Utilizing ChatGPT for Benchmarking in Commodity Risk Management Technology: Enhancing Efficiency and Accuracy
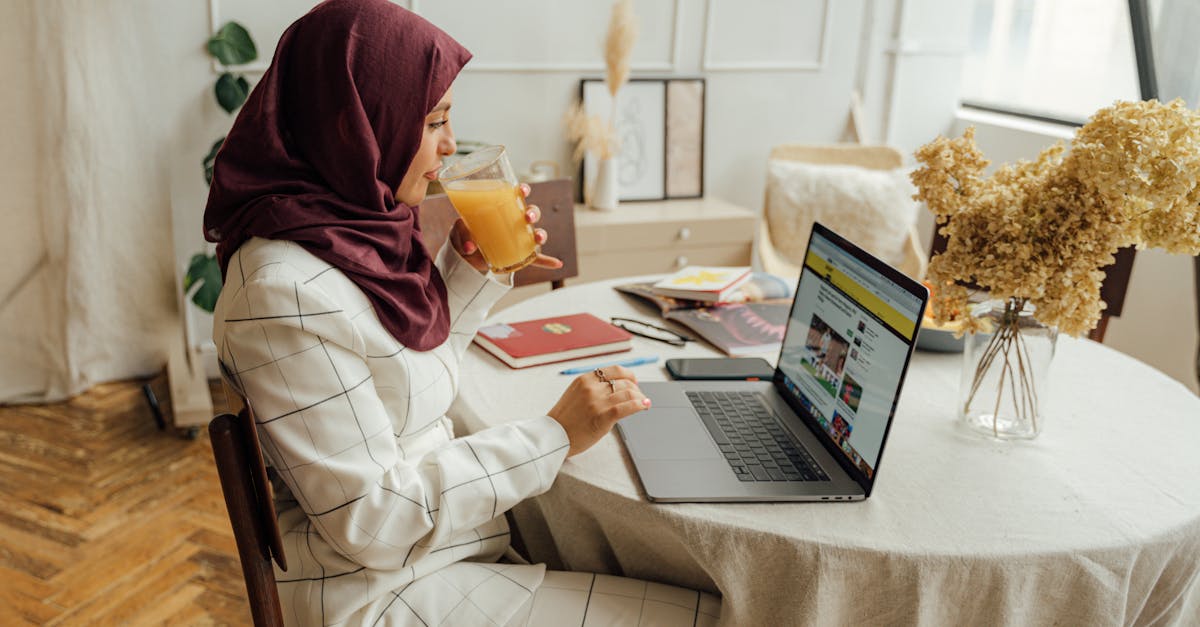
Commodity Risk Management is a crucial aspect of the financial industry, as it involves assessing and managing the risks associated with trading commodities such as oil, gas, metals, agricultural products, and more. One of the key tools used in this field is benchmarking, where commodity performances are compared against industry standards to evaluate their risk levels and ensure effective risk management.
The Role of AI in Benchmarking
With the advancements in technology, specifically Artificial Intelligence (AI), benchmarking commodity performances has become more efficient and accurate. AI algorithms can analyze vast amounts of data and identify patterns that humans may overlook. This enables better decision-making in commodity risk management, as benchmarking using AI can provide insights into a commodity's performance compared to industry standards.
Benefits of AI-Enabled Benchmarking in Commodity Risk Management
Utilizing AI in benchmarking commodity performances against industry standards offers several advantages in commodity risk management:
- Improved Accuracy: AI algorithms can analyze large datasets and identify intricate patterns, thereby providing more accurate benchmarking results.
- Efficient Risk Assessment: AI enables faster processing of data, enabling quicker risk assessments and timely decision-making.
- Identifying Outliers: AI algorithms can recognize outliers and deviations from industry standards, allowing risk managers to take appropriate actions to mitigate potential risks.
- Enhanced Decision-Making: By providing comprehensive insights into a commodity's performance, AI-enabled benchmarking empowers risk managers to make informed decisions, improving overall risk management strategies.
AI Techniques in Benchmarking Commodity Performances
Various AI techniques can be employed in benchmarking commodity performances. Some of the commonly used techniques include:
- Machine Learning: Machine learning algorithms can be trained on historical data to identify patterns and predict future performance, aiding in benchmarking commodities against industry standards.
- Natural Language Processing (NLP): NLP algorithms can process unstructured data, such as news articles and market reports, to extract valuable insights for benchmarking.
- Data Visualization: AI-powered data visualization tools can present benchmarking results in a visually appealing and easy-to-understand manner, facilitating better comprehension and analysis by risk managers.
Conclusion
AI has revolutionized benchmarking in commodity risk management by providing accurate and timely insights into commodity performances. By harnessing AI techniques like machine learning and NLP, risk managers can effectively benchmark commodities against industry standards, enabling proactive risk management strategies. This, in turn, helps financial institutions and companies navigate the complexities of commodity trading and mitigate risks effectively.
Comments:
Thank you all for joining this discussion! I'm excited to hear your thoughts on utilizing ChatGPT for benchmarking in commodity risk management technology.
I found the article quite informative. ChatGPT has definitely revolutionized the field of risk management technology by enhancing efficiency and accuracy. Kudos to the advancements in AI!
Thank you, John Anderson! The advancements in AI have indeed opened up new possibilities for improving risk management processes. Do you have any specific examples of how ChatGPT can be utilized in this context?
I have some concerns about using AI for such critical tasks. How can we ensure the model's reliability and prevent potential biases or errors?
I agree with Jane Smith. While AI can be powerful, it's crucial to have strict validation and testing processes in place to mitigate any risks associated with biases and errors.
Valid concerns, Jane Smith and Amy Richards! It's essential to thoroughly validate and test AI models to ensure reliability. Continuous monitoring and updating of the models can help in addressing biases and errors.
I wonder if ChatGPT can handle the complexity of commodity risk management. Has it been widely adopted in the industry?
That's a great question, Mark Thompson! ChatGPT's flexibility allows it to handle complex scenarios, making it a valuable tool in commodity risk management. While adoption is increasing, it's still relatively new in the industry.
I appreciate the potential benefits of utilizing ChatGPT in risk management. However, what are the challenges involved in implementing and integrating the technology?
Good question, Sarah Johnson! Implementing ChatGPT requires robust data infrastructure and training processes. Integration with existing risk management systems can also pose technical challenges, but the benefits outweigh the efforts in the long run.
The article mentions benchmarking. How does ChatGPT compare with traditional benchmarking methods in terms of accuracy and efficiency?
An excellent query, Robert Davis! ChatGPT offers the advantage of faster analysis and adaptation to changing market conditions compared to traditional benchmarking methods. Its accuracy depends on the quality of data used for training and continual fine-tuning.
I'm curious about the scalability of ChatGPT in commodity risk management. Can it handle a large volume of data and multiple risk scenarios simultaneously?
Great curiosity, Linda Adams! ChatGPT's scalability is a key strength. It can analyze and process large volumes of data efficiently while tackling multiple risk scenarios simultaneously, making it a valuable tool for commodity risk management.
Considering the sensitive nature of commodity markets, security becomes a top concern. How does ChatGPT ensure the protection of sensitive information?
Absolutely, Michael Brown! ChatGPT's deployment requires proper security protocols. Encryption, access controls, and adherence to data privacy regulations are crucial to ensure the protection of sensitive information in commodity risk management.
Has ChatGPT been tested in real-world scenarios? I'm interested in hearing about any successful implementations or use cases.
Indeed, Grace Lee! ChatGPT has been tested in various real-world scenarios, showing promising results. It has been utilized for risk analysis, decision support, and market trend predictions, among other applications, with successful outcomes.
It's worth mentioning that ChatGPT shouldn't replace human expertise in risk management. It should be viewed as a valuable tool that augments human decision-making.
Absolutely, John Anderson! ChatGPT is designed to assist and enhance human decision-making rather than replace it. Combining AI capabilities with domain expertise leads to better risk management outcomes.
Are there any limitations to ChatGPT's performance in commodity risk management? It would be interesting to understand its boundaries.
Great question, Emily Clark! ChatGPT's limitations include sensitivity to input phrasing, susceptibility to biased training data, and occasionally generating responses that may not align with human expectations. Continued research and development aim to address these limitations.
Considering the rapidly evolving nature of the commodity market, can ChatGPT adapt and learn from real-time market data?
Absolutely, Patrick Lee! ChatGPT can learn from real-time market data and adapt its analysis and decision-making accordingly. This ability to leverage up-to-date information is a significant advantage in the dynamic commodity market.
Does the adoption of ChatGPT require extensive training for risk management professionals to understand and use the technology effectively?
Excellent question, Karen Young! While familiarity with AI concepts would be beneficial, dedicated training programs and user-friendly interfaces can significantly reduce the learning curve, enabling risk management professionals to effectively utilize ChatGPT.
This article raises ethical concerns, particularly regarding the potential impact on employment in the risk management sector. How can we address this issue?
Ethical considerations are crucial, David White! Adoption of AI in risk management should be accompanied by reskilling efforts to equip professionals with new roles aligned with AI capabilities. This can ensure a smooth transition while maintaining employment opportunities.
I'm fascinated by the application of AI in risk management. Does ChatGPT have the potential to uncover new insights that might be missed through traditional analysis?
Absolutely, Sophia Green! ChatGPT's ability to analyze vast amounts of data and identify complex patterns can indeed uncover new insights that may not be apparent through traditional analysis methods. It has the potential to enhance risk management strategies with valuable discoveries.
Are there any regulatory frameworks or guidelines in place to govern the adoption and use of AI in commodity risk management?
Regulatory frameworks are being developed to govern AI adoption, Daniel Moore! Organizations should adhere to existing data protection and privacy regulations. Additionally, industry-specific guidelines and best practices are emerging to help guide responsible AI use in commodity risk management.
One concern often raised about AI is explainability. How can we overcome the challenge of interpreting ChatGPT's decision-making process in risk management contexts?
Explainability is an important aspect, Olivia Baker! Efforts are underway to develop methods that provide insights into AI decision-making. Techniques like interpretable models, attention mechanisms, and transparent AI frameworks can offer helpful explanations in risk management contexts.
What types of commodities can benefit the most from leveraging ChatGPT for risk management?
Great question, Jacob Turner! ChatGPT can benefit a wide range of commodities, from energy and agriculture to metals and financial instruments. Its versatility allows for customization based on specific commodity characteristics and risk factors.
Can ChatGPT be used to assist with decision-making related to market entry or exit in the commodity sector?
Absolutely, Natalie Wright! ChatGPT can assist in decision-making related to market entry or exit by analyzing various factors, market conditions, and risk indicators in real-time. It provides valuable insights to support strategic decisions in the commodity sector.
How do you foresee the future evolution of AI in commodity risk management? Any exciting possibilities?
The future holds exciting possibilities, Max Turner! We can expect AI to further enhance risk management capabilities, enabling more accurate forecasting, real-time monitoring, and adaptive decision-making. Continued research, innovation, and collaboration will drive these advancements.