Using ChatGPT for Predictive Analytics in Energy: Expanding Possibilities with conversational AI
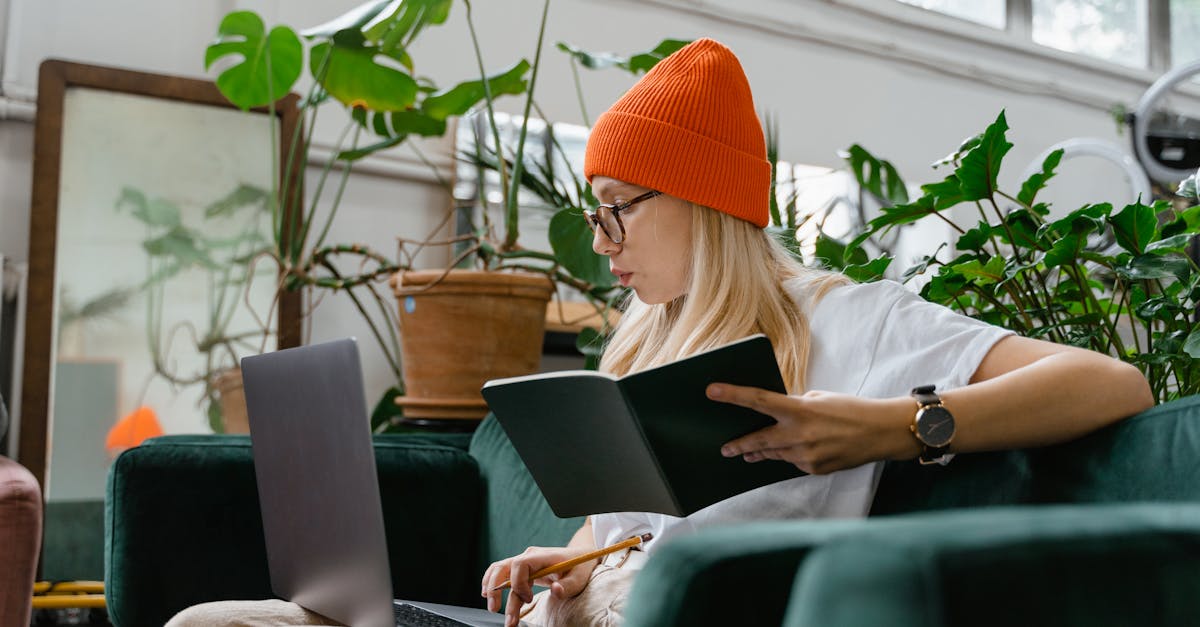
In today's world, where energy consumption and sustainability are major concerns, the application of predictive analytics in energy management has gained significant importance. Predictive analytics is a powerful technology that uses statistical algorithms and machine learning techniques to analyze historical data and predict future outcomes. In the energy sector, predictive analytics plays a crucial role in understanding energy usage patterns and forecasting energy demand, enabling the development of efficient energy management solutions.
Understanding Predictive Analytics
Predictive analytics involves the collection, analysis, and interpretation of data to uncover patterns, insights, and predictions. In the context of energy management, this technology focuses on predicting energy usage based on various factors such as weather conditions, time of day, occupancy patterns, and historical data.
By using predictive analytics, energy service providers and facility managers can develop accurate forecasts and make informed decisions regarding energy supply and demand. This helps in optimizing energy usage, reducing wastage, and improving overall energy efficiency.
Applications of Predictive Analytics in Energy Management
1. Demand Forecasting: Predictive analytics can be utilized to forecast energy demand by analyzing historical data and real-time factors such as weather conditions and building occupancy. This enables energy service providers to optimize energy generation and allocation, reducing costs and ensuring a steady supply of energy.
2. Load Balancing: Predictive analytics helps in balancing the load across the energy grid by predicting peak demand periods. By forecasting peak usage, energy service providers can distribute energy resources efficiently, reducing the risk of blackouts and enhancing grid stability.
3. Energy Efficiency: By analyzing consumption patterns and identifying areas of high energy usage, predictive analytics can provide insights for developing energy efficiency strategies. Facility managers can use these insights to implement energy-saving measures, such as optimizing HVAC systems, improving insulation, and promoting energy-conscious behavior among occupants.
4. Equipment Maintenance: Predictive analytics can also be used to predict equipment failures and maintenance requirements. By analyzing data from sensors and historical records, energy providers can proactively identify faulty equipment and schedule maintenance activities, reducing downtime and ensuring smooth operations.
Benefits of Predictive Analytics in Energy Management
1. Cost Reduction: By accurately predicting energy usage and demand, energy service providers can optimize resource allocation, reduce oversupply, and avoid unnecessary expenses. This leads to significant cost savings for both energy providers and consumers.
2. Enhanced Sustainability: Predictive analytics enables the development of energy efficiency strategies, reducing carbon footprint and promoting sustainable practices. By optimizing energy generation, consumption, and distribution, environmental impact can be minimized.
3. Improved Decision Making: Predictive analytics provides valuable insights and predictions, empowering decision-makers to make informed choices regarding energy management. By leveraging accurate forecasts, they can effectively plan for future energy needs and address potential challenges.
4. Increased Operational Efficiency: By optimizing energy usage and maintenance activities, predictive analytics helps in improving operational efficiency. It enables energy providers to predict equipment failures, schedule maintenance activities, and streamline resource allocation, resulting in improved performance and reduced downtime.
Conclusion
Predictive analytics plays a vital role in energy management, allowing energy service providers and facility managers to accurately forecast energy demand, optimize energy consumption, and develop efficient energy management solutions. By leveraging historical and real-time data, this technology empowers decision-makers to make data-driven choices, leading to cost savings, increased sustainability, and improved operational efficiency. As the demand for energy continues to grow, predictive analytics will play an increasingly significant role in developing sustainable and efficient energy management practices.
Comments:
Thank you all for taking the time to read my article! I'm excited to hear your thoughts on using ChatGPT for predictive analytics in the energy sector.
Great article, Vicki! ChatGPT seems like a powerful tool for enhancing predictive analytics in the energy industry. Can you share some specific use cases where it has been successfully applied?
Thanks, Erin! ChatGPT has been used in energy demand forecasting, where it analyzes historical data to predict future energy consumption. It has also been applied to optimize energy distribution networks and improve maintenance scheduling.
I'm curious about the accuracy of ChatGPT in energy predictions. Does it outperform other traditional forecasting models?
Good question, Ethan! ChatGPT has shown competitive performance compared to traditional models in energy prediction tasks. Its advantage lies in its ability to interpret unstructured data and capture complex patterns that might be missed by other models.
I can see how the conversational aspect of ChatGPT can make predictive analytics more accessible to non-technical users. Is there a steep learning curve for implementing and using the tool?
That's a great point, Catherine. While ChatGPT is designed to be user-friendly, implementing it for predictive analytics does require some technical expertise. However, there are resources available, such as API documentation and tutorials, to guide users through the process.
I'm concerned about the potential bias in ChatGPT's predictions, especially regarding energy consumption in marginalized communities. How does the tool address this issue?
Valid concern, Dylan. ChatGPT's predictions are based on training data, which can include biases. However, efforts are made to ensure fairness by using diverse and representative training data. Regular evaluations and feedback loops are implemented to address biases and improve the tool's performance.
I think ChatGPT's versatility makes it a promising tool for other industries as well. Can you provide some insights into how it can be utilized outside of the energy sector?
Absolutely, Sophia! ChatGPT can be applied to customer support, virtual assistants, content generation, and more. Its ability to understand and generate human-like text opens up possibilities for various use cases across industries.
This article convinced me to explore ChatGPT for predictive analytics in my energy company. Are there any limitations or challenges to be aware of before implementation?
Glad to hear that, Liam! It's important to note that ChatGPT may generate plausible but incorrect answers, so careful validation and testing are necessary. Also, the tool relies on historical data, so sudden shifts or unprecedented events may affect its predictions. It's always good to have validation from domain experts.
I'm concerned about the computational resources required for implementing ChatGPT. Are there any recommendations for managing the tool's resource needs?
Valid concern, Oliver. Managing the computational resources is crucial for utilizing ChatGPT effectively. It's recommended to batch requests and cache outputs whenever possible. Monitoring resource usage and optimizing the implementation, such as using GPU acceleration, can also help manage the tool's resource requirements.
Has ChatGPT been tested with real-world energy data? I'd like to see some results before considering its adoption.
Definitely, Nora! ChatGPT has been tested and validated with real-world energy datasets. It has shown promising results in energy prediction tasks and has the potential to enhance decision-making in the industry.
How does ChatGPT handle data privacy and security? Since it's a cloud-based tool, are there any risks associated with sensitive data?
Data privacy and security are essential considerations, Daniel. ChatGPT processes user inputs on the OpenAI servers, but as of March 1st, 2023, OpenAI retains the data for 30 days and no longer uses it to improve their models. It's important to review OpenAI's privacy policy and take necessary precautions to protect sensitive information.
I'm curious if ChatGPT requires continuous training with new data to maintain its accuracy over time?
Great question, Emma! ChatGPT can benefit from retraining with new data to adapt to changing patterns and maintain accuracy over time. Regular updates and fine-tuning can help ensure optimal performance.
What kind of technical infrastructure is needed to implement ChatGPT? Is it compatible with popular programming languages?
Good question, Isabella. ChatGPT provides an API, offering compatibility with popular programming languages such as Python, JavaScript, etc. You need a functioning web server and libraries to make API requests. OpenAI provides detailed documentation to guide users through the implementation process.
Do you think ChatGPT can completely replace traditional predictive models? Are there scenarios where traditional models still outperform ChatGPT?
It's unlikely that ChatGPT will completely replace traditional predictive models, James. While it excels in certain areas, there are still scenarios where traditional models, especially when working with structured data and well-defined features, may outperform ChatGPT. A hybrid approach combining the strengths of both could be a promising direction.
How does ChatGPT handle uncertainties and provide confidence intervals for its predictions?
Good question, Hannah! ChatGPT doesn't provide explicit confidence intervals, but it can offer suggestions and alternative scenarios based on the input uncertainty. It's essential to interpret and evaluate its responses while considering the inherent uncertainties.
Are there any ongoing research efforts to improve ChatGPT's capabilities in predictive analytics?
Absolutely, Mason! OpenAI is actively researching and optimizing ChatGPT. They are continuously improving the model, exploring techniques to address its limitations, and incorporating user feedback to enhance its performance in predictive analytics and other domains.
What is the approximate training time for ChatGPT? Does it require any specialized hardware to train the models?
Training ChatGPT is a labor and resource-intensive process, Naomi. It involves training on powerful hardware, including GPUs and TPUs. The exact training time can vary depending on the scale and specific requirements, but it typically takes several days or even weeks to train a high-performing model.
How does ChatGPT handle out-of-distribution or out-of-domain requests? Does it gracefully handle such scenarios?
ChatGPT can sometimes generate responses that sound plausible but may be incorrect or unrelated to the input, especially for out-of-distribution or out-of-domain requests, Robert. Handling and mitigating such scenarios is an ongoing challenge, and user feedback plays a crucial role in identifying and addressing these issues.
Can ChatGPT be used for real-time energy monitoring and anomaly detection?
While ChatGPT's primary focus is on generating text-based responses, it can be integrated with other systems for real-time energy monitoring and anomaly detection, Eva. By leveraging its predictions and incorporating real-time data feeds, it has the potential to enhance such applications.
Are there any free alternatives or open-source options available for those who want to experiment with similar conversational AI models like ChatGPT?
Certainly, Lucas! OpenAI provides some free access to ChatGPT, but there are also alternative open-source models like GPT-3 by EleutherAI and GPT-J by EleutherAI and Hugging Face. These models allow researchers and developers to experiment and build upon the advances in conversational AI.
What is the general latency or response time when making predictions with ChatGPT?
The response time or latency can vary, Sophie. With the API, users typically send requests and receive responses within a few seconds, but the actual timing depends on factors like network conditions and server load. OpenAI is continuously working on optimizations to reduce the latency and improve the overall user experience.
Could you provide some resources or documentation for beginners who want to start implementing ChatGPT for predictive analytics?
Certainly, William! OpenAI provides comprehensive documentation and guides on their website, including API documentation, tutorials, and examples to help beginners get started with implementing ChatGPT for predictive analytics. It's a great resource to explore.
Are there any ethical considerations to keep in mind while using ChatGPT for predictive analytics in the energy industry?
Ethical considerations are crucial when utilizing any AI tool, Penelope. Transparency, fairness, data privacy, and addressing biases should always be key considerations. Responsible and ethical use of ChatGPT in the energy industry, or any other domain, is essential for its positive impact.
Are there any limits on the number of requests or tokens when using ChatGPT through the API?
Yes, Luke. Usage limits and costs for ChatGPT via the API vary depending on the subscription plan. OpenAI provides detailed pricing information and documentation that outlines the limits on the number of requests and tokens. It's important to review those details and plan accordingly.
How adaptable is ChatGPT to various energy market dynamics and regulatory frameworks?
ChatGPT's adaptability to different energy market dynamics and regulatory frameworks is dependent on the availability and relevance of training data, Evelyn. While it can provide valuable insights, it's essential to consider the specific contexts, local regulations, and expert knowledge while interpreting and making decisions based on its predictions.
Could ChatGPT be used to help consumers make informed decisions about their energy consumption and promote sustainability?
Absolutely, Maxwell! ChatGPT has the potential to assist consumers in understanding their energy consumption patterns, suggesting energy-saving strategies, and promoting sustainability. By empowering individuals with personalized insights, it can contribute to more informed and sustainable energy usage.
Thank you all for your engaging comments and insightful questions! It was wonderful discussing the possibilities of ChatGPT in predictive analytics for the energy sector with you. Feel free to reach out if you have any further inquiries or thoughts!