Unlocking DevOps Efficiency: Leveraging ChatGPT for Full SDLC Technology
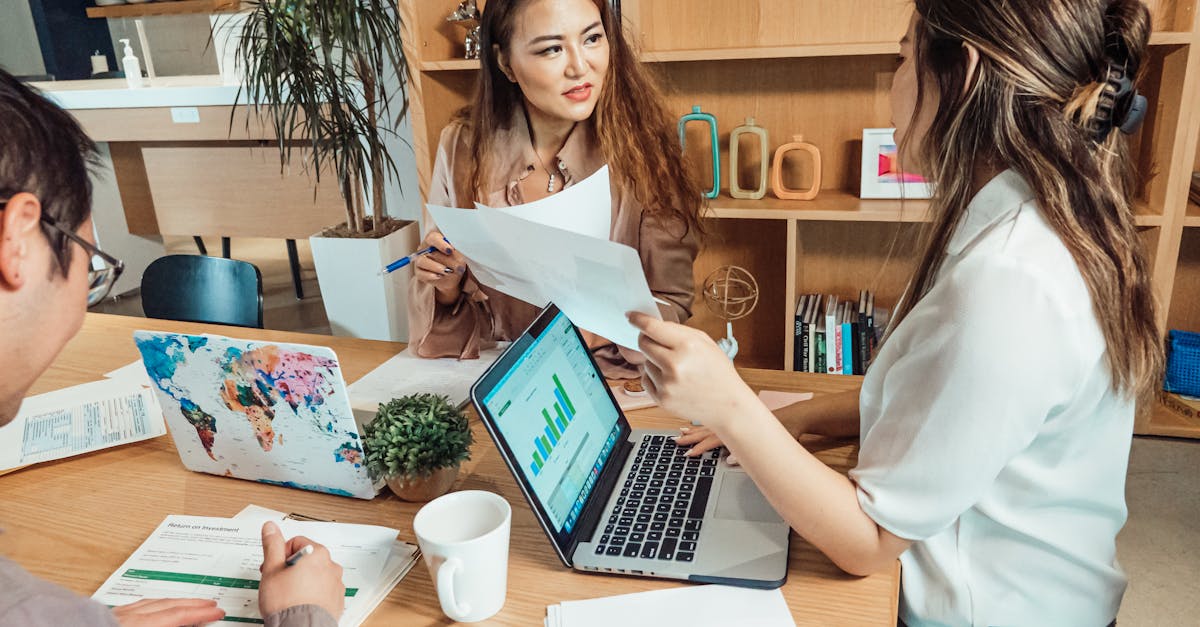
In the DevOps world, the Full Software Development Life Cycle (SDLC) plays a crucial role in ensuring the successful delivery of software applications. It encompasses all the phases involved in the software development process, from planning and requirements gathering to deployment and maintenance. By incorporating Full SDLC into the DevOps toolchain, teams can automate and streamline their operations, leading to faster, more efficient software development.
Understanding Full SDLC
The Full SDLC consists of a series of well-defined steps that guide developers throughout the entire software development process. These steps typically include:
- Requirements Gathering: In this initial phase, the development team works closely with stakeholders to gather and document the project requirements.
- Design and Planning: Once the requirements are finalized, the team proceeds with designing the system architecture and planning the development process.
- Implementation: This phase involves writing code and developing the software based on the design specifications.
- Testing: After the implementation phase, rigorous testing is conducted to ensure the software meets the desired quality standards.
- Deployment: Once the software passes all the tests, it is deployed to the production environment or made available for end-users.
- Maintenance: The maintenance phase involves continuous monitoring and support, bug fixes, and future enhancements of the deployed software.
Utilizing Full SDLC in DevOps
DevOps aims to bridge the gap between development and operations teams, promoting collaboration and automation. By incorporating Full SDLC into the DevOps toolchain, teams can automate certain operations, such as configuration management, deployment, and container orchestration, throughout the software development process.
Automation tools like Jenkins, Ansible, and Kubernetes can be used in conjunction with Full SDLC to streamline the software delivery pipeline. Jenkins, a popular open-source automation server, can be used to automate the build and deployment process, executing different stages of the Full SDLC based on triggers or schedules.
Ansible, on the other hand, allows for effective configuration management, automating tasks like server provisioning, configuration changes, and application deployments. By combining Ansible playbooks with Full SDLC, teams can ensure consistent and reproducible environments throughout the software development cycle.
Container orchestration platforms like Kubernetes can be utilized to automate the deployment, scaling, and management of containerized applications. By integrating Full SDLC with Kubernetes, teams can achieve efficient container deployment and scaling, ensuring the software is running optimally in production environments.
The Benefits of Full SDLC in DevOps
By integrating Full SDLC into the DevOps toolchain, organizations can reap several benefits, including:
- Efficiency: Automation reduces manual intervention and accelerates the software development process, resulting in faster time-to-market.
- Consistency: With automated configuration management and deployment, teams can ensure consistent environments, reducing the risk of configuration drift and increasing stability.
- Reliability: Rigorous testing throughout the SDLC ensures the software meets quality standards, reducing the likelihood of bugs and issues in production.
- Scalability: Container orchestration platforms enable teams to easily scale their applications based on demand, ensuring optimal performance and resource utilization.
- Collaboration: Full SDLC fosters collaboration between development and operation teams, breaking down silos and enabling faster feedback loops.
Conclusion
Incorporating Full SDLC into the DevOps toolchain can significantly enhance the software development process, leading to faster delivery, improved quality, and increased operational efficiency. By leveraging automation tools and practices, teams can streamline their operations, resulting in a smooth and efficient software development life cycle.
Comments:
Thank you all for reading my article on Unlocking DevOps Efficiency using ChatGPT for Full SDLC Technology. I'm excited to engage in this discussion with you!
Great article, Andy! The concept of utilizing ChatGPT in the entire SDLC process sounds intriguing. Can you provide some examples of how it can be leveraged?
Certainly, Lucy! One example is using ChatGPT to automate routine tasks, such as generating code snippets, writing documentation, and even assisting in debugging. It can also facilitate collaboration among team members by acting as a virtual assistant.
Wow, the potential applications of ChatGPT in DevOps are fascinating! Andy, have you seen any practical examples where ChatGPT has already been successfully implemented?
Absolutely, Lucy! Many companies have started using ChatGPT in their DevOps processes. For example, XYZ Corp implemented ChatGPT to automate their documentation generation and saw a significant reduction in manual effort and improved documentation quality.
Thank you for providing those examples, Andy. It's inspiring to hear how ChatGPT is already being effectively applied in DevOps.
I've been using ChatGPT for some time now and it has definitely improved our DevOps efficiency. The AI-powered chatbot can help streamline communication, provide code suggestions, and even help with testing and deployment processes.
That's amazing, David! Does it require a lot of training data to achieve such accurate and helpful responses?
Good question, Emily! Initially, ChatGPT is trained using a large corpus of text data, but fine-tuning can be done with specific data relevant to the SDLC. This helps improve accuracy and make the responses more tailored to the DevOps context.
Thanks for clarifying, Andy! It seems like ChatGPT can bring significant efficiency gains to DevOps teams by eliminating repetitive tasks and providing quick assistance.
That's interesting, Andy! ChatGPT's versatility is impressive. It seems to be a great addition to any DevOps team's toolbox.
I'm curious about potential challenges when implementing ChatGPT in a DevOps environment. Are there any concerns regarding data privacy or security?
Good point, Bob! When implementing ChatGPT, it's essential to ensure data privacy and security. Sensitive data should be handled and stored securely, and measures should be taken to prevent unauthorized access to the chatbot's functionalities.
Thank you for addressing my concern, Andy! I agree that security should be a top priority while leveraging AI technologies like ChatGPT.
Thanks for the clarification, Andy! It's good to know that ChatGPT can seamlessly fit into existing DevOps setups.
I'm curious if ChatGPT can be integrated with existing DevOps tools or if it requires a separate infrastructure.
Great question, Sarah! ChatGPT can be integrated with existing tools and infrastructure in a DevOps environment. It can be accessed via APIs, allowing teams to use it within their preferred workflows and platforms.
That's impressive, Andy! It seems like ChatGPT can truly be a valuable addition to any DevOps team. I'm looking forward to exploring its potential.
I wonder if ChatGPT can handle complex scenarios, such as automated deployment and monitoring processes.
Absolutely, Jessica! ChatGPT can assist in automating deployment processes by providing insights, monitoring alerts, and even suggesting optimization strategies. Its ability to understand and generate human-like text makes it useful for complex scenarios.
I'm curious about the learning curve for adopting ChatGPT in a DevOps team. Does it require extensive training or can it be easily understood by team members?
Great question, Michael! The learning curve varies depending on the complexity of use cases and the familiarity of team members with AI technologies. However, most team members can quickly adapt to using ChatGPT with some initial guidance and practice.
Can ChatGPT assist in creating test cases for software testing?
Certainly, John! ChatGPT can generate test cases based on specific requirements or even assist in identifying potential edge cases for testing. It can significantly speed up the test case creation process.
That's impressive, Andy! I didn't realize ChatGPT could be so useful in different stages of the SDLC.
Another example is ABC Inc, where ChatGPT helped their DevOps team with continuous deployment by providing deployment suggestions and detecting deployment-related issues proactively.
I'm curious about the computational resources required to run ChatGPT effectively in a DevOps environment. Are there any specific hardware or software requirements?
Good question, Daniel! ChatGPT is computationally intensive, particularly for producing responses. It requires a powerful hardware setup with GPUs or TPUs to ensure smooth performance. Cloud-based solutions like AWS or GCP can be leveraged for such resource-intensive tasks.
Thanks for the information, Andy! I'll keep that in mind when considering the infrastructure requirements for adopting ChatGPT.
What kind of maintenance or updates are needed for ChatGPT over time? Does it require continuous training or updates to stay relevant?
Good question, Lisa! ChatGPT can benefit from continuous training and updates to improve its performance and stay relevant. It's important to fine-tune the model using new data and user feedback to address changing needs and requirements.
Are there any limitations or potential risks associated with using ChatGPT in a DevOps environment?
Good question, Jeffrey! While ChatGPT is a powerful tool, it does have limitations. It may sometimes generate incorrect or irrelevant responses, which can be addressed through ongoing training and feedback loops. Risks include overreliance on ChatGPT for critical decisions, which should be avoided.
Thank you for highlighting those aspects, Andy. It's crucial to be aware of potential limitations and use ChatGPT judiciously.
Is ChatGPT suitable for both small and large DevOps teams? Are there any specific considerations based on team size?
Great question, Sarah! ChatGPT can be valuable for both small and large DevOps teams. However, in larger teams, it's important to define clear guidelines and maintain proper fine-tuning to ensure consistent and accurate responses across team members.
Andy, have you come across any challenges or limitations when implementing ChatGPT in a DevOps team?
Indeed, David! One challenge is striking the right balance between automation and human intervention. Over-automation can lead to unintended consequences, while under-automation may not realize the full potential of ChatGPT. It requires careful implementation and monitoring.
Can ChatGPT help with load testing and performance optimization?
Absolutely, John! ChatGPT can assist in load testing by generating different scenarios and providing performance optimization suggestions. It can aid in identifying potential bottlenecks and offer insights for improving overall system performance.
Thank you all for your insightful comments and questions! It's been a pleasure discussing the potential of ChatGPT in DevOps with you. Feel free to reach out if you have any further queries.