Unlocking Insights: Exploring the Role of ChatGPT in Microstructure Analysis for Polymer Characterization Technology
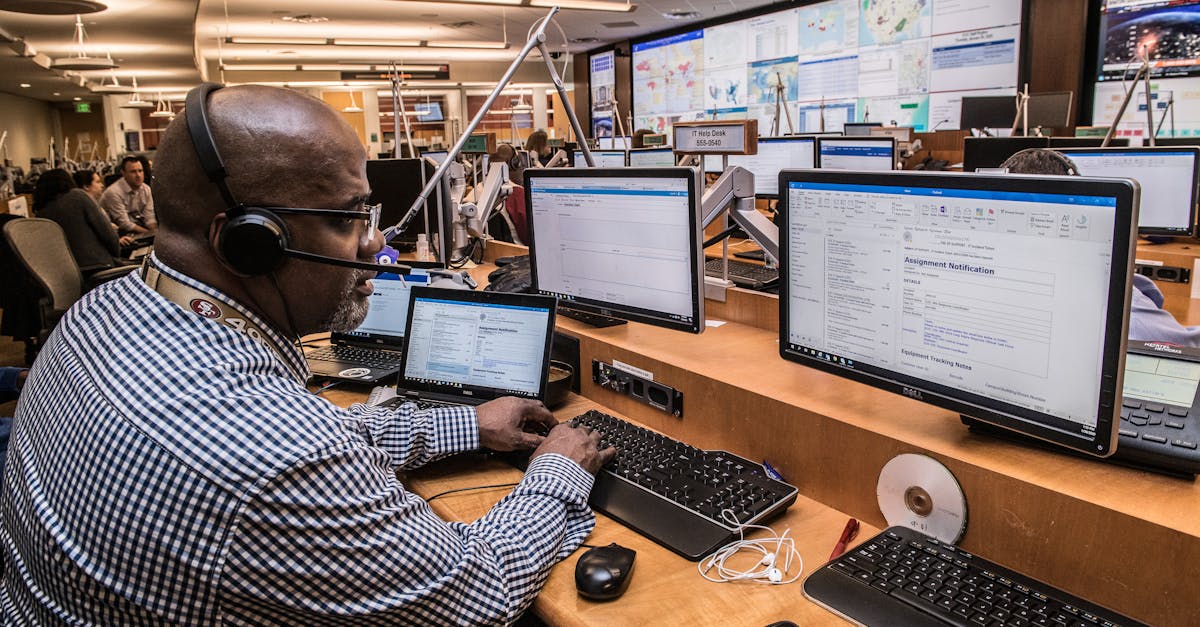
Advancements in the field of polymer characterization have significantly contributed to the development of novel materials with improved properties and performance. One crucial aspect of polymer characterization is the analysis of microstructure, which provides valuable insights into the arrangement, size, and distribution of polymer constituents at the nano- and microscale. Techniques such as Transmission Electron Microscopy (TEM) and Scanning Electron Microscopy (SEM) are commonly used for microstructural characterization. These techniques generate vast amounts of data, making interpretation challenging and time-consuming.
To address this issue, the latest language model, ChatGPT-4, can be a valuable tool in assisting with the interpretation of results obtained from microstructural characterization techniques. ChatGPT-4 is an advanced AI language model developed by OpenAI, capable of natural language processing and understanding.
Interpreting Microstructural Characterization
Polymer microstructural characterization techniques such as TEM and SEM provide detailed information about the arrangement of polymer chains, crystallinity, phase separation, and defects present within the material. However, making sense of the obtained data and extracting meaningful conclusions can be challenging due to its complexity and the need for expert knowledge in polymer science.
ChatGPT-4 can be trained on a vast amount of relevant data related to polymer science and microstructure analysis. By implementing this technology, researchers can ask specific questions about their acquired TEM or SEM images, such as the size distribution of polymer particles, degree of crystallinity, presence of defects, or composition analysis. ChatGPT-4 can then generate intelligible responses, providing valuable insights into the obtained results.
Benefits of ChatGPT-4 in Polymer Characterization
Utilizing ChatGPT-4 can offer several benefits in the field of polymer characterization:
- Accelerating Analysis: ChatGPT-4 can quickly process and analyze large amounts of data, allowing researchers to interpret microstructural results more efficiently. This enables faster decision-making and accelerates the overall research process.
- Expert Knowledge: The language model can leverage its knowledge on polymer science and microstructure analysis, providing expert-level guidance to researchers who may not have extensive expertise in the field.
- Improved Accuracy: The AI model reduces the risk of human error during interpretation and analysis, ensuring more accurate and reliable results.
- Advanced Insights: ChatGPT-4 has the potential to provide researchers with advanced insights and predictions based on the microstructural data, enabling them to make informed decisions and develop better materials.
Conclusion
Microstructural characterization plays a vital role in advancing polymer science by providing key information about the arrangement and behavior of polymer constituents. Employing advanced AI language models like ChatGPT-4 can significantly benefit researchers in interpreting the results obtained from microstructural techniques such as TEM and SEM. The ability of ChatGPT-4 to process vast amounts of data, offer expert knowledge, improve accuracy, and provide advanced insights can enhance the efficiency and effectiveness of polymer characterization studies. With the integration of these technologies, researchers can unlock new possibilities in the development of innovative polymer materials with tailored properties for various applications.
Comments:
Great article! The use of ChatGPT in microstructure analysis for polymer characterization technology seems very promising. I'm curious to know about the accuracy and reliability of the insights obtained through this approach.
Thank you, Michael! The accuracy of insights obtained through ChatGPT depends on the quality and comprehensiveness of the training data. However, it has shown promising results in microstructure analysis. Further research is still ongoing to improve accuracy and reliability.
Thank you for the response, Jesse. It's good to know that research is ongoing to improve accuracy. Are there any specific measures being taken to ensure reliability and minimize errors?
Michael, to ensure reliability, measures like training data diversity, expert validation, and continuous improvement through feedback loops are employed. Efforts are made to minimize errors by refining the training process and addressing biases.
That's reassuring, Jesse. It's essential to address and minimize biases. Have there been any validations or comparisons between ChatGPT and traditional methods to demonstrate its efficacy?
I completely agree, Michael. Combining experimental techniques with AI-powered analysis has the potential to provide a more comprehensive understanding of polymer characteristics.
Michael, there have been studies comparing ChatGPT with traditional methods, and while it shows promising results, it's important to note that it might not completely replace traditional techniques. The goal is to augment and enhance existing methods.
Thank you for sharing, Jesse. Augmenting existing methods rather than replacing them seems like a practical approach. It would be interesting to see how professionals in the field perceive the integration of ChatGPT with traditional methods.
Michael, I share the same curiosity. Hearing from experts who have experience with both traditional methods and ChatGPT integration would provide valuable insights into its efficacy and potential benefits.
Anna, I completely agree. The availability of training data can foster innovation and enable a wider range of researchers to contribute to improving the accuracy and reliability of ChatGPT for polymer analysis.
Michael, gathering feedback from professionals in the field is essential for further integration and refining of ChatGPT. Industry collaborations and academic partnerships are being explored to improve its capabilities.
That's great to hear, Jesse. Collaborations with experts, both in academia and industry, can provide valuable insights and help in steering the technology in the right direction for effective polymer analysis.
Indeed, David. Bringing together various stakeholders can lead to a holistic approach in material science research, propelling us towards innovative solutions in polymer characterization and related fields.
Partnerships and collaborations would be pivotal in harnessing the expertise from both academia and industry for the advancements of ChatGPT. Jesse, are there any specific areas where these collaborations are focused?
Michael, collaborations might focus on improving the interpretability of ChatGPT's insights for polymer analysis. The ability to explain the reasoning behind predictions might increase its reliability and acceptance.
Michael, the collaborations are primarily focused on refining the training data, improving the interpretability of ChatGPT's analysis for polymer-related domains, and exploring techniques to handle complex polymer features with higher accuracy.
Thank you for the insights, Jesse. Collaborations focused on refining training data, interpretability, and handling complex features sound promising. I'm excited to see how these partnerships shape the future of ChatGPT in polymer analysis.
Jesse, it's great to hear that interpretability is one of the focuses. Transparency in AI-powered analysis is crucial to gain trust and acceptance in critical fields such as polymer characterization.
Absolutely, David. Transparency is key, especially when the insights obtained from ChatGPT are used for important decision-making processes. I hope the future developments address this aspect effectively.
I completely agree, David. Combining the expertise of polymer scientists and materials engineers with AI developers can result in AI algorithms that truly understand the intricacies of polymer analysis.
Jane, that's an excellent point. Mutual understanding between domain experts and AI developers can lead to AI algorithms that not only perform well but also provide meaningful and relevant insights in polymer characterization.
Jesse, it's exciting to see the collaborative efforts in this field. The integration of AI analysis with polymer characterization techniques has immense potential to advance research and innovation in industries reliant on polymer-based materials.
Collaborations seem to hold the key for unlocking the full potential of ChatGPT in polymer analysis. Jesse, it's great to see the efforts being made to bring together experts from various domains.
Jesse, I believe collaborations between AI developers, polymer scientists, and materials engineers could be instrumental in refining the current algorithms and broadening the application scope of ChatGPT.
Absolutely, David. Collaborations can bridge the gap between AI expertise and domain-specific knowledge, ensuring that ChatGPT's algorithms are optimized for the unique demands of polymer characterization.
David, partnerships with materials engineering companies could also provide valuable real-world data and validation opportunities, reinforcing the practical applications of ChatGPT in polymer characterization technology.
Anna, you're right. Real-world data and industry collaboration can ensure that ChatGPT is not limited to theoretical scenarios but is tailored to handle the challenges faced in practice, making it more reliable and relevant.
Anna and David, the discussions and insights obtained through collaborations can help in refining AI algorithms, making ChatGPT a more valuable tool for not just researchers, but also industry professionals in polymer characterization.
Absolutely, Sara. The involvement of industry professionals ensures that the advancements in AI-based polymer analysis meet the practical requirements, enabling tangible benefits and improved outcomes.
Indeed, Jane. The development and adoption of AI algorithms that truly understand the intricacies of polymer analysis can revolutionize the way materials are analyzed and optimized for various applications.
Sara, I'm glad to see industry professionals involved in these collaborations. Their input is invaluable in ensuring that AI algorithms like ChatGPT align with the practical demands and challenges faced by polymer characterization experts.
Absolutely, David. The collective efforts of researchers, AI developers, and industry professionals can help bridge the gap between theoretical potential and practical applicability, making AI algorithms beneficial in real-world scenarios.
David, the involvement of materials engineering companies can also bring valuable industry data to improve the accuracy and applicability of ChatGPT for polymer analysis, ensuring its effectiveness in a commercial context.
Jesse, it's great to hear that measures are taken to enhance reliability. Are there any ongoing collaborations or partnerships to further improve ChatGPT's capabilities for polymer analysis?
Absolutely, David! The combination of AI and experimental approaches can have transformative effects in material science research. Collaboration between researchers, industries, and AI developers can drive progress in this domain.
Making the training data publicly available would be immensely helpful for researchers and enthusiasts who wish to explore and contribute to the development of ChatGPT in polymer characterization. I second that question, Jesse.
I agree, Michael. Combining the strengths of both AI and traditional methods can lead to more accurate and reliable analysis. It would be interesting to see the results obtained when they are used together.
Absolutely, Anna. Synergizing experimental data with AI analysis can provide a more comprehensive understanding of polymer characteristics, helping in further advancements.
Sara, I couldn't agree more. The combination of AI analysis and experimental techniques can lead to breakthroughs in material science and polymer characterization. Exciting times ahead!
I agree, Michael. The potential applications of ChatGPT in various fields are incredible. I wonder if it can also be used for other material analysis techniques apart from polymer characterization.
Interesting read, Jesse! How does ChatGPT compare to traditional methods of microstructure analysis in terms of speed and efficiency?
David, from what I understand, ChatGPT offers faster analysis compared to traditional methods due to the speed at which it can analyze large amounts of data. However, it's important to validate the accuracy of the results obtained through this technology.
Aiden, it's interesting to hear that ChatGPT can provide faster analysis. Validating accuracy is indeed crucial, especially in critical applications like polymer characterization. I'm excited to see the progress in this field.
Impressive article, Jesse Hertzberg. I'm wondering if there are any limitations or challenges when using ChatGPT for polymer characterization technology. It would be interesting to explore those aspects as well.
I'm also curious about the limitations, Anna. For instance, can ChatGPT accurately handle complex polymer structures with intricate features?
That's a great point, Jane. The complexity of polymer structures is definitely a challenge. Jesse, could you elaborate on how ChatGPT addresses such intricacies?
Anna, I think the challenge lies in providing ChatGPT with training data that encompasses a wide range of complex polymer structures. Training it with diverse examples might help it handle intricate features more accurately.
That makes sense, Jane. An extensive and diverse training dataset would be crucial to handle the complexities. Jesse, are there any plans to incorporate more diverse training data?
That's a valid point, Jane. Training ChatGPT with complex polymer structures might help improve its accuracy and understand the nuances of different polymer types. Jesse, are there any plans to make the training data publicly available?
I wonder if ChatGPT can be used in conjunction with experimental techniques for polymer characterization. Combining the power of AI with actual data measurements might lead to more robust analysis.