Unlocking Insights: Leveraging ChatGPT in Product Activity Data Analysis for EDI Technology
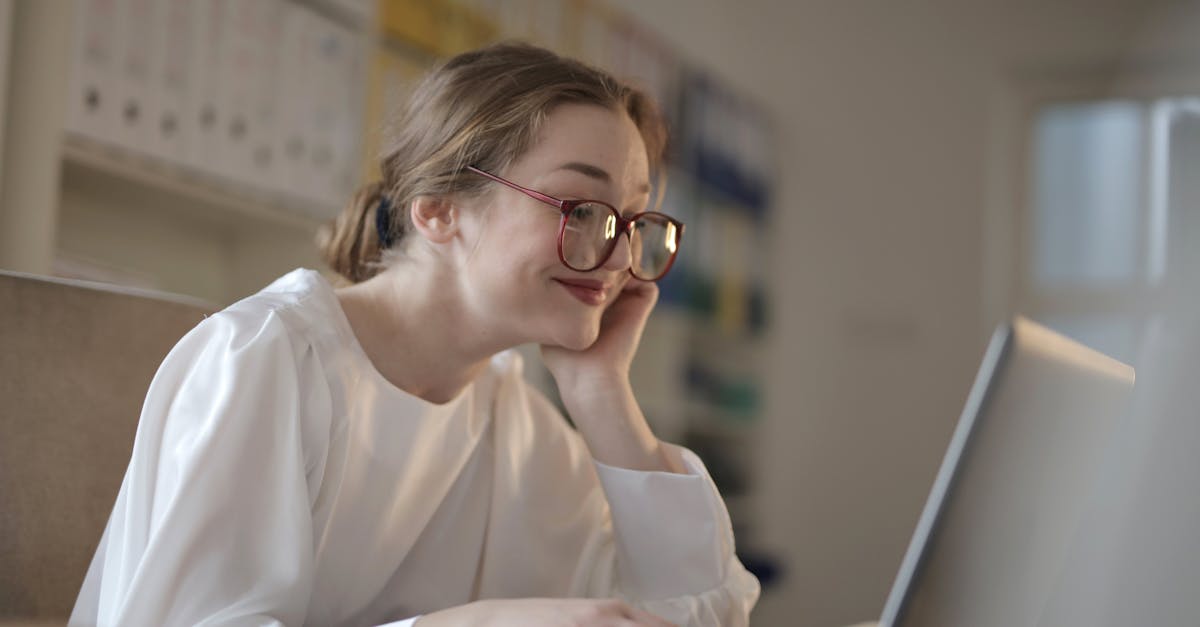
In today's fast-paced business environment, having accessible and accurate data is paramount to making informed and strategic business decisions. This is particularly true when it comes to the management of product sales and inventory data. Enter Electronic Data Interchange (EDI), a technology that allows businesses to exchange data in a standardized format electronically, eliminating the need for paperwork. The usage of this technology is prevalent across many industries, including retail, healthcare, transportation, and more. One aspect of EDI that we will discuss in depth is the interchange of EDI 852 or the Product Activity Data.
Understanding EDI and EDI 852
EDI is an established technology that promotes the computer-to-computer exchange of business documents in a standard electronic format. These documents can include purchase orders, invoices, and shipping notices, among others. By utilizing EDI, businesses can reduce the errors associated with manual data entry, speed up business cycles, and improve operational efficiency.
One of the various types of EDI transactions is EDI 852, also known as the Product Activity Data. It provides detailed sales and inventory information from the seller to the buyer. This report contains key information such as the product description, unit price, quantity sold, available stock, and more. Businesses can leverage this data to improve inventory management, analyze sales performance, forecast demand, and more.
The Value of Automating EDI 852 Reading
Automating the reading of EDI 852 provides businesses with several benefits. Here are the key ones:
Streamlined Operations
The use of EDI 852 removes the need for time-consuming and error-prone manual data entry. By automating the process, businesses can ensure that sales and inventory data is accurately and conveniently entered into their systems. This drastically reduces the resources spent on data management and allows businesses to focus on other critical operations.
Real-time Tracking
With automated reading of EDI 852, businesses can obtain close to real-time updates on sales and inventory status. This means they can quickly react to changes in demand, replenish stocks as needed, and avoid wasted inventory or stockouts.
Improved Decision-Making
The accurate data provided by EDI 852 equips businesses with valuable insights into their sales performance and inventory levels. These insights can inform strategic decisions such as when to run promotions, which products to discontinue, and how to manage supply chain logistics.
Conclusion
In conclusion, automation of reading EDI 852 brings about significant enhancements in tracking product activity data that contributes to improved operations, real-time tracking and better decision-making. As businesses strive for efficiency, adopting such technology could undoubtedly provide a competitive edge in today's data-driven business landscape.
Comments:
Thank you all for joining the discussion! I'm glad to see such engagement.
The use of ChatGPT in product activity data analysis seems promising. It could help uncover valuable insights and patterns that might have been missed before. Exciting times!
Absolutely, Laura! ChatGPT opens up new possibilities for analyzing complex data in a more interactive and conversational way. It can definitely enhance the accuracy and efficiency of EDI technology.
One concern I have is the potential bias and lack of accountability in the outputs generated by ChatGPT. How do we ensure fairness and reliability in the analysis?
Valid point, Michael. Bias detection and mitigation are crucial when using AI models like ChatGPT. It requires ongoing monitoring, evaluation, and fine-tuning to ensure fair and unbiased analysis. Transparency in the decision-making process is also essential to maintain accountability.
I'm curious to know how ChatGPT handles privacy concerns. Dealing with user's product activity data requires careful handling of sensitive information.
Great question, Olivia! Privacy is indeed a critical aspect. When using ChatGPT for product activity data analysis, it's important to ensure proper anonymization and adherence to data protection regulations. Implementing secure data handling practices and obtaining user consent are essential steps to mitigate privacy risks.
I wonder how well ChatGPT performs with unstructured data. Can it effectively derive insights from messy and diverse datasets?
That's a great point, Sophia. ChatGPT excels in handling unstructured data, including messy and diverse datasets. Its ability to generate context-aware responses makes it suitable for extracting meaningful insights from various data formats, such as text, images, and more.
ChatGPT sounds impressive, but what are its limitations? Are there specific use cases where it may not be as effective?
Good question, John. While ChatGPT is powerful, it has limitations. It may struggle with ambiguous queries or data with limited context. Also, it's important to carefully validate the outputs, especially when working with critical or sensitive data. Human oversight and validation are essential to ensure accuracy.
I'm curious about the scalability of using ChatGPT for large-scale data analysis. Can it handle high volumes of data efficiently?
Great question, Emily. ChatGPT's scalability depends on factors like computational resources, data size, and model optimization. It can handle considerable volumes of data, but for extremely large-scale analysis, distributed computing and optimization techniques may be required to ensure efficient processing.
This article provides a refreshing perspective on leveraging ChatGPT for product activity data analysis. It's exciting to see how AI models like ChatGPT are transforming the field of EDI technology!
Thank you for the kind words, Mark! Indeed, AI models like ChatGPT have immense potential to revolutionize data analysis in EDI technology. The advancements in natural language processing and machine learning continue to drive innovation and unlock new insights.
I can see ChatGPT being particularly useful in identifying trends and customer behavior patterns. It could assist businesses in improving their products and services based on data-driven insights.
Absolutely, Sarah! ChatGPT's ability to analyze and understand user-generated data can unlock valuable insights into customer behavior. With these insights, businesses can make informed decisions to enhance their offerings and meet customer demands effectively.
I'm wondering about the training process for ChatGPT. How do we ensure it's trained on diverse and representative datasets?
Great question, Maxwell. Ensuring diversity and representativeness in the training process is essential. It involves using a wide range of data sources, carefully curating training datasets, and fine-tuning the model to minimize biases. Continuous evaluation and improvement are necessary to enhance the model's performance and avoid undue generalizations.
I'm interested in the potential of ChatGPT for sentiment analysis of product reviews. Can it accurately determine the sentiment expressed by users?
Absolutely, Jessica! ChatGPT can be effectively utilized for sentiment analysis of product reviews. By processing user-generated text and understanding contextual cues, it can gauge sentiment accurately. However, it's still important to validate the results and consider domain-specific nuances.
I appreciate the article highlighting the benefits of leveraging ChatGPT. It's exciting to see how AI is pushing the boundaries of data analysis.
Thank you for your support, Jason! AI indeed plays a vital role in pushing the boundaries of data analysis. ChatGPT's conversational capabilities bring a new dimension to understanding and deriving insights from complex datasets.
What steps should organizations take to incorporate ChatGPT into their existing EDI technology stack?
Great question, Hannah! Incorporating ChatGPT into an existing EDI technology stack requires careful planning and implementation. Organizations should consider factors like data integration, infrastructure readiness, and user training. Assessing the specific use cases, evaluating the model's performance, and ensuring data security and privacy are crucial steps in the integration process.
The potential of ChatGPT for data analysis is intriguing, but what about explainability? Can we understand how it arrives at the insights it provides?
Valid concern, Daniel. Explainability is essential, especially in critical domains. While ChatGPT isn't inherently designed for explicit explanations, there are techniques like attention mechanisms that provide insights into the model's decision-making process. However, developing robust explainability methods for AI models remains an active area of research.
The potential of ChatGPT for data analysis is impressive, but what about bias in the training data? How can we ensure equitable outcomes?
Great point, Alexis! Bias in training data can impact the fairness of the analysis. Addressing this requires diverse and representative training data, continuous monitoring, and evaluation to identify and mitigate biases. It's also crucial to involve diverse perspectives during the training data curation and model evaluation phases to ensure equitable outcomes.
Has there been any comparative evaluation of using ChatGPT in product activity data analysis versus traditional methods? How does it stack up in terms of accuracy?
Good question, Ethan. Comparative evaluations are important in assessing the performance of ChatGPT. While it offers flexibility and interactivity, the accuracy depends on factors like data quality, model training, and specific use cases. Conducting well-designed evaluations, comparing results with traditional methods, and considering domain-specific requirements are essential in determining its effectiveness.
I can see how ChatGPT can facilitate exploratory data analysis. Its conversational nature could assist researchers in uncovering hidden patterns and relationships within datasets.
Absolutely, Grace! ChatGPT's conversational approach provides a unique way for researchers to explore and interact with data. Its ability to understand natural language queries and generate informative responses can help uncover hidden patterns and relationships, enabling more effective exploratory data analysis.
The advancements in AI and NLP are impressive. It's fascinating to witness how technologies like ChatGPT are transforming the data analysis landscape.
Indeed, Benjamin! The advancements in AI and NLP bring exciting possibilities for data analysis. ChatGPT's contribution to transforming the landscape is notable, and it's exciting to envision the future innovations that lie ahead.
I'm curious about the computational resources required for running ChatGPT. Do organizations need significant infrastructure investments?
Great question, Natalie. Running ChatGPT typically requires substantial computational resources, especially for larger models and high volumes of data. However, with cloud-based solutions and infrastructure providers, organizations can leverage scalable resources without significant upfront investments. It's important to consider the specific requirements and choose the appropriate infrastructure accordingly.
I wonder if ChatGPT could be used for predictive analytics based on historical product activity data. That could be valuable for decision making.
Absolutely, Leo! ChatGPT can be applied to analyze historical product activity data and provide insights for predictive analytics. By identifying patterns, trends, and correlations, businesses can make data-driven decisions and effectively plan for the future. It adds a valuable dimension to the decision-making process.
Considering potential limitations and biases, what are some best practices for using ChatGPT in product data analysis?
Good question, David. Some best practices include: 1) Training the model on diverse and representative data, 2) Constantly monitoring for biases and evaluating the model's outputs, 3) Validating the results against ground truth or human expertise, 4) Incorporating human oversight in critical decision-making, and 5) Iteratively improving the model through user feedback and ongoing evaluation.
James, could you provide some insights into the implementation challenges organizations might face when adopting ChatGPT for data analysis?
Certainly, Michael. Implementation challenges could include: 1) Acquiring or generating high-quality training data, 2) Defining the appropriate scope and use cases for ChatGPT, 3) Balancing model complexity, resource requirements, and infrastructure considerations, 4) Addressing privacy and security concerns in handling user data, and 5) Training and educating personnel to effectively work with ChatGPT. Overcoming these challenges requires careful planning, collaboration, and continuous improvement.
Given the dynamic nature of data analysis, how can ChatGPT adapt to evolving business needs and incorporate domain-specific knowledge?
Great question, Sophia. Adapting ChatGPT to evolving business needs and incorporating domain-specific knowledge requires iterative refinement. Continuously retraining the model with updated data, fine-tuning based on specific use cases, and involving subject matter experts in the training process can help ensure that ChatGPT stays aligned with evolving business requirements and incorporates relevant domain knowledge.
What are some potential risks or ethical considerations associated with leveraging ChatGPT in product data analysis?
Excellent question, Daniel. Risks and ethical considerations can include: 1) Privacy breaches when handling user data, 2) Biased or unfair analysis due to training data or algorithmic limitations, 3) Overreliance on ChatGPT without human validation, 4) Misinterpretation of results leading to incorrect decisions, and 5) Ensuring compliance with data protection and privacy regulations. Organizations must proactively address these risks and implement safeguards to mitigate ethical concerns.
Considering the rapid development of AI models, what do you envision for the future of ChatGPT in product data analysis?
Great question, Laura! The future of ChatGPT in product data analysis looks promising. As models evolve, we can expect improved accuracy, enhanced explainability, and better handling of complex datasets. Integrating ChatGPT with advanced analytics techniques, such as automated feature engineering and anomaly detection, could further augment its capabilities. Collaboration between AI and domain experts will continue to drive innovation, unlocking deeper insights from product data.
Thank you all for this insightful discussion! Your questions and perspectives have shed light on various aspects of leveraging ChatGPT in product data analysis. Let's stay connected and explore the exciting possibilities together!