Unlocking Efficiency and Scalability: Leveraging ChatGPT for Multi-tasking and High-volume Product Recommendations
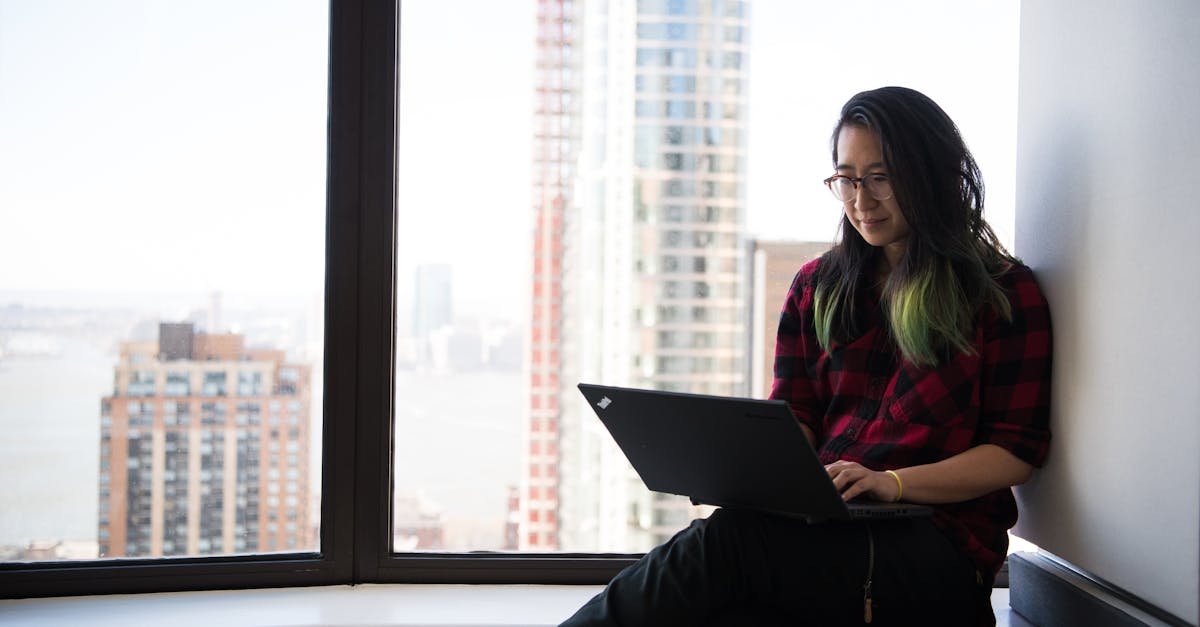
Technology: Multi-task & Handle High-volume Workloads
ChatGPT-4 is an advanced AI language model powered by state-of-the-art technology that allows it to efficiently multi-task and handle high-volume workloads. Built upon deep learning algorithms, ChatGPT-4 excels in understanding and generating human-like text, making it ideal for a wide range of applications.
Area: Product Recommendations
One of the key areas where ChatGPT-4 shines is in providing personalized product recommendations. With the ability to analyze user data and preferences, ChatGPT-4 can offer tailored suggestions to users, helping them discover products or services that align with their interests and needs.
Usage: ChatGPT-4 for Personalized Recommendations
By leveraging the power of ChatGPT-4, businesses can enhance their recommendation systems and deliver a more personalized experience to their customers. Whether it's an online retail platform, a streaming service, or a travel agency, ChatGPT-4 can effectively analyze user data, such as past purchases, browsing history, and feedback, to generate accurate and targeted recommendations.
The usage of ChatGPT-4 for personalized product recommendations goes beyond simple automated suggestions. It can engage in interactive conversations with users, understanding their preferences, and providing real-time recommendations based on individual contexts. This human-like interaction creates a more natural and intuitive user experience, increasing customer satisfaction and boosting conversions.
Additionally, ChatGPT-4's ability to handle high-volume workloads enables it to cater to a large number of users simultaneously. This scalability ensures that businesses can offer personalized recommendations to their entire user base, no matter the size, without compromising the quality or speed of the recommendations delivered.
Conclusion
With its multi-tasking capabilities and high-volume workload handling, ChatGPT-4 is a game-changer in the field of personalized product recommendations. By integrating this advanced AI language model into recommendation systems, businesses can unlock new levels of customization and provide users with more relevant and engaging suggestions. As technology continues to advance, ChatGPT-4 represents a significant step towards creating seamless and personalized user experiences across various industries.
Comments:
Thank you all for joining this discussion! I'm excited to hear your thoughts on leveraging ChatGPT for multi-tasking and high-volume product recommendations.
The article highlights some interesting points about using ChatGPT for efficiency and scalability. I wonder how well it performs in real-world scenarios. Has anyone here tried implementing it?
I haven't personally implemented ChatGPT for high-volume product recommendations, but I've used it for other tasks. It has shown great potential for natural language understanding and generating responses.
I have implemented ChatGPT for a customer support scenario, and it worked quite well. The model was able to handle various inquiries and provide helpful responses.
Scalability is crucial when dealing with high-volume product recommendations. I'm curious to know if ChatGPT has any limitations in terms of handling large datasets.
ChatGPT's performance can degrade with larger datasets due to the model's attention limitations. However, techniques like batching and truncation can mitigate this issue to some extent.
One of the challenges in multi-tasking with ChatGPT is ensuring that the model maintains good performance across different tasks. Has anyone encountered any difficulties in that regard?
I've faced some difficulties when using ChatGPT for both text generation and summarization tasks simultaneously. The model struggled to consistently produce high-quality summaries while generating responses.
It's fascinating how ChatGPT can handle multi-turn conversations. I'd love to hear about any use cases where it has been successfully applied in real-world applications.
I've seen ChatGPT being used in customer service chatbots. It can effectively engage in meaningful conversations and resolve user queries, enhancing the overall user experience.
I've noticed that ChatGPT's performance in multi-tasking varies depending on the complexity and similarity of the tasks. Handling diverse tasks simultaneously can sometimes lead to inconsistent output quality.
Apart from scalability and multi-tasking, how reliable is ChatGPT for accurate product recommendations? Is it able to understand user preferences and tailor recommendations accordingly?
ChatGPT can capture user preferences to a certain extent, but it might not always provide highly personalized recommendations. Additional techniques like collaborative filtering can be used to enhance personalization.
Considering the potential of ChatGPT, do you think it can completely replace traditional recommendation systems, or are there specific scenarios where it may not be suitable?
ChatGPT's strength lies in its conversational abilities, but it may not completely replace traditional recommendation systems. Domain-specific models tailored for specific industries might be more effective in certain cases.
I can see ChatGPT being valuable in scenarios where personalized recommendations and interactive conversations are important. It can provide a more engaging and dynamic user experience.
Thank you all for your valuable insights! It is clear that ChatGPT has its advantages, especially in multi-tasking and conversational contexts. However, it's important to consider its limitations and use it in conjunction with other techniques for optimal results.
I'm curious about the training process for ChatGPT. Can anyone shed some light on the data requirements and training methodology involved?
Training ChatGPT requires a large dataset that covers diverse conversational scenarios. It uses a version of Reinforcement Learning from Human Feedback (RLHF) with data from human AI trainers to fine-tune the model.
To create a dataset for training, a prompt is given to AI trainers who then have conversations playing both the user and the AI assistant. This dialogue data is used to train the model through a two-step process.
That's interesting! Could you elaborate on the two-step process of training ChatGPT using the dialogue data?
In the first step, supervised fine-tuning, the model is trained on the dialogue dataset with human AI trainers providing responses. In the second step, reinforcement learning, the model is fine-tuned using comparison data and an RL training process.
During reinforcement learning, the model generates responses that are compared, ranked, and used to guide further training. This iterative process helps improve the model's performance over time.
The training process involves several iterations of fine-tuning using Proximal Policy Optimization, where the model interacts with the dialogue dataset and learns to generate more coherent and contextually relevant responses.
As ChatGPT learns from human AI trainers, is there a risk of biases being introduced into the model's responses? How is this addressed?
OpenAI takes the issue of biases seriously. They strive to reduce both glaring and subtle biases in ChatGPT's responses. They invest in research and engineering to improve the robustness of the fine-tuning process and actively address biases.
I have a question regarding response generation. Does ChatGPT provide any control over the style or persona of the AI assistant's responses?
ChatGPT doesn't have a built-in control mechanism for style or persona. However, you can modify the user prompt to specify the desired style and encourage the AI assistant to respond in a specific manner.
Are there any privacy concerns when using ChatGPT, given the nature of the conversations it engages in?
Privacy is indeed a concern. ChatGPT conversations are used for training and improving the model, but OpenAI has strict policies in place to anonymize and minimize exposure of personal information during this process.
In terms of pricing, how does ChatGPT's usage compare to other OpenAI APIs?
The pricing details can be found on OpenAI's website. ChatGPT API has separate pricing compared to other OpenAI APIs like Language, Translation, or Summarization.
Has anyone encountered any specific challenges or limitations when integrating ChatGPT into their applications?
One challenge I faced was managing response latency when handling high volumes of concurrent requests. Ensuring efficient integration and handling spikes in traffic were key to overcoming this limitation.
Another challenge is handling user input errors or ambiguous queries. ChatGPT's responses might not always provide the desired clarifications or prompt the user for more specific information, leading to suboptimal outcomes.
Your comments have provided valuable insights into various aspects of leveraging ChatGPT. Let's continue the discussion and address any other questions or concerns you may have.
Could ChatGPT be used for generating other types of recommendations beyond products, such as content recommendations or personalized suggestions?
Yes, ChatGPT can be adapted for generating content recommendations or providing personalized suggestions in various domains. Its versatility makes it suitable for a wide range of recommendation tasks.
Given ChatGPT's capabilities, do you think it has the potential to revolutionize the way we interact with AI assistants and recommendation engines?
ChatGPT certainly represents a significant step forward in conversational AI and recommendation systems. However, complete revolution would require further advancements and integration with industry-specific models.
I'm excited to see how ChatGPT evolves and becomes more accessible to developers. The potential to create interactive and intelligent applications is truly promising!
Thanks to everyone who participated in this discussion. Hearing different perspectives and experiences has been enlightening. Let's stay connected and continue exploring the potential of ChatGPT!