Unlocking Error Insights: Enhancing FPLC Technology with ChatGPT
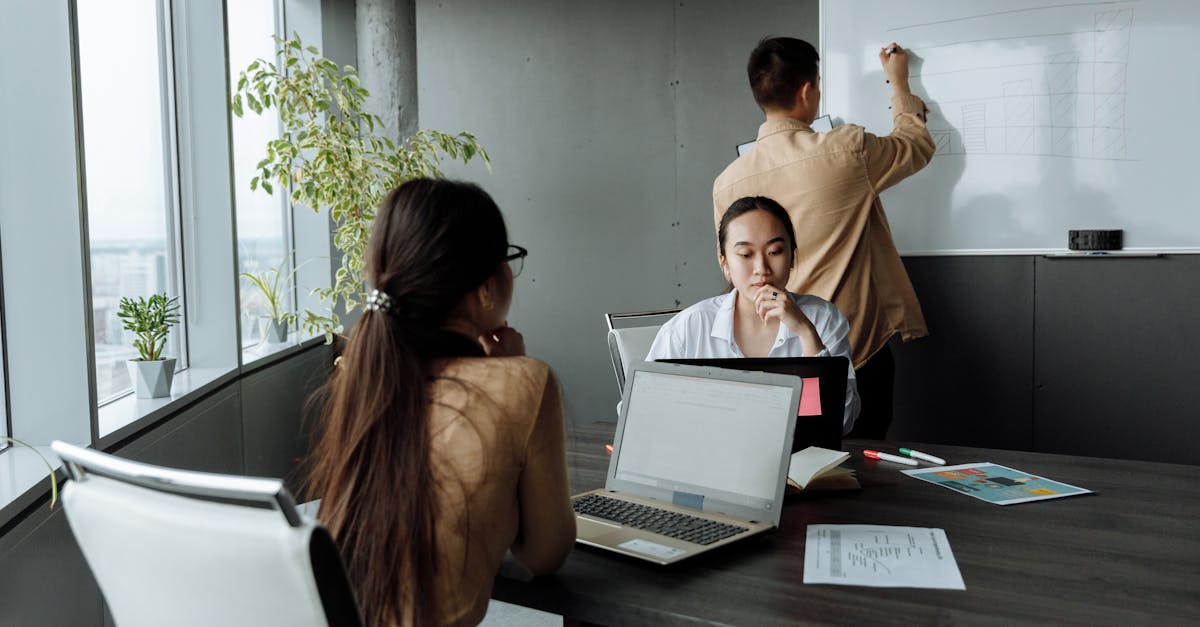
Fast Protein Liquid Chromatography (FPLC) is a powerful analytical technique used in biochemistry and protein purification. However, like any complex system, FPLC instruments can sometimes encounter errors and troubleshooting these issues can be time-consuming and frustrating. This is where Artificial Intelligence (AI) comes into play.
AI technology has undergone significant advancements in recent years, making it a valuable tool in many scientific disciplines. When it comes to FPLC, AI can be trained to provide insights into errors detected by FPLC systems, helping to reduce the time required to troubleshoot issues.
The main area where AI proves its usefulness in FPLC is error insight. FPLC systems are equipped with various sensors and detectors that monitor different aspects of the chromatographic process. These sensors generate a plethora of data, including pressure, flow rates, UV absorbance, and conductivity, among others.
Traditionally, troubleshooting FPLC errors involved manually analyzing these data streams and comparing them to known error patterns. This process is not only tedious but also prone to human error. AI-powered error insight eliminates these inefficiencies by automatically analyzing the data and applying machine learning algorithms to detect patterns that correlate with different errors.
The usage of AI in FPLC error insight has numerous benefits. Firstly, it reduces the time required to identify and resolve errors. Instead of spending hours manually sifting through data, scientists and technicians can rely on AI algorithms to quickly pinpoint the root cause of the error.
Secondly, AI can learn from previous error instances and continuously improve its ability to detect and diagnose errors accurately. This self-learning capability ensures that the AI system becomes more proficient over time, leading to more efficient troubleshooting and minimizing errors in FPLC experiments.
Furthermore, the availability of error insights provided by AI can also aid in preventive maintenance. By analyzing error patterns and identifying potential issues before they escalate, maintenance tasks can be scheduled in advance, reducing downtime and optimizing FPLC instrument performance.
With the increasing prevalence of AI in various scientific fields, it is no surprise that FPLC error insight is gaining traction. Laboratories and research facilities that rely heavily on FPLC systems can greatly benefit from AI-powered troubleshooting, enabling them to focus more on their scientific pursuits rather than spending valuable time on error detection and resolution.
In conclusion, the application of AI in FPLC error insight is a game-changer. By swiftly identifying and diagnosing errors, AI can significantly reduce the time and effort required for troubleshooting FPLC systems. Its self-learning capabilities and potential for preventive maintenance make it an invaluable tool in the field of biochemistry and protein purification.
Comments:
Thank you all for your interest in my article on enhancing FPLC technology with ChatGPT! I'm excited to hear your thoughts.
Great article, Kyle! I've been using FPLC for years, and the idea of combining it with ChatGPT sounds intriguing. Can you please explain how exactly it enhances the technology?
Hi Jessica! ChatGPT enhances FPLC technology by providing real-time error insights. It can monitor the system, analyze potential errors, and provide recommendations to optimize the chromatography process. It essentially acts as a virtual assistant for troubleshooting and improving the efficiency of FPLC runs.
I'm impressed, Kyle! This combination could save a lot of time and effort in the lab. Do you have any real-world examples of how ChatGPT has helped identify errors?
Absolutely, Daniel! In one instance, ChatGPT detected a clogging issue in the chromatography column and recommended adjusting the flow rate accordingly. This prevented a costly column replacement. In another case, it identified an improper buffer preparation that was affecting the separation. ChatGPT suggested the correct buffer concentration and helped achieve better results.
This technology sounds promising! Are there any limitations or challenges associated with using ChatGPT for FPLC troubleshooting?
Good question, Maria. While ChatGPT is a powerful tool, it relies on accurate data input for optimal performance. If the system is not calibrated or if incorrect information is provided, the error insights may not be reliable. Additionally, it's important to note that ChatGPT is not a replacement for expert knowledge but rather a supportive tool to assist in the troubleshooting process.
Kyle, I appreciate the potential of ChatGPT in FPLC. Are there any plans to integrate this technology into FPLC systems as a built-in feature?
Thanks, Emily! There is indeed ongoing research on integrating ChatGPT directly into FPLC systems. The aim is to make it a seamless component that can be accessed through the system's interface. This would enable real-time error detection and recommendations without the need for external devices or software.
Kyle, great job on this article! I am curious about the implementation process of ChatGPT with FPLC. Is it a complex setup?
Thank you, James! The setup process is relatively straightforward. ChatGPT is integrated into the FPLC system's software through APIs. Configuration involves training the model on relevant chromatography data and setting up the error detection parameters. Once set up, ChatGPT continuously monitors the system and provides insights when potential errors occur.
Kyle, do you have any plans to extend the use of ChatGPT to other chromatography techniques, such as HPLC or GC?
Hi Sarah! While the focus of this article is on FPLC, the concept of integrating ChatGPT can certainly be applied to other chromatography techniques. Each technique would require some customization due to differences in operation, but the underlying principle of real-time error insights remains the same. There is potential for broader application in the future.
Kyle, I'm intrigued by the potential cost savings this integration can bring. Have you conducted any cost analyses comparing FPLC with and without ChatGPT?
Great point, Robert! Preliminary cost analyses indicate that using ChatGPT in FPLC can lead to significant savings. By avoiding errors that would require column replacements, optimizing buffer usage, and reducing troubleshooting time, laboratories can potentially save both money and resources. However, further comprehensive studies are needed to quantify these savings accurately.
Kyle, as someone unfamiliar with FPLC, I found your article very informative. Can you provide a brief overview of how FPLC works?
Certainly, Linda! FPLC, which stands for Fast Protein Liquid Chromatography, is a technique used to separate, purify, and analyze proteins and biomolecules. It involves the passage of a sample through a column packed with a matrix that selectively interacts with different molecules based on their characteristics. Through controlled flow rates and buffer compositions, proteins can be separated and collected for further analysis.
Kyle, congratulations on the article! Are there any plans to develop a user-friendly interface for ChatGPT to make it accessible to a wider range of users?
Thank you, Andrew! Ease of use is indeed a priority. The aim is to develop a user-friendly interface that doesn't require extensive technical knowledge. Researchers and laboratory personnel should be able to interact with ChatGPT intuitively, allowing them to make the most out of its error insights without being hindered by complex interfaces.
Kyle, your article has sparked my interest in FPLC technology. Are there any resources you would recommend for someone who wants to learn more about it?
I'm glad to hear that, Sophia! There are several excellent resources available to learn more about FPLC. 'Principles and Methods of Protein Purification' by Richard Burgess is a comprehensive book on protein purification techniques, including FPLC. Additionally, websites like ResearchGate and PubMed offer numerous scientific articles and papers on the subject.
Fantastic article, Kyle! I have a question regarding the integration of ChatGPT into existing FPLC systems. Can it be retrofitted to older models or is it primarily for new systems?
Thank you, Olivia! ChatGPT integration is not limited to new systems. With proper integration support, it can be retrofitted to existing FPLC models, making it a viable option for laboratories that already have established systems. This allows broader adoption and utilization across the scientific community.
Kyle, I appreciate the practicality of combining FPLC with ChatGPT. Is it compatible with all FPLC column types and sizes?
Hi Michael! ChatGPT can be used with various FPLC column types and sizes, as long as the system is properly calibrated and the training data includes relevant information for those specific columns. Compatibility and optimal performance can be ensured by tailoring the model's training to the specific chromatography setup being used.
Kyle, your article sheds light on an exciting integration. Do you foresee any challenges in terms of system compatibility when integrating ChatGPT into FPLC?
Good question, Mark. System compatibility can be a challenge when integrating ChatGPT into FPLC, especially considering different manufacturers and models. However, with standardization efforts and collaboration between software developers and FPLC manufacturers, compatibility challenges can be overcome. It requires a collective effort to ensure ChatGPT's integration becomes widely accessible across various FPLC systems.
Kyle, this integration could be a game-changer in the field of chromatography! Are there any plans to expand the error insights beyond just FPLC troubleshooting?
Absolutely, Sophie! While the focus currently lies on FPLC, the potential for expanding error insights to other areas of chromatography is recognized. By developing models and training data specific to other techniques like HPLC or GC, similar real-time error insights can be provided for various chromatographic processes. The future holds exciting possibilities!
Kyle, I found your article fascinating! In terms of learning and adaptation, how does ChatGPT improve its error detection accuracy over time?
Thank you, Lucas! ChatGPT improves its error detection accuracy through continuous learning and adaptation. It utilizes feedback from users and the scientific community to refine its insights. By integrating user experiences and incorporating novel error patterns, the model keeps evolving, thereby enhancing its accuracy over time.
Kyle, your article presents an exciting convergence of technology and chromatography! What are the potential implications of implementing ChatGPT in terms of reducing experimental errors and ensuring reproducibility?
Great question, Ethan! Implementing ChatGPT can significantly reduce experimental errors by providing real-time error insights and recommendations. This ensures timely detection and resolution of issues, leading to more reproducible chromatography runs. By standardizing the troubleshooting process and optimizing experimental conditions, researchers can improve the overall reproducibility of their experiments.
Kyle, excellent article! Could ChatGPT also assist in method development for FPLC?
Thank you, Benjamin! While the primary focus of ChatGPT is error insights, it can certainly provide suggestions and guidance in method development for FPLC. By analyzing data and recommending adjustments to parameters like column choice, buffer composition, flow rates, and gradient conditions, it can aid in optimizing separations and improving method development outcomes.
Kyle, I thoroughly enjoyed your article! Are there any plans to make ChatGPT available as open-source software?
Thank you, Alexandra! While I don't have details on open-sourcing ChatGPT specifically, the broader trend in AI and machine learning suggests a growing movement towards open-source models. Making ChatGPT open-source could foster collaboration, transparency, and encourage further improvements from the scientific community. It's an area that warrants exploration.
Kyle, your article has piqued my curiosity! Does ChatGPT require an internet connection to function, or can it operate offline once set up?
Hi Sophia! Once ChatGPT is set up and the model is trained, it can operate offline without relying on an internet connection. This is beneficial for laboratories and facilities with restricted internet access or for scenarios where continuous monitoring is required, regardless of connectivity. ChatGPT's offline capability ensures uninterrupted error insights within the FPLC system.
Kyle, fascinating article! How would you suggest laboratory personnel become proficient in using ChatGPT effectively for FPLC troubleshooting?
Thank you, Liam! Developing proficiency in using ChatGPT effectively for FPLC troubleshooting requires hands-on experience and familiarity with the system. Training sessions, workshops, and interactive tutorials can help laboratory personnel understand the capabilities and limitations of ChatGPT. Practical exposure to real-world scenarios and continuous engagement with the virtual assistant would further enhance proficiency in utilizing its error insights.
Kyle, your article has the potential to revolutionize FPLC troubleshooting! Can ChatGPT also provide insights into analytical method validation?
I'm glad you think so, Hannah! While the focus of ChatGPT is currently on error insights during operation, there is potential for it to provide insights into analytical method validation as well. By analyzing data trends, identifying potential sources of error, and suggesting optimization strategies, it can offer support in achieving reliable and validated analytical methods.
Kyle, excellent work on this article! Are there any plans to incorporate machine learning techniques to make ChatGPT more adaptable and proactive in error prevention?
Thank you, David! Incorporating machine learning techniques is indeed a consideration for ChatGPT's future development. By leveraging historical data and patterns, the model can learn from past errors and suggest preventive measures. This proactive approach will enhance error prevention, further improving the efficiency and reliability of the FPLC system.
Kyle, your article highlights an exciting avenue for FPLC optimization! How can one get access to ChatGPT for FPLC integration?
Hi Ella! Access to ChatGPT for FPLC integration can be facilitated through collaborations and partnerships with software developers working on this technology. Establishing contacts with research groups, industry experts, or engaging with companies specializing in AI and machine learning for chromatography would be a good starting point to explore this avenue further.
Kyle, your article is quite insightful! Do you foresee the integration of ChatGPT with FPLC leading to advancements in other scientific fields beyond chromatography?
Thank you, Grace! While the immediate focus is on enhancing FPLC, the concept of integrating ChatGPT with other scientific fields is undoubtedly promising. The ability to provide real-time error insights and recommendations can benefit various experimental and analytical techniques. With tailored models and training, similar approaches could be explored and applied in other scientific domains.
Thank you all for the engaging discussion! Your questions and insights have been valuable. If you have any further queries or would like additional information, please don't hesitate to reach out.