Utilizing ChatGPT for Enhanced Predictive Modelling in Translational Medicine Technology
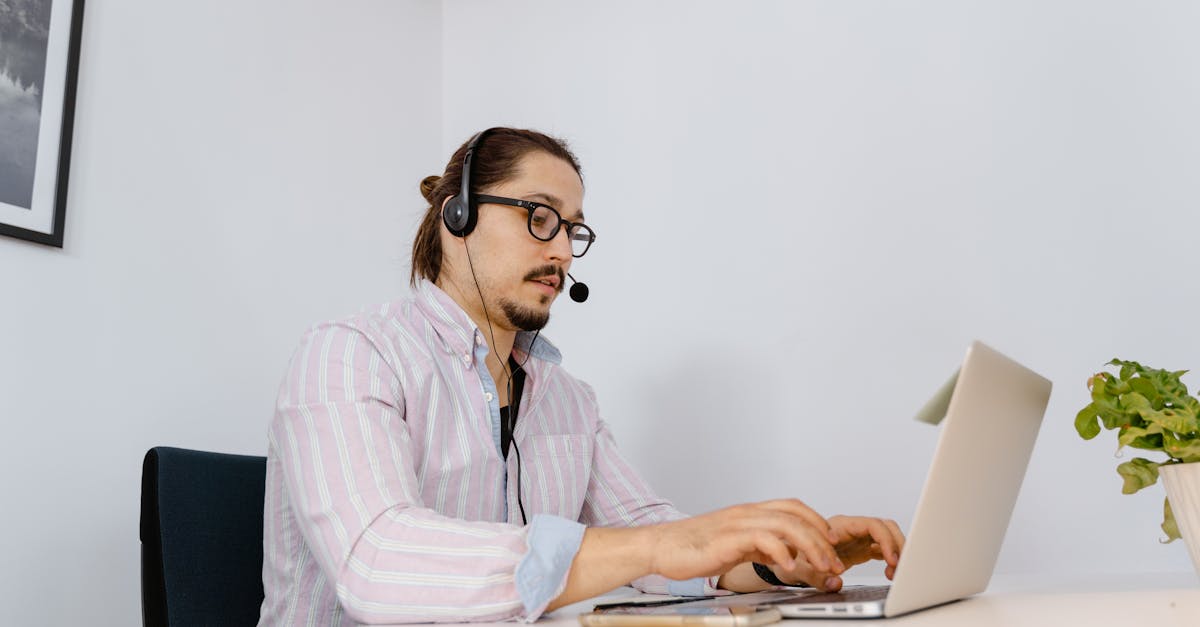
Translational medicine is a field that seeks to bridge the gap between scientific research and clinical practice. It involves the application of scientific findings to improve patient care and outcomes. One area of translational medicine that has gained significant attention is predictive modelling, which can help in developing models for disease progression and dynamic patient status.
What is Predictive Modelling?
Predictive modelling is a process that involves the use of statistical and computational techniques to create models that can forecast future outcomes. In the context of translational medicine, predictive modelling helps in predicting disease progression and patient status based on various factors such as genetic markers, lifestyle choices, and environmental factors.
Applications in Disease Progression
Predictive modelling offers immense potential in understanding disease progression. By analyzing large datasets and identifying patterns, researchers can develop models that can predict the likelihood of disease progression in individuals. This information can be utilized to personalize treatment plans and interventions for patients, thereby improving the effectiveness of treatment and reducing complications.
For example, in the field of oncology, predictive modelling can help in determining the likelihood of tumor growth, metastasis, and response to specific treatments. This information can guide physicians in making informed decisions regarding treatment options and timelines.
Dynamic Patient Status
In addition to disease progression, predictive modelling can also assist in understanding the dynamic nature of a patient's status. Healthcare professionals often need to monitor patients closely and make real-time decisions based on changing circumstances. Predictive models can help in assessing the likelihood of adverse events or complications, which can inform clinical decision-making and improve patient outcomes.
Advantages and Challenges
Predictive modelling in translational medicine offers several advantages. It can help save time and resources by focusing interventions on individuals who are at the greatest risk of disease progression. It can also aid in the development of personalized treatment plans, improving patient satisfaction and compliance.
However, there are challenges associated with predictive modelling. Developing accurate models requires access to large, high-quality datasets and robust computational resources. Additionally, ethical considerations such as patient privacy and data security need to be addressed when handling sensitive healthcare information.
Conclusion
Predictive modelling holds tremendous potential in advancing translational medicine. By utilizing predictive models, researchers and healthcare professionals can gain valuable insights into disease progression and dynamic patient status. This knowledge can lead to improved patient outcomes, personalized treatment plans, and more efficient allocation of resources. However, it is essential to address the challenges associated with predictive modelling to ensure its successful implementation in clinical practice.
Comments:
Thank you all for your comments and feedback on my article! I appreciate your insights.
I found the article very informative. It's fascinating to see how ChatGPT can be utilized in predictive modelling for translational medicine technology.
I agree, Emily. The potential applications of ChatGPT in this field are promising. It could potentially improve efficiency and accuracy in drug development.
David, indeed. Faster and more accurate prediction models can speed up drug discovery, which is crucial for addressing urgent medical needs.
As a medical researcher, I have concerns about the reliability of using AI models like ChatGPT for predictive modelling in such a critical field.
Sarah, that's a valid concern. While AI models can offer great support, they shouldn't replace human expertise. They should rather be used as a tool to complement human decision-making process.
Michael, I agree. AI should be a tool that supports medical professionals and fosters collaborative decision-making.
I think one area where ChatGPT could be especially helpful is in analyzing large volumes of medical data. It could potentially uncover patterns and insights that might be missed by humans.
But Andrew, AI models like ChatGPT can also introduce errors and false positives. We must be cautious before fully relying on them.
Sarah, while that's true, AI models are continuously improving. They have the potential to alleviate the burden on medical professionals and accelerate advancements.
I'm curious to know if there are any inherent biases in the training data that ChatGPT relies on. Bias can have serious consequences in healthcare.
Claire, you raise an important point. Bias in training data can lead to biased predictions. It's essential to ensure diverse and representative data is utilized for training AI models in healthcare.
Absolutely, Michael. Creating guidelines for data collection and ensuring representation can help minimize bias in healthcare AI.
Agreed, Claire. Transparency in the training data and model development process is crucial to detect and address biases effectively.
Michael, I'm glad you're highlighting the importance of transparency. Accountability and trust in AI-assisted healthcare are key.
Claire, overcoming biases in AI models will be a continuous effort. Regular audits and validation can help identify and rectify any biases that may arise.
Claire, accountability and rigorous testing are critical. The use of AI models should always be guided and supervised by experts in the field.
Michael, absolutely. No AI model should operate in isolation; it should be integrated into an ecosystem where human experts are involved.
Michael, how can we ensure that AI models like ChatGPT are continuously updated with new medical research and findings?
Sophia, regular model updates and integrating new research into training data can help AI models stay up-to-date and relevant.
Claire, continuous monitoring and updates are vital to address biases and ensure AI models reflect the most current medical knowledge.
Sarah, collaborative decision-making is crucial in the context of AI-powered healthcare. It combines the expertise of both humans and machines.
Sarah, precisely. Staying up-to-date with evolving medical knowledge ensures that AI models are providing the most accurate and relevant insights.
Claire, maintaining human involvement is key to ensuring ethical and responsible use of AI models in healthcare.
David, absolutely. The expertise of medical professionals can help interpret and validate the insights provided by AI models.
Exactly, Claire. Ethical and regulatory frameworks need to evolve alongside advancements in AI to ensure patient well-being and safety.
Sarah, AI models are meant to augment decision-making, not replace it. Medical professionals play a crucial role in the final treatment decision.
Emily, collaboration between experts from different fields is essential to drive progress in AI-assisted healthcare.
Claire, absolutely. AI models should be considered as trusted partners that collaborate with medical professionals.
Claire, clinical guidelines and regulatory bodies must also adapt to the challenges and opportunities presented by AI in healthcare.
Claire, an interdisciplinary approach that combines AI and medical expertise is crucial for the successful implementation of such models.
Oliver, interdisciplinary collaboration ensures AI models are developed and used responsibly and address the actual needs of healthcare.
David, you're right. Collaborative efforts help implement AI models effectively, targeted towards solving real-world healthcare challenges.
Claire, I agree. Collaborations between AI experts and medical professionals can help bridge the gap between technology and healthcare.
Sophia, AI models should be designed with flexibility in mind so that they can adapt to the evolving research and medical landscape.
Michael, continuous learning for AI models is crucial, especially in a field as rapidly evolving as medicine.
Sophia, staying informed about the latest research and updating AI models accordingly can lead to more accurate predictions.
Emily, collaboration can bridge the gap between innovative technologies like AI and the practical aspects of medicine.
Oliver, interdisciplinary research and collaboration will drive innovations that truly benefit patients and healthcare providers alike.
Emily, the collaboration between AI models and medical professionals can lead to the best outcomes for patients.
Sophia, continuous learning and adaptation are indeed key factors to ensure AI models stay relevant and aligned with the latest medical advancements.
Michael, ensuring AI models adapt to new research ensures they continue to provide relevant and accurate information for future medical discoveries.
Michael, AI models should be designed to incorporate new discoveries and evidence-based medicine as they emerge.
Sophia, continuous improvement and adaptation are crucial for AI models to keep up with the advancements in medicine.
I believe ChatGPT could be a game-changer in personalized medicine. It has the potential to analyze a patient's medical history, genetic data, and other factors to tailor treatments specifically to them.
I think it's still too early to fully trust AI models like ChatGPT with critical decisions in the field of medicine. More research and validation are required.
Hannah, I understand your concern, but with proper validation and fine-tuning, AI models can become valuable tools in enhancing patient care.
Daniel, personalized medicine is indeed an exciting prospect. Utilizing AI models like ChatGPT can contribute to more targeted and efficient treatments.
Oliver, imagine if customized treatment plans could be created for patients based on their unique biological characteristics.
Daniel, AI models could potentially lead to more efficient and targeted clinical trials by identifying patient subgroups that respond better to treatments.
Oliver, identifying patient subgroups for clinical trials more accurately could indeed improve the success rates of treatments.
David, precisely. AI models can help optimize resource allocation by identifying patient populations that are most likely to benefit from treatments.
Exactly, Emily. Collaborative decision-making between AI and medical professionals can yield the best outcomes for patients.
Emily, AI models may eventually reduce healthcare costs too, as more accurate predictions can result in optimized resource allocation.
Andrew, cost reduction is one of the potential benefits of AI models like ChatGPT in healthcare. It's an important aspect to consider.
Absolutely, Sarah. AI models should be considered as tools to support decision-making and not as replacements for medical professionals.
Emily, I couldn't agree more. The human element in medicine is irreplaceable.
Daniel, personalized treatments could provide better outcomes, but how do we maintain patient privacy and security with AI models?
Hannah, patient privacy is a crucial concern. AI models need to adhere to strict data privacy regulations to ensure patient confidentiality.
Oliver, strict data governance and anonymization protocols are necessary for protecting patient privacy when using AI models.
Hannah, you're absolutely right. Data privacy is of utmost importance, and we need robust measures to safeguard patient information.
Hannah, you're right. We should proceed with caution and use AI models as aids rather than sole decision-makers in medicine.
I think a balanced approach is needed. AI models can assist researchers and doctors in making better decisions, but they shouldn't solely drive the decision-making process.
David, I completely agree. AI models should augment medical expertise and not replace it.
Imagine if ChatGPT could assist in diagnosing rare diseases more accurately by analyzing symptoms and medical history.
Absolutely, Emily! AI models have the potential to improve disease identification and provide valuable decision support in challenging cases.
Michael, it would be interesting to explore how ChatGPT can assist in identifying potential drug targets for various diseases.
Exactly, Emily. It's vital to acknowledge the limitations and potential risks associated with AI models in medicine.
Sarah, while AI models can introduce errors, they can also reduce human errors that may occur due to fatigue or oversight.
I think it's essential for medical professionals to stay up-to-date with AI advancements and to be involved in the development and evaluation of such models.
I enjoyed reading the article. It's exciting to see how AI can impact translational medicine.
Sophia, AI's potential in medicine is vast, and it's still being explored. ChatGPT is just one of the many AI models making a difference.
AI models should augment medical professionals' expertise and provide them with additional insights that can guide decision-making.
Andrew, AI models can help medical professionals leverage their domain knowledge effectively, ultimately leading to better patient care.
Andrew, indeed. Cost reduction can have a significant impact on healthcare accessibility, especially in underprivileged areas.
Sarah, the ethical considerations around AI models in medicine need to be addressed thoroughly before widespread implementation.
Andrew, AI-assisted predictions can also optimize resource allocation by identifying patients who may require more intensive interventions.
Sarah, absolutely. Optimal resource allocation is vital for providing efficient and effective healthcare.
Emily, humans and machines working together can achieve the best results. Collaboration and partnership are key.
Sarah, I couldn't agree more. It's by combining our strengths that we can truly unlock the potential of AI-assisted healthcare.
Emily, precisely. Combining human expertise and AI capabilities allows us to provide the most effective and compassionate healthcare.
Sarah, cost savings through AI can be redirected towards improving healthcare infrastructure and overall patient care.
Claire, medical professionals can provide valuable context and interpretation to AI models, which is critical in making informed decisions.
Oliver, collaborative efforts can help overcome any biases or limitations that AI models might face on their own.
Oliver, human judgment and contextual insight can mitigate the risks and challenges associated with AI model predictions.
Claire, cost savings through AI can be reinvested into improving healthcare accessibility, quality, and affordability.
Sarah, the regulatory landscape needs to adapt to the advancements in AI while keeping patient safety and privacy as top priorities.
Oliver, collaborative efforts ensure that AI models are responsible, practical, and aligned with the needs of healthcare providers and patients.
Emily, the combination of empathy and technological advancements can revolutionize the way we approach healthcare.
Emily, optimizing resource allocation is crucial for healthcare systems to operate efficiently and provide quality care to all patients.
Sarah, empathy and compassion are at the core of healthcare. AI models should assist us in delivering the highest quality care.
Emily, interdisciplinary collaboration is key to understanding the diverse needs and challenges of healthcare and translating them into effective AI solutions.
Emily, AI models should be developed and deployed with a patient-centric approach, always keeping their well-being and best interests in mind.
Sarah, regulatory frameworks should strike a careful balance to encourage innovation while maintaining ethical and responsible AI use.
David, you're absolutely right. Regulatory frameworks must encourage innovation while safeguarding patient rights and well-being.
Sarah, optimizing resource allocation through AI can help healthcare systems be more efficient and sustainable in the long run.
Emily, AI-powered resource optimization can also help address healthcare disparities and ensure equitable access to quality care.
Sarah, optimization of resource allocation is crucial for healthcare systems to meet the demands of growing populations efficiently.
Claire, interdisciplinary collaboration fosters innovation and helps AI models serve the specific needs of healthcare.
Sophia, embracing dynamic learning and incorporating new research findings are vital for maintaining the relevance and accuracy of AI models.
Michael, research collaborations between academia, industry, and healthcare professionals are crucial for advancing the field of AI in medicine.
Sophia, I fully agree. Collaborations help pool expertise and resources, leading to more robust AI models and their effective application in medicine.
Michael, collaboration is essential to address the complex challenges surrounding AI in medicine and develop solutions that benefit patients.
Michael, collaborative efforts also ensure that AI models are tailored to meet the specific needs and challenges of translational medicine.
Sophia, collaboration among various stakeholders in the healthcare domain is crucial for fostering responsible and effective AI adoption.
Michael, collaboration helps align AI models with the needs of translational medicine and enables the translation of research into practical healthcare applications.
Sophia, collaboration can enhance the translation of innovative AI solutions from research to real-world impact in translational medicine.
Michael, interdisciplinary collaboration across scientific, medical, and technical domains can lead to groundbreaking innovations in translational medicine and AI.
Sophia, interdisciplinary collaboration brings together diverse expertise and viewpoints, driving innovation and fostering the effective use of AI in medicine.
Michael, interdisciplinary collaborations play a vital role in leveraging AI's capabilities to develop solutions that address complex medical challenges.
David, regular validation, audits, and bias analysis should be integrated into the lifecycle of AI models to ensure their continued accuracy and fairness.
Sarah, continuous validation and auditing of AI models are essential to ensure their alignment with ethical guidelines and the highest standards of patient care.
Sarah, regular validation and audits allow for the identification and mitigation of biases in AI models, ensuring equitable and fair outcomes for all.
Oliver, interdisciplinary collaboration facilitates the development and implementation of AI models that address healthcare challenges effectively.
David, interdisciplinary collaboration helps bridge the gap between AI research and healthcare expertise, enabling the development of targeted and impactful models.
Oliver, interdisciplinary collaboration fosters a comprehensive understanding of healthcare needs while ensuring AI models are developed and deployed responsibly.
Sarah, continuous validation and auditing ensure that AI models remain accountable, reliable, and unbiased in their decision-making processes.
Michael and David, interdisciplinary collaborations have the potential to reshape the landscape of translational medicine and unlock new possibilities in AI.
Sophia, interdisciplinary collaboration is crucial for AI applications to address the challenges specific to the healthcare domain.
Emily, indeed. We have the opportunity to harness the power of AI while preserving the values and empathy associated with healthcare.
Andrew, reducing healthcare costs through AI can lead to higher affordability and improved access to quality care for patients.
Emily, AI models can help ensure that healthcare resources are directed to where they are most needed, ultimately benefiting patients.
Emily, optimization of healthcare costs through AI can make quality care more accessible to a broader range of individuals.
Sarah, resource optimization is crucial, especially when healthcare resources are limited and need to be deployed efficiently.
Claire, optimizing resource allocation using AI can help reduce waiting times and enhance the overall patient experience.
Emily, optimization of resource allocation can streamline healthcare delivery, ensuring patients receive the right care at the right time.
Andrew, optimized resource allocation supported by AI models can lead to improved efficiency and patient satisfaction in healthcare.
Sarah, AI models have the potential to bridge resource gaps, ensuring equitable and fair access to healthcare for all.
Emily, AI can assist in managing limited resources more effectively, leading to better patient outcomes and treatment experiences.
Emily, AI-powered resource optimization can help reduce healthcare disparities and provide equitable access to quality care.
Sarah, optimized resource allocation is a critical aspect of healthcare management, and AI models can assist in achieving it.
Emily, optimizing resource usage through AI can help healthcare systems be more resilient and responsive to patient needs.
Claire, optimizing resource allocation can lead to cost savings, which can then be reinvested in expanding and improving healthcare services.
Claire, redirected cost savings can significantly improve the overall quality, accessibility, and affordability of healthcare services.
Sarah, AI models can help guide the allocation of resources, ensuring that limited resources are utilized efficiently and fairly.
David, a well-balanced regulatory environment is crucial to foster innovation in AI while prioritizing patient safety and ethical practices.
Sarah, patient-centric AI models place the patient's health and well-being at the forefront, improving overall quality of care.
Emily, optimizing resource allocation is essential, as it ensures patients receive timely access to the necessary healthcare services.
Andrew, optimizing resource allocation using AI models can maximize the impact and efficiency of healthcare services.
Sarah, regulatory bodies must walk the fine line of encouraging innovation while safeguarding patient welfare in the era of AI.
Sarah, the use of AI in healthcare has the potential to level the playing field and ensure equitable access to quality care.
Emily, patient-centric AI models foster a more holistic and personalized approach to healthcare, leading to better patient outcomes.
Sarah, resource optimization using AI models can potentially address the issue of healthcare resource scarcity more effectively.
Emily, resource optimization using AI can help healthcare systems respond better to emergencies and evolving patient needs.
Claire, AI can help healthcare systems become more resilient and adaptable, improving patient care even during challenging times.
Sarah, optimizing resource allocation can help healthcare systems deal with varying demands efficiently, shaping a more sustainable future.
Claire, the scalability and adaptability of AI models can help healthcare systems respond to dynamic and evolving healthcare needs.
Sarah, AI models that can flexibly adapt to changing healthcare requirements can ensure the best possible outcomes for patients.
Claire, regulatory frameworks that encourage responsible innovation can foster the development and adoption of AI models in healthcare.
David, striking the right balance between regulation and innovation is crucial in realizing the potential of AI in healthcare.
Oliver, a forward-thinking regulatory environment can foster innovation and ensure the ethical implementation of AI models in healthcare.
David, a forward-looking regulatory environment is crucial to support the responsible and safe use of AI models in healthcare.
David, regulatory frameworks should be designed to ensure the ethical and equitable use of AI models, benefiting both patients and providers.
David, regulatory frameworks should foster an environment that promotes responsible AI use and ensures equitable access to its benefits.
David, regulatory frameworks should address potential risks, protect patient rights, and continually evolve to keep pace with advancements in AI.
Oliver, a sound regulatory landscape can provide the necessary support and guidance for innovation and responsible AI use in healthcare.
Emily, regulatory frameworks can also help encourage interoperability and data sharing between different systems, facilitating better healthcare coordination.
Emily, ethical guidelines and legal frameworks can foster the development of AI models that prioritize patient welfare and ensure responsible use.
Claire, ethical guidelines play a crucial role in ensuring the development, deployment, and use of AI models that align with societal values and expectations.
Claire, resource optimization facilitated by AI models can significantly contribute to the overall efficiency and sustainability of healthcare systems.
Sarah, curated resource allocation powered by AI models can help healthcare systems use available resources efficiently to improve patient outcomes.
Emily, clear ethical guidelines and regulatory frameworks can encourage responsible AI usage and ensure alignment with societal expectations.
Claire, ethical guidelines can support AI implementation in healthcare, promoting trust and the responsible use of AI models in patient care.
Claire, ethical guidelines should provide a framework for AI models that promotes accountable, transparent, and unbiased decision-making in healthcare.
Emily, adherence to ethical guidelines can foster public trust and confidence in AI models, promoting their acceptance and adoption across healthcare.
Claire, ethical frameworks can guide AI model development, training, validation, and deployment, ensuring responsible and accountable AI usage in healthcare.
Emily, ethical guidelines can help guide the development of AI models in healthcare that prioritize fairness, accountability, and patient-centric care.
Claire, ethical guidelines can create a framework that helps build trust and establishes AI models as reliable and beneficial tools in healthcare.
Claire, ethical guidelines should emphasize transparency, accountability, and the responsibility of organizations to prioritize patient welfare when leveraging AI models.
David, regulatory frameworks must be versatile and adaptive, capable of managing the pace of technological advancements and addressing new challenges.
Hannah, regulatory frameworks should strike a balance to encourage innovation while protecting patient rights, privacy, and safety.
David, regulatory frameworks should encompass predefined standards, evaluation criteria, and periodic reviews to ensure the responsible use of AI models.
Oliver, regulatory frameworks should provide clear guidelines that address not only the development and use of AI models but also transparent documentation of their limitations.
David, regulatory frameworks should address validation and certification processes to establish the credibility and quality of AI models in healthcare.
Oliver, involving regulatory bodies and standardization organizations in the development of AI guidelines can help ensure high standards and safety in healthcare.
David, ethical frameworks and guidelines must keep pace with the rapid advancements in AI technology to ensure responsible and safe use in healthcare.
Sarah, it is indeed vital to update ethical frameworks to stay aligned with the evolving AI landscape and protect the interests of patients.
David, continuous review and updating of ethical frameworks and guidelines are necessary to reflect societal values and protect patient welfare.
Sarah, the integration of AI models in healthcare should always prioritize patient safety, equity, and respect for individual autonomy.
David, incorporating ethical values into the development and implementation of AI models is essential for building a responsible and equitable future of healthcare.
Sarah, AI models should always be held accountable for providing explainable and unbiased outcomes and aligning with ethical standards.
David, AI technologies in healthcare must align with ethical principles and ensure transparency, accountability, and privacy protection for patients.
Sarah, embedding ethical principles within AI models is essential to ensure they support human values and uphold patient rights and welfare.
Sarah, ethical considerations should be at the forefront when developing and deploying AI models, ensuring fairness, inclusivity, and justice in healthcare.
Emily, ethical frameworks can guide AI in healthcare towards meaningful and responsible implementations that prioritize patient well-being.
Claire, ethical guidelines can ensure that AI models adhere to principles of transparency, explainability, and fairness in healthcare decision-making.
Emily, ethical guidelines help shape AI models that are responsible, unbiased, and transparent in supporting healthcare decision-making.
David, ethical guidelines should be dynamic, continuously evolving to address new challenges and avoid potential biases inherent in AI models.
Oliver, ethical guidelines should consider the potential impact of AI models on patients, healthcare professionals, and underprivileged communities.
David, ethical guidelines need to account for the potential biases that could be embedded in AI models and promote fair, unbiased outcomes for all patients.
Oliver, collaborations between researchers, practitioners, and industry professionals can accelerate the development and adoption of AI models in healthcare.
Michael, collaborative efforts help ensure AI models are relevant, effective, and ethically sound for their intended applications in translational medicine.
Oliver, collaborations lead to shared expertise, enabling the development of AI models that are both scientifically accurate and practically relevant.
Michael, collaboration ensures that AI models are developed and implemented keeping in mind real-world needs, limitations, and ethical considerations.
Michael, bringing together diverse expertise enables AI models to be developed and applied with a holistic understanding of healthcare challenges.
David, inclusivity and addressing biases from both the training data and the AI model itself are essential considerations when developing ethical guidelines.
David, bias detection, and mitigation techniques should be incorporated into the development and continuous evaluation of AI models in healthcare.
Claire, ethical frameworks should promote the responsible creation and use of AI models that align with the broader goals and values of the healthcare sector.
Claire, ongoing monitoring and auditing of AI models can help identify and address biases and ensure that they remain up to date and reliable.
Sarah, regular audits and validation of AI models are crucial to ensure they remain accurate, unbiased, and in line with the latest medical knowledge.
Emily, continuous monitoring helps us understand if AI models are evolving effectively and allows for regular updates to counteract any bias issues.
Sarah, regular validation, auditing, and bias mitigation strategies are crucial to ensure that AI models remain both reliable and unbiased.
David, AI models should undergo rigorous testing and validation to ensure fairness, transparency, and accurate predictions without undue biases.
Sarah, continuous monitoring and improvement of AI models can help identify and address potential biases to ensure equitable outcomes and patient well-being.
David, continuously improving or revising ethical frameworks is crucial to address the evolving AI landscape, foster inclusivity, and mitigate potential biases.
Oliver, regulatory frameworks need to encourage innovation while addressing concerns regarding accountability, transparency, and patient welfare.
Claire, striking a balance between ensuring patient safety and encouraging innovation is key for successful AI integration in health systems.
Emily, ethical considerations and clear guidelines can help regulatory frameworks adapt to the evolving landscape of AI in healthcare.
Emily, AI can be a powerful tool in reducing healthcare disparities by optimizing resource allocation and enhancing decision-making processes.
Sarah, absolutely. AI-assisted cost reduction can enable more accessible and affordable healthcare solutions for all.
Sarah, reducing costs can make healthcare more accessible to a larger population, ensuring better health outcomes overall.
Transparency in AI model development and encouraging collaboration between researchers and medical professionals can lead to better outcomes.
Collaboration is critical to ensuring AI models are used responsibly, safely, and ethically in healthcare.
Daniel, developing robust security measures and ensuring compliance with privacy regulations are essential when dealing with patient data.
Hannah, privacy and data protection should be paramount in the development and use of AI models in healthcare.
Daniel, privacy and security should never be compromised when using AI models, especially when it comes to sensitive patient data.
Daniel, when patient data is handled responsibly, AI models can bring valuable insights for improving health outcomes without compromising privacy.
Daniel, an ethical framework should guide the development and deployment of AI models to ensure patient privacy is protected at all times.
Hannah, ethical guidelines should govern the collection, storage, and use of patient data when employing AI models.
Oliver, privacy regulations and security measures should be prioritized to foster patient trust in AI applications.
Daniel, patient trust is the foundation of successful implementation of AI models in healthcare. Privacy and security should never be compromised.
Hannah, privacy regulations like HIPAA (Health Insurance Portability and Accountability Act) provide a framework to protect patient confidentiality.
Hannah, building trust in AI applications requires transparency about data handling and robust security measures.
Oliver, patient trust is crucial. Transparent communication about data usage and adherence to privacy regulations can help establish trust in AI models.
Hannah, trust and data privacy are essential considerations for patients to feel comfortable with AI-assisted healthcare approaches.
Daniel, patients need to feel that their information is being handled responsibly and that privacy safeguards are in place when AI models are involved.
Oliver, ethical considerations and robust safeguards are crucial to maintain patient trust in AI-powered healthcare solutions.
Hannah, patients should have control over how their data is utilized and ensure that AI models respect their privacy preferences.
Emily, AI-powered resource optimization can play a significant role in alleviating healthcare resource scarcity and improving outcomes.
Emily, active patient participation in the development and implementation of AI models can foster trust and enhance patient autonomy.
Hannah, patient involvement in decision-making around AI models is crucial for ensuring trust and safeguarding their rights.
Oliver, absolutely. Transparency and consent when it comes to patient data usage are key to establishing trust in AI applications.
Daniel, patients should always be informed about how their data will be used and have the right to provide or withdraw consent.
Daniel, transparency and clear communication regarding data usage can help patients understand the benefits and limitations of AI models in healthcare.
Oliver, patient awareness and understanding of AI models can lead to more informed decision-making about the use of their data.
Oliver, patients should have the right to access and manage their data within AI models, ensuring transparency and control.
Oliver, organizations utilizing AI models must take responsibility for ensuring data privacy, security, and ethical use.
Hannah, data privacy is a joint responsibility, and organizations must be accountable for ethical AI implementation in healthcare.
Daniel, providing patients with understandable explanations of how their data is used by AI models helps build trust and promote informed decision-making.
Daniel, patients should have control over their data, including the right to know how it's used and the ability to make informed choices about its utilization.
Oliver, adaptable AI models can ensure that healthcare systems effectively respond to emerging challenges and changing medical landscapes.
Sarah, respecting patient privacy and establishing patient-centric AI models will drive greater acceptance and adoption of AI applications in healthcare.
Sarah, AI-powered optimization can help healthcare systems prioritize resource allocation to provide high-quality care while managing costs effectively.
Sarah, resource optimization using AI models can lead to improvements in wait times, better healthcare access, and more efficient service delivery.
Daniel, patient empowerment through informed consent and data control enhances the ethical use of AI models in healthcare.
Daniel, patient-centered AI models foster trust and respect patient autonomy by allowing individuals to actively participate in their healthcare decisions.
Hannah, active involvement of patients in the decision-making process surrounding AI models can minimize the risk of bias and enhance transparency.
Daniel, patient participation in AI model development helps ensure that healthcare solutions are designed to meet their unique needs effectively.
Oliver, establishing robust data protection measures and providing transparent information to patients is essential for fostering trust in AI models.
Hannah, patient engagement in AI development ensures that models align with patient values, preferences, and privacy concerns.
Emily, AI can play a crucial role in augmenting healthcare providers' capabilities but should always respect patient rights and privacy.
Sarah, respecting patient privacy and data protection is crucial for AI models to have long-term trust and acceptance in healthcare.
Oliver, strong governance and compliance measures are necessary to ensure AI models are developed and utilized ethically.
Hannah, privacy and security measures should align with the highest standards to ensure patient data is handled responsibly.
ChatGPT, sometimes more influential than a group of doctors. When processing information from several dozen medical articles, the system was able to identify numerous connections hardly recognizable even to trained professionals. That is impressive.
You are absolutely right. It is practically impossible for one person to have information about all possible interactions for one component that may be used in another. In this case, AI can help.