Unlocking the Power of Natural Language Processing: Leveraging ChatGPT for Data Analysis
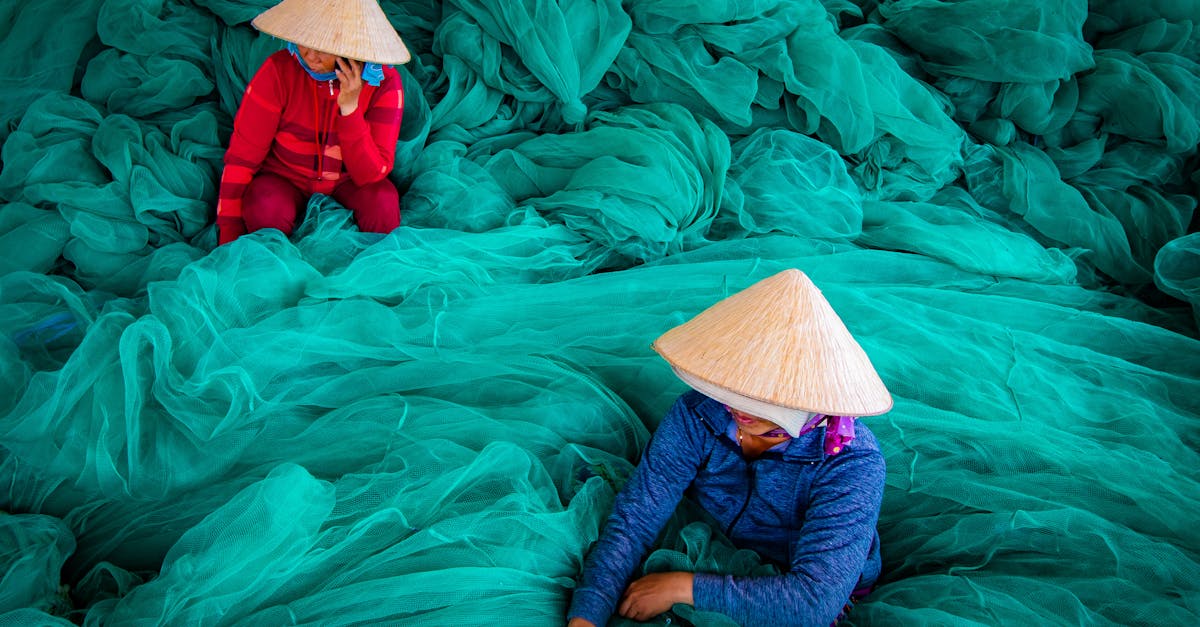
As technology continues to advance, the field of data analysis has been significantly transformed. One of the major developments in this field is the integration of Natural Language Processing (NLP). With the advent of ChatGPT-4, the capabilities of data analysis have been further enhanced, as it can now understand, analyze, generate, and make sense of human language in a valuable way.
What is Natural Language Processing (NLP)?
Natural Language Processing is a subfield of artificial intelligence that focuses on the interaction between computers and human language. It encompasses various techniques and algorithms aimed at understanding, interpreting, and generating human language in a meaningful way.
ChatGPT-4: The Power of Understanding Human Language
ChatGPT-4 is an advanced language model that has revolutionized data analysis by integrating powerful Natural Language Processing capabilities. This model has been trained on vast amounts of text data, enabling it to comprehend a wide range of topics and accurately process human language.
One of the primary applications of ChatGPT-4 in data analysis is in processing unstructured data. Unstructured data, such as customer feedback, social media posts, and online reviews, contain valuable insights that can help organizations make informed decisions. By utilizing ChatGPT-4's language understanding abilities, businesses can extract meaningful information and gain a deeper understanding of customer sentiment and preferences.
Analyzing and Generating Insights
ChatGPT-4's NLP capabilities extend beyond understanding language to generating insights and valuable analyses. It can process large volumes of textual data and identify patterns, trends, and outliers. This allows data analysts to gain comprehensive insights from various sources, including customer interactions, surveys, and other written documents.
Furthermore, ChatGPT-4 can generate coherent summaries and reports by summarizing complex information in a concise and understandable manner. This feature significantly reduces the time and effort required to sift through extensive textual data, enabling data analysts to focus their attention on interpreting and leveraging the insights generated by the model.
Improving Decision-making and Automation
By leveraging NLP capabilities, ChatGPT-4 assists businesses in improving decision-making processes. It can analyze textual data to identify emerging trends, predict customer behavior, or detect potential issues before they escalate.
The integration of ChatGPT-4 into automated systems enables organizations to provide personalized and contextually relevant responses to customer queries, support ticket management, and sentiment analysis. This helps businesses enhance customer experience and streamline operations by automating routine tasks.
The Future of Data Analysis with NLP
As advancements in NLP continue, we can expect further innovation in data analysis. ChatGPT-4 is just the latest example of how NLP is transforming the field, unlocking new possibilities for businesses across various industries. The ability to extract valuable insights from unstructured data, understand customer sentiment, and automate processes will undoubtedly have a profound impact on decision-making and overall business success.
Conclusion
Natural Language Processing has revolutionized the data analysis landscape, and ChatGPT-4 is at the forefront of this transformation. Its ability to understand, analyze, generate, and make sense of human language in a valuable way opens up a multitude of possibilities for extracting insights, streamlining operations, and enhancing decision-making processes. As NLP continues to evolve, businesses can expect even more significant advancements in the field of data analysis.
Comments:
Thank you all for joining this discussion! I'm Kerry Raminiak, the author of the article. I'm excited to talk about leveraging ChatGPT for data analysis.
Great article, Kerry! I've been using NLP for data analysis, and ChatGPT seems like a powerful tool to enhance the capabilities. Have you personally used it for any projects?
Hi Sarah! Yes, I've had the opportunity to work with ChatGPT for a few projects. It's quite remarkable how it can assist in tasks like text classification, sentiment analysis, and even generating summaries. The flexibility of fine-tuning the model makes it highly adaptable.
Hi everyone! Joshua here. Kerry, could you elaborate on the challenges you faced while leveraging ChatGPT for data analysis? And any tips for getting started?
Hi Joshua! One challenge I encountered was the need for careful input preprocessing, as the model can be sensitive to input phrasing. It's important to experiment and iterate to find the best approach for your specific data analysis tasks. As for tips, starting with smaller datasets and gradually scaling up can help in understanding the capabilities of ChatGPT and fine-tuning it effectively.
Hi all! Liam here. Kerry, do you think ChatGPT can be useful for real-time analysis of streaming data, or is it more suited for batch processing?
Hi Liam! While ChatGPT can be used for real-time analysis, it is primarily designed for batch processing. However, fine-tuning the model with specific streaming data might enable real-time analysis to an extent. It ultimately depends on the specific use case and requirements.
Thank you, Kerry, for sharing your insights! I'm curious, have you noticed any limitations or potential biases when using ChatGPT for data analysis?
Hi Emma! Good question. ChatGPT, like many other NLP models, can sometimes exhibit biases in its responses, since it learns from large datasets. It's important to be mindful of this and apply additional steps to mitigate bias during data analysis. Regular evaluation and monitoring can help ensure fairness and accuracy.
Hey everyone! Sophia here. Kerry, what potential applications do you foresee for ChatGPT in industries like finance or healthcare?
Hi Sophia! In finance, ChatGPT can aid in analyzing text-based financial news, customer support, or even fraud detection. In healthcare, it could assist in extracting insights from medical literature, patient records analysis, and automated triaging of symptoms. These are just a few examples, and the potential use cases are extensive.
Hello, all! Oliver here. Kerry, considering the continuous advancements in NLP, where do you see the future of ChatGPT heading?
Hi Oliver! As NLP progresses, ChatGPT holds great potential. With further advancements, we can expect even better accuracy, enhanced contextual understanding, and improved handling of nuanced queries. There is also potential for domain-specific fine-tuning, enabling tailored analysis for various industries and use cases.
Hello everyone! Julia here. Kerry, I'm curious, would you recommend using pre-trained ChatGPT directly or fine-tuning it for specific data analysis tasks?
Hi Julia! It depends on the complexity and uniqueness of the data analysis task. If you have a large and diverse dataset, fine-tuning ChatGPT could yield better results. However, for more general tasks or when starting with limited data, using the pre-trained model and fine-tuning specific layers might suffice. It's always good to experiment and evaluate based on your specific requirements.
Hey all! Aiden here. Kerry, could you share any resources or tutorials for those interested in getting started with ChatGPT for data analysis?
Hi Aiden! Sure, there are some great resources available. OpenAI provides documentation on fine-tuning GPT models, which would be beneficial. Additionally, there are community tutorials and GitHub repositories with code examples showcasing the use of ChatGPT for data analysis. Exploring these resources will help you get started on the right track.
Hi everyone! Ella here. Kerry, how does ChatGPT handle multilingual data analysis? Can it analyze text in multiple languages simultaneously?
Hi Ella! ChatGPT is primarily trained on English text, so its performance may be better in English language analysis. While it can handle some level of multilingual tasks, it's not designed specifically for simultaneous analysis across multiple languages. For multilingual analysis, there are other NLP models and approaches available with dedicated multilingual capabilities.
Hello, all! Ava here. Kerry, based on your experience, can ChatGPT effectively analyze unstructured or noisy text data?
Hi Ava! ChatGPT can indeed handle unstructured and noisy text data to some extent, but it is always important to preprocess and clean the data to improve analysis accuracy. Noise reduction methods, data cleaning techniques, and domain-specific preprocessing can help in effectively utilizing ChatGPT for analyzing such data.
Hey everyone! Henry here. Kerry, do you see any potential ethical considerations when using ChatGPT for data analysis?
Hi Henry! Ethical considerations are crucial. As with any AI model, ChatGPT requires responsible usage. Ensuring data privacy, preventing biases, and being transparent about the limitations and risks associated with automated analysis are vital. Regular audits and addressing societal concerns through ethical guidelines contribute to responsible utilization of NLP models like ChatGPT.
Hello, everyone! Sophie here. Kerry, have you ever faced any challenges with scaling ChatGPT for large datasets?
Hi Sophie! Scaling ChatGPT for large datasets can be challenging due to computational resources required for training and inference. Breaking down the dataset into smaller parts or using techniques like mini-batching can help ease the process. Moreover, distributed training frameworks and infrastructure can be utilized to handle larger datasets efficiently.
Hey all! Isabella here. Kerry, how does ChatGPT handle ambiguous queries or incomplete text during analysis?
Hi Isabella! ChatGPT might struggle with ambiguous queries or incomplete text, as it heavily relies on context. In such cases, it's helpful to provide clearer context or prompt specifications to guide the analysis. Experimenting with multiple iterations and variations in input can assist in refining the responses and tackling ambiguity to some extent.
Hi everyone! Lucas here. Kerry, how do you think ChatGPT compares to other NLP models in terms of accuracy and efficiency for data analysis?
Hi Lucas! ChatGPT offers impressive accuracy for many tasks, especially when fine-tuned for specific analysis. However, it is a relatively larger model, which affects efficiency. For tasks where efficiency is critical, smaller and more specialized models might be more suitable. It's important to consider the trade-off between accuracy and efficiency based on the specific requirements of the data analysis task.
Hello, all! Lily here. Kerry, what are the potential risks associated with using ChatGPT for sensitive data analysis?
Hi Lily! Sensitive data analysis requires special care. If sensitive data is used, it's essential to ensure strict data privacy measures, comply with legal regulations, and take necessary steps to prevent unauthorized access. Anonymization and de-identification techniques can be employed to reduce risks associated with personally identifiable information (PII). Prioritizing data security should be the top concern when dealing with sensitive information.
Hey everyone! Mia here. Kerry, can you explain the concept of fine-tuning ChatGPT and its significance for data analysis?
Hi Mia! Fine-tuning ChatGPT involves training the pre-trained model on specific data to adapt it to a particular task or domain. This process helps improve its performance by making it more accurate and specialized for the desired analysis. Fine-tuning allows for customization and refinement based on the specific requirements, making ChatGPT a powerful tool for data analysis.
Hello, all! Adam here. Kerry, do you think ChatGPT can assist with exploratory data analysis (EDA) tasks?
Hi Adam! ChatGPT can indeed assist with certain aspects of exploratory data analysis. It can help in extracting insights, summarizing text, and understanding patterns within the data. However, EDA often requires statistical methods, visualizations, and domain expertise, which should be complemented with the capabilities of ChatGPT for holistic analysis.
Hey everyone! Noah here. Kerry, what are some considerations to keep in mind while evaluating the results obtained from ChatGPT for data analysis?
Hi Noah! When evaluating results from ChatGPT, it's important to consider factors like accuracy, relevance, and coherence of the responses. Additionally, evaluating against ground truth or expert knowledge, comparing with baseline models, and employing metrics specific to the analysis task can provide a comprehensive assessment. Regular evaluation and validation are essential to ensure the quality and reliability of the analysis.
Hello, all! Ethan here. Kerry, can ChatGPT be used for real-time sentiment analysis of social media data?
Hi Ethan! While ChatGPT is primarily designed for batch processing, it can still be used for sentiment analysis of social media data. However, real-time analysis would require appropriate infrastructure and systems to capture, preprocess, and analyze the data quickly. Considering the scale and velocity of social media data, specialized real-time sentiment analysis models might be more efficient for continuous monitoring and instant feedback.
Hey everyone! Avery here. Kerry, have you observed any limitations in ChatGPT's understanding of industry-specific jargon or technical terms during data analysis?
Hi Avery! ChatGPT might not inherently understand all industry-specific jargon or technical terms. However, by fine-tuning the model with domain-specific data or providing clear context, you can enhance its understanding and analysis capabilities within a specific industry or technical domain. Incorporating appropriate glossaries and data sources can help bridge the gap and improve accuracy in analyzing such specialized language.
Hello, all! Evelyn here. Kerry, what are the potential benefits of using ChatGPT for data analysis compared to traditional methods?
Hi Evelyn! ChatGPT offers several potential benefits over traditional methods. It can handle unstructured text data effectively, provide automated insights on a large scale, and even assist in generating summaries or responses based on the analysis. Its adaptability through fine-tuning and the ability to understand context make it a versatile tool for various data analysis tasks, reducing manual effort and improving efficiency.
Hey everyone! Jack here. Kerry, are there any particular programming languages or frameworks that integrate well with ChatGPT for data analysis?
Hi Jack! ChatGPT can be integrated with several popular programming languages and frameworks. Python is commonly used due to its rich ecosystem of NLP libraries and frameworks like TensorFlow, PyTorch, or Hugging Face's Transformers. These frameworks provide convenient interfaces and pre-built tools for interacting with and applying ChatGPT efficiently in data analysis pipelines.
Hello, all! Harper here. Kerry, can you share any success stories or notable use cases where ChatGPT significantly contributed to data analysis?
Hi Harper! ChatGPT has been successfully applied in various domains. Some notable use cases include analyzing customer feedback, automating email responses, summarizing large documents into key insights, and assisting in legal document analysis. It has also proven helpful in content classification and recommendation systems. These applications demonstrate the versatility and effectiveness of ChatGPT in data analysis tasks.
Hey everyone! Leo here. Kerry, what measures can be taken to ensure the ethical usage of ChatGPT for data analysis?
Hi Leo! Ethical usage is crucial when using ChatGPT. Implementing guidelines and policies for data privacy, bias prevention, and fair analysis are essential. OpenAI has shared guidelines and best practices for responsible AI usage, which should be followed. Encouraging transparency and incorporating diverse perspectives in the training data can also help mitigate potential biases and ensure ethical utilization of ChatGPT.
Thank you all for this insightful discussion! Your questions and perspectives have added valuable depth to the topic. Feel free to reach out if you have any more questions or thoughts regarding leveraging ChatGPT for data analysis.