Using ChatGPT for Fault Detection: Innovating Design for Manufacturing Technology
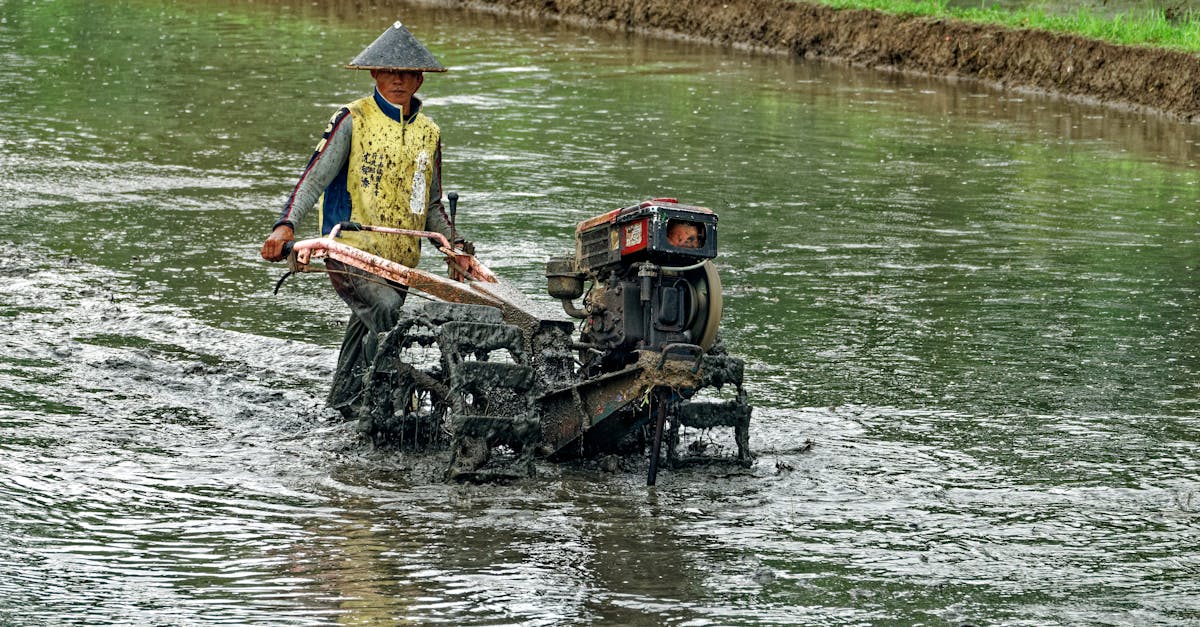
In the realm of manufacturing, the process of designing and producing consistent and high-quality goods is of utmost importance. As technology continues to advance, new tools and methods are being developed to ensure the success of this process. Design for Manufacturing (DFM) is one such technology that focuses on identifying potential faults in design plans before they can lead to production inconsistencies.
DFM combines various techniques and algorithms to analyze design blueprints and identify any flaws or errors that may impact the manufacturing process or the quality of the final product. ChatGPT-4, an advanced language model developed by OpenAI, holds vast potential in assisting with this crucial step of fault detection in manufacturing design plans.
ChatGPT-4, powered by its deep learning algorithms, possesses an in-depth understanding of various industries and domains, including manufacturing. By conversing with ChatGPT-4, engineers, designers, and manufacturers can leverage its capabilities to identify any faults or design inconsistencies that may have been overlooked during the initial review process.
The usage of ChatGPT-4 in fault detection starts by providing the model with the design plans or blueprints for analysis. The model can comprehend and interpret the design specifications, allowing it to evaluate the design from multiple perspectives. ChatGPT-4 can identify potential areas where the design may not align with the manufacturing requirements.
For instance, ChatGPT-4 can analyze the structural integrity of a design and assess potential weak points that may result in product failures or malfunctions. It can also evaluate material choices and highlight any potential limitations or incorrect usage of materials that could impact the manufacturing process or product durability.
Additionally, ChatGPT-4's natural language processing capabilities allow it to understand and analyze textual design descriptions, which are often prone to errors or ambiguities. By parsing through the descriptions, ChatGPT-4 can pinpoint conflicting or confusing information and help clarify them for designers or engineers, thus reducing the likelihood of faulty or inconsistent designs.
Furthermore, the extensive knowledge base of ChatGPT-4 enables it to learn from past manufacturing experiences and standards. It can provide recommendations based on best practices and industry-specific regulations, ensuring that design plans meet all necessary criteria for successful manufacturing.
By utilizing ChatGPT-4 for fault detection in design plans, manufacturers can reduce the risk of production inconsistencies and faulty products. The model's ability to identify design flaws before entering the production phase saves time, resources, and ultimately, safeguards the reputation and reliability of the final product.
In conclusion, Design for Manufacturing is a critical process in the manufacturing industry. Using advanced technologies such as ChatGPT-4, manufacturers can leverage the power of artificial intelligence and deep learning to detect faults and inconsistencies in design plans. By ensuring that design flaws are identified and rectified early on, manufacturers can streamline their production processes and deliver high-quality goods that meet customer expectations. ChatGPT-4's ability to assist in this area is a testament to the potential of AI in enhancing manufacturing processes and driving continuous improvement.
Comments:
Thank you all for reading my article on using ChatGPT for fault detection in manufacturing technology. I hope you find it informative. Please feel free to share your thoughts and opinions!
Great article, Sam! I found the concept of using ChatGPT for fault detection quite intriguing. It seems like a promising application for AI in the manufacturing industry.
I agree, Michael! The potential of AI in manufacturing is immense. Sam, can you provide some examples of how ChatGPT can be utilized in fault detection?
Certainly, Lisa! ChatGPT can be trained using historical data to identify patterns in manufacturing processes. By analyzing real-time production data, it can quickly detect anomalies and potential faults, enabling proactive maintenance and minimizing downtime.
That sounds amazing, Sam! I can see how it can significantly improve efficiency in manufacturing. Do you have any insights on the implementation challenges?
Great question, Ellen! One challenge is the need for high-quality training data to ensure accurate fault detection. It's also important to address the interpretability of the ChatGPT model's decision-making process, especially when it comes to critical manufacturing systems.
Sam, I have a question regarding data privacy. How can manufacturers ensure that sensitive production data is protected when implementing AI-based fault detection systems?
Data privacy is crucial, David. Manufacturers should implement robust security measures, including encryption and access controls, to safeguard sensitive production data. They should also comply with relevant data privacy regulations to protect both their own interests and customer data.
Sam, what level of expertise is required to develop and deploy ChatGPT models for fault detection in manufacturing? Can manufacturers with limited AI knowledge leverage this technology?
Manufacturers with limited AI knowledge can still leverage this technology, David. Collaboration with AI experts or partnering with experienced AI solution providers can help bridge the knowledge gap. While some level of expertise is required for training and fine-tuning the models, there are user-friendly tools and platforms available that simplify the development and deployment processes.
I'm curious, Sam, how scalable is ChatGPT for large-scale manufacturing operations? Can it handle the complexity and volume of data generated by extensive production lines?
Scalability is important, Emily. ChatGPT can be optimized to handle the complexity and volume of data in large-scale manufacturing operations. By utilizing distributed systems and advanced hardware, it can process and analyze vast amounts of data in real-time.
Sam, what are the limitations of using ChatGPT for fault detection? Are there specific types of faults that it may struggle to detect?
Good question, Daniel. ChatGPT relies on historical data to identify patterns, so if a fault is entirely new or significantly different from what it has learned, it may struggle to detect it accurately. Continuous model improvement and human oversight are essential for addressing such limitations.
Sam, have you encountered any challenges in labeling and annotating the training data for ChatGPT in fault detection?
Labeling training data can be challenging, Daniel. It requires domain expertise and clear guidelines to ensure consistent and accurate annotation of faults. Involving subject matter experts and conducting thorough quality control checks are essential to maintain the integrity of the training dataset.
I'm impressed by the potential of ChatGPT in manufacturing. Sam, how do you see this technology evolving in the future?
The future of AI in manufacturing looks promising, Michelle. We can expect advancements in combining multiple AI models, such as vision-based systems, with ChatGPT to increase fault detection accuracy further. Additionally, the integration of real-time sensor data will greatly enhance the capabilities of AI-based systems.
Sam, do you think ChatGPT can entirely replace manual fault detection in manufacturing, or is it more of a complementary tool?
Excellent question, Robert. ChatGPT is not meant to replace manual fault detection entirely, but it can greatly augment and assist human operators by providing real-time insights and early fault warnings. It allows proactive maintenance and empowers operators to make data-driven decisions more efficiently.
Sam, what kind of industries can benefit the most from implementing ChatGPT for fault detection? Is it limited to specific sectors?
Good question, Sophia. ChatGPT can be applied across various industries where fault detection and proactive maintenance are critical, such as automotive, electronics, aerospace, and even pharmaceutical manufacturing. It has versatile applications and can adapt to different manufacturing environments.
To add to Jacob's question, Sam, what volume of training data is generally required for effective ChatGPT models in fault detection?
Good point, Sophia. The volume of training data required can vary depending on the complexity of the manufacturing processes and the desired detection accuracy. In general, a larger and more diverse training dataset tends to improve the model's performance. However, it's essential to strike a balance, avoiding overfitting and focusing on relevant data.
As an engineer, I find this article inspiring. Sam, how long does it usually take to train a ChatGPT model for fault detection in manufacturing?
Training time can vary, Brian. It depends on factors like the complexity of the manufacturing process, the volume of training data, and the computational resources available. However, with advanced hardware and optimization techniques, it's possible to train a ChatGPT model for fault detection within a reasonable timeframe.
Sam, I'm interested in the overall cost implications of implementing ChatGPT for fault detection. Could you shed some light on the upfront and long-term costs for manufacturers?
Certainly, Rachel. The upfront costs include developing and training the ChatGPT model, setting up the necessary infrastructure, and ensuring data compatibility. However, in the long term, the benefits can outweigh the costs, including improved efficiency, reduced downtime, and optimized maintenance schedules.
Great article, Sam! I'm curious about the accuracy of ChatGPT in real-world manufacturing environments. Have there been any case studies or experiments conducted?
Thank you, Alex! Yes, there have been several case studies and experiments evaluating ChatGPT's accuracy in real-world manufacturing. The results have been promising, showing its effectiveness in proactive fault detection and improving overall manufacturing processes.
Sam, are there any ethical considerations that manufacturers should keep in mind when implementing AI-based fault detection systems?
Absolutely, William. Ethical considerations are crucial. Manufacturers should ensure transparency in how they utilize AI, addressing any biases in the training data and making clear the limitations of AI-based systems. Additionally, they should prioritize human oversight and accountability in decision-making.
Sam, what are the hardware requirements for implementing ChatGPT in manufacturing facilities? Do manufacturers need to make significant investments?
Good question, Grace. The hardware requirements can vary depending on the scale of implementation and the desired performance. While it may require some initial investment in robust computing resources, manufacturers can leverage cloud platforms and distributed systems to minimize hardware costs.
Sam, do you have any predictions on the adoption rate of AI-based fault detection in manufacturing? How long do you think it will take for widespread implementation?
Predicting adoption rates is challenging, Liam. However, with the increasing focus on automation and digital transformation in manufacturing, I believe we will see a gradual but steady adoption of AI-based fault detection systems. Widespread implementation may take several years as manufacturers assess benefits and overcome implementation challenges.
Sam, I'm curious about the integration of ChatGPT with existing manufacturing systems. How can manufacturers effectively integrate this technology without disrupting their current operations?
Integration is a key consideration, Olivia. Manufacturers should plan the integration process carefully, collaborate with AI experts, and conduct thorough testing to minimize disruptions. It's important to start with pilot projects and gradually scale up, ensuring a smooth transition and effective collaboration between AI tools and existing systems.
Sam, what are some of the real-world benefits that manufacturers have experienced by implementing ChatGPT for fault detection?
Good question, Andrew. Manufacturers have reported improved operational efficiency, reduced downtime, and optimized maintenance schedules as some of the key benefits. Early fault warnings provided by ChatGPT enable proactive action, preventing costly breakdowns and ensuring high-quality production.
I can see the potential, Sam! However, are there any legal implications or regulations that manufacturers need to consider when implementing AI-based fault detection?
Absolutely, Natalie. Manufacturers should comply with relevant regulations regarding data privacy, security, and ethical AI usage. Depending on the industry and location, there may be sector-specific regulations that need to be considered. Staying informed about legal implications is essential for successful implementation.
Sam, how does the accuracy of ChatGPT compare to traditional fault detection methods in manufacturing? Are there any specific metrics to assess its performance?
Good question, Emma. ChatGPT's accuracy can be comparable to or even surpass traditional fault detection methods when trained and fine-tuned correctly. Metrics like precision, recall, and F1 score can be used to assess its performance, along with domain-specific metrics based on the nature of faults in manufacturing processes.
Sam, are there any strategies to deal with class imbalance in the training data, considering that some fault types may occur less frequently?
Great question, Emma! Class imbalance can be addressed by employing techniques like oversampling minority classes, undersampling majority classes, or utilizing data augmentation methods. It's crucial to ensure a balanced representation of different fault types to prevent biases and maximize the model's fault detection capabilities.
Sam, do you have any tips for manufacturers who are considering implementing ChatGPT for fault detection? Where should they start?
Great question, Sophie! Manufacturers should begin by assessing their specific needs and processes, identifying areas where AI-based fault detection can bring the most value. Starting with small-scale pilot projects allows for testing and validation before scaling up. Collaborating with AI experts and seeking industry-specific guidance can also help ensure successful implementation.
Sam, what considerations should be taken when selecting and preparing the training data for ChatGPT in manufacturing?
Excellent question, Jacob! The training data should be representative of the manufacturing processes and faults manufacturers want to detect. It should include a wide range of fault scenarios while ensuring data quality and accuracy. Data cleaning and preprocessing are essential to remove noise and irrelevant information, enhancing the model's performance.