Unlocking the Power of ChatGPT for Predictive Maintenance in Broadcast Engineering Technology
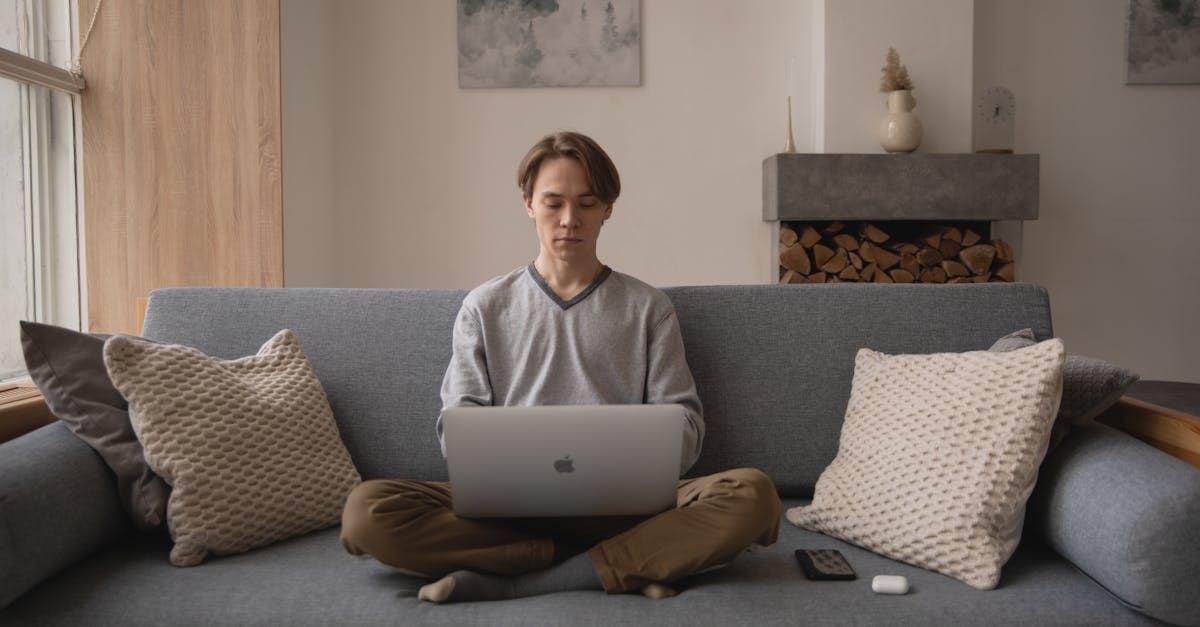
Introduction
Predictive maintenance is an essential component of broadcast engineering, aimed at identifying and addressing equipment failures before they impact the broadcast. As technology continues to evolve and become an integral part of the broadcasting industry, the need for accurate and timely predictive maintenance solutions grows.
Understanding Predictive Maintenance
Predictive maintenance involves the use of advanced data analytics and machine learning algorithms to analyze real-time and historical equipment data. By monitoring key parameters such as temperature, pressure, vibration, and more, broadcasters can predict faults and failures, allowing them to perform proactive maintenance and avoid unexpected downtime.
Benefits of Predictive Maintenance
Implementing predictive maintenance in the broadcast industry offers several advantages:
- Reduced Downtime: Predictive maintenance helps minimize unplanned outages by addressing equipment issues proactively. By identifying potential failures in advance, broadcasters can schedule maintenance activities during planned downtime, ensuring uninterrupted broadcasts.
- Cost Savings: By preventing major equipment failures, broadcasters can avoid expensive repairs or replacements. Additionally, predictive maintenance allows for more efficient use of resources as maintenance activities are planned and scheduled based on actual equipment conditions.
- Improved Operational Efficiency: Predictive maintenance enables broadcasters to optimize their operations by ensuring equipment reliability and availability. By identifying equipment defects early, preventive measures can be taken, resulting in smoother broadcasting operations.
Predictive Maintenance Technologies
Several technologies are employed in predictive maintenance for broadcast engineering:
- Sensor Technology: Sensors are used to measure and monitor various equipment parameters, allowing broadcasters to collect data for analysis. These sensors can be attached to different parts of the broadcasting equipment to monitor performance and detect anomalies.
- Big Data Analytics: Advanced analytics techniques are employed to process the vast amount of data collected from sensors. By analyzing patterns and trends, predictive maintenance algorithms can identify potential failures and generate early warnings or notifications.
- Machine Learning: Machine learning algorithms enable predictive maintenance systems to learn from historical data and continuously improve their predictive capabilities. These algorithms can predict equipment failures with higher accuracy as they gather more data over time.
Current Usage of Predictive Maintenance in Broadcasting
Today, many broadcasters are adopting predictive maintenance strategies to enhance their operational efficiency and reduce downtime. By leveraging real-time data and predictive analytics, they can:
- Detect Anomalies: Predictive maintenance systems can detect abnormal equipment behavior and trigger alerts to notify technicians. This early detection allows for prompt intervention before the issue escalates.
- Optimize Maintenance Schedules: By analyzing equipment data and performance trends, broadcasters can optimize their maintenance schedules, ensuring that maintenance activities are carried out at the most opportune times, minimizing disruption to live broadcasts.
- Preventive Repairs: By identifying potential failures in advance, broadcasters can proactively address equipment issues through preventive repairs or part replacements. This reduces the risk of sudden equipment failures during live broadcasts.
Conclusion
Predictive maintenance plays a vital role in the broadcast engineering industry, helping broadcasters operate with greater efficiency, minimize downtime, and reduce costs. By leveraging advanced technologies and data analytics, predictive maintenance enables broadcasters to detect equipment failures before they impact the broadcast, ensuring uninterrupted transmission and optimal performance.
Comments:
Thank you all for your interest in this article. I'm excited to discuss the potential of ChatGPT for predictive maintenance in broadcast engineering technology.
This is an incredibly interesting topic! I can see how leveraging ChatGPT could improve maintenance efficiency in the broadcast industry. Have there been any practical implementations so far?
I've come across a couple of case studies where ChatGPT was used for predictive maintenance in broadcast engineering. It helped identify potential faults in equipment before they caused major issues.
That's impressive, Stephen! Do you know what kind of data inputs ChatGPT relies on to make accurate predictions?
Emily, ChatGPT requires historical equipment performance data and maintenance logs to learn patterns and predict potential failures.
I'm curious about the limitations of ChatGPT in this context. Can it handle the complexity of broadcast engineering systems?
Michael, while ChatGPT has shown promise, it does have limitations. It may struggle with rare or unprecedented failure scenarios where there isn't enough past data to rely on.
That's an important point, Lisa. It's crucial to have a combination of AI-driven predictive models like ChatGPT and domain experts to address such scenarios.
I'm wondering if ChatGPT can be applied to optimize broadcast workflows as well. Any thoughts on that?
Tom, absolutely! ChatGPT can help identify bottlenecks, propose workflow improvements, and even suggest optimal scheduling for maintenance routines.
I agree, Lisa! With its ability to learn from historical data, ChatGPT can provide valuable insights to optimize broadcast workflows.
Dan, congratulations on an informative article! I can see how ChatGPT can revolutionize predictive maintenance in broadcast engineering.
Thank you, John! It's exciting to witness the potential impact of ChatGPT on improving maintenance practices in the broadcast industry.
This article highlights an exciting use case of AI in broadcast engineering. I'm looking forward to seeing more practical implementations of ChatGPT in this field.
David, the potential of ChatGPT in predictive maintenance for broadcast engineering is indeed promising. Practical implementations will surely bring further advancements.
As someone new to the field, this article gave me insights into an innovative approach. It's great to see how AI can revolutionize maintenance practices in broadcast engineering.
Absolutely, Jessica! The application of ChatGPT in this context opens up new possibilities and enhances the efficiency of maintenance operations.
The use of AI in predictive maintenance is fascinating. How scalable is ChatGPT when dealing with large-scale broadcast systems?
John, ChatGPT can be scaled to handle large-scale systems, but it's crucial to ensure a robust infrastructure to support the increased computational demands.
That's a valid point, Emily. Scalability is crucial, especially when dealing with complex broadcast systems with multiple components.
Thanks for the insights, Lisa and Emily! It's important to set the right expectations when implementing ChatGPT for predictive maintenance.
This sounds like a great application of AI in the broadcast industry. Can ChatGPT also aid in reducing maintenance costs?
Emma, definitely! By identifying potential failures in advance, ChatGPT can help minimize downtime, reduce repair costs, and optimize maintenance schedules.
Reducing maintenance costs is always a priority. ChatGPT's predictive capabilities can contribute to more efficient allocation of resources.
Great article! I'm curious about the level of accuracy ChatGPT achieves in predicting maintenance issues. Can it achieve high accuracy?
Olivia, ChatGPT achieves good accuracy when trained on comprehensive and relevant historical data. However, it's important to validate its predictions against real-world insights.
Validation is essential to ensure the reliability of ChatGPT's predictions. Combining AI models with human expertise in the verification process can further enhance accuracy.
I find the application of ChatGPT in broadcast engineering fascinating. Are there any potential ethical concerns to address?
Sophia, ethical concerns can arise, especially when it comes to data privacy and bias in models. Proactive steps should be taken to address these concerns and ensure fairness.
I completely agree, Emily. AI algorithms like ChatGPT need to be designed and implemented with careful consideration of ethical implications.
Lisa, you mentioned ChatGPT's limitations earlier. In your opinion, what other challenges should be considered before adopting it for predictive maintenance?
Olivia, apart from limitations in handling rare scenarios, the interpretability of ChatGPT's decisions and its integration with existing infrastructure are key challenges to address.
I agree, Lisa. Interpreting and explaining the decisions made by AI models like ChatGPT is crucial to gain trust and ensure system reliability.
This article highlights the potential of AI in transforming predictive maintenance tasks. I'm excited to see how ChatGPT further evolves in this field.
Daniel, the field of predictive maintenance is constantly evolving, and ChatGPT's evolution holds promise for addressing the challenges in this domain.
As a broadcast engineer, I'm excited about the possibilities ChatGPT brings. Its predictive abilities can greatly impact maintenance efficiency.
Alexandra, ChatGPT indeed has the potential to optimize maintenance routines and improve the overall operational efficiency in broadcast engineering.
I couldn't agree more, David! The practical implementation of ChatGPT in broadcast engineering will provide valuable insights and enhance system reliability.
This article sparked my interest in predictive maintenance. Are there any specific prerequisites to implement ChatGPT successfully in this domain?
Henry, having a well-maintained equipment database, access to historical data, and a collaborative approach between AI experts and maintenance personnel are important prerequisites.
Collaboration between various stakeholders is indeed critical, Henry. It ensures effective implementation and continuous improvement of ChatGPT's predictive maintenance capabilities.
This article sheds light on the innovative application of AI in broadcast engineering maintenance. Looking forward to more advancements in this area.
Richard, the application of AI in predictive maintenance is an exciting field with immense potential. Continued advancements will undoubtedly lead to significant improvements.
As an AI enthusiast, I'm intrigued by the possibilities ChatGPT offers for predictive maintenance. I'm excited to see how it can be integrated into real-world broadcast systems.
Rebecca, the integration of ChatGPT into real-world broadcast systems holds great promise for improving maintenance practices and operational efficiencies.
This article presents a compelling argument for integrating ChatGPT into broadcast engineering maintenance. It's refreshing to see AI-driven advancements in this field.
William, indeed! Integrating AI models like ChatGPT can revolutionize maintenance practices in the dynamic and evolving field of broadcast engineering.
Exciting times lie ahead for the broadcast engineering industry! The potential impact of ChatGPT on maintenance practices is promising.
Indeed, Emily! By leveraging AI-driven predictive models, broadcast engineering can enhance operational efficiency and minimize costly downtimes.
This article showcases an intriguing application of AI in broadcast engineering. It's exciting to witness the advancements in this field.
Laura, the advancements in AI, particularly in predictive maintenance, hold immense potential to revolutionize the broadcast engineering landscape.
Exactly, Stephen! These advancements bring new opportunities for optimizing maintenance operations and delivering high-quality broadcast services.
Thank you, Dan, for sharing this insightful article! It's impressive to see the impact AI can have on predictive maintenance in broadcast engineering.
Michael, thank you for your kind words! The potential of ChatGPT in this field is indeed exciting, and I'm glad you found the article insightful.