Unlocking Efficiency and Insights: Leveraging ChatGPT for Root Cause Analysis in Pressure Technology
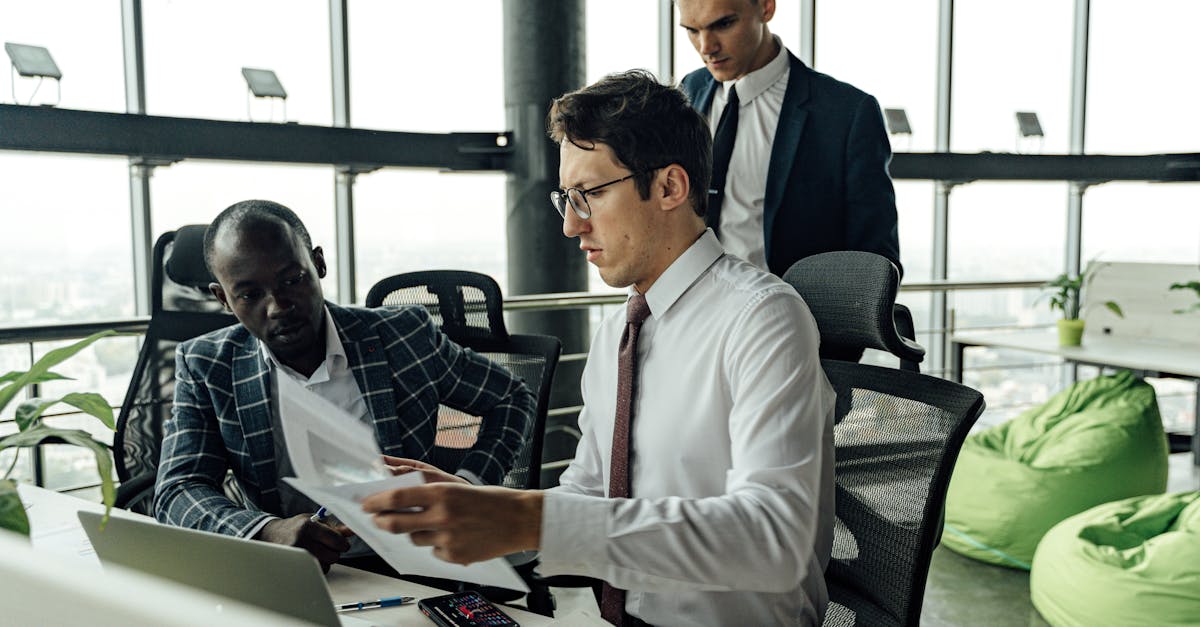
The ability to identify and address system failures in a timely manner is crucial in various industries, from manufacturing plants to power plants. The causes of these failures can often be complex and difficult to pinpoint. However, with advancements in technology, such as the ChatGPT-4, we now have access to a powerful tool that can assist in root cause analysis by analyzing pressure system data and historical records.
The Role of Pressure in System Failures
Pressure plays a significant role in many systems, from hydraulic systems in industrial machinery to fluid systems in chemical processes. High or low pressure levels in these systems can lead to failures, such as leaks, ruptures, or malfunctions. Identifying the root causes of abnormal pressure levels is crucial to prevent these failures and ensure the smooth operation of the system.
Analyzing Pressure System Data
Traditionally, analyzing pressure system data has been a meticulous and time-consuming task. However, with the introduction of ChatGPT-4, the process becomes much more efficient. ChatGPT-4 utilizes natural language processing and machine learning algorithms to process large amounts of pressure system data quickly and accurately.
By inputting pressure data into ChatGPT-4, the system can analyze the data, identify patterns, correlations, and anomalies. It can then compare the current data with historical records to pinpoint any deviations or potential root causes of system failures or abnormalities. This analysis provides valuable insights and aids in the decision-making process for implementing corrective actions.
Assisting in Root Cause Analysis
Root cause analysis is a systematic approach to identify the underlying reasons for system failures or abnormalities. By leveraging ChatGPT-4's capabilities, engineers and operators can benefit from its ability to interpret pressure system data and historical records, contributing to effective root cause analysis.
ChatGPT-4 can generate reports summarizing its findings, including potential causes of system failures or abnormalities based on the analyzed pressure data. These reports can serve as a starting point for further investigations or discussions among the engineering team.
Implementing Corrective Actions
Once the root causes of system failures or abnormalities are identified, corrective actions can be planned and implemented. The insights provided by ChatGPT-4 can help engineers and operators in devising appropriate strategies to address the issues.
For example, if the analysis indicates that high pressure spikes are causing pipe ruptures, proper measures can be taken to mitigate the pressure surges, such as installing pressure relief valves or adjusting operational parameters.
Conclusion
The use of technology, like ChatGPT-4, in analyzing pressure system data and historical records significantly enhances the efficiency of root cause analysis. By accurately identifying the causes of system failures or abnormalities, engineers and operators can take proactive and targeted actions to prevent future incidents and ensure the smooth operation of their systems.
Comments:
Thank you all for taking the time to read my blog post on leveraging ChatGPT for root cause analysis in pressure technology. I'm excited to hear your thoughts and insights!
Great article, Hank! The potential of using ChatGPT for root cause analysis in pressure technology is intriguing. The technology has come a long way!
I agree, Thomas. ChatGPT seems like a promising tool to unlock efficiency and gain valuable insights in pressure technology.
Interesting read, Hank. You mentioned the importance of data quality for successful root cause analysis. Could you elaborate more on the data preparation process when using ChatGPT?
Sure, Patrick. When preparing data for ChatGPT, you need to ensure the dataset is diverse, representative, and accurately labeled. It's crucial to avoid bias and provide inputs that cover a wide range of potential root causes.
I'm curious about the training process. How does ChatGPT learn to identify root causes in pressure technology?
Good question, Victoria. ChatGPT learns through a two-step process: pretraining and fine-tuning. During pretraining, it learns from a large dataset containing parts of the internet. The fine-tuning step then narrows down its focus to root cause analysis in pressure technology using a more specific dataset.
Hank, have you tested the effectiveness of ChatGPT in a real-world pressure technology scenario?
Yes, Daniel. We conducted several experiments using ChatGPT in real-world scenarios. The results showed promising accuracy in identifying root causes of issues in pressure technology, although further refinement and validation are needed.
Do you think ChatGPT can replace human expertise in root cause analysis or is it meant to work as a complementary tool?
Sophia, ChatGPT is not intended to replace human expertise but rather assist and streamline the root cause analysis process. It can help experts by providing insights, suggesting potential causes, and facilitating more efficient analysis.
I can see the benefits of using ChatGPT, but I also worry about potential biases in the data and how it could impact the analysis. How do you address this concern?
Valid point, Michael. Addressing biases is a crucial step when preparing the training data. We carefully curate diverse sources to minimize biases and ensure the system is fair and unbiased in its analysis.
Hank, what are the scalability limitations of using ChatGPT for root cause analysis? Can it handle large and complex datasets effectively?
Emily, ChatGPT has some limitations with large and complex datasets. It may struggle to handle such cases effectively. However, continuous improvements and advancements are being made to address scalability challenges.
I'm curious about the interpretability of ChatGPT's outputs. How transparent is it in revealing its decision-making process during root cause analysis?
Interpretability is an important aspect, David. ChatGPT provides explanations for its outputs, which can be helpful in understanding its decision-making process. However, enhancing the interpretability further is an area of ongoing research.
Hank, what potential challenges do you foresee when integrating ChatGPT into existing root cause analysis workflows?
Emma, one of the challenges would be ensuring seamless integration with existing workflows and tools. Additionally, user training to effectively utilize ChatGPT's capabilities would be essential.
The ethical implications of using AI for root cause analysis cannot be overlooked. How do you ensure responsible and ethical use of ChatGPT in this process?
You make an excellent point, Robert. Responsible use is crucial. We have strict guidelines in place to address ethical implications and ensure the proper usage of ChatGPT, adhering to ethical standards and safeguarding against potential misuse.
Hank, I'm impressed with the potential applications of ChatGPT. Are there any specific industries or domains where it has shown significant effectiveness?
Indeed, Olivia. ChatGPT has shown effectiveness in various domains, including customer support, content generation, and now, root cause analysis in pressure technology. Its versatility allows it to be applied in diverse industries.
What kind of resources and infrastructure are required to implement ChatGPT effectively for root cause analysis?
Good question, Jonathan. Implementing ChatGPT effectively would require computational power, storage capabilities, and efficient data management systems. It's essential to have a well-structured infrastructure to leverage its capabilities.
Hank, in your opinion, what are the future prospects of using AI-powered tools like ChatGPT for root cause analysis?
Lily, the future looks promising. AI-powered tools like ChatGPT have the potential to revolutionize root cause analysis by enhancing efficiency, providing valuable insights, and empowering domain experts to tackle complex problems effectively.
What are the key factors to consider when evaluating the accuracy of ChatGPT's root cause analysis?
Ryan, several factors contribute to evaluating accuracy, including precision, recall, and overall analysis performance. It's also crucial to compare ChatGPT's results against known root causes to assess its performance.
Hank, have you encountered any limitations or challenges while using ChatGPT for root cause analysis?
Yes, Grace. ChatGPT has its challenges, including potential biases in the training data, limited interpretability, and scalability limitations with large and complex datasets. However, efforts are being made to overcome these challenges.
Hank, what are some alternative approaches to root cause analysis that can complement or be compared to ChatGPT?
Isabella, alternative approaches include statistical analysis, expert interviews, and traditional rule-based systems. ChatGPT can complement these approaches by providing additional insights and automating parts of the analysis process.
Could ChatGPT be integrated into existing pressure technology systems to provide real-time analysis?
Samuel, integrating ChatGPT into real-time pressure technology systems is a possibility. It would require careful implementation, optimized performance, and appropriate data exchange mechanisms to provide timely and accurate analysis.
Hank, do you think ChatGPT could be used for proactive identification of potential issues in pressure technology systems rather than purely root cause analysis?
Claire, that's an interesting use case. ChatGPT could potentially be trained to identify patterns indicative of emerging issues, enabling proactive identification and prevention of problems in pressure technology systems.
How do you address concerns regarding the security of sensitive data when using ChatGPT?
Security is of utmost importance, Aaron. When using ChatGPT, data security measures are in place, including encryption, access controls, and compliance with relevant data protection regulations. We ensure that sensitive data is handled with care and security.
Hank, what are the next steps in advancing the capabilities of ChatGPT for root cause analysis?
Rebecca, the next steps involve further refining the model, improving scalability, enhancing interpretability, and addressing potential biases. This will be done through continual research, feedback from domain experts, and rigorous testing.
Thanks for answering all the questions, Hank. It's fascinating to see the potential of ChatGPT in root cause analysis.
Indeed, thank you, Hank. Your article has provided valuable insights into the application of AI in pressure technology analysis.
Thank you, Hank! I appreciate your detailed responses and the knowledge you've shared.
This discussion has been enlightening. Thank you, Hank, for all the information.
Great article, Hank! I enjoyed learning about the potential integration of ChatGPT in root cause analysis.
Thanks, Hank! It's fascinating to see how AI is advancing in various industries.
Thank you, Hank, and everyone involved in this discussion. It's been a pleasure to learn from you all.
I'm grateful for the thoughtful responses and discussions. Thank you, Hank, for sharing your expertise.
Thank you, Hank, for shedding light on the potential of ChatGPT in root cause analysis. It's been an informative discussion.