Unlocking the Potential: Leveraging Gemini for Argus Modeling in the Technology Industry
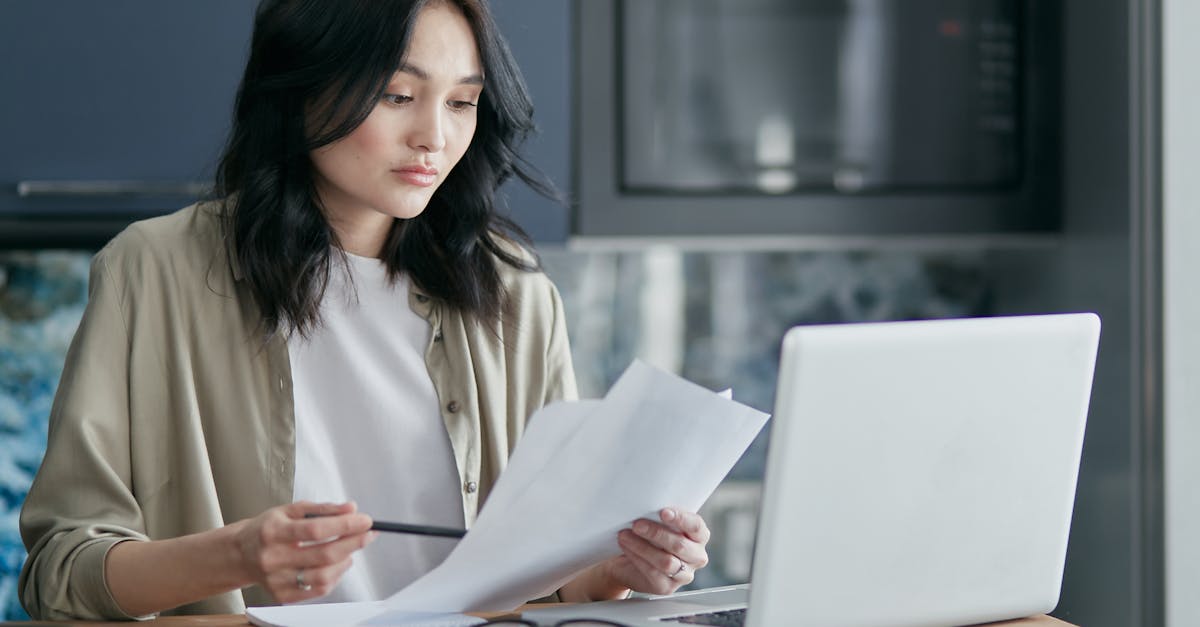
In today's fast-paced and competitive technology industry, companies are constantly seeking innovative ways to gain a competitive edge. One of the emerging technologies that has shown tremendous potential is Gemini. This advanced language model, developed by Google, has proven to be a valuable tool for various applications, including Argus modeling.
The Technology
Gemini is an artificial intelligence (AI) model that uses deep learning techniques to generate coherent and contextually relevant text. Based on the Transformer architecture, Gemini has been trained on a vast corpus of data from the internet, making it adept at understanding and producing human-like text. It can engage in natural conversational interactions and provide detailed responses.
The Area
Argus modeling, on the other hand, is a critical aspect of the technology industry. It involves predicting and analyzing potential risks or vulnerabilities in software systems, enabling companies to strengthen their defenses against threats. Traditionally, Argus modeling has required a significant amount of manual effort and expertise. However, with the advent of Gemini, there is an opportunity to leverage its capabilities to streamline and enhance the Argus modeling process.
The Usage
By incorporating Gemini into Argus modeling workflows, technology companies can unlock several benefits. Firstly, Gemini can assist in generating potential attack scenarios and vulnerabilities more efficiently. With its vast knowledge and conversational abilities, Gemini can engage in dialogue with human experts, asking and answering relevant questions to refine the modeling process.
Furthermore, Gemini can help in automating parts of the Argus modeling pipeline, reducing manual labor and allowing experts to focus on more complex tasks. It can assist in data preprocessing, feature extraction, and even in the evaluation of different risk mitigation strategies. Its ability to provide detailed explanations for its reasoning can also aid in decision-making processes.
Another valuable aspect of leveraging Gemini in Argus modeling is its potential for collaborative and interactive modeling. Multiple experts can collaborate with Gemini simultaneously, generating ideas, identifying potential threats, and even evaluating model outputs. This can foster innovation, knowledge sharing, and ultimately lead to more comprehensive and robust Argus models.
Conclusion
Gemini presents a significant opportunity for the technology industry to enhance Argus modeling processes. By leveraging the conversational abilities, vast knowledge, and reasoning capabilities of Gemini, companies can unlock new possibilities in predicting and mitigating software vulnerabilities. The collaborative and interactive nature of Gemini enables experts to work together and foster innovation in Argus modeling workflows. As technology continues to evolve, embracing AI models like Gemini will ensure that companies stay one step ahead in securing their software systems.
Comments:
Thank you all for reading my article on leveraging Gemini for Argus modeling in the technology industry! I'm excited to hear your thoughts and answer any questions you may have.
Great article, Lan! It's fascinating to see how language models like Gemini can be applied to industry-specific tasks. I'm curious, do you think this technology will have any privacy implications?
Hi Sarah, thanks for your question! Privacy is definitely a concern when using language models like Gemini. It's essential to handle sensitive data carefully and ensure proper anonymization measures are in place. Additionally, it's important to train models on a diverse range of data to avoid biases. Overall, while there are considerations, with appropriate precautions, Gemini can bring significant value to various industries.
Lan, I found your article very informative. As someone working in the technology industry, I can see the potential of leveraging Gemini for Argus modeling. Are there any limitations or challenges that you've encountered while using this approach?
Hi Michael, I'm glad you found the article informative! While Gemini has shown promise, there are some limitations. It can sometimes generate incorrect or nonsensical outputs, and it requires careful fine-tuning to ensure accurate results. The model may also be sensitive to slight changes in input phrasing, leading to varying responses. Overcoming these challenges involves rigorous testing, iterative feedback collection, and continuous improvement of the training pipeline.
Lan, thank you for sharing your insights into Gemini within the technology industry. How do you envision this technology being used in the realm of customer support?
Hi Emily, you're welcome! Gemini holds significant potential for customer support applications. It can be used to automate responses to frequently asked questions, provide initial troubleshooting guidance, and offer personalized recommendations based on user preferences. However, it's important to remember that human involvement and oversight are crucial to handle complex scenarios and ensure customer satisfaction.
Lan, your article gave me a fresh perspective on how language models can enhance Argus modeling in the technology industry. How do you see this technology evolving in the future, and what other areas do you think it could be applied to?
Hi David, I'm glad the article provided a fresh perspective! Language models like Gemini are evolving rapidly, and in the future, we expect even more powerful and specialized models to emerge. As for applications, besides the technology industry, such models can be utilized in fields like education, legal assistance, content creation, and even creative writing. The possibilities are vast, and it's an exciting time to explore the potential of AI-powered language models.
Interesting article, Lan! I can certainly see the benefits of leveraging Gemini for Argus modeling. Have you encountered any ethical concerns during your work?
Hi Mark, thank you for your feedback! Ethical concerns are indeed important to address. It's crucial to ensure fairness, transparency, and accountability in the deployment of language models. This includes avoiding biased training data, handling potential misuse of the technology, and being transparent about the limitations of the models. Continued research and collaboration with the AI community are key to navigating ethical considerations appropriately.
Lan, excellent article! I'm curious, how do you handle the challenge of controlling output quality and maintaining consistent performance when using Gemini for Argus modeling?
Hi Jessica, thank you for your kind words! Controlling output quality and maintaining performance consistency involves a multi-step process. It starts with collecting feedback to identify problematic outputs and understanding model failure modes. Iterate on the model and fine-tune it using curated datasets. Set up human reviewers for ongoing monitoring and apply safety mitigations to avoid harmful or biased outputs. It's crucial to strike a balance between allowing creativity and controlling for correctness and safety.
Lan, thank you for shedding light on the potential of Gemini for Argus modeling in the technology industry. How can companies effectively integrate this technology within their existing infrastructure?
Hi Sophia, you're welcome! Integrating Gemini effectively involves a phased approach. Start with a narrow domain and gradually expand the model's capabilities. Integration requires defining clear use cases, ensuring scalable infrastructure, and providing model API access for developers. Extensive testing and user feedback are crucial to identifying and addressing any limitations or issues. Collaboration between AI experts, engineers, and domain specialists is key for successful integration and deployment.
Lan, your article made me rethink the potential applications of Gemini in the technology industry. How do you address security concerns while leveraging this technology?
Hi Andrew, I'm glad the article sparked new ideas! Security concerns are vital when leveraging Gemini. To mitigate risks, it's crucial to handle user data securely, implement proper access controls, and employ encryption techniques where required. Companies must adhere to best practices for data privacy and ensure compliance with relevant regulations. Thorough security assessments and regular audits help maintain a secure environment when using language models like Gemini.
Lan, I enjoyed reading your article on leveraging Gemini for Argus modeling. How can we address potential biases that may be present in the language model?
Hi Melissa, thank you for your feedback! Addressing biases is crucial. During training, it's essential to use a diverse range of data sources and carefully review potential biases in the training data. By involving human reviewers from different backgrounds, we can achieve a broader perspective and mitigate biases. It's an ongoing effort to improve models and make them fair and inclusive. Open dialogue and collaboration with the AI community are vital to address biases effectively.
Lan, great article! The potential applications of Gemini in the technology industry are impressive. Could you share any insights on how Argus modeling can be enhanced in specific domains?
Hi Robert, I'm glad you found the article great! Argus modeling can be enhanced in specific domains by leveraging the strengths of Gemini. For instance, in the technology industry, it can be used to enhance product recommendation systems, automate customer support, assist in software testing, and provide real-time analytics for network optimization. The key is to identify the specific pain points and tailor the application of Gemini to address those needs effectively.
Lan, your article was eye-opening. How do you ensure that the outputs generated by Gemini for Argus modeling are accurate and reliable?
Hi Jennifer, thank you for your kind words! Ensuring accuracy and reliability involves rigorous testing and continuous feedback loops. We establish evaluation metrics, curate datasets for fine-tuning, and continually refine the model based on user feedback. By combining human reviewers and user feedback, we iteratively improve the model's performance and address any potential inaccuracies or reliability concerns. Ongoing monitoring and collaboration with the user community play a crucial role in ensuring accurate and reliable outputs.
Lan, as a data scientist, I'm impressed by the potential of Gemini for Argus modeling. How do you handle the challenge of domain specificity when applying this technology?
Hi Chris, I'm glad you're impressed! Handling domain specificity involves an iterative approach. Start with a narrow domain and gradually expand the model's knowledge and capabilities as the training data expands. Introduce domain-specific prompts and use-case-specific fine-tuning to tailor the model's responses to industry-specific needs. Incorporating user feedback and leveraging human reviewers specialized in the target domain help refine the model and enhance its domain-specific performance over time.
Lan, your article was insightful. How do you address the challenge of Gemini generating incorrect or nonsensical outputs?
Hi Alexandra, thank you for your feedback! Addressing incorrect or nonsensical outputs is an ongoing challenge with language models like Gemini. We employ techniques like improving model training using human feedback, collecting erroneous outputs to fine-tune the model, and refining the decoding processes. Dataset curation and careful engineering help minimize the occurrence of incorrect outputs. However, continuous efforts are required to identify and fix such issues through close collaboration with the model's user community.
Lan, I appreciate your in-depth explanation of leveraging Gemini for Argus modeling. Are there any known limitations in terms of the amount of data required for training these models?
Hi Daniel, thanks for your question! Training language models like Gemini requires a large amount of diverse and high-quality data. While more data can generally contribute to better performance, there is a practical constraint on the computational resources needed for training. Balancing the available resources, the quality of the data, and the required model capacity is a crucial part of the training process. Continuous improvement of data collection processes helps refine and enhance the model's performance.
Lan, your article shed light on the potential of Gemini for Argus modeling. How do you ensure that the model's responses are understandable and meaningful to the end users?
Hi Julia, I'm glad the article provided insight! Ensuring understandable and meaningful responses to end users is a priority. We employ techniques like training models on human-generated feedback, collecting a range of responses and selecting the most appropriate ones, and iteratively improving the model's performance based on user evaluations. Continuous user testing and feedback loops are crucial to refine the model's language generation and ensure meaningful and user-friendly outputs.
Lan, your approach to leveraging Gemini for Argus modeling is impressive. How can we deploy the models without exposing confidential information or sensitive data?
Hi Timothy, thank you for your kind words! Protecting confidential information and sensitive data is essential when deploying language models. Techniques like data anonymization, secure data handling, and strict access controls help mitigate risks. It's important to have comprehensive policies and procedures in place to ensure compliance with data privacy regulations. By design, models like Gemini are intended to respect user privacy while providing valuable insights and assistance.
Lan, your insights on leveraging Gemini for Argus modeling are valuable. How do you handle challenges related to target user populations with varying levels of technical knowledge?
Hi Michelle, I'm glad you find the insights valuable! Addressing the varying levels of technical knowledge in target user populations is crucial. We consider user segmentation based on their technical expertise, offer different levels of guidance, and provide contextual information to assist users with different knowledge levels. Evaluating user satisfaction through feedback and user testing helps us identify areas for improvement and refine the model's responses to cater to diverse user populations.
Lan, your article has broadened my understanding of how Gemini can be employed in Argus modeling. Can you share any future research directions in this field?
Hi Edward, I'm glad the article broadened your understanding! Future research directions in Gemini and Argus modeling include developing more domain-specific models with improved accuracy, exploring interactive learning paradigms to incorporate user preferences, and enhancing long-term context handling for more coherent conversations. There's also ongoing research on interpretability and understandability aspects to make these models more transparent and accountable. Exciting times lie ahead as we continue to push the boundaries of AI language models!
Lan, your article provides valuable insights into leveraging Gemini for Argus modeling. What strategies do you employ to ensure model updates align with the evolving needs of the technology industry?
Hi Samantha, thank you for your feedback! Ensuring model updates align with evolving industry needs is a collaborative effort. We closely monitor user feedback, engage with industry experts, and gather insights from the community to identify specific pain points and areas for improvement. User surveys, feedback mechanisms, and partnerships with industry leaders help us stay informed about the evolving needs and challenges. By leveraging collective knowledge and ongoing collaboration, we strive to enhance and align the models with industry requirements.
Lan, your article gave me a better understanding of the potential of Gemini for Argus modeling. How do you ensure the model is unbiased and inclusive for users with diverse backgrounds?
Hi Richard, I'm glad the article provided a better understanding! Ensuring an unbiased and inclusive model is essential. We work closely with a diverse set of reviewers to identify and address biases. Feedback from users with diverse backgrounds helps us uncover any potential issues and improve the model's responses. The AI community's collective vigilance is vital in identifying and mitigating biases as we strive to create AI systems that are fair, respectful, and inclusive for all users.
Lan, your insights on leveraging Gemini for Argus modeling are inspiring. How can potential biases in the training data be addressed?
Hi Laura, thank you for your kind words! Addressing potential biases in the training data is essential for responsible use of language models. We employ a combination of techniques such as carefully curating datasets from diverse sources, including the perspectives of reviewers from different backgrounds, and continuously refining the training process. Open dialogue, transparency, and collaboration within the AI community are key elements to identify and address biases and strive for fair and inclusive models.
Lan, I found your article informative. How do you handle situations where Gemini generates responses that resemble human-written content but are incorrect or misleading?
Hi Peter, I'm glad you found the article informative! Addressing situations where Gemini generates incorrect or misleading responses is an ongoing challenge. We employ techniques like reinforcement learning from human feedback to improve the model's performance, iterate on the model architecture to control and reduce such instances, and actively seek user feedback to identify problematic scenarios. The combination of human reviewers and user feedback is crucial in identifying and improving the model's accuracy over time.
Lan, your article sheds light on the potential of Gemini for Argus modeling. How can we approach bias detection and mitigation when using language models in sensitive applications?
Hi Emma, thanks for your question! Bias detection and mitigation in sensitive applications require a proactive approach. It involves conducting comprehensive audits of training data, monitoring the model's outputs for potential bias, and leveraging diverse human reviewers with varying backgrounds. Establishing clear guidelines for reviewers and refining the fine-tuning process helps mitigate biases. Continuous feedback loops and collaborations with domain specialists assist in improving the model and addressing biases effectively in sensitive applications.
Lan, your article provided great insights into the potential of Gemini for Argus modeling. Can you share any experiences around instances where user trust in these models is crucial?
Hi Oliver, I'm glad you found the insights great! User trust is indeed crucial when using language models. Instances where user trust is paramount include fields like legal counseling, where users rely on accurate information, and mental health support, where empathetic responses are essential. In situations where user trust is paramount, models like Gemini should be deployed with human oversight and clear guidelines to ensure reliability, safety, and the best interests of the users.
Lan, your approach to leveraging Gemini for Argus modeling is impressive. How do you handle the challenge of model interpretability and explainability?
Hi Sophie, thank you for your kind words! Model interpretability and explainability are active areas of research. While language models like Gemini can be challenging in terms of transparency, there are efforts to develop techniques and methodologies for better interpretability. Techniques like rule-based post-filtering, attention visualization, and user interface designs that promote understanding are being explored. Making progress in these areas is essential to ensure transparency and to instill user confidence in these models.
Thank you all for your interest in my article. I'm really excited to discuss the potential of leveraging Gemini for Argus modeling in the technology industry!
Great article, Lan! It's fascinating to see how natural language processing can be applied to enhance Argus modeling. Can you share more about the specific use cases in the technology industry?
Thanks, Philip! Absolutely, Gemini can be leveraged in various ways. In the technology industry, it can be used for sentiment analysis on customer feedback, chatbot development, content generation, and even predicting future trends based on chat data.
Lan, do you think the accuracy and reliability of Gemini is sufficient for complex Argus modeling tasks? Are there any limitations we should consider?
That's a great question, Maria. While Gemini has shown impressive capabilities, it's important to note that it sometimes generates responses that may sound plausible but are incorrect or nonsensical. Also, it can be sensitive to input phrasing, sometimes producing different responses with slight variations. Proper validation and fine-tuning are crucial to ensuring accurate predictions.
Lan, I am curious about the computational resources required for implementing Gemini in the Argus modeling process. How demanding is it in terms of hardware and processing power?
Good point, James. Training and using large language models like Gemini can indeed be computationally expensive. It requires powerful GPUs or specialized hardware to handle the high computational load efficiently. Efficient infrastructure and resource management are crucial to leverage Gemini effectively.
Lan, what steps should organizations take to address potential ethical concerns when utilizing Gemini for Argus modeling? How can bias be mitigated?
Excellent question, Susan. It's crucial to prioritize ethical considerations when implementing Gemini. Transparency, fairness, and diversity in the training data are essential to mitigate biases. Additionally, ongoing monitoring, user feedback, and strong moderation systems can help identify and rectify potential ethical issues that may arise.
Lan, how would you compare the performance of Gemini with other language models in Argus modeling? Are there any specific areas where Gemini excels?
Thanks for your question, Robert. Gemini has demonstrated impressive performance in generating human-like responses and understanding context. It can excel in tasks requiring natural language interaction, such as customer support, virtual assistants, and content creation. However, in more specialized domains, task-specific models might still be more appropriate.
Lan, how scalable is the implementation of Gemini for large technology enterprises? Can it efficiently handle a high volume of real-time chat data?
Great question, Emily. The scalability of Gemini depends on the infrastructure and resources available. With proper design and efficient systems, it can handle a high volume of real-time chat data. However, it's essential to ensure that the infrastructure can handle the increased processing load and provide quick response times to meet enterprise-level demands.
Lan, how customizable is Gemini for industry-specific terminology and jargon? Can it be fine-tuned to handle specialized vocabulary in the technology sector?
Good question, Alex. Gemini can be fine-tuned on specific data to handle industry-specific terminology and jargon. By providing relevant examples during fine-tuning, the model can learn to better understand and generate responses aligned with the technology sector's vocabulary. Fine-tuning enables customization for improved performance in specific domains.
Lan, what are the main practical challenges organizations might face when implementing Gemini for Argus modeling, and how can they be overcome?
Good question, Olivia. One practical challenge is the availability of high-quality training data. Another challenge is the computational resources required for training and inference. Organizations need to ensure access to diverse and representative datasets and invest in infrastructure capable of handling the resource demands. Collaboration with experts in NLP and continuous model monitoring also helps overcome challenges.
Lan, could you provide some insights into the potential risks associated with using Gemini in the Argus modeling process? How can they be mitigated?
Certainly, Daniel. Some potential risks include generating misleading content, biases in responses, or inappropriate language usage. To mitigate these risks, organizations should implement strong validation and moderation systems, ensure diverse training data, actively seek user feedback, and regularly monitor model outputs. Proactive measures can help minimize the impact of these risks.
Lan, how can organizations evaluate the effectiveness and performance of Gemini in their Argus modeling tasks? Are there any specific metrics to consider?
Great question, Sophie. Evaluating Gemini's effectiveness requires assessing various metrics. Common metrics include accuracy, relevance, coherence, and user satisfaction. Organizations can conduct both automated evaluations and gather feedback from end-users to understand how well the model performs in their specific tasks. Continuous evaluation and improvement are essential to ensure desired outcomes.
Lan, what are the potential privacy concerns when utilizing Gemini for Argus modeling? How can sensitive information be protected?
That's an important point, Jack. Organizations must handle user data and sensitive information with utmost care. Anonymizing data, implementing strong security measures, and adhering to data privacy regulations are crucial. Additionally, regular audits and strict access controls can help safeguard sensitive information and protect user privacy throughout the Argus modeling process.
Lan, do you think Gemini has the potential to replace human experts in the technology industry's Argus modeling tasks?
Great question, Melissa. While Gemini can enhance the Argus modeling process, it's unlikely to entirely replace human experts. Human expertise is valuable in interpreting complex scenarios, context, and ensuring ethical considerations. Gemini can assist experts, automate certain tasks, and provide valuable insights, but a collaborative approach combining human and AI expertise is often the most effective.
Lan, what are your recommendations for organizations interested in experimenting with Gemini to enhance their Argus modeling capabilities?
Thanks for asking, Chris. Organizations should start by identifying specific use cases where Gemini can bring value. They should invest in quality training data, fine-tune the model, and establish proper validation and monitoring processes. Collaboration with experts in NLP and iterative experimentation can help organizations leverage Gemini effectively and unlock its full potential.
Lan, are there any legal implications organizations should be aware of when implementing Gemini for Argus modeling in the technology industry?
Absolutely, Emma. Organizations need to consider legal aspects when implementing Gemini. This includes compliance with privacy regulations, intellectual property rights, and usage restrictions of third-party data. Consulting legal experts to ensure lawful and ethical usage is essential to avoid any potential legal complications related to the implementation.
Lan, from your experience, what are the main benefits organizations can expect by leveraging Gemini in their technology industry Argus modeling tasks?
Great question, David. Leveraging Gemini can bring several benefits. These include improved customer interactions, enhanced scalability, reduced response times, automation of repetitive tasks, and gaining valuable insights from large volumes of chat data. It can empower organizations to make data-driven decisions, improve efficiency, and ultimately deliver better products and services to their customers.
Lan, what are the potential future advancements and developments we can expect in the field of Gemini and its application in the technology industry Argus modeling?
Thanks for your question, Julia. The field of Gemini is rapidly evolving. We can expect advancements in areas like improved language understanding, better fine-tuning techniques, addressing biases, and reducing nonsensical outputs. Continued research and innovation will likely lead to more powerful and customizable models that can better serve the Argus modeling needs of the technology industry.
Lan, how do you anticipate the integration of Gemini in Argus modeling to impact the tech industry in the next few years?
Excellent question, Richard. Gemini integration in Argus modeling has the potential to revolutionize the tech industry. It can enhance customer support experiences, reduce operational costs, drive innovation, and empower organizations to extract valuable insights from their chat data. As the technology matures and models improve, we can expect Gemini to become an indispensable tool for many tech companies.
Lan, what are the key considerations organizations should keep in mind before adopting Gemini for Argus modeling?
Thanks for your question, Daniel. Organizations should consider factors such as the availability of quality training data, infrastructure requirements, ethical implications, and potential risks associated with the technology. A thorough evaluation of the benefits and challenges specific to their use case is crucial. Proper planning, testing, and collaboration with experts can ensure a successful adoption of Gemini for Argus modeling.
Lan, what are the implications of integrating Gemini in Argus modeling for the end-users? How can organizations ensure a positive and user-friendly experience?
Great question, Grace. For end-users, Gemini integration can improve their experience by providing quicker and more accurate responses to their queries. To ensure a positive experience, organizations should invest in user-centric design, multi-step validation systems, regular user feedback loops, and effective moderation to prevent inappropriate content. Prioritizing user satisfaction and continuously refining the system contribute to a user-friendly experience.
Lan, how do you see the adoption of Gemini in Argus modeling affecting the job roles and responsibilities of professionals in the technology industry?
Interesting question, Michael. While Gemini adoption can automate certain tasks, it's unlikely to replace professionals. Instead, it can reshape job roles by shifting the focus towards more complex problem-solving, creativity, and providing strategic insights. Professionals can leverage Gemini to augment their capabilities, allowing them to focus on high-level decision-making and leveraging the model's outputs effectively.
Lan, what kind of computational resources and infrastructure are typically needed to run Gemini models effectively?
Great question, Sophia. Running Gemini effectively typically requires high-performance hardware, such as powerful GPUs or specialized accelerators. The computational requirements vary based on the model's size and complexity. Typically, larger models demand more computational resources. Access to efficient infrastructure that supports the high computational load is essential to run Gemini models effectively for Argus modeling in the technology industry.
Lan, are there any specific pre-training techniques or steps that can help improve the performance and mitigate the challenges associated with Gemini in Argus modeling?
Thanks for asking, Emily. Pre-training Gemini on high-quality and diverse datasets is vital to its performance. Techniques like curriculum learning, reinforcement learning, and data augmentation can be employed during pre-training to improve the model's capabilities. Additionally, fine-tuning with domain-specific data, continuous monitoring, and user feedback help identify and address challenges and improve the model's performance in Argus modeling tasks.
Lan, in scenarios where the generated response from Gemini is incorrect, how can organizations ensure proper validation to prevent misleading information?
Validating the responses from Gemini is crucial to prevent misleading information. Organizations can employ techniques like inter-rater agreement, human-in-the-loop validation, and continuous model monitoring. Building strong validation pipelines and involving subject-matter experts can help identify incorrect or nonsensical responses. User feedback loops are also valuable for iterative improvements and maintaining a reliable system.
Lan, how does the training data quality impact the performance of Gemini in Argus modeling? What steps can organizations take to ensure high-quality data?
Training data quality greatly impacts Gemini's performance in Argus modeling. High-quality data ensures the model learns meaningful patterns and generates accurate responses. Organizations can ensure data quality by carefully curating and preprocessing datasets, adhering to data collection guidelines, and implementing quality control measures. Balancing diversity, representation, and relevance in the training data is crucial to improve the model's performance.
Lan, what steps should organizations follow to ensure ongoing monitoring and maintenance of Gemini models to guarantee their continued effectiveness?
Ensuring ongoing monitoring and maintenance of Gemini models is vital for their continued effectiveness. Organizations should establish processes for continuous model evaluation, user feedback collection, and validation. It's important to regularly retrain, fine-tune, and update the models with new data to adapt to evolving requirements. Collaborative efforts between data scientists, domain experts, and end-users facilitate effective model monitoring and maintenance.