Using ChatGPT in Risk Analysis for Mathematical Programming Technology
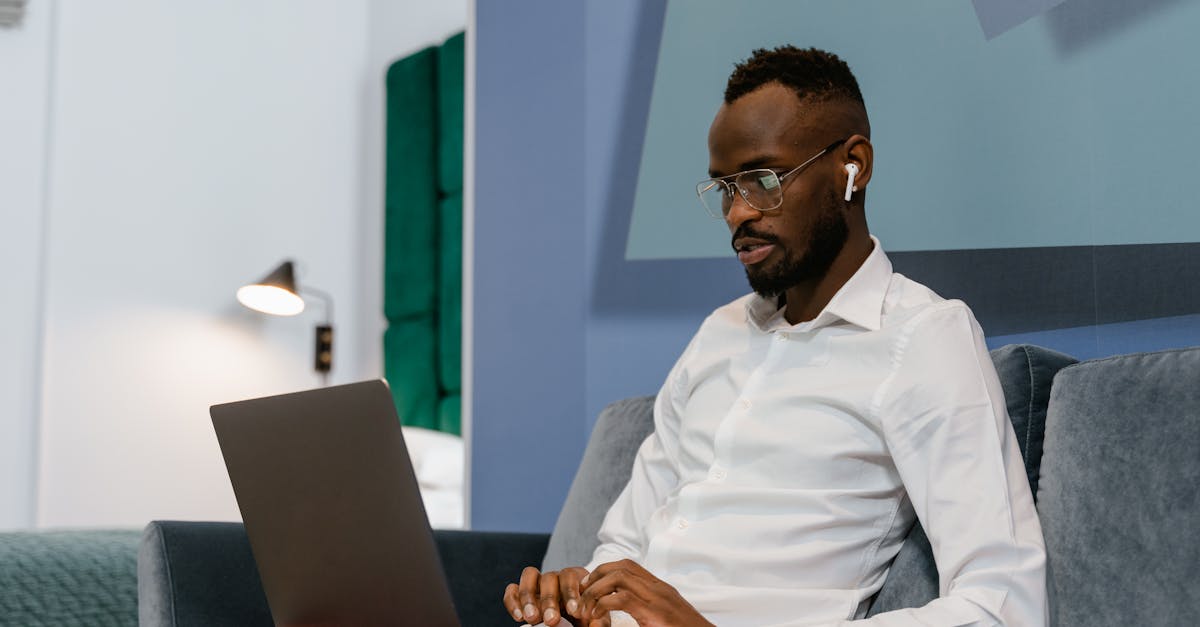
Risk analysis plays a significant role in various fields, including finance, engineering, and environmental science. It involves assessing and quantifying potential risks associated with different actions or decisions. Mathematical programming techniques have emerged as powerful tools in risk analysis, enabling analysts to analyze the potential for severe outcomes in response to chosen actions. This article explores the application of mathematical programming in risk analysis and its usage in various domains.
What is Mathematical Programming?
Mathematical programming, also known as optimization, is a branch of operations research that deals with the optimization of complex systems. It involves formulating mathematical models to represent decision-making problems and finding the best solutions within given constraints. Mathematical programming can be used to solve problems involving resource allocation, scheduling, production planning, and more.
Applying Mathematical Programming to Risk Analysis
Risk analysis aims to identify potential risks and estimate their likelihood and impact. By integrating mathematical programming into risk analysis, analysts can effectively evaluate the potential for severe outcomes in response to chosen actions. Mathematical programming models can incorporate various risk factors and constraints to simulate different scenarios and identify optimal solutions.
For example, in finance, mathematical programming can be used to assess the risks associated with investment portfolios. By considering various parameters, such as asset returns, correlations, and constraints, analysts can optimize portfolio allocation to minimize risks and maximize returns. This allows investors to make informed decisions based on their risk appetite and objectives.
In engineering, mathematical programming can be utilized to evaluate the potential risks and consequences of design choices. By considering factors such as material properties, load conditions, and safety constraints, engineers can optimize designs to minimize failure or maximize performance under uncertain conditions. This helps in ensuring the safety and reliability of engineered systems.
In environmental science, mathematical programming can aid in risk analysis related to disaster management and mitigation. By incorporating data on geographical features, weather patterns, and available resources, decision-makers can optimize preparedness strategies to minimize the potential impact of natural disasters. This allows for effective allocation of resources and timely response to minimize losses.
Benefits and Limitations
The usage of mathematical programming in risk analysis offers several benefits. It provides a quantitative framework to assess and compare potential risks, facilitating informed decision-making. It allows for the exploration of multiple scenarios and the identification of optimal solutions to mitigate risks. Furthermore, mathematical programming can handle complex problems with numerous variables and constraints, enabling a comprehensive analysis of risk factors.
However, mathematical programming also has certain limitations. It relies on the accuracy of input data and assumptions, which can introduce uncertainties. The models may oversimplify real-world complexities, leading to potential bias or inaccuracies. Additionally, the computational complexity of mathematical programming can be a challenge, especially for large-scale problems requiring significant computational resources and time.
Conclusion
The application of mathematical programming in risk analysis has proven to be a valuable approach across various domains. By leveraging mathematical models and optimization techniques, analysts can evaluate potential risks and identify optimal solutions to mitigate them. Although mathematical programming has certain limitations, its benefits in facilitating informed decision-making and comprehensive risk analysis outweigh the challenges. With further advances in computational capabilities and data availability, mathematical programming will continue to play a crucial role in risk analysis in the future.
Comments:
Great article, Claire! I really enjoyed reading about the use of ChatGPT in risk analysis for mathematical programming technology.
Thank you, David! I'm glad you found it interesting.
You're welcome, Claire. Keep up the great work!
Claire, have you considered any specific industries where ChatGPT could be applied effectively in risk analysis?
Great question, Emily! ChatGPT has potential applications in finance, insurance, supply chain management, and more.
I agree, David. This article highlights exciting progress in the field.
I found this article to be very informative. It's fascinating to see how AI technologies like ChatGPT are being applied in various domains.
As a mathematician, this is an exciting development. The potential applications of ChatGPT in risk analysis are vast.
Definitely, Michael. The advancements in AI continue to impress.
I have some concerns about using AI in risk analysis. It's essential to ensure the models are accurate and reliable.
Great point, Emily. AI models should go through rigorous testing and validation before being applied in critical applications like risk analysis.
I agree with Emily and Daniel. Trust and reliability are crucial when it comes to using AI in such important tasks.
Absolutely, Jennifer. Trustworthiness is key in AI-based risk analysis systems.
Indeed, Daniel. We must carefully evaluate AI models in high-stakes applications.
Absolutely, Daniel. We need reliable AI systems for effective risk management.
Indeed, Rachel. Trustworthy AI systems are crucial in risk analysis.
Interesting read! I wonder how ChatGPT compares to other AI models in risk analysis.
I think ChatGPT shows great promise. However, it's important to consider the limitations and biases that AI models can have.
That's a valid point, Sarah. Continuous monitoring and addressing biases are essential for responsible AI usage.
I agree, Emily. Ensuring model accuracy is of paramount importance.
I'm curious as well, Michael. Comparison studies would be insightful.
The progress in AI never fails to impress me. It's exciting to witness.
Monitoring for biases should be an ongoing effort in any AI-driven system.
Agreed, David. Bias monitoring should be a continuous process.
Indeed, ensuring the reliability of risk assessment is crucial.
We can't afford to overlook the potential risks associated with AI models.
Absolutely, Sarah. We must always be cautious when adopting new technologies.
Being cautious is essential, Robert. Thoughtful adoption leads to better outcomes.
A comparative study could shed light on the strengths of ChatGPT.
Exactly, Daniel. A thorough analysis would provide valuable insights.
Reliability must be paramount when using AI-driven risk analysis systems.
Absolutely, Emily. We need to be conscious of the challenges and limitations.
The potential for ChatGPT in a wide range of industries is exciting.
Trustworthiness is the foundation of successful risk analysis with AI.
Exactly, Jennifer. Trust in AI is vital for its integration in risk analysis.
Absolutely, Daniel. Informed decision-making is crucial in risk analysis.
Acknowledging limitations and challenges is crucial for responsible AI implementation.
Absolutely, Emily. We must ensure the reliability of AI-driven systems.
Continuously monitoring biases is vital to maintain fairness in AI.
Careful consideration during adoption is essential for successful implementation.
An in-depth analysis can help organizations make informed decisions.
Addressing biases is an ongoing process in AI systems.
Indeed, the possibilities for applying ChatGPT are immense.
Responsible implementation requires understanding and mitigating potential risks.
Precisely, Emily. Identifying and managing risks is essential in AI projects.
Reliability is critical in AI-driven systems for accurate risk assessment.
Thoughtful implementation can yield significant benefits in risk analysis.
Continuous monitoring and addressing biases ensure the fairness of AI.
Absolutely, Sarah. Bias mitigation is an ongoing responsibility.
Indeed, realizing the potential of ChatGPT requires careful exploration.