Unlocking Insights: Leveraging ChatGPT for MDX Technology in Data Analysis
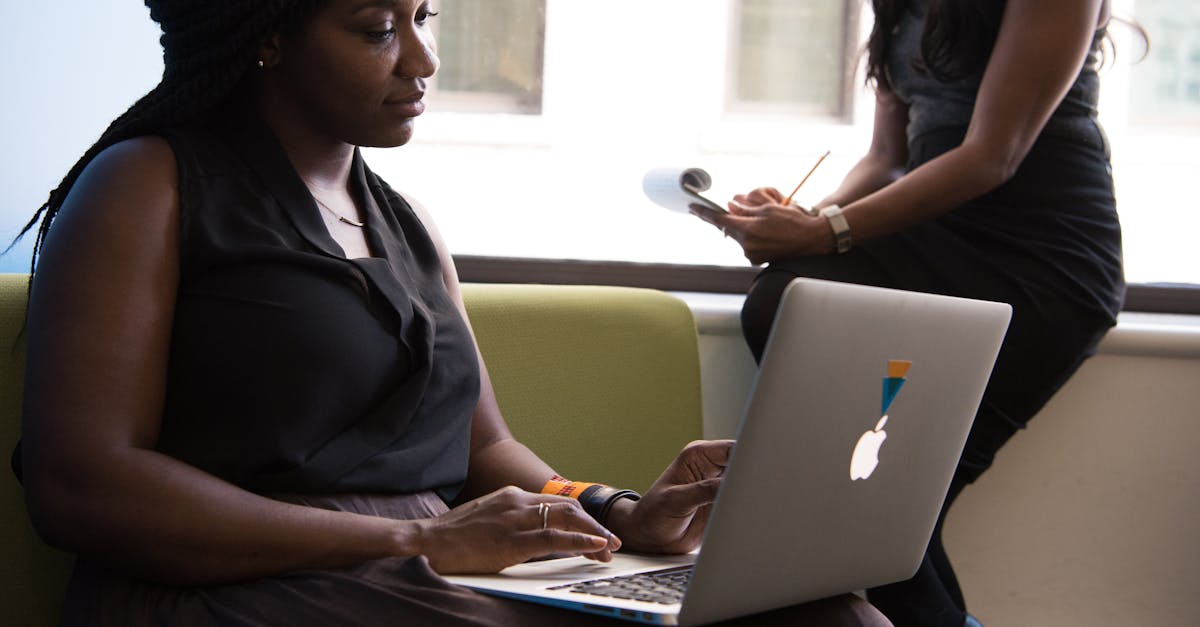
Introduction
MDX, which stands for Multi-Dimensional Expressions, is a query language designed for analyzing and manipulating data stored in multidimensional databases. It has been widely used in the field of data analysis and plays a crucial role in extracting meaningful information from large datasets.
What is MDX?
MDX is a language that enables analysts to express advanced calculations and aggregations on multidimensional data. It was developed by Microsoft in the late 1990s and has become the de facto standard for querying OLAP (Online Analytical Processing) databases.
How Does MDX Work?
MDX operates on a multidimensional database, which stores data in a structured manner that allows efficient analysis and reporting. The database is organized into dimensions, hierarchies, and measures. Dimensions represent various attributes of the data, such as time, location, or product. Hierarchies define the relationships between different levels of each dimension. Measures are the numerical data points that need to be analyzed.
MDX queries are written using a combination of functions, operators, and syntax specific to the language. Analysts can define complex calculations and aggregations, slice and dice data, and retrieve results in a structured format. The results can then be further analyzed or visualized to gain insights.
Applications of MDX
MDX is widely used in various domains that require data analysis, such as finance, sales forecasting, supply chain management, and customer segmentation. Its versatility makes it suitable for a variety of scenarios.
Data Analysis with ChatGPT-4
ChatGPT-4, the latest version of OpenAI's language model, incorporates the power of MDX for analyzing large datasets. By leveraging the capabilities of MDX, ChatGPT-4 can process and analyze massive amounts of data to extract meaningful information.
With ChatGPT-4, analysts can interactively explore and query datasets, perform complex calculations, and generate data-driven insights. The integration of MDX into ChatGPT-4's capabilities empowers users to conduct advanced data analysis without the need for specialized tools or expertise.
Conclusion
MDX is a powerful technology that enables efficient analysis and manipulation of multidimensional data. Its applications are vast and span across various industries. With the integration of MDX into ChatGPT-4's capabilities, data analysis becomes more accessible and intuitive. Analysts can harness the power of MDX to extract meaningful insights from large datasets, aiding decision-making and driving innovation.
Comments:
Great article! ChatGPT seems like a promising tool for data analysis.
I agree, Daniel. It's exciting to see how AI can enhance our analysis capabilities.
Thank you both! AI has indeed opened up new possibilities in data analysis.
Has anyone tried using ChatGPT in their data analysis projects?
I've been using it for a few months now, Emily. It's been quite helpful in generating insights.
That's interesting, David. Could you share some examples of how ChatGPT has provided valuable insights?
Sure, Daniel. One example is when I had a large dataset with missing values. ChatGPT suggested a novel imputation method that outperformed traditional approaches.
Another time was when I was exploring patterns in user behavior. ChatGPT helped me uncover hidden correlations that I hadn't considered before.
That sounds really powerful, David. I'm definitely considering giving ChatGPT a try.
I'm curious about the learning curve for ChatGPT. Is it easy to use for someone who's not very experienced in AI?
From my experience, Michael, ChatGPT has a user-friendly interface. It doesn't require deep AI knowledge to get started.
I agree with Daniel. It has a gentle learning curve, making it accessible to data analysts without extensive AI backgrounds.
That's correct. We aimed to design ChatGPT with usability in mind, so it can be readily used by professionals across different domains.
Thank you, Rene, for sharing this insightful article. It's great to see the potential of ChatGPT in data analysis.
You're welcome, Sarah! I'm glad you found it informative.
Rene, I appreciate your article. It's clear that ChatGPT has immense potential in the field of data analysis.
Thank you, Mark! I'm glad you found the potential of ChatGPT intriguing.
That's good to know. I'm excited to explore ChatGPT's potential for my own data analysis work.
I've read about ChatGPT's language limitations. Are there any specific constraints to consider when using it for data analysis?
Sarah, there are indeed limitations. ChatGPT can sometimes generate plausible but incorrect answers, so it's crucial to validate its suggestions.
Additionally, it's important to provide clear and specific instructions to avoid ambiguous or irrelevant responses.
That's a valid point, David. While ChatGPT is valuable, it's essential to exercise caution in interpreting its outputs.
Do you think ChatGPT will replace traditional statistical analysis tools in the future?
John, I don't think it will replace them entirely, but it will certainly become an invaluable complement to existing tools.
I agree with Jennifer. While ChatGPT brings a fresh perspective, traditional statistical analysis still has its place in certain scenarios.
That makes sense. It seems like incorporating AI will enhance data analysis, rather than completely replacing established methods.
I'm concerned about the ethical implications of using AI for data analysis. How can we ensure unbiased results?
Jessica, that's an important concern. It's crucial to train AI models on diverse and inclusive datasets to mitigate biases.
Additionally, continuously monitoring and auditing AI tools can help identify and address any biases that may emerge.
Thank you, Emily. Ensuring fairness and transparency in AI-based analysis is vital for responsible data practices.
Has anyone faced any challenges while using ChatGPT for data analysis?
Mark, one challenge I encountered was when ChatGPT failed to ask for additional clarifications when encountering incomplete or ambiguous queries.
That's interesting, Daniel. It highlights the importance of guiding ChatGPT effectively to optimize its performance.
Indeed, proper instructions and iterative refinement can help overcome challenges when using ChatGPT for data analysis.
How does the computational cost of using ChatGPT compare to traditional data analysis techniques?
David, using ChatGPT does come with a computational cost, especially for larger datasets. It's important to consider available resources for efficient usage.
In my experience, computational costs were manageable, but it's worth considering the trade-off between insights gained and resources required.
Absolutely, Daniel. Each project's requirements should be considered to determine if ChatGPT's benefits outweigh the costs.
I appreciate the lively discussion here. It's fascinating to explore the applications and implications of ChatGPT in data analysis!
Indeed, conversations like these help us broaden our understanding and make informed decisions about integrating AI in analysis workflows.