Using ChatGPT for Multivariate Analysis in Statistics
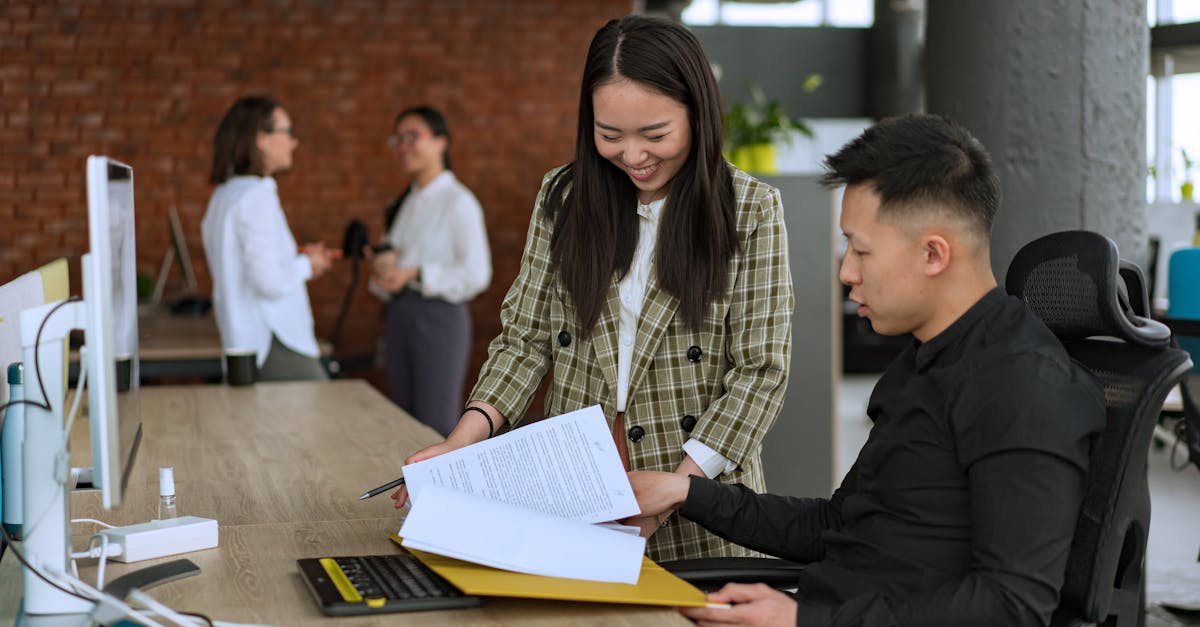
Statistics is a powerful field that enables us to make sense of data and draw meaningful conclusions. Multivariate analysis, a branch of statistics, focuses on the analysis of multiple variables simultaneously. In this article, we will explore how ChatGPT-4, a cutting-edge language model, can help us understand and explain key techniques in multivariate analysis and their practical applications.
Principal Component Analysis (PCA)
Principal Component Analysis, or PCA, is a widely used technique in multivariate analysis. It aims to identify the most important patterns and relationships in data by reducing the dimensionality of the original variables. ChatGPT-4 can provide detailed explanations of PCA, including the underlying mathematics and step-by-step procedures.
Factor Analysis
Factor Analysis is another powerful technique that allows us to uncover latent variables and identify underlying factors from a set of observed variables. ChatGPT-4 can help users understand the different types of factor analysis, such as exploratory factor analysis and confirmatory factor analysis, as well as their applications in various fields.
Cluster Analysis
Cluster Analysis is a method used to group similar objects or individuals based on their characteristics. It helps in identifying natural clusters within a dataset and understanding the similarities and differences between different groups. ChatGPT-4 can guide users through the various types of cluster analysis, such as hierarchical clustering and k-means clustering, explaining their strengths and limitations.
Discriminant Analysis
Discriminant Analysis is a statistical technique used to determine which variables discriminate between two or more groups. It helps in understanding the factors that contribute significantly to group separation and classification. ChatGPT-4 can offer insights into the different types of discriminant analysis, such as linear and quadratic discriminant analysis, and their interpretation.
Applications and Interpretation
Understanding the techniques mentioned above is essential, but knowing how to apply them to real-world problems is equally important. ChatGPT-4 can provide examples of practical applications, such as using PCA to analyze and visualize complex datasets or utilizing cluster analysis to segment customers for targeted marketing campaigns.
Furthermore, ChatGPT-4 can help users interpret the results obtained from these multivariate analysis techniques. It can explain how to interpret factor loadings in factor analysis, interpret cluster memberships, or understand the discriminant functions in discriminant analysis.
Conclusion
In conclusion, ChatGPT-4 is a valuable resource for anyone looking to explore and understand various techniques in multivariate analysis. Its ability to explain the concepts, methodologies, and applications of principal component analysis, factor analysis, cluster analysis, and discriminant analysis makes it an invaluable tool for statisticians, researchers, and data analysts alike.
By leveraging the power of ChatGPT-4, users can gain a deeper understanding of multivariate analysis, apply these techniques to real-world problems, and make informed decisions based on the insights obtained from their data.
Comments:
Great article, Virginia! ChatGPT seems like a powerful tool for multivariate analysis in statistics. It could revolutionize the way we approach data analysis and modeling.
I agree, Brian. The idea of using language models like ChatGPT for statistical analysis is fascinating. It could potentially automate tasks that currently require manual intervention and expertise.
Thank you, Brian and Amy! I'm glad you find the concept interesting. Indeed, ChatGPT offers new possibilities for statistical analysis. It can assist in exploring data relationships and generating insights.
As exciting as it sounds, how reliable is ChatGPT for complex statistical analysis? Has it been thoroughly tested in various scenarios, or are there limitations we should be aware of?
Valid concern, David. ChatGPT has its limitations. While it can provide valuable insights, it's important to verify its results and cross-validate with other methods. It's still an AI model, after all.
I've been using ChatGPT for statistical analysis, and I must say it's been quite helpful. It can quickly generate alternative models and perform sensitivity analysis. Definitely a time-saver!
Jennifer, that's awesome to hear! Could you provide some examples of how ChatGPT has assisted you in your statistical analysis? I'm curious about its practical applications.
Certainly, Brian. ChatGPT helped me identify hidden patterns in complex datasets, generate hypotheses, and even suggest possible model improvements. It acted as a valuable virtual collaborator!
Jennifer, do you have any advice for effectively utilizing ChatGPT in statistical analysis? Are there any specific guidelines or best practices to follow?
Absolutely, Amy. When using ChatGPT, it's crucial to clearly define the problem and have a well-curated dataset. It's also important to interpret and validate the results critically.
While ChatGPT seems promising, I wonder about its potential biases. Do you think the model can effectively handle and mitigate biases commonly present in statistical analysis?
Good point, Ethan. Bias mitigation is indeed a concern. ChatGPT can inadvertently learn biases from its training data. It's necessary to carefully curate the data and monitor its outputs for biases.
Virginia, what steps can we take to ensure the outputs from ChatGPT are fair and unbiased when conducting multivariate analysis? Are there any established practices or methodologies?
In the context of multivariate analysis, David, conducting fair analysis involves ensuring representative data, regular model evaluation, and adversarial testing to identify and mitigate potential biases.
I'm curious to know more about the implementation. What programming languages and libraries are commonly used when integrating ChatGPT with statistical analysis tools?
Good question, Sophia. ChatGPT relies on the OpenAI GPT model, which can be used with various programming languages. Popular options are Python with libraries like TensorFlow or PyTorch.
Thank you, Virginia! That's helpful. I'll explore integrating ChatGPT with statistical analysis in Python using TensorFlow. Exciting possibilities ahead!
While ChatGPT shows potential, I'm concerned about the privacy and security implications of integrating it into statistical analysis pipelines. How can we address these concerns?
Valid concern, Alex. When integrating ChatGPT, it's important to consider data privacy and security. Applying anonymization techniques, secure data storage, and complying with data regulations are essential.
I wonder about the computational requirements when using ChatGPT for multivariate analysis. Does it require substantial computing power, or can it run on standard hardware?
Good question, Liam. ChatGPT can be computationally demanding, especially with larger datasets or complex analyses. Running it on hardware with GPUs or utilizing cloud-based solutions can improve performance.
ChatGPT sounds fascinating! Do you think it can also help with dimensionality reduction or feature selection, two important aspects of statistical analysis?
Indeed, Julia. While not its primary focus, ChatGPT can offer guidance in dimensionality reduction or suggest relevant features to explore. It can assist in refining the analysis process.
That's impressive. It seems like ChatGPT can contribute to every stage of statistical analysis, from data exploration to model refinement. It's a versatile tool to have!
Has anyone experimented with combining ChatGPT with traditional statistical models like linear regression or decision trees? I'm curious to know about the results.
Sarah, I've used ChatGPT alongside traditional models. It can complement them by suggesting alternative approaches or revealing overlooked patterns. It's worth exploring!
Thanks, Brian! I'll definitely give it a try. The potential synergy between ChatGPT and traditional models can lead to more comprehensive and accurate statistical analyses.
I'm curious about any known limitations of ChatGPT when applied to statistical analysis. Are there any specific scenarios or types of data where it might not be suitable?
Good question, Olivia. ChatGPT may face challenges when handling noisy or unstructured data, and it might struggle with rare events or outliers. Domain-specific expertise can help address these limitations.
Thank you, Virginia! I'll keep those factors in mind when considering the applicability of ChatGPT in statistical analysis projects.
ChatGPT seems like a game-changer for exploratory data analysis. Are there any visualizations or interactive features that can be integrated to enhance the analysis process?
Absolutely, Henry! While ChatGPT focuses on language-based interaction, it can be combined with visualization libraries like matplotlib or plotly to create interactive visual analysis tools.
That's fantastic! The combination of natural language interaction and interactive visualizations would take data exploration to a whole new level. I can't wait to try it out!
I'm curious to know if ChatGPT can handle time series analysis effectively. Has it been tested in such scenarios, and how does it compare to traditional time series models?
Andrea, ChatGPT has shown promising results in time series analysis. It can capture patterns, suggest appropriate models, and aid in forecasting. Comparisons with traditional models are context-dependent but worth exploring.
Thank you, Virginia! It's exciting to think about a unified tool like ChatGPT supporting multiple aspects of statistical analysis, including time series analysis.
Are there any resources or tutorials available to get started with using ChatGPT for statistical analysis? I'd like to dive deeper into it.
Liam, the OpenAI website provides documentation and resources to get started with ChatGPT. There are also community-driven tutorials and collaborative forums where you can learn and share experiences.
Thank you, Virginia. I'll explore those resources and connect with the community to expand my understanding of using ChatGPT for statistical analysis.
Liam, based on my experience, ChatGPT can run well on standard hardware for smaller datasets and less computationally intensive tasks. However, for larger datasets or complex analyses, additional computing power can significantly improve performance.
Considering the potential impact of ChatGPT on statistical analysis, what do you think its limitations might be? Are there any risks associated with relying heavily on AI-assisted analysis?
Emily, valid concerns. ChatGPT might be limited in handling domain-specific knowledge, requiring expert guidance in certain contexts. Over-reliance on AI-assisted analysis without critical thinking could lead to biased or flawed results.
Thank you, Virginia. It's crucial to strike a balance between leveraging AI assistance and domain expertise to ensure robust and reliable statistical analysis outcomes.
Has anyone encountered ethical challenges when using ChatGPT in statistical analysis? How can we address ethical concerns that might arise?
Ethical challenges can arise, Mason. It's essential to prioritize fairness, transparency, and accountability. Establishing evaluative procedures, involving multiple perspectives, and upholding ethical guidelines are key to address such concerns.
Thank you, Virginia. By being mindful of ethical considerations and fostering a responsible AI culture, we can leverage ChatGPT for statistical analysis while minimizing potential ethical risks.
Considering the rapid advancements in AI, where do you see the future of AI-assisted statistical analysis heading? Any predictions?
Tom, the future of AI-assisted statistical analysis is exciting. We can anticipate advanced models, improved interpretability, increased automation, and AI-human collaboration becoming more seamless. The potential is vast!
I couldn't agree more, Virginia. The continued integration of AI technologies like ChatGPT will undoubtedly shape the future of statistical analysis, making it more efficient and insightful.
Thank you all for engaging in this insightful discussion on using ChatGPT for multivariate analysis in statistics. Your contributions and questions have been valuable. Let's continue exploring the potential of AI in advancing statistical analysis!