Unlocking Efficiency and Minimizing Commodity Risk: Leveraging ChatGPT in Logistics Management
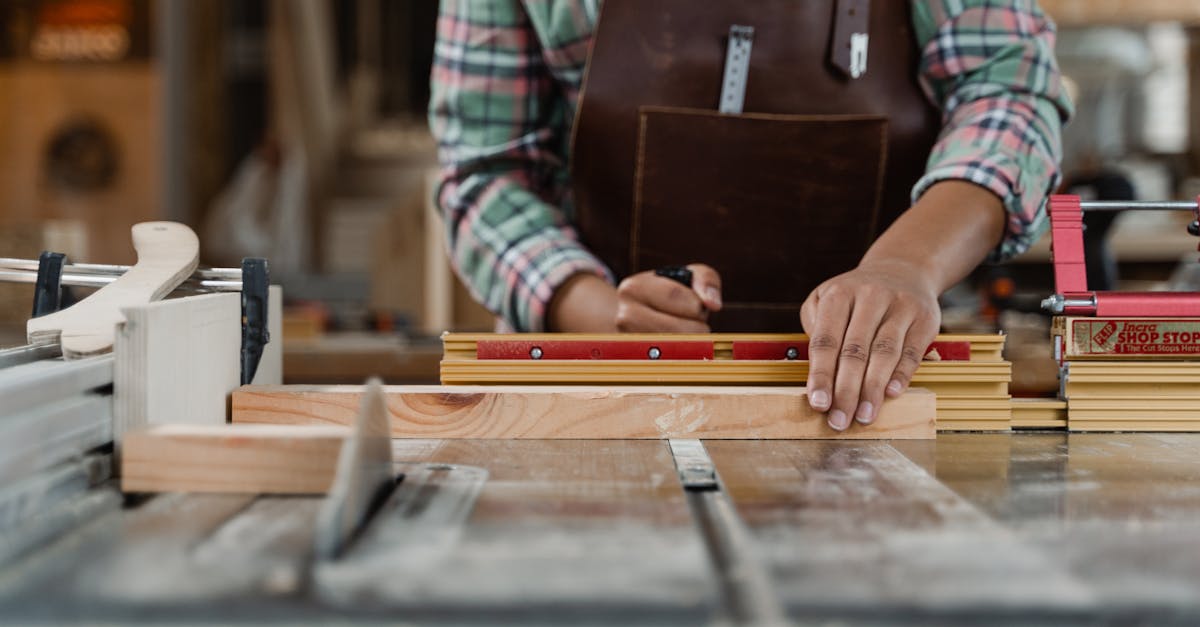
Commodity risk management is a critical aspect of logistics management. It involves assessing and addressing risks associated with the transportation and distribution of commodities, such as price volatility, supply chain disruptions, regulatory changes, and natural disasters. These risks can have a significant impact on businesses, leading to financial losses, operational inefficiencies, and damaged customer relationships.
Advancements in artificial intelligence (AI) have revolutionized the logistics industry, offering new opportunities for effective risk management. By harnessing the power of AI, businesses can optimize their logistics management processes to mitigate commodity-related risks and enhance overall supply chain performance.
Using AI for Commodity Risk Management
AI technologies, such as machine learning algorithms and predictive analytics, enable businesses to gain valuable insights from vast amounts of data. This data can include historical commodity prices, weather patterns, global market trends, transportation routes, and supplier performance metrics.
Machine learning algorithms can analyze historical data to identify patterns and predict future price movements. This information can help businesses make informed decisions regarding buying, selling, and storing commodities. By accurately forecasting commodity prices, businesses can minimize financial risks and optimize their procurement and inventory management processes.
Predictive analytics can also be used to assess risks associated with supply chain disruptions. By analyzing historical data on transportation routes, delivery times, and carrier performance, businesses can identify potential bottlenecks and delays. This information allows them to proactively plan alternative routes, secure backup carriers, or adjust delivery schedules to minimize the impact of disruptions.
Enhancing Supply Chain Visibility and Efficiency
AI technologies can also provide businesses with enhanced visibility and transparency across their supply chains. Real-time tracking and monitoring systems powered by AI can provide accurate and immediate updates on the location and condition of commodities during transportation.
By leveraging AI-powered tracking systems, businesses can quickly identify and address any abnormalities or potential risks in the supply chain. This proactive approach not only minimizes the chances of theft, damage, or loss but also allows businesses to take immediate actions to mitigate the impact of any such incidents.
AI can optimize route planning and carrier selection, ensuring efficient transportation and distribution of commodities. Algorithms can analyze various factors, such as transportation costs, delivery times, carrier reliability, and capacity constraints, to recommend the most optimized routes and carriers for each shipment. This enables businesses to reduce transportation costs, improve on-time delivery, and minimize the likelihood of bottlenecks or delays.
Conclusion
Commodity risk management is an essential component of logistics management. With the help of AI technologies, businesses can mitigate risks associated with the transportation and distribution of commodities. By using machine learning algorithms and predictive analytics, businesses can make informed decisions, minimize financial risks, and optimize their supply chain performance. Additionally, AI-powered tracking systems enhance supply chain visibility and efficiency by providing real-time updates on the location and condition of commodities. This enables businesses to proactively address any potential risks and ensure smooth and secure transportation and distribution processes.
As businesses continue to embrace AI technology, logistics management will become more efficient and effective in mitigating commodity-related risks. By leveraging AI's capabilities, businesses can stay competitive in a rapidly evolving global market and ensure the successful transportation and distribution of commodities.
Comments:
Thank you all for taking the time to read my article on leveraging ChatGPT in logistics management. I'm curious to hear your thoughts and opinions on this topic.
Great article, Ely! Leveraging AI in logistics management can be a game-changer. It has the potential to streamline operations, reduce costs, and enhance overall efficiency in the supply chain.
Thank you, Samantha! I completely agree. AI-powered tools like ChatGPT can analyze large volumes of data, optimize routes, and help minimize delays. It's exciting to see how logistics can benefit from these advancements.
While AI can certainly improve efficiency, do you think there are any potential risks or challenges in implementing ChatGPT in logistics management?
That's a valid concern, Robert. One challenge is ensuring the accuracy and reliability of AI models like ChatGPT. It's essential to continuously train and update the system to avoid any potential biases or incorrect decisions.
I'm curious about the impact of ChatGPT on human jobs in the logistics industry. Can it potentially replace human workers?
Good question, Sophie. ChatGPT and similar AI tools are designed to assist human workers, not replace them. They can handle repetitive or time-consuming tasks, allowing humans to focus on more strategic and complex aspects of logistics management.
I believe leveraging AI in logistics management can also help minimize commodity risk. ChatGPT can analyze market trends, predict demand, and optimize inventory levels. This can reduce the chances of overstocking or understocking.
Absolutely, Michael. AI-enabled tools can provide valuable insights into market conditions and help companies make data-driven decisions regarding procurement and inventory management. This can lead to improved efficiency and minimized commodity risk.
One concern I have is the potential for data breaches or misuse when implementing AI in logistics management. How can these risks be addressed?
Data security is indeed crucial, Emily. When implementing ChatGPT or any AI system, organizations must prioritize data protection, implement robust security measures, and ensure compliance with privacy regulations. Regular audits and threat assessments can help address these risks proactively.
I can see how AI can enhance efficiency in logistics, but what about the initial costs of implementing such technology? Small to medium-sized logistics companies might find it challenging to adopt AI due to budget constraints.
Valid point, Lucas. Implementing AI can indeed involve initial costs, including infrastructure setup and AI system integration. However, as technology advances and becomes more accessible, the costs are likely to reduce, making AI adoption more feasible for smaller companies.
While AI can analyze data and make recommendations, it's crucial to have human oversight and decision-making. AI may not always consider external factors or unforeseen circumstances that humans can account for.
Absolutely, Oliver. Human oversight is essential to ensure that AI recommendations align with business goals and account for complex factors. AI should be seen as an augmentation tool rather than a replacement for human decision-making.
What are your thoughts on the ethical implications of AI in logistics management? For example, bias in decision-making or potential job displacements?
Ethics is a critical consideration, Melissa. It's important to train AI models with diverse and unbiased datasets to mitigate potential biases. Regarding job displacements, companies should focus on reskilling and upskilling their workforce to adapt to the evolving demands of AI-powered logistics.
I'm excited about the potential of AI in logistics management, but isn't there a risk of overreliance on AI systems? What happens if the technology fails or malfunctions?
Valid concern, Jason. While AI can bring numerous benefits, it's crucial to have backup plans and contingency measures in place. Human oversight and intervention should always be available to address any potential failures or malfunctions.
I think it's essential to strike the right balance between AI and human involvement in logistics management. Embracing AI should enhance human capabilities rather than replacing them completely.
Well said, Vanessa. The combination of AI and human involvement can lead to optimal results in logistics management. AI can provide valuable insights and automation, while humans can contribute critical thinking, creativity, and adaptability.
Are there any specific use cases or success stories you can share where ChatGPT or similar AI systems have been successfully implemented in logistics management?
Certainly, Adam. Several logistics companies have leveraged AI for tasks like demand forecasting, route optimization, and real-time tracking. This has resulted in cost savings, improved delivery times, and better customer satisfaction. One notable example is a global e-commerce giant that utilized AI-powered logistics solutions to enhance their operations.
How can smaller logistics companies start leveraging AI in their operations? What steps can they take to adopt and integrate AI?
Great question, Hannah. Smaller logistics companies can start by identifying specific areas where AI can add value, such as inventory management or route optimization. Collaboration with AI solution providers, gradual implementation, and investing in relevant training and infrastructure can help smaller companies embrace AI in a manageable and cost-effective way.
I believe a data-driven approach is crucial when implementing AI in logistics management. Accurate data collection, analysis, and interpretation contribute to better decision-making. How can companies ensure data quality and integrity?
You're absolutely right, Daniel. Maintaining data quality and integrity is vital. Companies should invest in robust data collection processes, data cleansing, and validation techniques. Regular audits and data governance practices can help ensure the reliability of data used for AI-based logistics management.
Do you have any recommendations for companies that are considering integrating ChatGPT or similar AI tools into their logistics operations?
Certainly, Grace. First, it's important to define clear objectives and identify specific use cases where AI can deliver value. Companies should also consider factors like data readiness, infrastructure requirements, and change management. Collaborating with AI solution providers and leveraging their expertise can greatly facilitate the integration process.
Can AI solutions like ChatGPT help address last-mile delivery challenges, such as traffic congestion and customer preferences?
Absolutely, Sophia. AI can analyze real-time traffic data, optimize routes, and provide insights for efficient last-mile delivery. It can also analyze customer preferences and historical data to personalize delivery experiences, improving customer satisfaction.
What are your thoughts on the future of AI in logistics management? Can we expect further advancements and innovations?
The future of AI in logistics management looks promising, Luke. As technology continues to evolve, we can expect further advancements in areas like predictive analytics, autonomous vehicles, and cognitive automation. AI will play a vital role in shaping the logistics industry and driving innovation.
While AI can offer many benefits, how can companies ensure transparency and maintain the trust of customers and stakeholders when implementing AI-driven logistics management?
Transparency is indeed crucial, Natalie. Companies should be open about their adoption of AI and clearly communicate the purpose and benefits of its implementation. They should also address any concerns regarding data privacy, bias, or job displacement. Engaging stakeholders and seeking their feedback can help build trust and ensure transparency throughout the process.
What are some of the potential limitations or challenges of using ChatGPT or similar AI systems in logistics management?
Good question, Aiden. One limitation is the dependency on data quality and availability. AI systems like ChatGPT require large amounts of relevant data for accurate analysis and decision-making. Another challenge is interpretability, as AI models may not provide explicit reasoning behind their recommendations. Continuous monitoring and improvement of AI systems can help address these challenges.
To what extent can AI in logistics management contribute to sustainability and environmental impact reduction?
AI can significantly contribute to sustainability in logistics, Max. By optimizing routes and reducing empty miles, AI can minimize fuel consumption and carbon emissions. It can also facilitate intelligent resource allocation, leading to reduced waste and a more sustainable supply chain.
What are the key factors that companies should consider when selecting an AI solution for logistics management? Any specific criteria?
Companies should consider factors like the system's accuracy, scalability, integration capabilities, and compatibility with existing infrastructure. Additionally, evaluating the vendor's reputation, support, and track record can help ensure the selection of a reliable AI solution for logistics management.
How can logistics companies navigate the ethical challenges associated with AI, such as potential bias or discrimination in decision-making?
Logistics companies can address ethical challenges by continuously monitoring and auditing AI systems for biases. Diverse and representative datasets should be used during the training process to minimize discrimination. It's also important to have clear policies and guidelines in place to ensure fair decision-making and accountability.
How can AI-powered logistics management systems adapt to unforeseen disruptions, such as natural disasters or unforeseen events?
Adaptability is crucial, Liam. AI-powered logistics management systems should be designed to quickly respond to unforeseen disruptions. Real-time data analysis and predictive capabilities can help in identifying alternative routes, reallocating resources, and ensuring a rapid response to minimize the impact of such events.
Are there any legal or regulatory considerations that need to be considered when implementing AI in logistics management?
Absolutely, Sophia. Logistics companies should consider legal and regulatory frameworks related to data privacy, security, and AI usage. Compliance with regulations, such as GDPR or industry-specific guidelines, is crucial to ensure ethical and responsible AI implementation.
Is there a risk of companies relying too heavily on AI in logistics management and neglecting human expertise and judgment?
Balancing AI and human expertise is key, Jack. Companies must recognize the value of human judgment, adaptability, and critical thinking. AI should be used as a tool to augment human capabilities, fostering collaboration and leveraging the strengths of both AI and human workers.
Thank you all for your valuable comments and insights. It has been a great discussion. Keep embracing technological advancements in logistics management, and don't hesitate to adapt and explore the potential of AI. Feel free to reach out if you have any further questions or thoughts.