Unleashing the Power of ChatGPT: Revolutionizing Technology through Regression
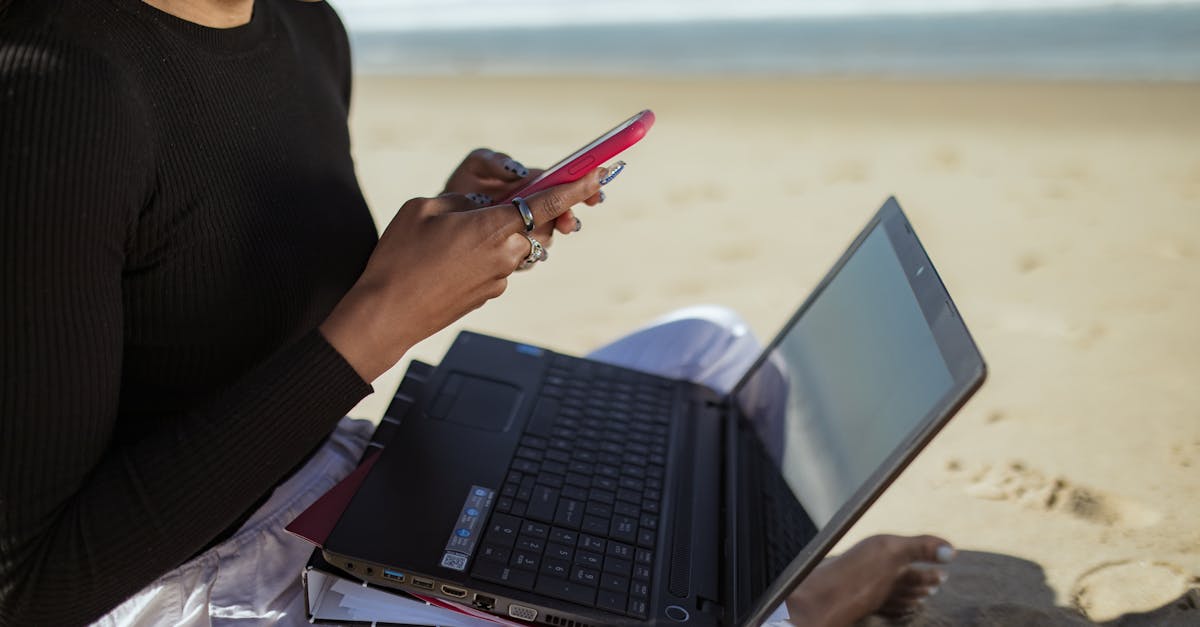
Regression analysis is a technology that has extensively been used in various fields to establish a relationship among variables. In the realm of Marketing Analytics, regression technology is applied to analyze and predict customer behavior, helping businesses to forecast market trends accurately. This article focuses on how GPT-4 (Generative Pretrained Transformer 4), the latest in cutting-edge AI technology, can be used in conjunction with regression for superior market predictions.
Understanding Regression
Regression analysis comprises statistical processes for estimating the relationships between a dependent variable (often called the 'outcome variable') and one or more independent variables (often termed 'predictors'). Regression is used frequently in forecasting and time series modeling. It enables marketers to quantify the strength of the correlation between the outcome and each predictor, while holding other predictors in the model constant.
The Role of Regression in Marketing Analytics
In Marketing Analytics, regression is essentially used to analyze how the dependent variable, such as sales revenue, is affected by independent variables, for example, advertising costs, price, product features, competitors' actions, etc. Besides, it can interpret the strength of the impact of the multiple independent variables on the dependent variable. The ultimate goal is to formulate optimized marketing strategies that yield maximum returns or desired marketing objectives.
GPT-4: The Game-changer in Regression Analysis
GPT-4, a product of OpenAI, is an artificial intelligence model primed to understand and generate human-like text based on the data it is fed. It has shown great potential in various fields, including but not limited to content writing, translation, and problem-solving. Interestingly, it can be employed in Regression Analysis for more accurate predictions and overall improved Marketing Analytics.
How GPT-4 Aids in Regression Analysis
The ability of GPT-4 to back-test billions of data points and scenarios in a fraction of a second enables it to identify intricate patterns in consumer behavior that are nearly impossible for humans to discern. It can also understand the contextual relevance of data, unlike traditional regression models, where interpretation of data is confined to pure numbers and statistics.
Moreover, GPT-4 being a part of AI technology, learns from the data it processes, hence it gets better with time. This continuous learning and evolving nature of GPT-4 brings a whole new dimension to Marketing Analytics, allowing it to stay in tune with ever-changing consumer sentiments and evolving market trends.
Benefits of Using GPT-4 in Marketing Analytics
By integrating regression analysis with GPT-4 in Marketing Analytics, businesses can reap several benefits. Firstly, they can predict consumer behavior patterns and preference trends more accurately, thereby making more precise market forecasts. Secondly, the speed and efficiency of analyzing vast amounts of data save valuable time, which can be utilized in strategic decision making and other essential operations. Lastly, continuous learning and improvement mean that your analytics keep pace with market and consumer changes, ensuring your Marketing Analytics approach continues to be effective.
In conclusion, the integration of GPT-4 and regression technology in Marketing Analytics presents a promising way forward. While the potential benefits are promising, it is important to acknowledge that the successful implementation of this approach requires a robust understanding of both regression analysis and the complexities of GPT-4. Therefore, businesses must invest in training resources and development to ensure the right skills and knowledge are in place to leverage these technologies to their full advantages.
Comments:
Thank you all for your interest in my article 'Unleashing the Power of ChatGPT: Revolutionizing Technology through Regression'. I'm pleased to see such an engaged community here. I'll be addressing your comments and questions one by one. Let's get started!
Great article, Joseph! Regression is such a powerful tool in machine learning. I'm curious, have you considered any potential drawbacks or limitations of using regression with ChatGPT?
Thank you, Megan! You bring up an excellent point. While regression is indeed a powerful technique, it does have certain limitations. One key drawback is that it assumes a linear relationship between the input features and the target. This may not hold true in more complex scenarios. Additionally, regression models are sensitive to outliers and noise in the data, which can negatively impact their performance. It's crucial to carefully preprocess the data and consider alternative techniques when necessary.
I enjoyed reading your article, Joseph. I'm particularly impressed by the potential impact of ChatGPT in revolutionizing technology. Can you elaborate on how you see regression specifically contributing to this revolution?
Thanks, Chris! Regression plays a vital role in harnessing the power of ChatGPT. By using regression, we can effectively train the model to predict continuous values, opening up avenues for more sophisticated applications. For instance, in natural language processing, regression can help us generate more accurate sentiment analysis, semantic similarity scores, or even predict the sentiment of chat responses generated by ChatGPT itself. The possibilities are truly exciting!
Joseph, your article is very interesting! I've been using ChatGPT for my customer support needs, and it has been fantastic. I'm wondering if regression can also be applied to enhance customer satisfaction ratings?
Hi Emily! I'm glad you found the article interesting. Absolutely, regression can be employed to improve customer satisfaction ratings. By collecting relevant features such as response time, sentiment analysis scores, or user feedback, you can train a regression model to predict customer satisfaction ratings based on those features. This can help identify areas for improvement in customer support and enhance overall satisfaction levels.
Joseph, as a developer, I'm always concerned about model interpretability. Do regression models used with ChatGPT provide any insights into the decision-making process or feature importance?
Hi Mark! Model interpretability is indeed an important aspect. While regression models themselves provide insights into feature importance, ChatGPT, being a language model, can pose challenges in interpretability. However, techniques such as feature selection, dimensionality reduction, and model-agnostic interpretability methods can be employed to gain insights into feature importance and decision-making. It's a trade-off between model complexity and interpretability.
Joseph, your article is well-written and informative. I believe regression can bring significant advancements to various fields. What potential future developments do you see for ChatGPT and regression?
Thank you, Sarah! I appreciate your kind words. Looking ahead, I see a couple of exciting prospects. Firstly, refining regression techniques specifically tailored for ChatGPT can lead to more accurate language generation and better chatbot performance. Secondly, integrating regression with other advanced techniques, such as deep learning and reinforcement learning, can unlock even greater potential for ChatGPT in various domains. The combination of regression's interpretability with the power of ChatGPT can push the boundaries of what's possible!
Joseph, fascinating article! Regression seems like a versatile tool. Could you provide any examples of practical applications where regression has already been successfully used with ChatGPT?
Hi Grace! Absolutely, regression has found success in numerous practical applications. One example is personalized chat-based recommendation systems, where regression models are used to predict user preferences and make tailored recommendations. Another example is automated chat moderation, where regression helps classify messages as toxic or non-toxic. By training regression models on labeled data, we can enable ChatGPT to make more informed decisions in real-time chat scenarios.
Joseph, I appreciate your insights into regression and ChatGPT. How does the size and quality of the dataset impact the performance and reliability of regression models used with ChatGPT?
Hi Jason! The dataset's size and quality play crucial roles in the performance and reliability of regression models. Larger datasets generally provide more representative samples and can enhance model generalization. On the other hand, poor quality data or insufficient data can lead to biased or inaccurate models. It's vital to ensure the training data is diverse, accurately labeled, and captures different scenarios to build reliable regression models and leverage their full potential in conjunction with ChatGPT.
Joseph, your article certainly highlights the potential of regression with ChatGPT. However, as models become increasingly complex, what challenges do you foresee in training regression models effectively?
Hi Nathan! As models grow more complex, training regression models effectively can indeed pose challenges. The increasing dimensionality of data and the need for extensive computational resources are some hurdles. Additionally, overfitting becomes a concern as models become complex, requiring techniques like regularization and cross-validation to mitigate it. Balancing model complexity, interpretability, and performance is crucial for achieving effective training of regression models, especially when combined with powerful models like ChatGPT.
Joseph, your article is very informative. Is there any ongoing research or future directions within the realm of regression in ChatGPT that you find particularly exciting?
Hi Linda! Indeed, there's ongoing research and exciting future directions. One area of interest is applying transfer learning and fine-tuning techniques to regression models used with ChatGPT. By leveraging pre-trained models and adapting them to specific regression tasks, we can potentially achieve even better performance with limited labeled data. Another exciting direction is exploring ensemble methods and combining multiple regression models to further enhance accuracy and robustness. These avenues hold great promise in pushing the boundaries of regression in ChatGPT!
Joseph, thank you for sharing your insights on regression and its applications with ChatGPT. Are there any additional resources you recommend for those interested in diving deeper into this topic?
Hi Edward! You're welcome. For those interested in delving deeper into regression and its applications with ChatGPT, I recommend exploring books like 'Applied Regression Analysis' by Draper and Smith and 'Pattern Recognition and Machine Learning' by Bishop. Online platforms such as Coursera and Udemy also offer excellent courses on regression and machine learning. Additionally, research papers in the field provide valuable insights into the latest advancements. I hope these resources help you further explore this fascinating topic!
Joseph, fantastic article! Regression is fundamental, and your explanations are clear. I'm curious, what challenges do you anticipate in deploying ChatGPT models that rely heavily on regression?
Thank you, Sophia! When deploying ChatGPT models that rely heavily on regression, several challenges may arise. Scalability and computational resources can be a concern, particularly for real-time applications or in cases requiring large-scale deployment. Ensuring model reliability, interpretability, and adaptability to changing data distributions are other challenges. Robust testing, monitoring, and continuous improvement processes become crucial for maintaining model performance and addressing potential biases or limitations. It's important to strike a balance between model complexity and practical deployment considerations when relying heavily on regression in ChatGPT models.
Joseph, your article shed light on the potential of regression in revolutionizing technology through ChatGPT. Are there any interesting real-world use cases where you see ChatGPT and regression working hand in hand?
Hi Oliver! Absolutely, ChatGPT and regression can synergize in various real-world use cases. One example is in the healthcare industry, where ChatGPT can provide personalized medical advice, and regression can predict patients' potential outcomes or help identify risk factors based on their characteristics. Another interesting use case is in the financial sector, where ChatGPT can assist in investment strategies, while regression models can predict stock prices or credit risk. The collaboration between ChatGPT and regression can unlock immense potential across industries!
Joseph, your article is enlightening. With the exponential growth of data, have you considered using big data analytics techniques with regression models in ChatGPT?
Hi Julia! Big data analytics techniques can indeed complement regression models in ChatGPT. Techniques like distributed computing, parallel processing, and data ingestion frameworks can handle large-scale datasets more efficiently, enhancing regression model training and inference. Moreover, big data analytics opens possibilities for feature engineering, data exploration, and discovering hidden patterns that can further improve the performance of regression models used with ChatGPT. When dealing with vast amounts of data, leveraging big data analytics techniques becomes essential!
Joseph, your article on ChatGPT and regression is insightful. Considering privacy concerns, how can regression models balance the need for accurate predictions while maintaining data privacy?
Hi Daniel! Privacy concerns are indeed crucial, especially when dealing with sensitive data. Regression models can strike a balance between accurate predictions and data privacy through techniques like differential privacy, secure multiparty computation, and federated learning. By anonymizing or aggregating data, encrypting computations, or decentralizing model training, we can mitigate privacy risks while still obtaining valuable insights and accurate predictions from regression models. Ensuring proper data anonymization and secure infrastructure is pivotal in maintaining the delicate balance between prediction accuracy and data privacy in ChatGPT.
Joseph, your article is a great introduction to the power of regression in ChatGPT. Are there any ethical considerations or challenges to be aware of when using regression in AI systems?
Thank you, Emma! Ethical considerations are vital in the development and deployment of AI systems. When using regression in AI systems like ChatGPT, some ethical challenges include potential biases in the training data, user privacy concerns, and the responsibility to ensure fair and transparent decision-making processes. It's crucial to address biases, validate models across different demographic groups, and prioritize strong ethical guidelines to prevent unintended harm or unfairness. Combining regression techniques with robust ethical frameworks and ongoing scrutiny can help ensure the responsible and accountable use of AI systems.
Joseph, your insights on regression's role in ChatGPT are enlightening. Can you describe any scenarios where feature engineering has significantly improved regression model performance with ChatGPT?
Hi Aaron! Feature engineering plays a pivotal role in improving regression model performance. In ChatGPT, scenarios where feature engineering has proven beneficial include sentiment analysis, where capturing subtle linguistic features, word embeddings, or semantic similarity scores can enhance sentiment prediction accuracy. Another example is chat response quality assessment, where features like response length, readability, grammaticality, and coherence can provide valuable signals to regression models. By carefully selecting and engineering relevant features, we can effectively boost regression performance with ChatGPT.
Joseph, I really enjoyed your article. When it comes to implementing regression models with ChatGPT, what are the typical challenges in obtaining labeled data for training?
Thank you, Sophie! Obtaining labeled data for training regression models with ChatGPT can indeed be challenging. A common obstacle is the need for domain-specific annotated data, which can be costly and time-consuming to acquire. Additionally, ensuring accurate labeling can be subjective, depending on the task. Human annotator biases and inter-rater reliability are factors to consider. Various approaches like active learning, semi-supervised learning, or leveraging existing labeled datasets can help alleviate the data labeling challenge. It's essential to strike a balance between data quantity, quality, and obtaining labeled data effectively when training regression models with ChatGPT.
Joseph, your article paints an exciting picture of regression's potential with ChatGPT. Can you share any best practices or tips for effectively training regression models that align with ChatGPT?
Hi Rachel! Glad you found the article exciting. When training regression models that align with ChatGPT, some best practices include: 1) Data preprocessing: clean and preprocess the data while paying attention to outliers, missing values, and suitable normalization techniques. 2) Feature selection: carefully choose relevant features to capture the characteristics that drive the regression task. 3) Regularization: incorporate regularization techniques to mitigate overfitting and improve model generalization. 4) Evaluation metrics: select appropriate evaluation metrics to gauge the model's performance. Continuous monitoring and refining of regression models based on evaluation results are also crucial for sustained success. I hope these tips help!
Joseph, your article opens up new avenues for applying regression techniques in ChatGPT. Have you encountered any performance limitations or bottlenecks specifically related to regression with ChatGPT?
Hi Ethan! While regression offers substantial benefits, a few performance limitations and bottlenecks can arise when combined with ChatGPT. As models grow more complex or datasets become larger, training and inference times can increase significantly. Balancing model complexity, resource requirements, and performance is crucial to overcome such limitations. Additionally, handling high-dimensional or sparse input features can pose challenges. Feature selection or dimensionality reduction techniques can help mitigate these issues. By carefully considering these factors and optimizing the regression-ChatGPT integration, we can ensure efficient performance and maximize their potential.
Joseph, your insights into the combination of regression and ChatGPT are fascinating. How can we ensure the model's fairness and avoid biases when training regression models for ChatGPT?
Hi Michael! Ensuring fairness and avoiding biases in regression models for ChatGPT is crucial. Some key steps to promote fairness include: 1) Diverse and representative training data: ensuring the dataset covers demographics, cultural backgrounds, and various user groups to reduce biases. 2) Regular bias audits: periodically evaluating the model's performance across different demographic slices and addressing any observed disparities. 3) Explainability and transparency: adopting transparent and interpretable regression techniques to understand how features impact predictions, aiding in identifying potential biases. By implementing checks, incorporating diverse perspectives, and continuous monitoring, we can strive for fair regression models within ChatGPT.
Joseph, your article provides valuable insights into the potential of regression with ChatGPT. Can you share any real-world success stories where regression models have transformed existing technology?
Hi Elizabeth! Regression models have indeed transformed various technologies. One notable success story is the automotive industry, where regression models have revolutionized autonomous driving. By predicting vehicle behavior, road conditions, and object detection, regression models help make critical decisions in real-time, enhancing safety and performance. Another example is in the energy sector, where regression models have optimally forecasted energy demands, aiding in managing resources effectively and minimizing waste. These are just a few instances where regression's transformative power has left a lasting impact on existing technology!
Joseph, your article on ChatGPT and regression offers great insights. What are the key factors to consider when selecting regression techniques that align well with ChatGPT?
Thank you, Sophia! Selecting regression techniques that align well with ChatGPT entails considering several factors. Some key factors include: 1) Task requirements: different regression techniques suit specific tasks better, such as linear regression for simple relationships or more advanced techniques like support vector regression or random forest regression for complex interactions. 2) Model interpretability needs: if interpretability is critical, linear regression or decision tree-based methods may be preferred. 3) Scalability: depending on the dataset size and available resources, selecting techniques with scalable implementations becomes important. 4) Existing research and best practices: considering successful applications and industry standards can guide the selection process. By aligning these factors, we can choose regression techniques that complement ChatGPT effectively.
Joseph, I thoroughly enjoyed reading your article on ChatGPT and regression. Can you elaborate on the computational requirements and infrastructure needed for training regression models when combined with ChatGPT?
Hi Stephen! Training regression models when combined with ChatGPT can require significant computational resources and infrastructure. The exact requirements depend on various factors like dataset size, complexity of the regression task, and model architecture. For large datasets or complex models, distributed computing and accelerators (e.g., GPUs) can expedite training. Adequate storage and memory are important for processing datasets and models efficiently. High-performance computing clusters or cloud environments can provide the necessary infrastructure. When training regression models with ChatGPT, it's crucial to consider scalability, hardware resources, and infrastructure capabilities for optimal performance.
Joseph, your article provides valuable insights into the potential of combining regression with ChatGPT. Are there any challenges in deploying regression models in real-time chat scenarios?
Hi Maria! Deploying regression models in real-time chat scenarios can indeed present challenges. One challenge is the need for low latency, as real-time chat demands quick responses. Efficient algorithms and computational optimizations can help address this challenge. Another consideration is the scalability of the deployment infrastructure to handle a large number of concurrent users. Additionally, maintaining model reliability and adapting to changing chat dynamics require continuous monitoring and updates. Overall, deploying regression models in real-time chat scenarios requires striking a balance between speed, performance, scalability, and maintaining quality chat experiences.
Joseph, thanks for sharing your knowledge on regression and ChatGPT. How can we handle outliers effectively when training regression models for ChatGPT?
You're welcome, Robert! Handling outliers effectively in regression models for ChatGPT is crucial for robust performance. Several approaches can be employed, such as: 1) Identifying and removing outliers: using statistical techniques like z-scores or interquartile range to detect and exclude extreme values. 2) Transformation: applying data transformations like logarithmic or power transformations to normalize data and mitigate the effect of outliers. 3) Robust regression: utilizing robust regression techniques that are less sensitive to outlier influence. By applying these techniques, we can handle outliers more effectively and enhance regression model accuracy in ChatGPT.
Joseph, your article highlights the potential of regression in ChatGPT. Do you anticipate any advancements in regression techniques that could further enhance its integration with ChatGPT?
Hi Andrew! Advancements in regression techniques can undoubtedly enhance their integration with ChatGPT further. One exciting area is the exploration of hybrid regression techniques, combining traditional regression algorithms with neural networks or deep learning. Such hybrid models can leverage the strengths of both approaches and potentially achieve improved accuracy and performance. Additionally, advancements in regularization techniques, handling missing data, and addressing high-dimensional feature spaces can contribute to refining regression models' integration with ChatGPT. As research progresses, these developments hold tremendous potential to augment the power of regression in conjunction with ChatGPT!
Joseph, your article on regression in ChatGPT is informative. Can regression models effectively handle non-linear relationships between input features and the target variable?
Thank you, David! Regression models have limitations in handling non-linear relationships between input features and the target variable. However, various techniques can help regression models capture and approximate non-linear patterns. Polynomial regression extends linear regression by introducing polynomial terms, thereby capturing non-linearities. Using basis functions or kernel methods, regression models can more effectively model complex relationships. Additionally, techniques like decision trees, random forests, or neural networks can better handle non-linear patterns. By applying these techniques, regression models can robustly capture non-linearities and enhance the representation power of ChatGPT!
Joseph, I found your article on ChatGPT and regression very informative. How can we optimize regression models to achieve better performance and computational efficiency with ChatGPT?
Hi Jennifer! Optimizing regression models can indeed yield better performance and computational efficiency when integrated into ChatGPT. Some optimization techniques include: 1) Feature selection and dimensionality reduction: identifying the most informative features and reducing the dimensionality of the feature space to minimize computational burden. 2) Regularization techniques: incorporating regularization methods like L1 or L2 regularization to prevent overfitting and improve generalization. 3) Algorithmic optimization: leveraging efficient algorithms and implementation frameworks for regression, such as stochastic gradient descent. By applying these optimizations, regression models can achieve improved performance and computational efficiency, enhancing their seamless integration with ChatGPT.
Joseph, your article showcases the potential of regression in ChatGPT. In scenarios where labeled data is limited, can semi-supervised or unsupervised regression techniques still be effective?
Hi Diana! Semi-supervised or unsupervised regression techniques can still be effective when labeled data is limited. In semi-supervised regression, the limited labeled data is complemented with a larger set of unlabeled data to improve model performance. Techniques like self-training or co-training can leverage unlabeled data to provide additional information and enhance regression accuracy. Unsupervised regression techniques can also explore patterns and clusters within the data to infer relationships and approximate target values. While labeled data is valuable, incorporating semi-supervised or unsupervised approaches can be beneficial in scenarios where labeled data is scarce.
Joseph, your article on regression in ChatGPT provides great insights. Can you elaborate on how regression models handle categorical or ordinal input features?
Thank you, Richard! Regression models can handle categorical or ordinal input features through appropriate encoding techniques. One-hot encoding is often used for categorical features, representing each category as a binary feature. In cases where ordinality matters, label encoding or ordinal encoding can be employed, mapping categories to ordered numerical values. These encoded features can then be seamlessly incorporated into regression models, allowing them to capture relationships between the encoded features and the target variable. By utilizing suitable encoding techniques, regression models can effectively handle categorical or ordinal input features within ChatGPT.
Joseph, your article offers enlightening insights into regression's integration with ChatGPT. Can you elaborate on any trade-offs between interpretability and model performance?
Hi Isabella! Trade-offs between interpretability and model performance are indeed noteworthy. More interpretable models like linear regression or decision trees offer better explainability due to their clear rules or coefficients. However, such models may not capture complex interactions and patterns as effectively as more advanced techniques like neural networks. These advanced models often sacrifice interpretability for improved performance. Striking a balance involves understanding the specific requirements of the problem at hand. In settings where model interpretability is crucial, sacrificing some performance for a more explainable model may be necessary. Evaluating trade-offs and choosing the right level of interpretability is an essential consideration when integrating regression with ChatGPT.
Joseph, your article is a great resource for understanding the power of regression in ChatGPT. Can you explain how feature selection techniques can benefit regression models for optimal performance?
Thank you, Sophie! Feature selection techniques significantly contribute to optimal performance in regression models. By identifying and selecting relevant features, regression models can focus on the most informative aspects, reducing noise and enhancing accuracy. Feature selection avoids overfitting and reduces computational complexity. Techniques like forward selection, backward elimination, or regularization-based approaches help determine which features contribute the most to the prediction task. By discarding irrelevant or redundant features, regression models become more interpretable, efficient, and less prone to overfitting. Feature selection techniques play a crucial role in fine-tuning regression models for optimal performance within ChatGPT.
Joseph, your article on ChatGPT and regression is insightful. Can you shed light on any potential pitfalls or challenges when interpreting regression models within ChatGPT?
Hi Adam! Interpreting regression models within ChatGPT can present challenges. As chat responses are generated by complex and deep neural networks, uncovering the decision-making process at a granular level can be difficult. Traditional regression models provide interpretability through coefficient analysis, but this is less straightforward in ChatGPT. Techniques like feature importance analysis or attention mechanisms can provide some insights but suffer from their inherent limitations. Ensuring the transparency of training data, incorporating model-agnostic interpretability methods, and leveraging auxiliary techniques can help tackle interpretability challenges. Employing a combination of these approaches can enable a more comprehensive understanding of regression models within ChatGPT.
Joseph, your article presents an exciting perspective on regression and ChatGPT. Are there any challenges in maintaining model reliability and avoiding biases when predicting continuous values?
Hi Benjamin! Maintaining model reliability and avoiding biases in predicting continuous values can be challenging. Biases can arise from skewed or unrepresentative training data. It is crucial to regularly evaluate the model's performance across different groups to identify potential biases and take necessary corrective actions. Addressing outliers and ensuring that the training data encompasses a diverse range of values aids in maintaining model reliability. Regular monitoring, model updates, and addressing potential biases promptly are key steps to mitigate these challenges and ensure the accurate prediction of continuous values with minimal biases within ChatGPT.
Joseph, your article on regression in ChatGPT is captivating. Can you explain any considerations or precautions when dealing with high-dimensional input features?
Thank you, Jessica! Dealing with high-dimensional input features in regression models requires careful considerations. High-dimensional features can lead to overfitting, increased computational complexity, and the curse of dimensionality. Feature selection or dimensionality reduction techniques, such as principal component analysis (PCA) or t-distributed stochastic neighbor embedding (t-SNE), can help alleviate these challenges. Additionally, regularization techniques like L1 or L2 regularization can provide control over feature importance and help mitigate overfitting. Careful feature engineering and employing suitable dimensionality reduction approaches are necessary to handle high-dimensional input features effectively within ChatGPT.
Joseph, your article on ChatGPT and regression provides valuable insights. How do you see the integration of regression models evolving with the advancements in language models like ChatGPT?
Hi Melissa! The integration of regression models with advancements in language models like ChatGPT holds immense potential. As language models advance, they become more capable of generating nuanced responses, while regression models add interpretability and accuracy to the mix. I see regression models evolving to handle complex linguistic relationships more effectively, capturing sentiments, emotion detection, and personalized responses. Specialized regression techniques that align well with language models can emerge, tailoring the algorithms to exploit language model capabilities. The integration of regression models with language models like ChatGPT offers exciting opportunities to revolutionize various applications, enhancing their interpretability, accuracy, and sophistication.
Joseph, your article on ChatGPT and regression is eye-opening. Can you explain any applications where regression models can enhance the reliability of AI-driven decision-making?
Hi Gabriel! Regression models can indeed enhance the reliability of AI-driven decision-making in various applications. One notable example is in predictive maintenance, where regression can help predict equipment failure probabilities, optimizing maintenance schedules and reducing costly downtimes. In fraud detection systems, regression techniques can assist in identifying suspicious transactions or patterns, aiding in making reliable decisions. Furthermore, regression models can provide insights into customer behavior, helping companies make data-driven decisions for marketing strategies or supply chain management. By applying regression in AI-driven systems, decision-making becomes more reliable, leading to improved outcomes and operational efficiency.
Joseph, your article on ChatGPT and regression is thought-provoking. Can you describe any potential challenges in dealing with noisy or unstructured data when training regression models for ChatGPT?
Thank you, Joshua! Dealing with noisy or unstructured data when training regression models for ChatGPT presents challenges. Noisy data can introduce errors and hinder model performance. Robust preprocessing techniques like noise removal, data cleaning, or outlier handling are essential to mitigate these challenges. Unstructured data, such as free-text inputs, increases the difficulty in capturing relevant features for regression. Techniques like text preprocessing, feature extraction, or leveraging pre-trained models can help organize and structure unstructured data, improving regression model performance. By addressing these challenges through proper preprocessing and modeling techniques, regression models can effectively handle noisy or unstructured data within ChatGPT.
Joseph, your article on regression in ChatGPT is insightful. Can you explain how feature scaling can impact the performance of regression models when combined with ChatGPT?
Hi Victoria! Feature scaling plays a crucial role in the performance of regression models when combined with ChatGPT. Scaling input features can prevent certain variables from dominating others due to differences in their scales. For instance, if a feature is measured in kilograms and another in millimeters, the latter's impact on the regression model may be overshadowed without proper scaling. Common scaling techniques include standardization (mean normalization and variance scaling) or normalization (scaling to a specific range). Feature scaling ensures that variables contribute uniformly to the regression model, leading to better model convergence, improved performance, and unbiased coefficients within ChatGPT.
Joseph, your article provides excellent insights into regression and its integration with ChatGPT. How can we address multicollinearity among input features when training regression models?
Thank you, Samantha! Addressing multicollinearity among input features is vital for reliable regression models. To handle multicollinearity, we can employ techniques such as: 1) Feature selection: choosing a subset of features that contribute the most to the regression task, thereby minimizing collinear relationships. 2) Dimensionality reduction: techniques like principal component analysis (PCA) can transform the correlated features into a reduced set of uncorrelated principal components. 3) VIF (Variance Inflation Factor) analysis: identifying and removing features with high VIF values, indicating strong collinearity. By addressing multicollinearity, regression models become more robust, stable, and better suited for integration with ChatGPT.
Joseph, your article on ChatGPT and regression is highly informative. Can you describe any potential challenges in model performance generalization across different domains or user groups?
Hi Thomas! Model performance generalization across different domains or user groups can indeed present challenges. Regression models trained on a specific domain may not perform optimally when applied to new or unseen domains. Performance generalization can be hindered by domain-specific nuances, variations in data distributions, or lack of representativeness in the training data for certain user groups. Techniques like domain adaptation, transfer learning, or fine-tuning on domain-specific data can help alleviate such challenges. Continuous evaluation and monitoring across different domains or demographic groups are key to achieving better generalization in regression models integrated with ChatGPT.
Joseph, your article on regression and ChatGPT is highly insightful. Can you explain any precautions or techniques to avoid overfitting when training regression models?
Hi Emma! Avoiding overfitting is crucial when training regression models. Some precautions and techniques to mitigate overfitting include: 1) Incorporating regularization techniques like L1 or L2 regularization to penalize large regression coefficients and improve model generalization. 2) Using k-fold cross-validation to assess model performance on different subsets of the data, ensuring better estimates of the model's true performance. 3) Applying early stopping during model training to prevent excessive optimization on the training data at the expense of generalization. By leveraging these techniques and finding the right balance between model complexity and generalization, regression models can minimize overfitting and perform effectively in ChatGPT scenarios.
Joseph, your article on ChatGPT and regression is enlightening. Can you elaborate on the potential impact of incorporating time-series analysis with regression in ChatGPT?
Thank you, Sophia! Incorporating time-series analysis with regression in ChatGPT opens new possibilities. Time-series regression techniques can help capture temporal dependencies and trends in the data. By considering time as an essential feature, regression models gain the ability to predict and understand how continuous values change dynamically over time. This integration can be instrumental in forecasting, anomaly detection, or predicting trends in various domains. The combined power of time-series analysis and regression within ChatGPT enables accurate predictions while taking into account the temporal dynamics present in the data, thereby enhancing decision-making capabilities.
Joseph, your article on regression and ChatGPT is fascinating. Can you shed light on any strategies to handle missing data effectively when training regression models?
Hi Emily! Handling missing data effectively in regression models is crucial for reliable performance. Some strategies include: 1) Data imputation: utilizing techniques like mean imputation, regression imputation, or K-nearest neighbors imputation to fill in missing values based on other features' observed data. 2) Leveraging advanced imputation methods like multiple imputation, where missing values are imputed multiple times to capture uncertainty. 3) Considering the missingness pattern itself as a variable or employing missingness indicators if its presence carries valuable information. By leveraging suitable strategies, regression models become more robust in the presence of missing data and perform optimally within ChatGPT.
Joseph, your article on ChatGPT and regression is very insightful. How can we ensure the reliability and accuracy of regression models when dealing with imbalanced datasets?
Thank you, Peter! Ensuring reliability and accuracy of regression models with imbalanced datasets requires attention to the following aspects: 1) Correct evaluation metrics: accuracy alone may misrepresent the model's actual performance, especially when dealing with imbalanced classes. Metrics like precision, recall, or F1 score provide a more comprehensive understanding. 2) Appropriate sampling techniques: employing methods like undersampling the majority class or oversampling the minority class (e.g., SMOTE) helps balance the data distribution and prevent biased models. 3) Algorithmic considerations: adjusting the class weighting during training or utilizing algorithms with built-in handling of imbalanced data. By adopting these strategies, regression models become more reliable and accurate, even in the presence of imbalanced datasets within ChatGPT.
Joseph, your article on regression's potential with ChatGPT is captivating. Can you elaborate on any challenges in obtaining high-quality labeled data for training regression models?
Hi Laura! Obtaining high-quality labeled data for training regression models presents challenges. Some common challenges include: 1) Expert labeling: relying on domain experts or qualified annotators to ensure accurate labeling, which may be time-consuming and expensive. 2) Annotation consistency: as multiple annotators may be involved, ensuring consistent labeling quality across different annotators becomes critical. 3) Labeling costs: having a large volume of data can increase labeling costs, especially when dealing with complex tasks. Addressing these challenges requires careful planning, iterative feedback loops with annotators, and validation steps to ensure high-quality labeled data, ultimately improving the reliability and accuracy of training regression models with ChatGPT.
Joseph, your article on ChatGPT and regression is eye-opening. Can you elaborate on how regression models handle non-numeric features and convert them into meaningful representations?
Thank you, Jordan! Regression models handle non-numeric features by employing appropriate techniques to convert them into meaningful numerical representations. Common approaches include: 1) One-hot encoding: assigning binary values to each category, creating multiple binary features representing the original non-numeric feature. 2) Label encoding: assigning numerical labels to each category, replacing non-numeric values with their corresponding labels. 3) Embeddings: learning low-dimensional representations for non-numeric features, capturing their semantic relationships and allowing regression models to effectively utilize them. By applying these techniques, regression models can transform non-numeric features into formats that facilitate meaningful computations and modeling within ChatGPT.
Joseph, your article provides valuable insights into regression and its combination with ChatGPT. Can you elaborate on any potential challenges in training regression models with limited labeled data?
Hi Sarah! Training regression models with limited labeled data can present challenges. The scarcity of labeled data reduces the model's exposure to diverse instances, potentially leading to overfitting and poor generalization. Techniques like semi-supervised learning or transfer learning can help address this challenge, leveraging either limited labeled data or pre-trained models on related tasks to improve performance. Active learning, where the model actively selects the most informative instances for labeling, can also optimize the use of labeled data. By combining suitable techniques and carefully selecting regularization methods, regression models can achieve good performance with limited labeled data when integrated with ChatGPT.
Joseph, your article on regression in ChatGPT is enlightening. Can you explain how regression models handle skewed target variables and any potential considerations to ensure accurate predictions?
Thank you, Tyler! Regression models can handle skewed target variables through appropriate transformation techniques. When faced with positively skewed targets, logarithmic transformations or power transformations like Box-Cox can normalize the distribution, aiding in accurate predictions. For negatively skewed targets, inverse transformations or root transformations can be suitable. Choosing the right transformation involves considering the data distribution and desired model behavior. Additionally, techniques like weighted regression can balance the model's learning to account for skewed targets. By applying these considerations, regression models can effectively handle skewed target variables and make accurate predictions within ChatGPT.
Joseph, your article is highly informative. While regression has its advantages, are there any scenarios where alternative machine learning techniques might be more suitable in combination with ChatGPT?
Hi Lauren! Regression shines in several scenarios, but alternative machine learning techniques might be more suitable in certain situations when combined with ChatGPT. For example, for image-related regression tasks, convolutional neural networks (CNNs) or deep learning architectures like transformers might offer better performance. In cases where capturing complex non-linear relationships is critical, techniques like decision trees, ensemble methods (e.g., random forests, gradient boosting), or neural networks might outperform regression. Moreover, if temporal dependencies play a crucial role, recurrent neural networks (RNNs) or temporal convolutional networks (TCNs) could be more appropriate. Assessing task requirements, dataset characteristics, and model performance can guide the integration of alternative techniques in combination with ChatGPT.
Thank you all for reading my article! I'm excited to discuss the potential impact of ChatGPT.
Great article, Joseph! ChatGPT indeed has the potential to revolutionize technology by enhancing conversational AI systems.
I agree, Emily. The advancements in language generation are impressive. It opens up new possibilities in various fields.
ChatGPT's regression feature seems promising. It allows fine-grained control over the responses. Do you think it can aid in addressing biases in AI?
Absolutely, Sophie. By using regression, we can continuously improve the outputs of AI systems and reduce biases. It's a step in the right direction.
While ChatGPT is impressive, we should also be cautious. Bias mitigation and ethical concerns should be given equal importance.
I completely agree, David. Ethical considerations shouldn't be overlooked in the pursuit of technological advancements.
The regression feature could be useful not only for biases but also for improving the overall quality of responses.
Leah, you're right. It gives developers more control and allows them to shape the AI's behavior.
I'm curious about the training process of ChatGPT. Joseph, could you shed some light on that?
Sure, Natalie! ChatGPT is trained using Reinforcement Learning from Human Feedback (RLHF), with an initial model trained using supervised fine-tuning.
For reinforcement learning, a reward model is built by collecting comparison data. The model is then fine-tuned using Proximal Policy Optimization.
That's interesting, Joseph. How do you ensure the model's responses aren't modified maliciously during reinforcement learning?
During RL training, safety mitigations and a strong moderation system are in place to prevent malicious behavior. User feedback is highly valued to address any concerns.
Joseph, can ChatGPT be fine-tuned for domain-specific applications? For instance, in customer support or legal services?
Absolutely, Daniel. Fine-tuning allows tailoring ChatGPT to specific use cases. It can be trained on custom datasets, making it adaptable to domain-specific applications.
That's fantastic. It brings flexibility and scalability to AI adoption in various industries.
I'm excited to see how ChatGPT could revolutionize human-computer interaction. It could enhance virtual assistants and chatbots.
However, we should keep the limitations in mind. ChatGPT still tends to produce incorrect or nonsensical answers in certain situations.
That's a valid point, Thomas. ChatGPT's limitations are crucial to consider, especially in safety-critical applications.
I agree, Josephine. While the technology is impressive, it's important to set realistic expectations and use it responsibly.
I'm curious about the responsiveness of ChatGPT. How does it handle user queries in real-time scenarios?
Great question, Hannah. ChatGPT aims to respond reasonably quickly, but there might be delays or occasionally prolonged thinking times, especially for complex queries.
Could ChatGPT's regression feature be used to counteract adversarial attacks or misleading inputs?
While regression offers some control, it's primarily designed to reduce biases and align with human values. Defending against adversarial attacks requires additional measures.
I appreciate the transparency, Joseph. It's crucial to understand the limitations to responsibly deploy AI systems.
Indeed, Sophie. Transparency and responsible deployment go hand in hand when it comes to AI advancements.
I'm curious about the feedback process. As an AI language model, how does ChatGPT incorporate user feedback to improve?
User feedback is extremely valuable, Leah. OpenAI collects feedback through the user interface and actively works on addressing the limitations and mistakes through regular model updates.
Joseph, do you foresee any challenges while scaling up ChatGPT to be accessible to a larger user base?
Scaling up ChatGPT indeed poses challenges, Paul. Addressing user demand while ensuring quality, safety, and accessibility at scale remains a top priority.
OpenAI is actively researching and refining deployment strategies to make ChatGPT more widely accessible while managing potential risks.
Joseph, can ChatGPT be used to generate code snippets or technical documentation?
ChatGPT has the potential to assist in code-related tasks, Emma. While it's not specifically trained for such purposes, it can generate code snippets or provide insights to help with technical documentation.
The applications of ChatGPT seem endless. Do you think it will eventually replace human interactions in certain domains?
Oliver, while ChatGPT can augment human interactions, it's unlikely to comprehensively replace them. Instead, it can enhance productivity and provide valuable support in various domains.
That's a reassuring perspective, Joseph. Collaboration between humans and AI can lead to improved outcomes rather than complete replacement.
The potential of ChatGPT is exciting, but ongoing improvements and user feedback will be pivotal in refining its capabilities.
I'm glad to see OpenAI actively seeking user feedback. It ensures inclusivity and diverse perspectives in the development process.
ChatGPT can have significant societal implications. It's crucial for developers and researchers to consider the potential ethical and societal impact while iterating on the technology.
Agreed, Josephine. Ethical considerations shouldn't be an afterthought but rather a foundation for responsible AI development.
It's refreshing to see OpenAI's commitment to transparency, safety, and user feedback in the development of novel AI systems like ChatGPT.
Indeed, David. By involving the community and continuously iterating, we can collectively shape the future of AI in a positive way.
Joseph, thank you for sharing insights into the potential of ChatGPT. I'm excited to see how it transforms various industries.
This discussion has been insightful. I appreciate everyone's perspectives and the opportunity to engage in these conversations.
Likewise, Leah. It's been stimulating to explore the possibilities and considerations around ChatGPT's advancements.
Thank you, Joseph, for initiating this discussion. Understanding the potential and limitations of ChatGPT is essential for its responsible usage.
Agreed, Olivia. It's been a thought-provoking conversation, and I look forward to witnessing how ChatGPT evolves.
Thank you, everyone, for sharing your thoughts. This discussion has been enlightening, and it highlights the importance of responsible AI development.
Thank you all for the engaging discussion! Your insights and perspectives contribute to shaping the future of AI in a responsible and inclusive manner.