Utilizing ChatGPT for Channel Capacity Evaluation in Signal Integrity Technology
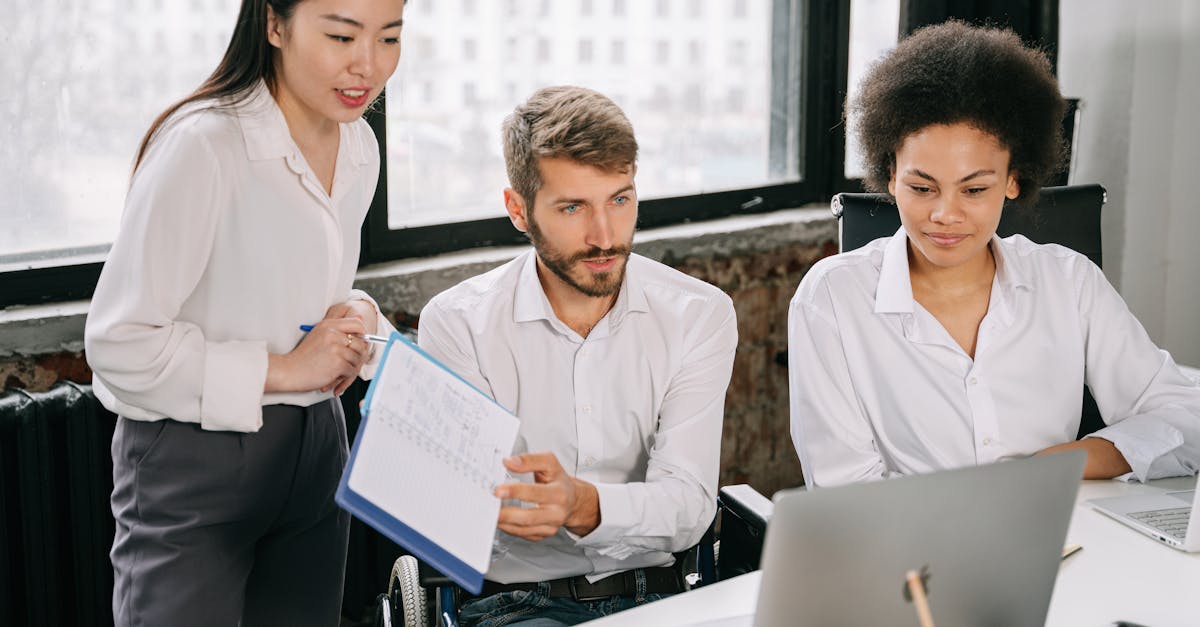
In the field of signal processing and communication systems, signal integrity refers to the ability of a signal to transmit efficiently and accurately through a given channel. It is crucial for ensuring good signal performance, especially in high-speed data transmission scenarios. One important aspect of evaluating signal integrity is the assessment of channel capacity, which measures the maximum amount of information that can be transmitted reliably over a given communication channel.
What is Channel Capacity?
Channel capacity is a fundamental concept in information theory that quantifies the maximum data rate, in bits per second, at which information can be reliably transmitted through a communication channel. It represents the upper limit of achievable data rate without errors or information loss. The measure of channel capacity takes into account various factors such as signal-to-noise ratio, bandwidth, and distortion effects.
Importance of Channel Capacity Evaluation
Evaluating channel capacity is crucial for designing and optimizing communication systems. By assessing the channel capacity, engineers can determine the maximum data rate that can be reliably transmitted over a specific channel. This information helps in selecting suitable modulation schemes, coding techniques, and other system parameters to achieve the desired performance. Channel capacity evaluation also aids in identifying potential limitations and bottlenecks in the system, allowing for improvements in signal integrity and overall system efficiency.
Signal Integrity and Channel Capacity Evaluation in Train ChatGPT-4
Train ChatGPT-4 is an advanced natural language processing model developed by OpenAI. It aims to provide highly accurate and contextually relevant responses in conversational AI applications. To ensure a robust and reliable communication system for Train ChatGPT-4, signal integrity and channel capacity evaluation play a vital role.
Channel Capacity Evaluation for Good Signal Performance
The evaluation of channel capacity in the context of Train ChatGPT-4 involves analyzing the efficiency of data transmission between the user and the model. By assessing the channel capacity, it becomes possible to identify potential communication bottlenecks and optimize the system for improved signal performance. This includes evaluating factors such as latency, noise, and data rate to enhance the overall user experience.
Optimizing Modulation Schemes and Coding Techniques
Channel capacity evaluation helps in selecting appropriate modulation schemes and coding techniques for Train ChatGPT-4. By considering the characteristics of the communication channel, engineers can choose the most suitable modulation schemes to maximize the data rate while maintaining reliable signal transmission. Similarly, optimizing coding techniques helps in minimizing errors and improving overall data integrity.
Identifying and Mitigating Distortion Effects
Distortion effects such as inter-symbol interference and noise can significantly impact signal integrity. Through channel capacity evaluation, it becomes possible to identify these distortion effects and develop mitigation strategies. By understanding the limitations imposed by a given communication channel, engineers can design techniques to reduce distortions and optimize the overall performance of Train ChatGPT-4.
Conclusion
Signal integrity and channel capacity evaluation are essential in ensuring good signal performance and reliable data transmission in communication systems. In the context of Train ChatGPT-4, these evaluations enable engineers to optimize the system for improved user experience by selecting appropriate modulation schemes, coding techniques, and mitigating distortion effects. By understanding and analyzing channel capacity, OpenAI can continue to develop advanced conversational AI models that offer reliable and efficient communication channels in various applications.
Comments:
Thank you all for participating in this discussion! I'm excited to hear your thoughts on utilizing ChatGPT for channel capacity evaluation in signal integrity technology.
I found the article quite fascinating. The idea of leveraging ChatGPT for channel capacity evaluation could revolutionize the field. It has the potential to provide more accurate and efficient analysis. Great work!
I agree, Lauren. By using ChatGPT, we can automate the evaluation process, saving valuable time and resources. It can also assist in conducting complex analyses that might be challenging without AI support.
While the idea is compelling, I am concerned about the reliability of AI-based evaluations. How do we ensure that the results are accurate and trustworthy?
Good point, Mark. The reliability of AI-based evaluations largely depends on the training data and the algorithm itself. Proper validation and testing protocols can help establish the accuracy of results.
I think incorporating ChatGPT in channel capacity evaluation is a step in the right direction. However, we should also conduct thorough comparative studies to assess its effectiveness compared to traditional methods.
Absolutely, Emily. Comparative studies can assess the strengths and limitations of AI-based evaluations and help identify scenarios where traditional methods might still be more suitable.
This article showcases the potential of AI in signal integrity technology. I appreciate the inclusion of practical examples that demonstrate the benefits of using ChatGPT in channel capacity evaluation.
Agreed, Oliver. The practical examples make it easier for us to envision the impact of ChatGPT in real-world signal integrity analysis. Exciting times!
I found the case study particularly interesting. It highlighted how ChatGPT can handle complex channel scenarios and provide useful insights. I believe this technology has great potential!
Incorporating AI for channel capacity evaluation could significantly enhance our ability to analyze and optimize signal integrity. However, there may be challenges in training the ChatGPT model to handle various signal types and scenarios.
Indeed, Benjamin. Extensive training with diverse signal types and scenarios is crucial to ensure that ChatGPT can provide reliable and accurate evaluations across different use cases.
I believe the challenges can be addressed by incorporating domain expertise during the training phase. By providing specific guidelines and validation checks, we can enhance the model's accuracy.
Another concern is the interpretability of AI-based evaluations. How can we ensure that the decision-making process of ChatGPT is transparent and understandable?
Great points, everyone! Reliability, interpretability, and comparative studies are all important considerations. I appreciate your insights and concerns regarding the use of ChatGPT in channel capacity evaluation.
One way to address interpretability concerns is by adopting techniques to visualize and explain the decision-making process of the AI model. XAI (Explainable AI) methodologies can be employed for this purpose.
Thank you for suggesting XAI, Daniel. It indeed offers promising methods to unravel the black box of AI models, making the evaluations more transparent and understandable to experts and stakeholders.
Moreover, AI should be seen as a complementary tool rather than a complete replacement. Integrating AI-based evaluations with traditional methods can leverage the strengths of both approaches.
Absolutely, Michael. AI can bring valuable insights and efficiency, but we should always consider it as a tool to support our expertise rather than replacing our expertise entirely.
Including XAI techniques in the evaluation process can enhance trustworthiness and acceptance among the users who heavily rely on the reports generated by ChatGPT.
I believe involving the end-users and stakeholders in the evaluation and decision-making process can help address their concerns and increase their confidence in AI-based evaluations.
I'm curious about the potential limitations of ChatGPT in channel capacity evaluation. What challenges may arise when applying this approach?
One challenge relates to the complexity of certain channel scenarios and the generalization capabilities of ChatGPT. Extensive testing and refinement are necessary to ensure accurate evaluations.
Are there any ethical considerations when implementing ChatGPT for channel capacity evaluation? I'm interested in hearing your opinions on this matter.
Ethical considerations are indeed important, Mark. Use of appropriate and unbiased training data, transparency, and addressing potential biases are crucial to ensure ethical AI implementation.
Involving stakeholders throughout the evaluation process can also help identify any hidden biases or concerns that may arise due to the use of ChatGPT in signal integrity analysis.
Absolutely, Rachel. Continuous monitoring and improvement of the AI model's performance should be prioritized to ensure fairness and accuracy in the evaluations.
Absolutely, Rachel. Continuous monitoring and improvement of the AI model's performance should be prioritized to ensure fairness and accuracy in the evaluations.
Indeed, Olivia. ChatGPT's potential goes beyond channel capacity evaluation. Exploring its applications in other areas of signal integrity analysis can lead to exciting breakthroughs!
Indeed, Olivia. Exploring the applicability of ChatGPT in design optimization, simulation, and even fault diagnosis can significantly enhance signal integrity analysis capabilities.
Fully agreed, Benjamin. ChatGPT can assist in automating various design and analysis processes, empowering engineers and researchers in the field of signal integrity.
Collaboration between AI experts, signal integrity professionals, and policymakers can help establish guidelines to address the ethical considerations associated with ChatGPT implementation.
I couldn't agree more, Michael. A multidisciplinary approach ensures that ethical concerns are adequately considered and that the implementation benefits all stakeholders.
Did anyone else notice the discussion on potential applications of ChatGPT for signal integrity analysis beyond channel capacity evaluation? It opens up exciting possibilities!
Another challenge can be the availability of diverse and comprehensive training datasets. Access to such datasets is crucial to ensure reliable evaluations across different signal types.
Absolutely! I can imagine ChatGPT assisting in tasks like impedance matching, noise analysis, and designing optimal transmission lines. The possibilities are vast!
When dealing with high-frequency signals, accuracy and computational efficiency are paramount. Are there any benchmarks highlighting the performance of ChatGPT in such scenarios?
Valid point, Daniel. Providing benchmarking results demonstrating ChatGPT's performance in high-frequency scenarios would strengthen confidence in its applicability to signal integrity technology.
I appreciate the discussion on both the potential and challenges of implementing ChatGPT. It's essential to consider the practical applicability and limitations of AI-based evaluations.
Agreed, Mark. Real-world signal integrity challenges can differ significantly, and ensuring ChatGPT's performance across a range of scenarios will be key to its successful implementation.
Another aspect to consider is the potential impact of incorrect evaluations. We should emphasize proper validation and testing protocols to minimize the chances of misleading results.
Absolutely, Sophia. The awareness of potential errors and uncertainties should be paramount in the development and adoption of AI-based evaluation tools like ChatGPT.
Collaboration between AI experts and signal integrity practitioners can help develop robust validation methodologies, ensuring accurate and reliable evaluations with minimal error rates.
I think incorporating domain expertise during ChatGPT's training can lead to more nuanced and accurate evaluations, catering specifically to signal integrity needs.
Absolutely, Sophia. The involvement of domain experts can enhance the AI model's understanding of signal integrity nuances, enabling it to deliver more precise evaluations.
I have seen similar approaches in other domains where AI models are trained with expert knowledge. It can indeed improve the accuracy and reliability of the evaluations.
Integrating AI with our existing knowledge and expertise can lead to hybrid approaches that boost the efficiency and accuracy of signal integrity analyses.
I want to thank each one of you for your valuable insights and engaging in this discussion. It's been an enriching conversation, highlighting both the opportunities and challenges of employing ChatGPT in signal integrity technology. Your contributions will undoubtedly influence future advancements in the field.
Additionally, as high-frequency signals often involve RF characteristics, we must ensure that ChatGPT can handle the unique requirements and complexities associated with RF analysis.
With that, let's conclude this discussion. Your participation and thoughtful comments have been instrumental in advancing our understanding of utilizing ChatGPT in channel capacity evaluation. Thank you all once again!