Unlocking the Power of Delegation: Leveraging ChatGPT for Advanced Data Analysis in the Digital Age
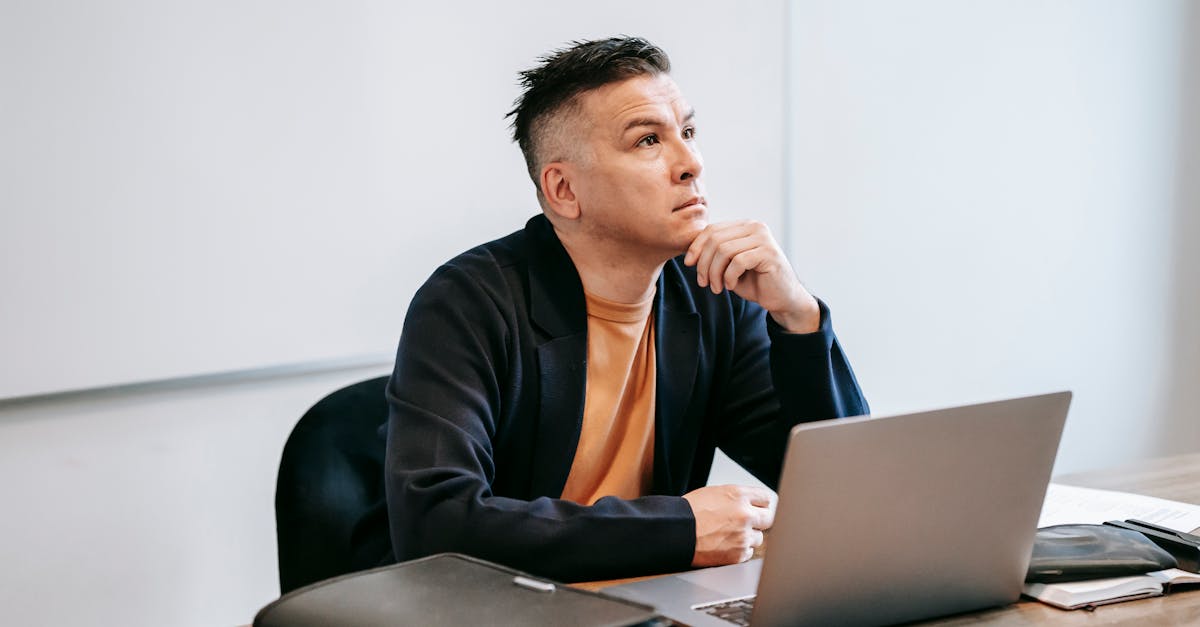
In the field of data analysis, one important technology that has gained significant attention in recent years is delegation. Delegation refers to the process of assigning tasks and responsibilities to automated systems or algorithms to assist analysts in their work. This technology has revolutionized the way data is collected, interpreted, insights are generated, and reports are built.
Automating the Data Collection Process
One of the primary areas where delegation has been utilized is in automating the data collection process. Traditionally, data collection was a labor-intensive task that involved manual extraction and transformation of data from multiple sources. With delegation, analysts can now delegate this task to automated systems that can efficiently collect data from various sources in real-time, saving time and reducing errors.
Automated systems can also streamline the data cleansing process by identifying and removing duplicate or irrelevant data points. This ensures that the data used for analysis is accurate and reliable. Delegation allows analysts to focus on higher-value tasks, such as analyzing the data and extracting meaningful insights.
Generating Insights and Building Reports
Delegation has also enabled analysts to efficiently generate insights from large volumes of data. With the help of machine learning algorithms and advanced analytical techniques, automated systems can identify patterns, trends, and correlations within the data that may not be easily recognizable by analysts manually. These insights can be crucial in making informed business decisions.
Furthermore, delegation can assist in building comprehensive reports that present data analysis results in a clear and concise manner. Automated systems can create visually appealing dashboards and charts that provide a holistic view of the data. This allows analysts to communicate their findings effectively to stakeholders, facilitating better decision-making at all levels of the organization.
Benefits and Challenges of Delegation in Data Analysis
The use of delegation in data analysis brings several benefits to analysts and organizations. Firstly, it improves efficiency by automating time-consuming tasks, allowing analysts to focus on higher-value activities. Secondly, it enhances accuracy by reducing the probability of manual errors during data collection and analysis. Finally, it enables scalability, as automated systems can handle large volumes of data efficiently.
However, the adoption of delegation in data analysis also poses certain challenges. Analysts need to ensure that the automated systems are adequately trained and calibrated to produce accurate insights. Additionally, concerns regarding data privacy and security should be addressed to protect sensitive information from unauthorized access.
Conclusion
Delegation is a powerful technology that has transformed the field of data analysis. By automating data collection, interpretation, generating insights, and building reports, delegation has improved efficiency, accuracy, and scalability. However, its successful implementation requires careful training and calibration of automated systems, as well as addressing privacy and security concerns.
As the field of data analysis continues to evolve, delegation is expected to play an increasingly crucial role in assisting analysts and organizations in extracting valuable insights from the ever-growing amount of data available.
Comments:
Thank you all for taking the time to read my article on unlocking the power of delegation and leveraging ChatGPT for advanced data analysis in the digital age. I'm excited to hear your thoughts and discuss further!
Great article, Timothy! I completely agree that delegation can be a powerful tool in today's digital age. With the increasing complexity of data analysis, leveraging AI models like ChatGPT can certainly help in speeding up the process. It's a game-changer!
Thank you, Sarah! I appreciate your positive feedback. Indeed, AI models like ChatGPT can substantially accelerate data analysis, allowing professionals to focus on higher-value tasks. It's wonderful to witness such advancements!
While I agree that AI models like ChatGPT can be useful for data analysis, doesn't it also raise concerns about accuracy? How can we ensure that the AI-generated insights are reliable?
Valid point, Jake! Ensuring the accuracy of AI-generated insights is crucial. Although AI models have made significant progress, it's still important for human experts to review and validate the outputs. Combining AI capabilities with human expertise can lead to more reliable results.
I find the idea of leveraging ChatGPT for data analysis intriguing. It could potentially save a lot of time. But is it suitable for all types of data analysis tasks, or are there specific areas where it shines?
Great question, Emily! While ChatGPT can be helpful in many data analysis tasks, its strength lies in tasks that involve understanding and generating human language. It excels in tasks like summarization, natural language processing, and contextual understanding. Domain-specific models might be more appropriate for specialized analyses in certain fields.
I'm concerned about the potential job displacement caused by delegating data analysis to AI models. Are there any ethical considerations we need to address?
Ethical concerns are definitely worth discussing, Ben. While AI models can automate certain tasks, they are tools that can assist analysts, not fully replace them. It's important to ensure appropriate use of AI, provide training opportunities for upskilling, and consider potential societal impacts during this transition.
I've used ChatGPT for data analysis, and it's impressive how it can generate insights from complex datasets. However, I've also faced challenges in interpreting the AI-generated results accurately. Any tips on overcoming this?
That's a valid concern, Linda. Interpreting AI-generated results can indeed be challenging. It's important to validate the outputs with other data sources, conduct thorough sensitivity analyses, and involve domain experts in reviewing and interpreting the insights. A collaborative approach can help in overcoming these challenges.
I believe leveraging AI models like ChatGPT for data analysis can provide significant advantages. However, what are the potential limitations or risks that we should be aware of?
Great question, Alex! While AI models offer numerous benefits, limitations to consider include biases present in training data, sensitivity to input variations, and potential adversarial attacks. It's crucial to be aware of these risks and establish robust evaluation mechanisms to mitigate them.
I've seen a rise in AI-powered data analysis tools lately. How does ChatGPT differentiate itself from other similar models in the market?
Good question, Sophie! ChatGPT offers a more conversational interface, enabling users to interact and clarify their queries in a more human-like manner. It excels in generating human-readable responses, making it suitable for a wide range of data analysis scenarios. Its versatility and usability set it apart!
Timothy, I appreciate your article, but I'm curious about the performance limitations of ChatGPT. Does the model struggle with large datasets or complex analyses?
Thank you, Mark! ChatGPT indeed has limitations when dealing with extremely large datasets or highly complex analyses due to its computational constraints. In such cases, breaking down the analysis or leveraging more specialized models might be more suitable. It's crucial to consider the task requirements while choosing the right approach.
I'm amazed at how AI has progressed in recent years. Timothy, do you think we'll reach a point where AI can entirely handle data analysis without any human intervention?
AI has undoubtedly advanced, Tom, but it's unlikely that we'll entirely eliminate the need for human intervention in data analysis. AI models can automate certain aspects, but human understanding, creativity, and critical thinking are invaluable in deriving meaningful insights and making strategic decisions. It's a collaborative partnership!
Great article, Timothy! I'm excited about the potential ChatGPT has for data analysis. How can someone get started with leveraging ChatGPT effectively?
Thank you, Robert! To get started with leveraging ChatGPT for data analysis, it's essential to understand the model's capabilities and limitations. Experimentation, practice, and testing with a variety of datasets can help in becoming familiar with its behavior and optimizing for specific tasks. Participating in online forums and communities can also provide valuable insights and tips.
I love the idea of leveraging AI for data analysis, but how accessible is ChatGPT? Are there any limitations in accessing and utilizing the model effectively?
Accessibility is an important aspect, Lisa. OpenAI aims to provide accessible AI, and efforts are being made to make it easier to access and utilize ChatGPT effectively. However, limitations such as cost, resource requirements, and technical expertise can still pose challenges for some users. It's an evolving landscape, and continuous improvements are being made.
Timothy, can you share any success stories or real-world examples of organizations leveraging ChatGPT for advanced data analysis?
Certainly, Sam! Several organizations have started utilizing ChatGPT for various data analysis tasks. For example, in the finance industry, it has been used for analyzing market trends and generating executive summaries. Healthcare providers have employed it to analyze patient data for research purposes. The technology holds promise across multiple sectors!
This article opened my eyes to the potential of ChatGPT for advanced data analysis. Timothy, what are your thoughts on the future of AI in this field?
Glad to hear that, Olivia! The future of AI in data analysis is undoubtedly promising. As models improve, become more accessible, and ethical considerations are addressed, we are likely to witness increased adoption and the development of more specialized analysis capabilities. AI will augment human analysts and unlock new insights, shaping the future of data analysis.
Timothy, what are some potential use cases beyond data analysis where ChatGPT can be leveraged?
Great question, Jack! Besides data analysis, ChatGPT can be leveraged for tasks like customer support chatbots, content generation, language translation, and even as personal assistants. Its ability to understand and generate human language makes it versatile across various applications. The possibilities are vast!
Timothy, do you think AI models like ChatGPT will eventually surpass human capabilities in data analysis?
AI models continue to evolve and improve, Sophia, but surpassing human capabilities in data analysis is highly unlikely. While AI can automate repetitive tasks and generate insights, human analysts bring unique perspectives, intuition, and contextual understanding that are hard to replicate. It's the combination of human intellect and AI assistance that truly shines!
Timothy, in your article, you mentioned leveraging ChatGPT for 'advanced' data analysis. What defines 'advanced' in this context?
Good question, David! In the context of 'advanced' data analysis, it refers to sophisticated analyses that involve complex data sets, nuanced insights, and high-dimensional problems. It entails going beyond basic descriptive statistics and delving into predictive modeling, sentiment analysis, anomaly detection, and similar advanced analytical techniques. ChatGPT can assist in these endeavors!
Thanks for the insightful article, Timothy! I'm curious about the data requirements for leveraging ChatGPT effectively. Could you shed some light on that?
You're welcome, Grace! Leveraging ChatGPT effectively requires sufficient training data that is representative of the task you want to accomplish. While fine-tuning on domain-specific data can yield better results, even pre-trained models like ChatGPT can provide valuable insights with more generalized datasets. The quality and relevance of data play a significant role in achieving optimal performance.
Timothy, how can an organization ensure a smooth integration of ChatGPT into their existing data analysis workflows?
Excellent question, Richard! Smooth integration begins with assessing the specific needs of the organization and the alignment of ChatGPT's capabilities. Organizations should invest in facilitating seamless data access, provide the necessary infrastructure for running the model efficiently, and create mechanisms for capturing feedback from analysts. Collaborating with data scientists and technology experts can ensure a successful integration process.
I enjoyed reading your article, Timothy! Are there any recommended resources or tutorials for learning more about leveraging ChatGPT for data analysis?
Thank you, Emma! OpenAI provides resources and tutorials on their platform to learn more about leveraging ChatGPT for various tasks, including data analysis. Additionally, online communities like forums, blogs, and tutorials shared by AI enthusiasts and experts can be valuable in understanding practical use cases and best practices. Learning by doing and experimenting is often the most effective approach!
Timothy, how do you foresee the regulatory landscape evolving concerning the use of AI models like ChatGPT for data analysis?
Regulatory considerations are crucial, Chris. As AI technology continues to advance, it's likely that governments and regulatory bodies will develop frameworks and guidelines to govern its responsible use. Ensuring transparency, addressing bias, data privacy, and establishing mechanisms for accountability and auditability are some areas that are likely to receive increased attention. Striking the right balance is key!
Timothy, do you think there could be potential risks in relying heavily on AI models like ChatGPT for critical data analysis tasks?
Indeed, Sophie. Relying heavily on AI models for critical data analysis tasks carries risks. Models can still make errors, and over-reliance without proper human oversight can lead to wrong decisions or missed insights. Implementing robust validation processes, ensuring explainability, and having human analysts in the loop are essential to mitigate these risks and uphold the integrity of critical analyses.
Great article, Timothy! I have a question about the scalability of ChatGPT. Can it handle large-scale data analysis with millions of records effectively?
Thank you, John! ChatGPT's scalability is limited due to computational constraints. While it can handle moderate-sized datasets effectively, scaling up to millions of records might require dividing the analysis into smaller chunks or leveraging distributed computing. Choosing the right approach depends on multiple factors, including resource availability and the specific requirements of the analysis.
Timothy, how important is data preprocessing and cleaning when using ChatGPT for data analysis?
Data preprocessing and cleaning are critical steps, Peter. High-quality data input leads to more accurate and reliable outputs. Preprocessing tasks like removing noise, addressing missing values, and ensuring consistent formatting set the foundation for effective utilization of ChatGPT. These processes contribute significantly to minimizing biases and maximizing the overall performance.
I appreciate the insights shared in your article, Timothy! Can you shed some light on the computational requirements for utilizing ChatGPT effectively?
You're welcome, Mary! Utilizing ChatGPT effectively requires a decent amount of computational resources, especially for complex analyses or large-scale tasks. While the model can be run on reasonably powerful hardware, certain resource-intensive scenarios might require more advanced setups like GPU acceleration or cloud-based solutions. Right-sizing the infrastructure is crucial for optimal performance!
Thank you all for the engaging discussion on the potential of ChatGPT for advanced data analysis. I've enjoyed sharing insights and hearing your perspectives. Let's continue exploring the possibilities and shaping the future of data analysis together!