Unlocking Advanced Insights: Leveraging ChatGPT for Data Analysis in Flight Test Technology
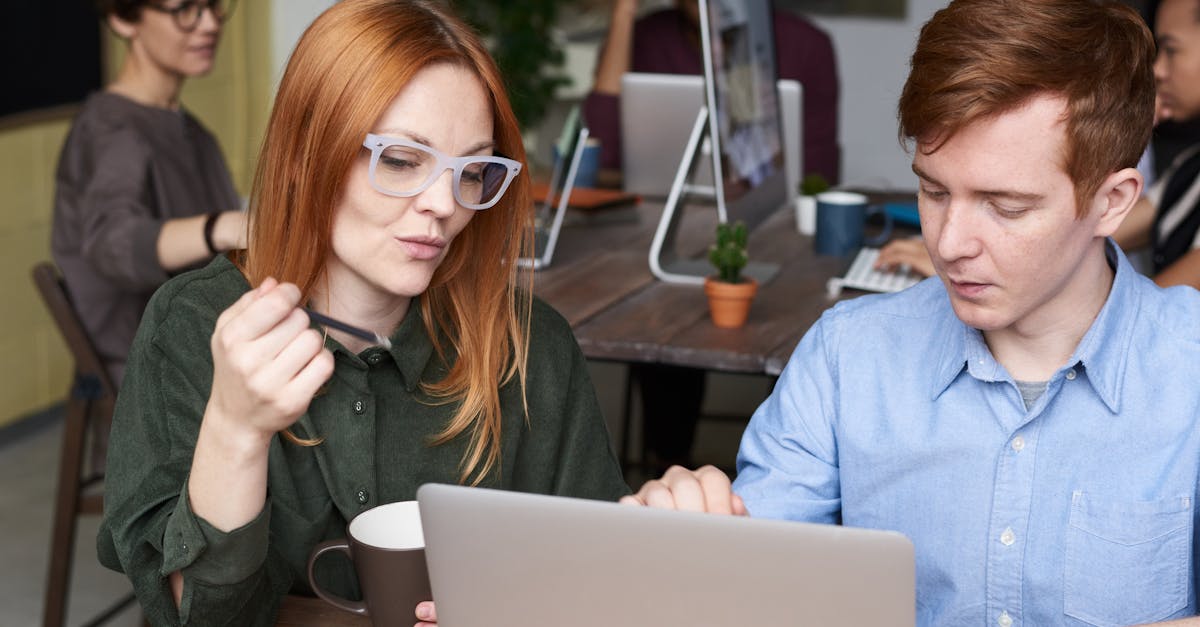
Flight test data analysis plays a crucial role in aviation industry. It helps in evaluating the performance of aircraft components, assessing the impact of design modifications, and understanding the behavior of the aircraft under different operating conditions. With the advancements in technology, flight test data analysis has become even more efficient and accurate.
One technology that has revolutionized flight test data analysis is machine learning. Machine learning algorithms can be trained to analyze flight test data, providing quick and accurate interpretations. These algorithms can process large volumes of data, extract relevant patterns, and identify anomalies or deviations from expected behavior.
Machine learning models are trained on historical flight test data, which includes information such as aircraft performance parameters, sensor readings, and environmental conditions. By learning from this data, the models can make predictions and perform analysis on new flight test data.
The usage of machine learning in flight test data analysis has several benefits. Firstly, it significantly reduces the time required to analyze complex data sets. Traditional manual analysis methods can be time-consuming and prone to errors. With machine learning, the analysis process is automated, saving both time and resources.
Secondly, machine learning can provide more accurate interpretations of flight test data. The algorithms are designed to identify subtle patterns and correlations that may not be apparent to human analysts. By leveraging the power of machine learning, aviation engineers can gain deeper insights into the performance of aircraft systems.
Moreover, machine learning algorithms can adapt and improve over time. The more data they are exposed to, the better they become at making predictions and identifying complex relationships within the flight test data. This continuous learning process enhances the accuracy and reliability of the analysis results.
Flight test data analysis using machine learning technology can be applied in various scenarios. For example, it can be used to identify potential issues or anomalies in real-time flight data, enabling timely interventions and preventive measures. It can also be used to optimize aircraft performance by analyzing the impact of different design configurations or operational parameters.
In conclusion, flight test data analysis has been greatly enhanced by the application of technology, particularly machine learning. The ability of machine learning algorithms to efficiently process large volumes of data and provide quick and accurate interpretations has revolutionized the field. With its usage, aviation engineers can gain valuable insights into the performance of aircraft systems, enabling them to make informed decisions and improve overall aviation safety and efficiency.
Comments:
Thank you all for joining this discussion on leveraging ChatGPT for data analysis in flight test technology. I'm excited to hear your thoughts!
Great article, Melissa! It's fascinating to see how AI can assist in data analysis. I think ChatGPT has the potential to revolutionize the field of flight test technology.
I agree, Michael. ChatGPT offers new possibilities for advanced insights in flight tests. It can help analyze complex data sets more effectively.
Michael, could you explain how ChatGPT can improve predictive analysis in flight test technology?
Certainly, Emily! ChatGPT can analyze historical flight test data, learn patterns, and make predictions based on similar previous scenarios. It can help identify potential issues earlier and provide insights for improved decision-making during flight tests.
Indeed, AI has come a long way. Melissa, could you share more about the specific applications of ChatGPT in flight test technology?
Sure, James! ChatGPT can assist in data preprocessing tasks, predictive analysis, anomaly detection, and even help optimize flight test parameters. It has the potential to improve efficiency and enhance safety.
Melissa, thank you for sharing the applications of ChatGPT in flight test technology. It's interesting to see how AI is transforming the field.
Melissa, is there a learning curve associated with using ChatGPT for flight test analysis? Will it require additional training for analysts?
James, there is a learning curve involved in using ChatGPT effectively. Analysts may need some additional training and guidance to maximize its potential and ensure accurate results.
Melissa, it might be helpful to provide resources or training opportunities for analysts to facilitate the adoption of ChatGPT in flight test analysis.
Melissa, incorporating ChatGPT's optimization suggestions can lead to more effective flight tests and improve overall aircraft performance, right?
What are the limitations of using ChatGPT? Can it handle large and complex flight test data sets?
That's a good question, Michelle. ChatGPT is powerful, but it does have limitations. Handling large and complex data sets can be challenging. It might require fine-tuning and careful consideration of the model's limitations.
Melissa, what are the risks involved in fine-tuning ChatGPT for flight test data analysis?
Michelle, fine-tuning ChatGPT comes with the risk of overfitting or bias if not done carefully. It requires diverse and representative training data, proper validation, and ongoing monitoring to mitigate these risks.
Thank you, Melissa. It's important to consider biases and maintain the integrity of the analysis when working with AI models like ChatGPT.
I'm impressed with the potential of ChatGPT. But what are the privacy and security concerns when using AI in flight test data analysis?
Excellent point, David. Privacy and security are of utmost importance. When using AI for flight test data analysis, it's crucial to ensure proper data anonymization, secure access controls, and compliance with relevant regulations.
Melissa, what steps can be taken to ensure compliance with privacy regulations while using AI in flight test data analysis?
David, to ensure compliance, flight test organizations can implement data anonymization techniques, encrypt sensitive data, establish access controls based on roles and responsibilities, and regularly conduct privacy impact assessments to identify and address potential risks.
Thank you, Melissa. These are indeed essential steps to safeguard privacy and data security in flight test data analysis.
Melissa, I appreciate your insights on ensuring compliance while utilizing AI in flight test data analysis. It's crucial to prioritize privacy and security in such applications.
Privacy and security considerations cannot be overlooked when utilizing AI in sensitive domains like flight test analysis. Thanks for addressing that, Melissa.
Is ChatGPT widely adopted in the field? How extensively has it been tested in real-world flight test scenarios?
Jessica, while ChatGPT shows promise, it's still a relatively new technology. The field is actively researching and testing its capabilities in real-world flight test scenarios to validate its effectiveness and performance.
Michael, do you think ChatGPT can help in detecting anomalies during flight tests? How accurate is it?
Sarah, ChatGPT can assist in anomaly detection, but its accuracy is subject to the quality and diversity of training data. Fine-tuning the model specifically for flight test anomalies is crucial to improve its accuracy.
Thanks, Michael! That could significantly improve flight test efficiency and identify potential issues before they become critical.
Do you think ChatGPT can be integrated with other existing flight test analysis tools?
Absolutely, Sophia! ChatGPT can be integrated with other tools and software used in flight test analysis. By leveraging its natural language processing abilities, it can enhance data exploration, visualization, and collaborative analysis.
Can ChatGPT help optimize flight test parameters? How does it handle the complexity of multiple variables?
Sarah, ChatGPT can assist in optimizing flight test parameters by analyzing data and suggesting parameter combinations to achieve desired outcomes. It handles multiple variables by learning patterns and correlations from historical data, but careful validation is necessary to ensure effective optimization.
Melissa, how do you foresee the future adoption of ChatGPT in flight test technology? Will it become a standard tool?
Michael, while the adoption of ChatGPT will depend on further research and development, I believe it has the potential to become a standard tool in flight test technology, complementing existing analysis techniques and offering valuable insights.
I agree, Melissa. ChatGPT has the potential to augment the capabilities of flight test analysts and become an indispensable tool for analyzing complex data.
Michael, it's important to ensure the training data for ChatGPT includes diverse and realistic anomalies to improve detection accuracy.
Thank you, Michael. I'm curious to see how it progresses and if it becomes widely adopted in the near future.
Michael, that sounds very promising. It has the potential to save time and resources in flight test analysis.
Absolutely, Emily. Time savings and improved efficiency are some of the key benefits AI can bring to flight test technology.
Michael, are there any potential risks involved in relying heavily on AI for flight test analysis?
Sarah, over-reliance on AI without proper validation or human oversight can pose risks. It's crucial to maintain a balance and understand the limitations of AI models like ChatGPT.
Michael, what are the computational requirements for implementing ChatGPT in flight test analysis? Will it be feasible for organizations with limited resources?
James, implementing ChatGPT might require significant computational resources, especially for large-scale flight test data analysis. It could pose challenges for organizations with limited resources, but as technology evolves, more efficient implementations can be expected.
Melissa, by optimizing flight test parameters, do you mean it can help find the optimal combinations for better test results?
Exactly, Sarah! ChatGPT can help identify optimal parameter combinations to achieve desired test results. It aids in making informed decisions during flight tests and improves overall test effectiveness.
Melissa, what challenges do you foresee in the widespread adoption of ChatGPT in flight test analysis?
Sarah, there are challenges such as data availability, model interpretability, and integration with existing workflows. Addressing these challenges will be crucial for the successful adoption of ChatGPT in flight test analysis.
I agree, Melissa. Overcoming these challenges will be vital to harness the full potential of ChatGPT in the field of flight test technology.
Melissa, validation plays a crucial role in optimizing flight test parameters with ChatGPT. It's important to ensure the suggested combinations align with the test objectives.