can sometimes be hit or miss.
That's a valid concern, Nathan. ChatGPT's accuracy can be influenced by the quality and size of the training data. However, OpenAI continues to train and improve the model, making it more reliable over time.
I've had positive experiences with ChatGPT on other platforms, so I'm optimistic about its content recommendation capabilities. Of course, it's crucial to monitor and fine-tune the system to ensure accurate results.
I agree, Sara. Continuous monitoring and refinement are essential to maintain accuracy and improve results. OpenAI is committed to addressing any shortcomings and enhancing the system's recommendation capabilities.
The potential of ChatGPT to improve language services is impressive, but what about privacy concerns? Recommending personalized content requires user data, and that raises some ethical questions.
Privacy is indeed a critical aspect, Michael. OpenAI has a responsibility to handle user data with care and adhere to privacy regulations. It's important to strike a balance between personalization and safeguarding user privacy.
I appreciate the potential benefits of ChatGPT, but what about the potential for biased content recommendations? AI systems can inadvertently reinforce stereotypes or present a limited perspective.
You raise a valid concern, Erica. Addressing bias is a priority for OpenAI. They are actively working to improve data collection, reduce biases, and provide clearer guidelines to ensure fair and unbiased recommendations.
Je'quan, could ChatGPT be trained on industry-specific or domain-specific data to further enhance its recommendation capabilities? Tailoring the model to different sectors could bring even more value.
Definitely, Bethany! Fine-tuning ChatGPT with industry-specific data holds great promise. It could help customize content recommendations to better align with the unique needs and preferences of different sectors.
I believe ChatGPT's potential extends beyond language services. With appropriate adjustments, it could have applications in various other domains, such as e-commerce, customer support, and more.
Absolutely, Rahul! ChatGPT's natural language understanding capability makes it versatile and adaptable to a wide range of applications. It has the potential to transform various industries with its recommendation power.
As excited as I am about ChatGPT, I wonder about the potential impact on human professionals in language services. Could it potentially replace certain roles or diminish the need for extensive human involvement?
That's a valid concern, Amanda. While ChatGPT can automate certain tasks, it's important to view it as a tool to augment human professionals rather than replace them. The expertise and subjective judgment of humans will continue to play a crucial role in language services.
I agree with Je'quan. ChatGPT can streamline processes and boost efficiency, but human professionals bring a unique touch, especially when addressing complex language nuances and personalization that AI might struggle with.
Another concern I have is the potential for misuse or malicious content recommendations. How can we ensure that ChatGPT is not exploited for harmful purposes?
That's an important consideration, Nathan. OpenAI is actively working on designing safe and responsible use guidelines and exploring ways to mitigate potential misuse. Robust moderation and community input play significant roles in maintaining a safe environment.
Je'quan, are there any limitations to ChatGPT's recommendation capabilities that we should be aware of?
Certainly, Erica. ChatGPT may not always provide accurate recommendations when dealing with highly specialized or niche topics. Its training data heavily influences its ability to recommend content effectively.
It's fascinating to see how AI is transforming language services. I'm curious to learn more about the technical aspects of ChatGPT's recommendation system. Does it primarily rely on deep learning?
Indeed, Michael. ChatGPT's recommendation system is built on deep learning techniques, particularly utilizing transformer models like the GPT-3 architecture. These models enable in-depth understanding of input data and context for effective recommendations.
Je'quan, could you provide some real-world examples where ChatGPT's content recommendations have already made a positive impact?
Certainly, Bethany. ChatGPT's content recommendation abilities have been beneficial in applications like news article suggestions, personalized social media feeds, e-learning platforms, and enhancing customer engagement on websites.
Je'quan, what challenges do you foresee in scaling up ChatGPT's content recommendation system?
Great question, Rahul. Scaling up ChatGPT's recommendation system may face challenges regarding computational resources, model complexity, and ensuring efficient training with large and diverse datasets. Addressing these challenges will be crucial.
Je'quan, do you have any thoughts on how ChatGPT could handle multiple languages for content recommendations?
Language diversity is an area of active research, Nathan. OpenAI is actively exploring ways to improve ChatGPT's ability to handle multiple languages, ensuring that it can provide effective and relevant recommendations to users across different linguistic backgrounds.
Considering the rapid advancement of AI, where do you see ChatGPT's content recommendation system headed in the next few years?
It's an exciting time for language services, Erica. In the next few years, I foresee ChatGPT's content recommendation system becoming even more accurate, adaptable, and capable of providing personalized recommendations that cater to individual user needs.
Je'quan, is there any plan to make ChatGPT's recommendation system accessible to developers for integration into their own platforms?
Absolutely, Bethany! OpenAI recognizes the value of accessibility and is actively working towards providing APIs and developer tools to incorporate ChatGPT's content recommendation system into various applications.
Je'quan, can you shed some light on the computational requirements to deploy ChatGPT's recommendation system at a large scale?
Certainly, Amanda. Deploying ChatGPT's recommendation system at a large scale requires substantial computational resources, including powerful GPUs for training and efficient infrastructure to handle high volumes of user requests in real-time.
Je'quan, thank you for sharing your insights in this article. I'm excited to witness how ChatGPT's recommendation system transforms the language services landscape!
You're welcome, Sara! I'm thrilled by the potential of ChatGPT's recommendation system, and I look forward to its positive impact on language services. Thank you all for your engaging comments!
Thank you, Je'quan, for this insightful article. It has sparked an intriguing discussion on the possibilities and challenges of ChatGPT's recommendation system in language services.