Unlocking the Potential of ChatGPT: Revolutionizing Data Acquisition in Cloud Data Management
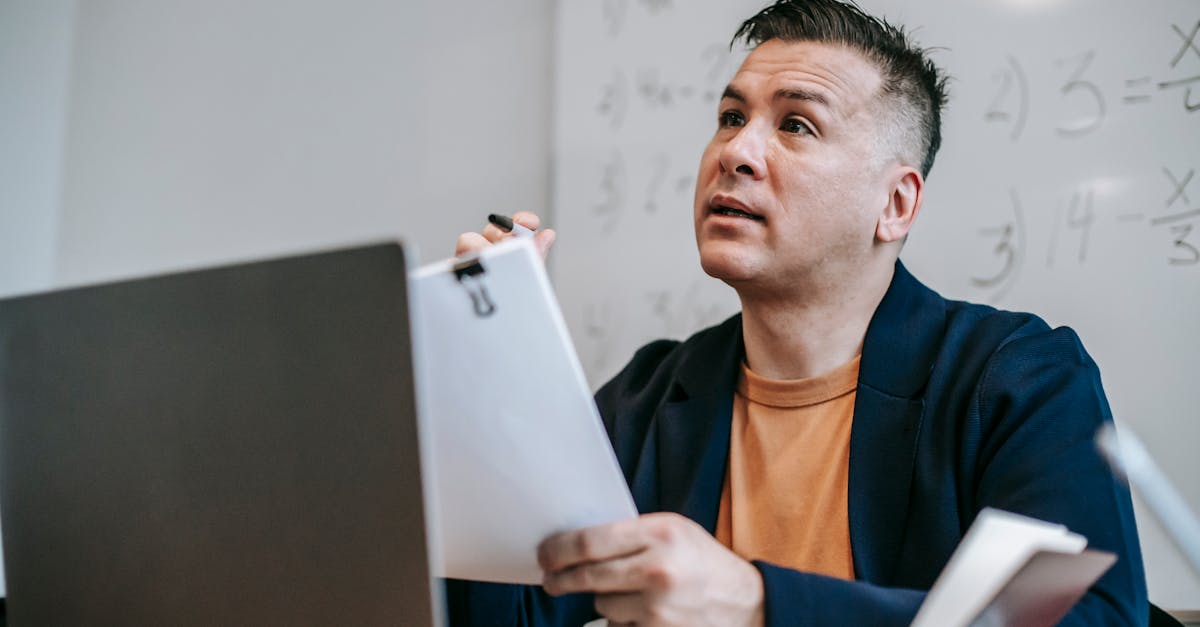
Data acquisition is a crucial aspect of cloud data management, especially when dealing with distributed data across various cloud environments. In this article, we will explore the role of data acquisition in maintaining and monitoring data in the cloud.
What is Data Acquisition?
Data acquisition refers to the process of collecting and acquiring data from various sources. It involves capturing raw data and transforming it into a usable format for analysis and storage. In the context of cloud data management, data acquisition plays a vital role in ensuring that data from different cloud environments is collected efficiently and accurately.
The Importance of Data Acquisition in Cloud Data Management
Cloud data management involves handling data distributed across multiple cloud environments, such as public, private, or hybrid clouds. It is essential to have a robust data acquisition system in place to effectively manage and monitor this data. Here are a few reasons why data acquisition is crucial in cloud data management:
- Data Consistency: Cloud data management requires maintaining data consistency across different cloud environments. Data acquisition ensures that the data collected from these environments is synchronized and up-to-date.
- Data Integration: Data acquisition enables the integration of data from various cloud environments into a unified system. This integration allows for better data analysis and decision-making.
- Data Quality: Acquiring data from different sources can introduce data quality issues. An effective data acquisition system includes data validation and cleansing processes to ensure the quality of the collected data.
- Data Security: With data distributed across different cloud environments, data acquisition includes security measures to protect the data during the collection process. This involves implementing encryption, access controls, and other security protocols.
Data Acquisition Techniques for Cloud Data Management
There are various techniques and technologies available for data acquisition in cloud data management. Some of the commonly used techniques include:
- API Integration: Cloud providers often offer APIs (Application Programming Interfaces) to access and retrieve data from their services. Data acquisition can be achieved by integrating these APIs into a centralized data management system.
- Data Replication: Replicating data from different cloud environments to a central database or data warehouse allows for easier data acquisition and management.
- Data Streaming: Data streaming technologies enable real-time data acquisition from cloud environments. This is particularly useful for monitoring and analyzing streaming data sources such as IoT devices or social media feeds.
- ETL (Extract, Transform, Load): ETL processes involve extracting data from various sources, transforming it into a standardized format, and loading it into a target system. ETL tools can be utilized for data acquisition in cloud data management.
Conclusion
Data acquisition is a critical component of cloud data management, particularly when dealing with distributed data across multiple cloud environments. By effectively acquiring and integrating data from various sources, cloud data management systems can ensure data consistency, integration, quality, and security. Various techniques such as API integration, data replication, data streaming, and ETL processes can be employed to achieve efficient data acquisition in cloud data management.
Comments:
This article on unlocking the potential of ChatGPT is really fascinating! It's amazing to see how AI technology is revolutionizing data acquisition in cloud data management.
I agree, Alice! The advancements in natural language processing are opening up new possibilities for managing and analyzing large amounts of data.
Absolutely! ChatGPT seems like a game-changer. I can see it greatly improving the efficiency and accuracy of data acquisition tasks.
This technology sounds promising, but I wonder about the potential ethical concerns surrounding AI-powered data acquisition. How can we ensure data privacy and prevent misuse?
Valid point, Adam. Ethical considerations are crucial when it comes to AI and data management. It's important to have proper regulations and measures in place to address privacy and security issues.
Thank you for raising this concern, Adam. Ensuring ethical use of AI technology is indeed essential. In the context of ChatGPT, it's crucial to adhere to strict privacy protocols and data usage guidelines.
I'm excited about the potential of ChatGPT in improving data acquisition processes. It could drastically reduce the manual effort involved in extracting information from various sources.
Exactly, Jennifer! ChatGPT's ability to understand and generate human-like responses can significantly enhance data acquisition efficiency.
I can see ChatGPT becoming an invaluable tool for organizations dealing with vast amounts of data. It'll save time and resources by automating data acquisition tasks.
While ChatGPT shows promise, I wonder how it handles complex and domain-specific queries. Can it adapt well to different industries and specific use cases?
Great question, Robert. ChatGPT is designed to be flexible and adaptable. It can be fine-tuned and trained on specific domains to address industry-specific data acquisition needs.
I'm curious about the potential limitations of ChatGPT. Are there any scenarios where it may struggle or produce inaccurate results?
That's a valid concern, Jessica. While ChatGPT is impressive, it still has limitations. In certain cases, it might generate incorrect responses or struggle with ambiguous queries.
You're right, Jessica. ChatGPT, like any AI model, has limitations. It's crucial to carefully evaluate and validate its responses, especially in critical data acquisition scenarios.
The potential of ChatGPT is immense, but I'm also concerned about the bias that might be ingrained within its training data. How can we ensure fair and unbiased data acquisition?
I share your concern, William. Bias in AI can have far-reaching consequences. It's crucial to have diverse and representative training data to minimize any biases in ChatGPT's responses.
Addressing bias is of utmost importance, William. It requires meticulous dataset curation and ongoing monitoring to minimize unfairness and ensure fair data acquisition practices.
The potential benefits of ChatGPT are evident, but what about its computational requirements? Are there any significant infrastructure considerations for implementation?
Good point, Oliver. Implementing ChatGPT effectively would require robust computational resources, such as powerful servers or cloud infrastructure, to handle the processing demands.
Indeed, Oliver. ChatGPT can be resource-intensive, especially for large-scale data acquisition. Addressing the computational requirements is a crucial aspect of successful implementation.
ChatGPT has great potential, but what are the potential security risks associated with using an AI-driven system for data acquisition? How is data integrity ensured?
Excellent question, Charles. Security is paramount in data acquisition. Implementing robust access controls, encryption, and regular security audits can help safeguard data integrity.
You're right, Charles. Security measures are crucial in an AI-driven data acquisition system. Regular vulnerability assessments and ensuring data encryption are essential to protect data integrity.
I wonder how interactive and customizable ChatGPT can be. Can it be easily tailored to specific business needs?
That's a valid concern, John. ChatGPT can be fine-tuned and customized for specific business needs, making it more interactive and aligned with specific requirements.
Absolutely, John. ChatGPT is designed to be customizable. With the right training and fine-tuning, it can be tailored to specific business contexts, improving interactivity.
I'm curious to know about the training data sources used for ChatGPT. How diverse and reliable are the sources to ensure accurate data acquisition?
Good question, Elizabeth. Training data diversity is essential to generalize ChatGPT's knowledge. Using diverse and reliable sources helps improve the accuracy of data acquisition.
Excellent point, Elizabeth. Training data for ChatGPT should be diverse and reliable, representing a wide range of information sources to enhance the accuracy of data acquisition.
ChatGPT certainly has impressive potential. How well does it handle unstructured or messy data? Can it extract meaningful insights from such data?
That's a good question, Anthony. While ChatGPT can handle a variety of data types, unstructured or messy data may present challenges in extracting accurate insights.
You're right, Anthony. Unstructured or messy data can pose challenges, but ChatGPT can still extract valuable insights. Preprocessing and data cleaning can help improve its performance.
The potential of AI in data acquisition is exciting. However, how can we ensure transparency and interpretability of the decisions made by ChatGPT during the process?
Good question, Henry. Ensuring transparency is crucial, especially in critical decision-making scenarios. Techniques like explainable AI can help shed light on ChatGPT's decisions.
Transparency is indeed important, Henry. Making AI systems like ChatGPT more interpretable through techniques like explainability can enhance user trust and facilitate understanding.
ChatGPT shows great potential, but how user-friendly is it for non-technical users who require data acquisition capabilities without extensive coding knowledge?
Good question, David. User-friendliness is important for wider adoption. ChatGPT can be made more accessible through intuitive interfaces and simplified workflows for non-technical users.
Indeed, David. Making ChatGPT more user-friendly by providing user-friendly interfaces and minimizing the need for extensive coding knowledge is crucial for wider usability.
I see the potential of ChatGPT, but how does it handle language nuances and context? Can it accurately interpret user queries in various languages?
Great question, Andrew. ChatGPT can handle language nuances to some extent, but its accuracy may vary for languages with significantly different linguistic structures.
Language nuances and context are indeed important, Andrew. While ChatGPT can handle multiple languages, ensuring accuracy may require additional fine-tuning and training for specific languages.
ChatGPT seems promising, but what are the potential bottlenecks or challenges in implementing and scaling it for large-scale data acquisition operations?
Good question, Richard. Implementing and scaling ChatGPT can indeed present challenges, such as high computational requirements and managing increased data volume.
You're right, Richard. Scaling and managing ChatGPT for large-scale data acquisition require careful infrastructure planning, resource allocation, and performance optimization.
The potential of ChatGPT to revolutionize data acquisition is exciting. What are the potential use cases and industries that can benefit the most from its applications?
That's a great question, Peter. ChatGPT can be applicable across various industries, including customer support, market research, content generation, and data analysis.
Absolutely, Peter. ChatGPT's potential use cases are diverse, spanning industries like healthcare, finance, e-commerce, and many more, where efficient data acquisition plays a vital role.
The potential of ChatGPT is impressive, but what are the potential limitations in terms of scale? Can it handle extremely large datasets efficiently?
Good question, Sarah. While ChatGPT can handle large datasets, processing extremely large volumes efficiently may require specialized distributed computing architectures.
You're right, Sarah. Efficient handling of extremely large datasets is a valid consideration. Techniques like parallel processing can help improve ChatGPT's scalability in such cases.