Unlocking the Power of Grid Computing with ChatGPT: Revolutionizing Collaborative Technological Innovations
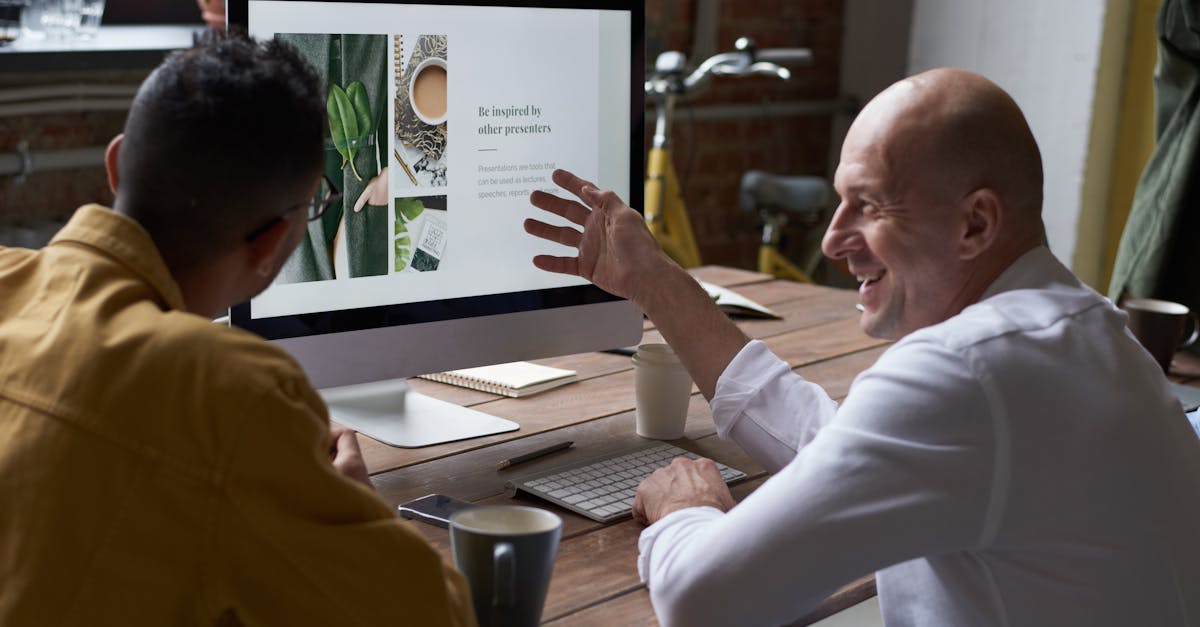
Grid Computing, a revolutionary technology, is utilized widely in running applications and solving complicated computing problems. Its potential in workload balancing is massive, particularly when we leverage tools like Chatgpt-4 to analyze the workload and distribute it efficiently among different nodes in the grid.
What is Grid Computing?
Grid Computing is a form of distributed computing that combines multiple computer resources from various domains to achieve a common goal. It's a network wherein each participating unit, known as a node, offers its resources to all others. These resources may be anything from processing power to storage capacities.
Workload Balancing in Grid Computing
The primary goal of Grid Computing is to make the most effective use of all computing resources available on the network. One of the key aspects of achieving this is Workload Balancing. Workload Balancing determines how to dispense computational tasks evenly across all machines in the grid to minimize the time taken to complete the workload and to prevent any one node from being overloaded while others are underused.
How can ChatGPT-4 be used in Workload Balancing?
ChatGPT-4, a new tool, is an AI language model developed by OpenAI. It has the capability to understand and generate human-like text based on the input provided. This capability can be used to analyze the workload functioning on a grid network and provide insights on how to distribute the workload across nodes for better productivity.
The advantage of using artificial intelligence in Workload Balancing is that it can analyze and predict elements that may be complicated or almost impossible manually and based on these predictions, it can make suitable decisions.
Step 1: Data Gathering
Chatgpt-4 can assist in the first step of analyzing the workload by gathering data about the workload running on each node. This includes details about the processing power each workload requires, the storage it needs, and the time it takes to complete execution.
Step 2: Data Analysis
The AI model will then analyze this data to understand patterns and trends in the usage of resources. This includes recognizing tasks that might result in a higher computational load or predicting when the workload might increase or decrease in the future.
Step 3: Decision Making
Based on the analysis, Chatgpt-4 can provide insights on how to distribute the workload. This could be anything from moving a small part of the workload to a lesser-used node or changing the time at which a particular task is executed to benefit from times when the workload is generally lower.
Step 4: Monitoring
Post the implementation of the suggestions by Chatgpt-4, it will continue monitoring the network to evaluate whether the changes have positively affected the network. If positive results are not obtained, it can learn from the situation and make better recommendations in the future.
Conclusion
Grid Computing holds immense potential and its utilization in workload balancing can provide an effective way to manage computational resources. By incorporating an advanced platform like Chatgpt-4, the process can be further optimized. As AI becomes more integrated into our computing networks, we can expect more efficient utilization and management of resources in the future.
Comments:
Thank you all for your feedback and comments on my article. I'm glad to see that there is interest in the potential of ChatGPT for grid computing. If you have any specific questions or ideas, feel free to ask!
Great article, Carl! I'm excited about the possibilities that ChatGPT brings to grid computing. It opens up new avenues for collaboration and technological advancements.
@Linda Smith I totally agree with you. The combination of grid computing and ChatGPT has the potential to revolutionize the way we collaborate on technological innovations. It's a game-changer!
This is fascinating! I can envision ChatGPT helping scientists and researchers worldwide to share their insights and work together more efficiently. The power of collective intelligence!
Indeed, @Michelle Thompson! ChatGPT can facilitate real-time communication and data sharing among experts, enabling multidisciplinary collaborations like never before. It's an exciting time for technological advancements!
I wonder how scalable ChatGPT is in the context of grid computing. Can it handle a massive number of users and complex computations simultaneously?
@Emily Davis Excellent question! ChatGPT's scalability is a crucial consideration. OpenAI has been actively working on enhancing its performance and capacity to support large-scale collaborative efforts. While challenges remain, promising progress is being made!
The ethical implications of ChatGPT in grid computing are worth examining. How can we ensure that the AI's responses are unbiased and reliable, especially in critical decision-making scenarios?
@Rachel Thompson Valid concern. OpenAI acknowledges the importance of addressing bias and ensuring reliability. Extensive research and development efforts are being made to mitigate biases and improve the overall trustworthiness of ChatGPT. Transparency and accountability are key focus areas!
As exciting as ChatGPT sounds, I wonder about the security risks associated with grid computing on such a platform. How can we protect sensitive data and prevent malicious activities?
@Jonathan Brown Security is paramount. ChatGPT's usage in grid computing calls for robust security measures. OpenAI is investing in research and collaborations to address potential vulnerabilities, establish secure protocols, and safeguard sensitive data. Ensuring user privacy and data protection is of utmost importance!
I'm curious about the potential applications of ChatGPT in the healthcare sector. Can it be utilized to improve medical research or assist healthcare professionals?
@Laura Rodriguez Absolutely! ChatGPT can be a valuable tool in healthcare. It can assist in medical research, provide insights into complex data, support diagnosis and treatment recommendations, and enable collaboration among healthcare professionals to address challenges collectively. Exciting possibilities lie ahead!
Carl, thank you for highlighting the potential of ChatGPT in grid computing. Your article provided a clear overview. I'm looking forward to seeing how this technology evolves and shapes future collaborations!
@Daniel Wilson You're welcome! I'm glad you found the article helpful. Indeed, the evolution of ChatGPT and its integration into grid computing holds immense promise. Together, we can unlock new frontiers of collaborative technological innovation!
This article got me thinking about the potential synergy between ChatGPT and Internet of Things (IoT) technologies. Can they complement each other in any way?
@Mark Patterson Absolutely! ChatGPT and IoT are complementary in many ways. The ability of IoT devices to generate massive amounts of data can be combined with ChatGPT's collaborative capabilities to extract valuable insights, optimize processes, and enhance decision-making. The integration of these technologies can lead to transformative outcomes!
The potential of ChatGPT seems immense, but what are some of the significant challenges that need to be overcome for its successful adoption in grid computing?
@Olivia Adams Valid point. While ChatGPT brings promising possibilities, notable challenges include scalability, bias mitigation, security, and addressing ethical concerns. OpenAI is actively working on these fronts, collaborating with the community to overcome hurdles and ensure responsible and effective integration of ChatGPT into grid computing.
Congratulations, Carl, on a well-written article. It's exciting to envision the combined potential of grid computing and ChatGPT. The future of technological innovation looks incredibly promising!
@Sandra Lewis Thank you for your kind words, Sandra! I share your enthusiasm about the future. By harnessing the power of grid computing and ChatGPT, we can unlock unprecedented collaborative potential and drive remarkable technological advancements. Together, we can shape the future!
Carl, your article left me pondering the potential impact of ChatGPT on education and learning. What are your thoughts on leveraging this technology in educational settings?
@Benjamin Morris Excellent question! The application of ChatGPT in education is promising. It can assist learners, provide personalized tutoring, and facilitate collaborative problem-solving. Furthermore, ChatGPT can be a valuable resource for educators, offering access to a vast array of knowledge and insights. The potential for enhanced learning experiences is immense!
I'm curious to know if ChatGPT can assist in the optimization of grid computing algorithms. Can it contribute to making grid computing more efficient and cost-effective?
@Sophia Hughes That's an interesting point! ChatGPT can indeed contribute to the optimization of grid computing algorithms. It can provide insights, recommendations, and collaborative problem-solving that may lead to improved efficiency and cost-effectiveness. The collective intelligence harnessed through ChatGPT can help drive innovations in grid computing!
Carl, I appreciate your article, but I have concerns about the potential for misuse of ChatGPT in grid computing. How do we ensure responsible use and prevent any unethical or malicious activities?
@Julian Anderson Responsible use is of utmost importance. OpenAI is committed to addressing these concerns. Mechanisms such as content moderation, user guidelines, and feedback loops are being actively explored and developed to prevent misuse. Collaborative efforts with the community will help shape the policies and safeguards necessary for responsible and ethical deployment of ChatGPT in grid computing!
The potential of ChatGPT in grid computing is immense, but how can we ensure inclusivity and accessibility? Are there any plans to make it available to a wide range of users?
@Sarah Adams Inclusivity and accessibility are key considerations. OpenAI is actively working on making ChatGPT more accessible and expanding its availability to a wider audience. The aim is to empower diverse users, including researchers, scientists, and developers, to harness the collaborative power of ChatGPT in grid computing. Emphasizing inclusivity and addressing barriers is a priority!
Grid computing is a promising field, and ChatGPT seems like a valuable addition. However, I'm curious about the required computational resources for running ChatGPT in a grid computing environment.
@Eric Roberts You raise a valid concern. ChatGPT's resource requirements in a grid computing environment depend on factors such as model size, usage patterns, and the scale of deployment. OpenAI is striving to optimize resource utilization and efficiency to ensure the seamless integration of ChatGPT in grid computing setups. Resource management is an active area of research and development!
Carl, your article highlighted the potential of ChatGPT for revolutionizing collaborative technological innovations. Can you provide some specific examples of how ChatGPT can be utilized in grid computing?
@Jeffrey Thompson Absolutely! ChatGPT can facilitate real-time communication and collaboration among researchers, scientists, and developers in grid computing. It can help in sharing ideas, refining research methodologies, debugging complex problems, optimizing algorithms, and creating technology roadmaps. The conversational nature of ChatGPT enhances coordination and accelerates collaborative work. The possibilities are vast!
Carl, what are some of the potential limitations of ChatGPT that we should be aware of when utilizing it in grid computing?
@Maria Garcia Good question! While ChatGPT offers tremendous benefits, its current limitations include sensitivity to input phrasing, occasional generation of incorrect answers, and being verbose when unnecessary. OpenAI is actively addressing these limitations through research and user feedback to make ChatGPT more robust and reliable. Ongoing improvements aim to enhance its usefulness for grid computing!
Thank you, Carl, for shedding light on the potential applications of ChatGPT in grid computing. It's exciting to think about the impact this can have on collaborative technological advancements!
@Jacob Carter You're welcome, Jacob! I'm thrilled that you found the potential applications of ChatGPT in grid computing exciting. By harnessing this technology collaboratively, we can drive remarkable advancements and accelerate innovation like never before. Thank you for your support!
Carl, your article got me thinking about the potential benefits of using ChatGPT in grid computing for sustainable energy solutions. Can it contribute to optimizing energy usage and improving resource efficiency?
@Emma Thompson Absolutely! ChatGPT can be harnessed in grid computing to optimize energy usage, improve resource allocation, and facilitate the adoption of sustainable energy solutions. By enabling collaboration and knowledge sharing among experts, ChatGPT can play a crucial role in driving innovation towards a more sustainable future!
ChatGPT has the potential to transform the way we approach grid computing. It can foster interdisciplinary exchange and spur novel ideas to advance various technological domains. Exciting times ahead!
@Robert Wilson Indeed, Robert! The interdisciplinary nature of grid computing combined with ChatGPT's collaborative power opens up possibilities for cross-pollination of ideas and innovation in diverse technological domains. By working together, we can forge new paths and accelerate progress. Thank you for your enthusiasm!
I'm amazed by the potential of ChatGPT in grid computing! It can enable global collaboration, transcending geographical boundaries and time zones. The world truly becomes a smaller place.
@Sophie Anderson Absolutely! ChatGPT's ability to foster global collaboration and transcend geographical constraints is a significant advantage. It breaks down barriers and facilitates the exchange of expertise, ultimately fueling grid computing with diverse perspectives and talent. Together, we can overcome challenges and achieve groundbreaking technological advancements!
Carl, your article left me wondering about the integration of ChatGPT with existing grid computing frameworks. Are there any specific challenges or requirements to consider?
@John Wilson Integration with existing grid computing frameworks is a crucial aspect. One of the challenges is ensuring compatibility and seamless interoperability between ChatGPT and diverse grids. OpenAI, in collaboration with the grid computing community, is exploring ways to address these challenges. Compatibility testing, standardized interfaces, and comprehensive documentation are being prioritized to simplify integration and maximize the benefits of ChatGPT!
I'm excited about ChatGPT's potential to democratize access to grid computing resources. Can it help level the playing field and empower individuals and smaller teams to participate in collaborative innovations?
@Anna Martinez Absolutely! ChatGPT can democratize access to grid computing resources by enabling individuals and smaller teams to collaborate, share ideas, and contribute to collaborative innovation. It reduces barriers to entry and empowers diverse talent to actively engage in technological advancements. By fostering inclusivity, we can unlock the full spectrum of human potential!
ChatGPT has the potential to transform grid computing into a more interactive and engaging experience. The ability to communicate with a conversational AI amplifies the collective intelligence and opens up new avenues for collaboration.
@Nathan Taylor You've hit the nail on the head, Nathan! ChatGPT's conversational capabilities amplify the collective intelligence in grid computing. It fosters engagement, enables clearer communication, and catalyzes collaboration among experts, leading to more impactful technological innovations. Thank you for highlighting this valuable aspect!
Carl, your article instilled curiosity in me about the potential impact of ChatGPT on computational research. Can it assist in accelerating simulations and modeling?
@Sarah Miller Absolutely! ChatGPT can contribute to accelerating simulations and modeling in computational research. By offering insights, refining parameters, suggesting improvements, and facilitating collaboration, ChatGPT can enhance the efficiency and effectiveness of modeling and simulation efforts. It has the potential to significantly impact a wide range of computational domains!
I can see ChatGPT being a game-changer in grid computing. It enhances communication, breaks down silos, and enables experts to work together seamlessly. Can't wait to see it in action!
@Tom Roberts Absolutely, Tom! ChatGPT is poised to be a game-changer in grid computing by revolutionizing collaborative communication, breaking down silos, and enabling seamless teamwork. The potential for accelerated progress and transformative innovations is truly exciting. Stay tuned for the incredible journey ahead!
I'm curious about the resources required for training and deploying ChatGPT on a large scale for grid computing. Can it be achieved feasibly?
@Sophie Wright Training and deploying ChatGPT on a large scale for grid computing indeed demands substantial resources. OpenAI is actively working on optimizing infrastructure, training methodologies, and deployment pipelines to make it increasingly feasible. The collaborative nature of grid computing assists in resource sharing and distribution, making large-scale deployment a viable possibility. Iterative improvements are being made to overcome resource constraints!
The combination of grid computing and ChatGPT brings immense potential for accelerating technological advancements. It's great to see the collaborative power of AI being harnessed for global progress!
@Andrew Thompson Absolutely, Andrew! The combined strength of grid computing and ChatGPT generates a powerful force for accelerating technological advancements. By leveraging the collaborative potential of AI, we can drive global progress, foster inclusive innovation, and tackle complex challenges more effectively. Thank you for your support!
Carl, what are some of the potential limitations or trade-offs of integrating ChatGPT into grid computing? Are there any scenarios where it might not be suitable?
@Lucy Carter Introducing ChatGPT into grid computing does involve certain limitations and trade-offs. While it enhances collaboration and communication, it might not be suitable for scenarios where real-time operations or immediate responses are critical. In such cases, other existing techniques or tools may be better suited. It is important to carefully evaluate the requirements and dynamics of the grid computing scenario before integrating ChatGPT!
Thanks for shedding light on the potential of ChatGPT in grid computing, Carl. I believe it can fundamentally transform the way we approach interdisciplinary research. Collaboration holds the key to unlocking breakthroughs!
@Jonathan Turner I appreciate your support, Jonathan! Collaboration is indeed at the heart of grid computing potential. By leveraging ChatGPT's capabilities, researchers and experts across disciplines can collaborate more effectively, driving interdisciplinary breakthroughs and reshaping the frontiers of knowledge. Let's unlock the power of collaboration together!
I'm thrilled about the potential application of ChatGPT in grid computing for addressing complex challenges. As an AI enthusiast, I see vast opportunities for transformative innovation!
@Sophia Stewart Your enthusiasm is contagious, Sophia! The potential for transformative innovation through ChatGPT in grid computing is immense. As we tackle complex challenges collaboratively, we can unlock new insights, devise novel solutions, and contribute to meaningful progress. I share your excitement for the incredible possibilities ahead!
Carl, your article creates a compelling vision for the future of grid computing with ChatGPT. I'm curious about the ongoing research and development efforts to improve and enhance its capabilities.
@Ethan Davis Ongoing research and development constitute a significant focus in improving and enhancing ChatGPT's capabilities. OpenAI is actively investing in areas such as model scaling, learning from user feedback, addressing biases and ethical concerns, and refining deployment approaches. By engaging with the community and leveraging collective expertise, we can drive continuous improvements and shape the future of AI-powered grid computing!
Grid computing has tremendous potential, and ChatGPT seems like an ideal tool to foster collaboration and knowledge-sharing in such a context. Exciting times for technological innovation!
@Jack Mitchell Absolutely, Jack! ChatGPT's role in fostering collaboration and knowledge-sharing in grid computing is instrumental. By leveraging its conversational capabilities, we can accelerate progress, generate new insights, and drive synergy among experts to push the boundaries of technological innovation. Thank you for your support!
Carl, your article highlighted the potential of ChatGPT for revolutionizing grid computing. Can you share any success stories or specific use cases where ChatGPT has already been applied?
@Mia Davis While ChatGPT is still an emerging technology, there have been successful applications in various fields. One notable use case is its deployment in collaborative writing scenarios, where it assists authors in refining and expanding ideas. Furthermore, in specific research domains, ChatGPT has demonstrated the ability to generate novel insights and aid in complex problem-solving. These early successes set the stage for further exploration and integration of ChatGPT in grid computing!
ChatGPT offers exciting potential for fostering global collaborations and accelerating technological breakthroughs in grid computing. We are entering a new era of collaborative innovation!
@Emily Turner You've summarized it beautifully, Emily! With ChatGPT's capability to enable global collaborations and accelerate technological breakthroughs, we are indeed entering a new era of collaborative innovation. By harnessing collective expertise and nurturing partnerships, we can unlock unparalleled potential and drive the future of grid computing. Thank you for your enthusiasm!
I'm excited about the potential of ChatGPT in grid computing, but what are some of the risks associated with relying on AI-powered decision-making or recommendations?
@Jordan Gonzalez Valid concern, Jordan. Relying solely on AI-powered decision-making poses risks that need to be addressed. Human oversight, interpretability of AI-generated outputs, and transparent accountability mechanisms are essential in ensuring responsible utilization of AI. A balanced approach that combines human expertise with AI-powered recommendations is key, mitigating risks and maximizing the benefits of ChatGPT in grid computing!
As a researcher, I find the potential of ChatGPT in grid computing extremely promising. It can facilitate collaboration, knowledge-sharing, and accelerate discoveries. Exciting times for researchers!
@Emma Reynolds Absolutely, Emma! ChatGPT is poised to revolutionize the research landscape in grid computing by facilitating collaboration and accelerating discoveries. The ability to share insights, validate methodologies, and refine ideas collaboratively can significantly enhance the pace of progress. It's an exciting time for researchers to leverage the power of ChatGPT and redefine what's possible!
The potential for ChatGPT in grid computing is immense. It can facilitate global collaboration, transcending barriers, and opening doors to collaborative breakthroughs. Kudos on highlighting this exciting prospect!
@Ryan Lee Thank you for your kind words, Ryan! The immense potential of ChatGPT in facilitating global collaboration cannot be understated. By enabling knowledge sharing, democratizing access to expertise, and fostering multidisciplinary collaboration, ChatGPT can unlock breakthroughs that were previously unattainable. Together, we can shape the future of grid computing and drive remarkable advancements!
Carl, your article gave me insight into the untapped potential of grid computing with ChatGPT. It's inspiring to think about the possibilities it holds for technological innovation!
@Anthony Richardson I'm glad you found the article insightful, Anthony! The untapped potential of grid computing with ChatGPT indeed holds immense possibilities for technological innovation. By applying collaborative intelligence to grid computing, we can push the boundaries of what's achievable, paving the way for transformative advancements across various domains. Thank you for your support!
ChatGPT has the potential to revolutionize the way we collaborate and advance technologies in the field of grid computing. Looking forward to witnessing its impact!
@William Thompson Revolutionizing collaboration and advancing technologies in grid computing is definitely within reach with ChatGPT's potential. By providing a platform for real-time communication, knowledge sharing, and collaborative problem-solving, we can accelerate progress and shape the future together. Thank you for your anticipation of the exciting times ahead!
I'm intrigued by the potential of ChatGPT in enhancing data analysis and interpretation in grid computing. Can it assist in extracting insights from vast datasets?
@Sophie Harris Absolutely, Sophie! ChatGPT's collaborative capabilities can be leveraged to enhance data analysis and interpretation in grid computing. It can assist in extracting valuable insights from vast datasets, facilitating understanding, and enabling decision-making based on comprehensive analyses. The fusion of human expertise and AI-powered collaboration can unlock hidden patterns and accelerate discoveries!
Carl, congratulations on an insightful article! I'm excited about the potential of ChatGPT in powering collaborative technological innovations and breaking new ground in grid computing.
@James Rogers Thank you for your kind words, James! I'm thrilled that you found the article insightful. The potential of ChatGPT in powering collaborative technological innovations is indeed exciting. By breaking new ground in grid computing, we can advance a wide range of domains and redefine what's achievable. Together, let's shape the future of collaborative technological innovation!
The possibilities of ChatGPT in grid computing seem limitless. Its collaborative capabilities lay the foundation for groundbreaking synergies and advancements. It's an exciting time for technology!
@Charlotte Walker Limitless possibilities indeed lie ahead with ChatGPT in grid computing! Its collaborative capabilities enable unprecedented synergies, harnessing collective intelligence and driving remarkable advancements. The possibilities for technological innovation are immense, and I'm thrilled to be a part of this exciting time. Thank you for your enthusiasm!
Carl, your article provided a compelling narrative about the potential of ChatGPT in grid computing. The vision of collaborative progress it paints is truly inspiring!
@Aaron Wilson I'm glad you found the article compelling, Aaron! The potential of ChatGPT in grid computing to drive collaborative progress is truly inspiring. By fostering collaboration, knowledge-sharing, and collective problem-solving, we can accelerate innovation and shape the future collaboratively. Thank you for your support!
I'm fascinated by the potential of ChatGPT in enhancing teamwork and collaboration in grid computing. It can truly transform the way we work together and unlock new frontiers!
@Ella Wilson Absolutely, Ella! The potential of ChatGPT in enhancing teamwork and collaboration in grid computing is fascinating. By enabling real-time communication, knowledge exchange, and collaborative problem-solving, ChatGPT can transform how we work together, foster innovation, and unlock new frontiers of possibility. Thank you for recognizing its transformative capacity!
I believe ChatGPT can bring the collective wisdom of experts from around the world to the world of grid computing. It's exciting to imagine the possibilities!
@Thomas Cook You've captured it perfectly, Thomas! ChatGPT's power lies in its ability to bring together the collective wisdom of experts worldwide in the realm of grid computing. By transcending boundaries, enabling global collaboration, and facilitating knowledge exchange, we can harness the power of diverse perspectives to unlock groundbreaking possibilities. Thank you for sharing your excitement!
Carl, your article got me thinking about the potential impact of ChatGPT on fostering innovation in grid computing. Can it support the generation of novel ideas and breakthrough concepts?
@Jessica Wilson Absolutely, Jessica! ChatGPT has the potential to support the generation of novel ideas and breakthrough concepts in grid computing. By facilitating collaboration, enabling cross-fertilization of ideas, and offering insights into complex problems, ChatGPT empowers experts to push the boundaries of innovation. It's an exciting time to explore uncharted territories and create transformative advancements!
Carl, your article left me wondering about the impact of ChatGPT on the scalability of grid computing. Can it help address the challenges associated with scaling computations?
@George Thompson Scalability is a crucial consideration for grid computing, and ChatGPT can contribute to addressing the challenges associated with scaling computations. By leveraging collaborative problem-solving, real-time communication, and knowledge-sharing, ChatGPT enables scalable collaboration and assists in optimizing computations. It amplifies the collective intelligence, leading to enhanced scalability and more efficient grid computing setups!
The potential of ChatGPT is promising, but it's important to address concerns related to its limitations and biases. How can we ensure ethical and unbiased utilization of ChatGPT in grid computing?
@Sophia Martinez I absolutely agree, Sophia. Ethical and unbiased utilization of ChatGPT in grid computing is of paramount importance. OpenAI is actively working on addressing limitations, biases, and ensuring transparency. Guidelines, user feedback mechanisms, and community collaborations are being leveraged for responsible deployment. By fostering accountability and addressing concerns head-on, we can ensure an ethical and unbiased utilization of ChatGPT in grid computing!
As a technologist, I'm excited about the collaborative possibilities that ChatGPT brings to grid computing. The ability to seamlessly communicate with AI systems opens up new horizons for solving complex problems.
@Alex Turner Collaboration is at the heart of technological progress, Alex. ChatGPT's ability to seamlessly communicate and collaborate with AI systems indeed opens up new horizons for tackling complex problems in grid computing. By embracing this technology, we can push the boundaries of what we can achieve together. Exciting times lie ahead!
Thank you all for reading my article on 'Unlocking the Power of Grid Computing with ChatGPT: Revolutionizing Collaborative Technological Innovations'. I'm excited to hear what you think about the possibilities that ChatGPT brings to collaborative innovation!
Great article, Carl! Grid computing coupled with the power of ChatGPT is indeed a game-changer. It opens up new avenues for enhancing collaboration and problem-solving in tech projects. The potential is enormous.
I completely agree, Sarah. The ability to harness the collective intelligence and creativity of individuals using ChatGPT in grid computing can lead to significant breakthroughs in various technological domains. It's exciting to witness the potential impact.
As a software developer, I find the concept fascinating. ChatGPT has already proven to be a valuable tool for generating human-like text, and when integrated into grid computing, it can boost productivity and innovation. Collaboration will never be the same again.
I'm curious about the security aspects of ChatGPT in grid computing. How can we ensure that sensitive information shared in collaborative projects remains confidential?
That's a valid concern, Michael. With ChatGPT in grid computing, privacy and security are crucial. One approach is to employ encryption techniques to protect sensitive data during communication and ensure access controls prevent unauthorized access. Additionally, continuous monitoring and audits can help maintain data integrity.
I think it's essential to establish robust security protocols while implementing ChatGPT in grid computing. Encrypting data, using secure channels for communication, and implementing strong authentication mechanisms will be vital to address potential privacy and security risks.
The potential of grid computing with ChatGPT is undeniable. It can accelerate the pace of innovation by facilitating collaboration among experts worldwide. I'm interested to see how this technology progresses and the impact it will have on the tech industry.
It's an exciting time for collaborative technological innovations. ChatGPT integrated into grid computing has the ability to connect and engage experts from different geographical locations. This amalgamation can lead to groundbreaking discoveries and expedite problem-solving.
I can see tremendous potential in using ChatGPT for collaborative projects. It makes knowledge sharing and brainstorming more efficient, helping to bridge the gaps between experts in various fields. Grid computing can provide the necessary infrastructure for these large-scale collaborations.
While the concept sounds promising, we need to ensure that ChatGPT in grid computing doesn't replace human creativity and expertise. It should augment our capabilities and support collaboration rather than substitute human intelligence.
I agree, Sophia. ChatGPT is a powerful tool, but human judgment and domain expertise should always play a crucial role in decision-making. The combination of artificial intelligence and human intelligence can lead to remarkable synergistic results.
The potential for innovation and breakthroughs with ChatGPT and grid computing is immense. By leveraging distributed computing power and the collaboration-enabling capabilities of ChatGPT, we can tackle complex problems more efficiently and come up with better solutions.
I wonder how ChatGPT could benefit industries like healthcare or climate research. The ability to harness collective knowledge and experience through grid computing, coupled with the conversational abilities of ChatGPT, could lead to significant advancements.
Those are excellent points, Emma. In healthcare, for example, ChatGPT integrated with grid computing can assist in analyzing large amounts of patient data and providing personalized recommendations. In climate research, it can aid in analyzing complex climate models and developing strategies to mitigate environmental challenges.
The potential impact of ChatGPT in healthcare is immense. It can help in diagnosing rare diseases, suggesting treatment options, and assisting doctors in decision-making. Leveraging grid computing can enhance its capabilities and allow for better collaboration among healthcare professionals.
The concept is intriguing, but what challenges do you foresee in adopting ChatGPT and grid computing on a larger scale? Are there any limitations we should be aware of?
Good question, Ethan. One of the challenges is ensuring the reliability of ChatGPT's responses when multiple users contribute simultaneously. It's important to maintain consistency and avoid conflicting suggestions. Balancing computational resources and optimizing the efficiency of grid computing infrastructure can also be a challenge.
Another challenge could be addressing biases in ChatGPT's responses. We need to ensure that the tool doesn't reinforce or perpetuate existing biases during collaborative conversations. Continuous monitoring and improvement of the underlying models will be crucial.
I'm excited about the potential of ChatGPT in grid computing. It can revolutionize education by providing personalized learning experiences and facilitating collaboration among students and educators. The possibilities for enhancing education are vast.
Indeed, Natalie. ChatGPT can act as a virtual learning companion, assisting students in various subjects and adapting to individual learning styles. Collaborative learning through grid computing can provide students access to diverse perspectives and foster an interactive educational experience.
I'm intrigued by the potential applications of ChatGPT in creative fields such as art or music. It can be a great tool for brainstorming ideas, generating creative content, and collaborating with other artists.
Absolutely, Sophie. ChatGPT integrated with grid computing can aid artists in exploring new artistic directions, generating unique compositions, and receiving feedback from peers. It has the potential to redefine the creative process and push the boundaries of artistic innovation.
I'm concerned about the ethical implications of using AI like ChatGPT in collaborative settings. How can we ensure responsible and ethical usage to avoid any unintended consequences?
Ethical considerations are vital when integrating AI like ChatGPT into collaborative environments. Clearly defining ethical guidelines, monitoring system outputs, and promoting transparency in decision-making can help mitigate unintended consequences. Additionally, involving diverse perspectives in the development and deployment of the technology is crucial.
Regular audits and reviews of the system's behavior can help identify and address any biases or ethical concerns that may arise. Responsible usage and continuous improvement should be the foundation of integrating AI technologies like ChatGPT in collaborative projects.
The integration of ChatGPT in grid computing can have profound implications for research and development. It can expedite scientific breakthroughs, optimize complex simulations, and enhance data analysis. Exciting times ahead!
I think there's also potential for ChatGPT in customer support and service. It can assist in providing more personalized and efficient responses to customer queries, improving overall customer experience.
Absolutely, Olivia. Integrating ChatGPT into customer support workflows can enhance self-service options, provide quick and accurate answers to frequently asked questions, and offer 24/7 support. It has the potential to revolutionize customer service interactions.
Valid point, Olivia. But we should be cautious to strike the right balance between automated responses and human support. Some customers may still prefer speaking to a real person for complex issues or personal interactions.
The collaborative power of ChatGPT in grid computing has immense potential, but what are the computational resource requirements for such large-scale collaborations?
You're right, Sophia. Large-scale collaborations using ChatGPT in grid computing require substantial computational resources. High-performance computing infrastructure, distributed systems, and efficient utilization of resources are key to enable seamless and efficient collaboration.
Optimizing resource allocation, load balancing, and reducing latencies in grid computing are critical for large-scale collaboration with ChatGPT. The scalability and flexibility of the infrastructure will play a significant role in supporting such computational demands.
In the context of grid computing, what challenges do you foresee in integrating ChatGPT with different programming languages and frameworks used in collaborative projects?
A good question, Maxwell. Seamless integration of ChatGPT with different programming languages and frameworks can be a challenge due to compatibility issues. Bridging the gap between various technologies and providing easy-to-use interfaces or APIs can simplify the integration process and foster collaboration.
Interoperability and standardization of interfaces and protocols will be crucial for the successful integration of ChatGPT across different programming languages and frameworks. Collaboration should not be limited by technological boundaries.
The potential of ChatGPT in grid computing also raises questions about intellectual property rights. How can we ensure proper attribution and protection of ideas generated through collaborative projects?
You bring up an important point, Zoe. Clearly defining ownership and attribution of ideas generated through collaborative projects is crucial. Establishing legal frameworks and agreements to protect intellectual property rights and fostering a culture of proper attribution can address these concerns.
The integration of ChatGPT in grid computing has the potential to create a global community of problem-solvers. It can democratize access to expertise and drive innovation by leveraging the collective intelligence of individuals. Exciting times await us!
Thank you all for your valuable insights and comments. It's inspiring to see the enthusiasm surrounding the possibilities of ChatGPT in grid computing. Let's continue pushing the boundaries of collaborative technological innovations!