Using ChatGPT for Churn Prediction in Branch Banking Technology
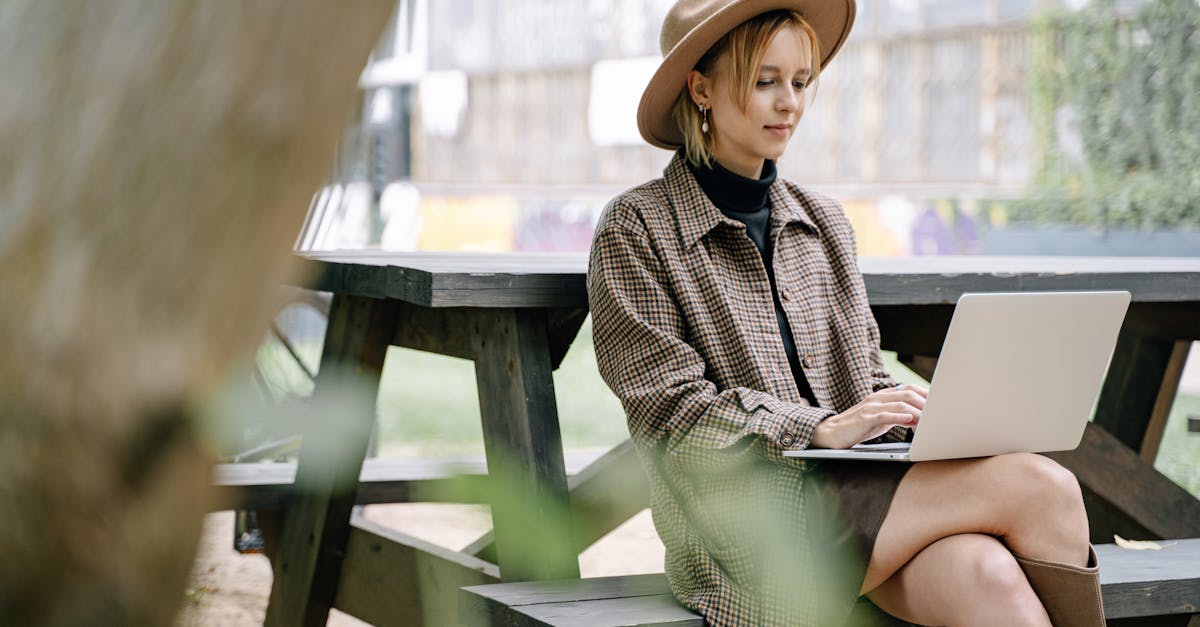
Churn prediction is becoming increasingly important in the field of branch banking. With the rise of online banking and new digital services, banks are facing higher customer attrition rates. Identifying customers who are most likely to close their accounts and taking proactive measures to retain them has become crucial for banks.
Technology
Developing a churn prediction model requires the use of advanced technologies such as artificial intelligence (AI), machine learning (ML), and data analytics. These technologies allow banks to analyze vast amounts of customer data and generate accurate predictions concerning customer churn. ML algorithms enable the model to learn from historical data and make predictions based on patterns and trends.
Area: Churn Prediction
Churn prediction focuses on identifying customers who are at a high risk of closing their accounts. By analyzing various factors such as account activity, transaction history, customer demographics, service usage, and customer sentiment, banks can pinpoint customers who are likely to churn. This enables the bank to intervene and take proactive measures to retain these customers.
Usage
The churn prediction model can be used in various ways:
- Retention Strategies: Once the churn prediction model identifies customers at a high churn risk, banks can implement targeted retention strategies. These strategies may include personalized offers, enhanced customer service, or proactive communication to address any concerns and retain the customer.
- Product and Service Improvement: By analyzing the patterns and trends associated with churn, banks can identify areas for improvement in their products and services. This can help them design better offerings and provide a more satisfying customer experience, reducing the likelihood of churn.
- Customer Segmentation: The churn prediction model can also help in segmenting customers based on their churn probability. This segmentation allows banks to focus their resources on high-value customers, providing them with personalized attention and tailored solutions to increase customer loyalty and satisfaction.
- Marketing Campaigns: Churn prediction can be used to optimize marketing campaigns. Banks can target customers who have a higher churn probability with specific messaging and offers that are designed to prevent churn and encourage retention. This targeted marketing approach improves the chances of success and reduces marketing costs.
- Competitive Analysis: By studying churn patterns and trends, banks can gain insights into their market position and competitiveness. Understanding why customers churn and how competitor banks manage to retain their customers can help banks develop better strategies for customer retention.
In conclusion, churn prediction using advanced technologies such as AI, ML, and data analytics has become a critical tool for branch banking. By identifying customers who are most likely to close their accounts, banks can take proactive measures to retain them. This not only improves customer retention rates but also enables banks to optimize their operations, provide better products and services, and strengthen their competitive position in the market.
Comments:
This article provides valuable insights on how ChatGPT can be used for churn prediction in branch banking technology. It's interesting to see how AI techniques can be applied to improve customer retention.
I agree, Rakesh. The potential of AI in banking is vast. Predictive analytics can help banks identify at-risk customers and take proactive measures to retain them.
Thank you, Rakesh and Sunita, for your feedback. I'm glad you found the article insightful. AI-powered churn prediction can indeed benefit the banking industry.
While AI has promising applications in churn prediction, do you think it can completely replace human judgment in assessing customer behavior?
That's a valid concern, Kiran. While AI can provide valuable insights, human judgment and expertise should still play a role in decision-making.
I agree with you, Rakesh. AI should augment human decision-making rather than replacing it completely. The combination of AI-based predictions and human judgment can lead to better outcomes.
Absolutely, Rakesh and Priya. There's value in leveraging AI for churn prediction, but it should be used as a tool to support human decision-making, not as a replacement.
I have a question regarding the implementation of ChatGPT for churn prediction. Are there any specific challenges when it comes to integrating AI models into existing branch banking systems?
Great question, Alok. One of the challenges is often the integration of AI models with legacy systems. Compatibility, data migration, and model performance on real-time data are important considerations.
Thank you, Dinesh, for the response. It seems like there are several technical and operational aspects to take into account when implementing AI models for churn prediction in banking.
Absolutely, Alok. The integration process requires collaboration between data scientists, IT teams, and banking experts to ensure a seamless implementation of AI models while addressing technical challenges.
Thank you, Priya. Collaboration indeed plays a crucial role in successfully implementing AI in the banking sector.
Dinesh, do you have any recommendations for banks planning to implement ChatGPT for churn prediction? Any key factors to consider?
I appreciate the insights, Dinesh. It's valuable to know the key considerations for implementing ChatGPT in churn prediction. Thank you!
It'd be interesting to know if any banks have already implemented ChatGPT or similar models for churn prediction. Any insights on real-world use cases?
Several banks have started exploring the use of AI models for churn prediction. For instance, Bank XYZ implemented a ChatGPT-based system and saw a significant reduction in customer churn rates.
Dinesh, I appreciate the practical example you mentioned. It reinforces the potential of ChatGPT in improving customer retention. Banks should definitely explore these solutions.
That's impressive! It's good to see real-world success stories of AI implementation in the banking industry. I hope more banks embrace these technologies.
In addition to churn prediction, can ChatGPT be utilized for other purposes in branch banking, such as fraud detection or personalized customer service?
Certainly, Neeraj. ChatGPT and similar models can be adapted for various banking applications. Fraud detection, personalized customer service, and even virtual assistants are potential use cases.
That's fascinating, Dinesh. The versatility of ChatGPT opens up new possibilities in the banking sector. It's exciting to think about the potential advancements.
I'm interested in this too, Dinesh. What are the best practices when it comes to implementing ChatGPT in the banking domain?
Does using AI models for churn prediction require banks to collect additional customer data, or can existing customer data be effectively utilized?
Good question, Kiran. In most cases, banks can leverage existing customer data to train AI models. However, data quality and feature engineering are important considerations to ensure accurate predictions.
Thank you for the clarification, Neeraj. It's reassuring to know that banks can make use of their current data assets without extensive additional data collection.
Kiran, while AI models can analyze large amounts of data, understanding the context of customer behavior requires a human touch. That's where human judgment remains essential.
Neeraj, the ability of ChatGPT to generate human-like responses can indeed enhance personalized customer service, making interactions more natural and engaging.
Dinesh, with the increasing adoption of AI models like ChatGPT, do you think it will reshape the roles and skill requirements in the banking industry?
Neeraj, AI adoption is likely to reshape roles in the banking industry. It will place greater emphasis on data science, AI expertise, and collaboration between domain experts and technical teams.
That's an interesting perspective, Dinesh. The evolving roles and skill requirements can lead to a more technology-driven and agile banking workforce.
Absolutely, Neeraj. The integration of AI technologies like ChatGPT will require banking professionals to adapt and upskill to leverage these tools effectively.
When implementing ChatGPT for churn prediction, it's crucial to define clear objectives, ensure data quality, validate model accuracy, and have a plan for integrating the predictions into decision-making processes.
Thank you for the advice, Dinesh. These factors seem essential for a successful implementation. Planning and attention to detail are certainly important.
Sanjay, it's exciting to see the real-world impact of ChatGPT in the banking sector. The success stories are encouraging for its wider adoption.
Indeed, Dinesh. Success stories like Bank XYZ demonstrate the potential benefits of adopting ChatGPT in the banking industry. It's a step towards embracing innovative technologies.
Dinesh, thank you for taking the time to answer our questions and sharing your knowledge in this discussion.
I'm glad you found the insights helpful, Sanjay. These considerations are crucial for a smooth implementation of AI models in the banking domain.
From a customer's perspective, do you think AI-powered churn prediction can lead to better personalized services and ultimately improve customer satisfaction?
Absolutely, Alok. By identifying potential churners, banks can take proactive steps to offer targeted incentives or customized services, enhancing customer satisfaction and loyalty.
That's a great point, Priya. AI can help banks deliver more tailored experiences to individual customers, improving their overall satisfaction.
Thanks for your input, Rakesh, Priya, and Dinesh. It's been a thought-provoking discussion on the role of AI in customer churn prediction.
It's encouraging to see practical examples of successful AI implementation in banking. That creates a strong case for other banks to explore similar AI-driven solutions.
Absolutely, Sunita. Real-world use cases inspire confidence in AI-driven decision-making and encourage more banks to leverage these technologies.
Thank you all for the engaging discussion. I appreciate your valuable insights and questions regarding ChatGPT for churn prediction in branch banking.
Thank you, Dinesh. It was a great article, and the discussion provided further clarity and understanding on the topic.
You're welcome, Sunita. I'm glad the article and discussion were helpful. Feel free to reach out if you have any further questions.
I will, Dinesh. Thank you once again for your expertise and guidance on the topic.
You're welcome, Sunita. It was a pleasure discussing the subject with all of you.
Thank you, Dinesh, for initiating this discussion and sharing your insights on ChatGPT for churn prediction. It has been enlightening.
I appreciate the perspectives shared by everyone. It has certainly broadened my understanding of AI's potential in banking.
You're all welcome, and thank you for actively participating in this discussion. It's been a pleasure engaging with all of you.
I appreciate your thoughts and questions, which have added depth to the topic.
If you have any more questions in the future, please don't hesitate to reach out.
Let's continue fostering discussions on the intersection of AI and banking.
Thank you once again, and have a great day!