Unlocking the Potential of ChatGPT: A Benchmarking Approach for Metrology Technology
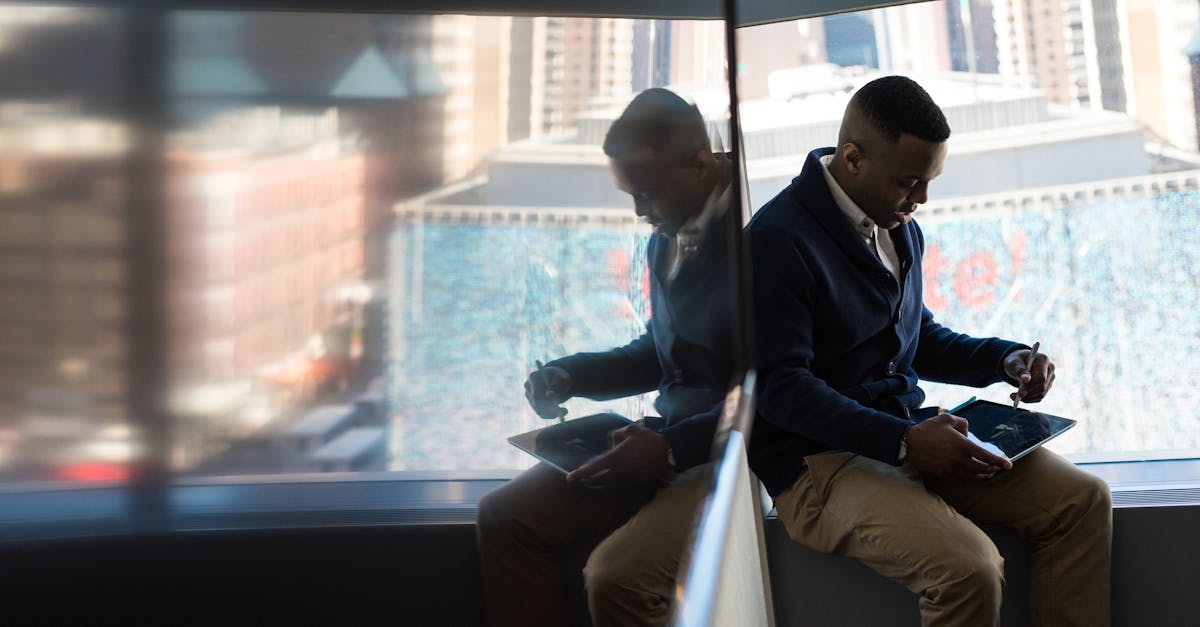
Metrology is the science of measurement and plays a crucial role in various industries, including manufacturing, engineering, and healthcare. The accuracy and traceability of measurements are essential for ensuring quality and compliance with industry standards. One of the key aspects of metrology is benchmarking, which involves comparing measurement processes and results against established industry benchmarks.
In recent years, the advancements in artificial intelligence (AI) and natural language processing (NLP) technologies have paved the way for AI-powered virtual assistants like ChatGPT-4. This sophisticated language model can assist in various tasks, including metrology benchmarking.
What is ChatGPT-4?
ChatGPT-4 is an AI-powered virtual assistant developed using state-of-the-art deep learning techniques. It is designed to understand and generate human-like text responses, making it an ideal tool for benchmarking metrology processes and comparing results against industry standards.
The Role of ChatGPT-4 in Metrology Benchmarking
ChatGPT-4 can assist metrology professionals and researchers in several ways:
- Process Evaluation: Metrology processes are constantly evolving, and new techniques are being developed. ChatGPT-4 can provide insights and recommendations on the latest industry practices and help evaluate the efficiency and effectiveness of existing measurement processes.
- Benchmark Comparison: Comparing measurement results against industry benchmarks is essential for ensuring accuracy and traceability. ChatGPT-4 can analyze the data and provide meaningful comparisons, highlighting areas where improvements can be made.
- Identifying Deviations: Detecting deviations from benchmarked measurement processes is crucial for maintaining quality standards. ChatGPT-4 can identify potential deviations and provide guidance on how to rectify them.
- Trend Analysis: Analyzing trends in metrology data can uncover patterns and insights that may be useful in process optimization. ChatGPT-4 can help identify such trends and provide recommendations based on the analysis.
- Data Integration: Metrology processes involve collecting and analyzing large amounts of data. ChatGPT-4 can assist in integrating and analyzing diverse datasets, facilitating a comprehensive benchmarking analysis.
The Benefits of Using ChatGPT-4 for Metrology Benchmarking
Here are some of the benefits of leveraging ChatGPT-4 for metrology benchmarking:
- Efficiency: ChatGPT-4 can rapidly process and analyze vast amounts of metrology data, significantly reducing the time and effort required for benchmarking.
- Accuracy: With its advanced language processing capabilities, ChatGPT-4 can provide accurate comparisons against industry benchmarks, ensuring reliable results.
- Insights: ChatGPT-4 can offer valuable insights into metrology processes, helping identify potential areas for improvement and optimization.
- Collaboration: ChatGPT-4 can facilitate collaboration among metrology professionals by providing a platform for sharing knowledge, discussing benchmarking results, and exchanging best practices.
- Continuous Learning: AI models like ChatGPT-4 continually learn and improve with more data and user interactions, ensuring that the benchmarking recommendations remain up-to-date and highly valuable.
Conclusion
ChatGPT-4 holds tremendous potential in assisting metrology professionals and researchers in benchmarking processes and comparing results against industry benchmarks. Its advanced AI capabilities can streamline the benchmarking analysis, offer valuable insights, and foster collaboration among stakeholders. With the assistance of ChatGPT-4, the field of metrology can continue to evolve and ensure accurate and reliable measurements for various industries.
Comments:
Thank you all for taking the time to read my article on unlocking the potential of ChatGPT for metrology technology. I look forward to hearing your thoughts and engaging in discussion!
Great article, Ryan! ChatGPT has shown enormous potential in various fields, and I'm glad to see its application in metrology technology being explored. I have a couple of questions though. How scalable is the benchmarking approach, and what are the key challenges in implementing it?
Thanks, Sarah! The benchmarking approach used for ChatGPT in metrology technology is designed to be scalable. It leverages large datasets of annotated metrology-related conversations to fine-tune the model. One of the challenges is ensuring that the model's responses consistently align with accurate and reliable measurement techniques.
Thank you, Ryan! It's good to know that benchmarking metrics cover both accuracy and contextual relevance. How do you tackle potential bias or subjective interpretation issues when fine-tuning the model?
Sarah, addressing bias and subjective interpretation is critical in fine-tuning the model. The benchmarking data is carefully annotated and reviewed to ensure fairness and minimize bias. Multiple annotators are involved to avoid individual subjectivity. The iterative refinement process helps in continually improving the model's objectivity and reducing biased responses.
Thanks for sharing the process, Ryan! I'm intrigued by the iterative refinement and validation steps in minimizing bias. How do you ensure that the fine-tuning process doesn't introduce new biases?
Sarah, avoiding the introduction of new biases during the fine-tuning process is indeed crucial. Multiple rounds of annotation review and consistency checks are performed to minimize individual subjectivity or biased interpretations. Diversity in the annotation team and periodic inter-annotator agreement assessments help maintain an objective perspective and reduce the risk of introducing new biases.
Thanks for your response, Ryan. In the future, do you anticipate incorporating unsupervised or reinforcement learning methods to further enhance ChatGPT's performance in metrology applications?
Sarah, the exploration of unsupervised and reinforcement learning methods to enhance ChatGPT's performance in metrology applications is definitely an area of interest. Such approaches can complement supervised fine-tuning and offer additional ways to improve the model's robustness, adaptability, and ability to handle out-of-distribution queries. Ongoing research aims to explore these avenues while addressing challenges specific to the metrology domain.
Interesting article, Ryan! Have you encountered any situations in which ChatGPT's responses surprised you or offered unique insights in metrology applications?
Mark, during the development and testing phase, there were indeed instances when ChatGPT's responses surprised me with unique insights. The model's ability to uncover subtle connections or provide alternative perspectives demonstrated its potential as a supportive tool for metrologists. However, it's crucial to balance such surprises with maintaining accountability and reliability in measurement-related tasks.
Interesting topic, Ryan! I'm curious about the accuracy and reliability of ChatGPT in metrology applications. How does it compare to traditional measurement methods in terms of precision?
Hi David, thanks for your question! ChatGPT's accuracy in metrology applications heavily depends on the training data quality and fine-tuning process. In comparison to traditional measurement methods, it may not achieve the same level of precision in some cases, but it shows promise in tasks that require context understanding and reasoning.
Thank you, Ryan! It's fascinating to see how ChatGPT's performance can differ based on the quality of training data and fine-tuning. Are there any limitations related to the model's domain comprehension or the potential for ambiguous responses?
David, while ChatGPT can comprehend a wide range of metrology domains, it may still struggle with highly specialized or obscure areas where comprehensive training data is lacking. Ambiguous queries can be challenging, and the model may sometimes provide responses that require further clarification. Efforts are made to ensure contextual accuracy and minimize misleading or inaccurate outputs.
Ryan, considering that measurement techniques can vary across different industries and applications, how does ChatGPT handle domain-specific nuances and ensure accurate responses?
David, handling domain-specific nuances in ChatGPT involves training the model on a diverse range of metrology domains. The annotated benchmarking datasets cover variations in measurement techniques and industry-specific requirements. While it may not encapsulate the entirety of each domain, fine-tuning helps ensure ChatGPT understands and responds accurately within the bounds of its training data.
Ryan, when validating and refining ChatGPT, how do you ensure that the model doesn't generate answers that are excessively verbose or instead provides concise responses?
David, striking a balance between verbosity and concise responses is considered during refinement. The training data and annotation guidelines help guide the model to generate responses of optimal length. Iterative training cycles also involve analyzing response length statistics and user feedback to avoid excessively verbose or overly concise answers, aiming for informative yet succinct responses.
Hi Ryan, thanks for sharing your research! I wonder if ChatGPT's performance is affected by the complexity of measurement tasks. Are there specific types of metrology applications where it excels?
Hi Emily! ChatGPT's performance can be affected by task complexity to some extent. It performs well in general metrology applications and excels in situations where the task involves complex measurement concepts, ambiguities, or context-dependent analysis. However, it may struggle with highly specialized, niche metrology domains where domain-specific knowledge is crucial.
Ryan, are there any plans to address potential biases that can be present in the benchmarking data used to train ChatGPT for metrology technology?
Emily, addressing potential biases in benchmarking data is an ongoing concern. Comprehensive data preprocessing involves detecting and reducing biases, ensuring diversity in the data sources, and balancing industry representation. Collaborating with experts and regularly evaluating annotation guidelines help in continuously improving the dataset to minimize biases.
Good work, Ryan! As metrology technology advances, what role do you see ChatGPT playing in improving the efficiency and productivity of measurement processes? Are there any potential limitations?
Thank you, Michael! In terms of efficiency and productivity, ChatGPT can provide quick and accessible answers to measurement-related queries, reducing the need for extensive manual research. It can assist metrologists in handling routine tasks, freeing up time for more complex analyses. However, as with any AI system, there can be limitations in handling out-of-distribution queries or cases where human judgments are necessary.
Ryan, could you please provide some insights into the specific benchmarking metrics used to evaluate the performance of ChatGPT in metrology applications?
Jessica, the benchmarking metrics for evaluating ChatGPT in metrology applications typically include accuracy, contextual relevance, and response coherence. Precision comparison with traditional methods can be assessed by comparing consistency and conformity with established measurement standards.
Thank you for explaining the benchmarking metrics, Ryan! How do you handle response coherence, especially in cases when the model produces plausible but incorrect answers?
Jessica, ensuring response coherence is vital for ChatGPT's performance. Plausible but incorrect answers are a known challenge. The iterative training process involves extensive validation and continuous feedback to guide the model towards providing more accurate and coherent responses. Post-processing techniques, such as error analysis and model confidence estimation, can also help identify potential inconsistencies.
Ryan, have you encountered any instances where ChatGPT's response confidently aligned with an incorrect measurement standard or ideology? How do you address such scenarios and prevent potential dissemination of inaccurate information?
Jessica, incorrect confident responses can occur, and it's a challenge we actively work to mitigate. Feedback loops with metrology experts and thorough review processes play a crucial role in identifying and correcting inaccuracies. Building mechanisms for users to report potential errors further aids in preventing the dissemination of misleading or incorrect information.
Ryan, do you have any insights into the potential risks associated with relying heavily on ChatGPT for measurement tasks? Is there any possibility of misleading or inaccurate responses that could compromise measurement accuracy?
Michael, relying solely on ChatGPT for measurement tasks can carry certain risks. Inaccurate or misleading responses are possible, especially in complex scenarios where human judgment is typically required. Metrologists should treat ChatGPT as a helpful tool, supporting their expertise rather than replacing it. It's important to establish confidence thresholds and have mechanisms for human verification.
Hi Ryan! I'm interested to know if there are any specific training techniques used to enhance ChatGPT's performance in metrology applications. Are there any unique considerations compared to training for other domains?
Emma, training ChatGPT for metrology applications involves using large datasets of annotated metrology-related conversations. The model is fine-tuned using supervised fine-tuning techniques, where high-quality human-generated responses act as training targets. Some unique considerations include incorporating domain-specific vocabulary and contextual understanding of measurement principles.
Thank you, Ryan! It's interesting how domain-specific vocabulary plays a role in training. In cases where new measurement techniques or concepts emerge, how adaptable is ChatGPT to learn and provide accurate responses?
Emma, ChatGPT can adapt to new measurement techniques or concepts to some extent. However, it heavily relies on the availability of training data that covers those new advancements. Incorporating emerging topics usually requires additional dataset curation and fine-tuning to ensure accurate and up-to-date responses for the evolving metrology landscape.
Hi Ryan! I believe ChatGPT can play a significant role in advancing measurement efficiency. Are there any efforts to make ChatGPT compatible with existing metrology software or systems?
Nathan, integrating ChatGPT with existing metrology software or systems is an ongoing area of exploration. APIs and interfaces can be developed to allow seamless integration, enabling metrologists to access ChatGPT's capabilities within their preferred software ecosystem. However, it requires careful consideration of security, compatibility, and adaptability to different software architectures.
Ryan, how important is natural language understanding for ChatGPT's performance in metrology applications? Are there any specific challenges in this aspect?
Nathan, natural language understanding is crucial for ChatGPT's performance in metrology applications. The model's ability to grasp context, understand nuanced questions, and handle domain-specific jargon significantly impacts its accuracy and relevance. Some challenges include dealing with ambiguities, disambiguation in measurement terminologies, and recognizing implicit assumptions or constraints that may be present in user queries.
Thanks for clarifying, Ryan! With the evolving metrology landscape, is there ongoing research to improve ChatGPT's ability to adapt and stay up-to-date with emerging measurement techniques?
Emma, staying up-to-date with emerging measurement techniques is an active research area. Continual data collection efforts, collaboration with domain experts, and incorporating user feedback are part of the process to improve ChatGPT's ability to handle new developments. It's essential to bridge the gap between research advancements and real-world metrology requirements to ensure timely adaptability.
Ryan, what measures are being taken to ensure user privacy and protection of sensitive measurement data while utilizing ChatGPT?
Emma, user privacy and protection of sensitive measurement data are of utmost importance. Safeguarding measures, such as secure data transmission protocols, data anonymization techniques, and adhering to privacy regulations, are taken into account. Additionally, user consent and explicit permissions are obtained before accessing or interacting with sensitive data to maintain confidentiality and uphold ethical standards.
Hi Ryan! Do you foresee any potential ethical concerns associated with the use of ChatGPT in metrology technology, and how can they be addressed?
Samuel, there are potential ethical concerns surrounding the use of ChatGPT in metrology technology. Addressing them involves transparently communicating the model's limitations and potential risks to users. Implementing mechanisms for flagging inaccuracies and collecting user feedback helps in identifying and mitigating ethical concerns. Regular audits and adherence to ethical frameworks can ensure responsible deployment and use of ChatGPT in metrological workflows.
Thank you for the response, Ryan! It's reassuring to know that ethical considerations are prioritized to ensure responsible adoption of ChatGPT in metrology technology.
Ryan, thank you for highlighting the importance of treating ChatGPT as a supportive tool. What guidelines or cautions should metrologists keep in mind when applying ChatGPT in their work?
Michael, applying ChatGPT as a supportive tool requires careful considerations. Metrologists should take into account the model's confidence levels and establish threshold criteria for accepting its responses. They should also be aware of the limitations and potential risks associated with entirely relying on an AI system. Regularly validating responses and having fallback mechanisms for human judgment can ensure the proper integration of ChatGPT in metrological workflows.