Unlocking the Power of Big Data Analytics: Leveraging ChatGPT for Advanced Data Acquisition
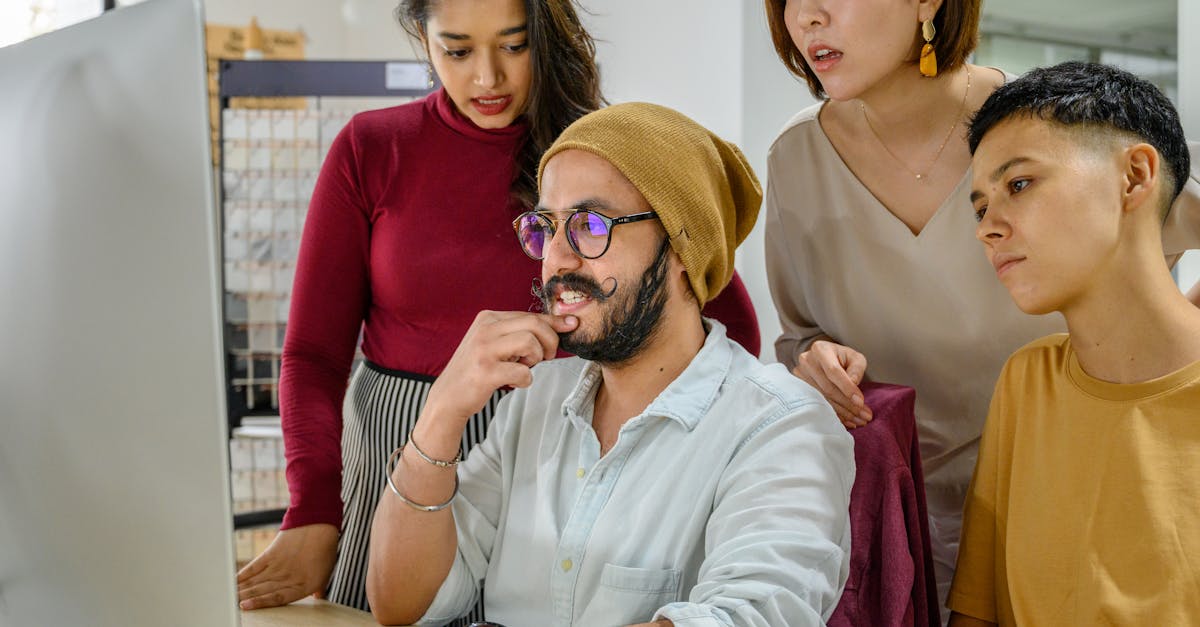
In today's digital age, the amount of data generated and collected is continuously increasing. Whether it's from online transactions, social media, sensors, or any other source, organizations are constantly looking for ways to extract valuable insights from these vast volumes of information. This is where data acquisition, especially in the context of big data analytics, plays a crucial role.
Data acquisition, at its core, refers to the process of collecting data from various sources and storing it in a central location for further analysis. In the realm of big data analytics, this process becomes even more vital as it involves gathering and interpreting large volumes of data to uncover hidden patterns and correlations that can drive informed decision-making.
There are several technologies and tools available for data acquisition in big data analytics. One of the most commonly used technologies is Extract, Transform, Load (ETL). ETL enables organizations to extract data from various sources, transform it into a standardized format, and load it into a data warehouse or data lake for analysis. ETL processes are designed to handle high volumes of data efficiently and accurately.
Another technology widely used in data acquisition is real-time stream processing. This approach involves capturing data in real-time as it is generated and processing it on the fly. Stream processing technologies enable organizations to analyze and make decisions based on up-to-date information, which is especially useful in dynamic environments where instant decision-making is required.
Once the data is acquired, it can be used for a wide range of analytical purposes. Big data analytics can help organizations identify trends, make predictions, and gain actionable insights. For example, in the healthcare industry, data acquisition and analysis can be used to predict disease outbreaks, optimize treatment plans, and improve patient outcomes. In the retail industry, data acquisition can help identify customer preferences and behaviors, enabling personalized marketing campaigns and enhancing customer satisfaction.
The usage of data acquisition for big data analytics extends beyond specific industries. It is relevant to any organization that deals with large volumes of data and aims to gain a competitive advantage through data-driven decision-making. By acquiring and analyzing data effectively, organizations can uncover hidden patterns and correlations that were once impossible to identify, leading to improved operational efficiency, cost savings, and innovation.
Data Acquisition Best Practices
While data acquisition can be a complex process, there are several best practices that organizations can follow to ensure successful implementation:
- Identify relevant data sources: Clearly identify the sources of data that are most relevant to your organization's objectives. This could include internal databases, external APIs, social media platforms, or any other sources that contain valuable information.
- Establish data quality standards: Accurate and reliable data is crucial for meaningful analysis. Define data quality standards and implement processes to ensure that the acquired data meets these standards.
- Implement robust data integration: Data integration is an essential part of the data acquisition process. Choose suitable integration technologies and tools that can handle the required data volumes and provide seamless connectivity between different systems.
- Ensure data security and compliance: With the increasing emphasis on privacy and data protection, organizations must establish robust security measures to protect acquired data from unauthorized access. Comply with relevant regulations and industry standards to avoid legal and ethical issues.
- Leverage automation and artificial intelligence: To handle large volumes of data efficiently, consider leveraging automation and AI technologies. These technologies can help streamline the data acquisition process, reduce manual effort, and improve accuracy.
- Continuously monitor and optimize: Data acquisition is an ongoing process. Continuously monitor the performance of data acquisition systems and make necessary optimizations to ensure timely and accurate data acquisition.
Comments:
Thank you all for your comments! I'm glad to see the discussion going. If anyone has any questions or would like further clarification on any aspect of the article, feel free to ask!
Great article, Maureen! I found it really insightful how you discussed leveraging ChatGPT for advanced data acquisition. It's amazing to see the potential of big data analytics in action.
I agree, Paul! The possibilities with big data analytics are truly endless. Maureen, could you provide some examples of industries that can benefit the most from leveraging ChatGPT for data acquisition?
Thank you, Paul and Emily! Industries such as customer service, market research, and content moderation can benefit greatly from leveraging ChatGPT for data acquisition. Its ability to analyze and extract insights from large amounts of unstructured data makes it a powerful tool.
I enjoyed reading your article, Maureen. Big data analytics has definitely revolutionized the way we extract value from data. ChatGPT seems like a great addition to the analytics toolkit!
I'm familiar with big data analytics, but can you explain how ChatGPT specifically aids in data acquisition? How does it differ from traditional methods?
Hi Nina, great question! ChatGPT aids in data acquisition by engaging with users in conversational interactions. It can extract insights from these interactions and help gather valuable data that might not be accessible through traditional methods. It enables a more interactive and dynamic approach to data acquisition.
Thanks for the explanation, Maureen! It seems like ChatGPT brings a more human-like element to data acquisition. I can see how that would enable a deeper understanding of user needs and preferences.
Maureen, I appreciate your article on leveraging ChatGPT for data acquisition. I'm curious about the potential limitations of using such technology in practice. Could you shed some light on that?
Certainly, Sophia! While ChatGPT has immense potential, it does have some limitations. One challenge is the possibility of generating biased or incorrect responses based on the training data it receives. Proper data curation and human oversight are essential to address these concerns.
Maureen, thank you for the informative article! I'm curious if ChatGPT can handle highly technical or domain-specific conversations, like in the field of medicine or engineering.
You're welcome, Oliver! ChatGPT can handle a wide range of subjects, including technical domains. However, it's worth noting that it performs best when the conversation is aligned with the data it was trained on. Fine-tuning the model on specific domains can further enhance its performance in those areas.
As a data scientist, I can see the enormous potential of ChatGPT for data acquisition. The ability to extract insights from conversational interactions opens up new possibilities. Maureen, do you have any personal experiences or success stories with using ChatGPT for data acquisition?
Hi Rebecca! Yes, I've personally worked on a project where ChatGPT was used for data acquisition in the customer service industry. By engaging with users via chat, we collected valuable feedback and insights that shaped our decision-making process. It helped improve our products and services based on real-time customer interactions.
Maureen, your article highlights the power of big data analytics and how ChatGPT plays a role in it. However, have you come across any challenges in implementing ChatGPT for data acquisition?
Absolutely, Matthew! One of the challenges we faced initially was ensuring the AI model's responses were accurate and aligned with our intended goals. Continuous monitoring, feedback loops, and iterating on the training process helped address these challenges.
Hi Maureen, great article! I can see the potential of ChatGPT. However, I'm concerned about privacy and security in using conversational AI for data acquisition. How can we ensure user data is protected?
Hi Catherine! Privacy and security are indeed crucial aspects. When leveraging ChatGPT for data acquisition, it's important to follow privacy guidelines, anonymize user data whenever possible, and obtain proper user consent. Implementing strong encryption and secure storage practices also help protect sensitive information.
Maureen, I found your article very informative. Do you think the future of data acquisition heavily relies on AI technologies like ChatGPT, and if so, what developments can we expect to see?
Thank you, Liam! AI technologies like ChatGPT certainly have a significant role in the future of data acquisition. We can expect advancements in natural language understanding, improved domain-specific conversational capabilities, and increased customization options. AI will continue to enhance and streamline the data acquisition process.
Great article, Maureen! Data acquisition is crucial for many businesses. I'm curious, how scalable is the use of ChatGPT for data acquisition? Can it handle large volumes of conversations?
Hi Daniel! ChatGPT is designed to handle large volumes of conversations. Its scalability depends on factors like computational resources and response time requirements. By optimizing infrastructure and utilizing technologies like distributed computing, it can effectively scale to accommodate high conversation volumes.
Maureen, great article! I can see the potential of ChatGPT. However, are there any ethical considerations when using it for data acquisition? How can we ensure responsible and unbiased data collection?
Thank you, Sophie! Ethical considerations are crucial. It's important to train AI models like ChatGPT on diverse and representative datasets to avoid bias. Transparent and accountable data acquisition practices, along with regular audits, can help ensure responsible and unbiased data collection.
Maureen, in your opinion, what are the key advantages of using ChatGPT for data acquisition compared to other methods?
Hi Ethan! One key advantage of using ChatGPT for data acquisition is its ability to engage users in a conversational manner. This allows for more contextual and interactive data collection compared to traditional methods. Additionally, its natural language capabilities enable the extraction of nuanced insights from unstructured conversational data.
Maureen, I found your article fascinating! Considering the rapid advancement in AI technologies, how do you foresee ChatGPT evolving in the future?
Thank you, Hannah! In the future, we can expect ChatGPT to enhance its understanding of specialized domains, become more adept at handling complex and nuanced conversations, and improve its ability to ask clarifying questions when faced with ambiguous queries. AI capabilities will continue to evolve, opening up new possibilities.
Maureen, your article shed light on the potential of ChatGPT for data acquisition. However, what precautions do we need to take to combat potential misuse of such technology?
Hi Grace! To combat the potential misuse of ChatGPT or any AI technology, it's essential to have robust ethical guidelines and governance frameworks in place. Clear policies, user consent mechanisms, and regular audits can help ensure responsible use and minimize the risk of harmful or unethical applications.
Maureen, I enjoyed reading your article. How do you envision the integration of ChatGPT with other data analytics tools to further unlock the potential of data acquisition?
Thank you, Lucas! Integrating ChatGPT with other data analytics tools can further enhance the data acquisition process. By combining its conversational capabilities with techniques like sentiment analysis, topic modeling, or network analysis, we can gain deeper insights and uncover hidden patterns within conversational data.
Maureen, your article emphasizes the power of big data analytics and how ChatGPT contributes to data acquisition. What are the factors to consider when selecting the right conversational AI model for a specific use case?
Hi David! When selecting a conversational AI model like ChatGPT, factors to consider include the model's performance on relevant tasks, its domain-specific capabilities, the availability of data in the desired domain, as well as computational resources required for training and deployment. Evaluating these factors helps in choosing the right model for a specific use case.
Maureen, I found your article very informative. In terms of scalability, is ChatGPT capable of handling different languages and multiple regions effectively?
Thank you, Amy! Yes, ChatGPT is capable of handling different languages and multiple regions. By training the model on diverse and multilingual datasets, it can effectively understand and generate responses in various languages. Regional adaptations can further enhance its performance for specific dialects and cultural contexts.
Maureen, your article highlighted the potential of ChatGPT for data acquisition. What are the significant challenges you foresee in implementing ChatGPT at an organizational level?
Hi Mia! One of the major challenges in implementing ChatGPT at an organizational level is the need for large amounts of high-quality training data. Acquiring and curating such data can be resource-intensive. Additionally, ensuring appropriate infrastructure, continuous monitoring, and addressing potential biases are also crucial considerations.
Maureen, your article provides valuable insights into ChatGPT for data acquisition. How do you see AI technologies like ChatGPT shaping the future of analytics?
Thank you, Olivia! AI technologies like ChatGPT will play a significant role in shaping the future of analytics. They will enable more efficient data acquisition, uncover deeper insights, and facilitate real-time decision-making. With ongoing advancements, AI will become an integral part of analytics, driving innovation and transformation in numerous industries.
Maureen, great article! What steps should organizations take to ensure effective implementation and adoption of ChatGPT for data acquisition?
Hi Joshua! Effective implementation and adoption of ChatGPT for data acquisition require careful planning. Organizations should start with pilot projects, defining clear objectives, and identifying suitable use cases. Ensuring proper training and awareness among users, as well as having monitoring mechanisms in place, can help drive successful implementation and adoption.
Maureen, I found your article on ChatGPT for data acquisition very informative. How do you see such AI technologies advancing in the coming years?
Thank you, Isabella! In the coming years, AI technologies like ChatGPT will advance in various aspects. We can expect improved contextual understanding, more precise and accurate responses, better handling of complex questions, and increased integration with other tools and platforms, making them even more powerful and versatile for data acquisition.
Maureen, your article sheds light on the potential of ChatGPT for data acquisition. Could you share any notable real-world examples where ChatGPT has been successfully utilized?
Hi Noah! One notable example of successful ChatGPT utilization is OpenAI's use of similar models to improve customer support. By leveraging interactive conversations, valuable insights were gathered to enhance user experiences and identify pain points. Many other industries are also adopting ChatGPT for data acquisition, such as e-commerce, healthcare, and finance.
Maureen, your article delves into the potential of ChatGPT for advanced data acquisition. How do you see the synergy between AI technologies and human experts in this field?
Thank you, Sarah! The synergy between AI technologies like ChatGPT and human experts is crucial. While AI models can provide efficient data acquisition and insights, human experts play a significant role in data curation, validation, and ensuring ethical practices. The collaboration of AI and human expertise leads to more accurate, unbiased, and valuable data acquisition.
Maureen, your article provides a comprehensive overview of ChatGPT for data acquisition. What are the key factors to consider when evaluating the success of implementing ChatGPT in a specific use case?
Hi Samuel! When evaluating the success of implementing ChatGPT, key factors to consider include the quality and relevance of obtained data, improvements in decision-making or business outcomes, user satisfaction, and reduction in data acquisition costs or time. Evaluating these factors against predefined objectives can help measure the success of implementing ChatGPT in a specific use case.