Utilizing ChatGPT for Pollutant Analysis in Powder X-ray Diffraction: A Cutting-Edge Approach
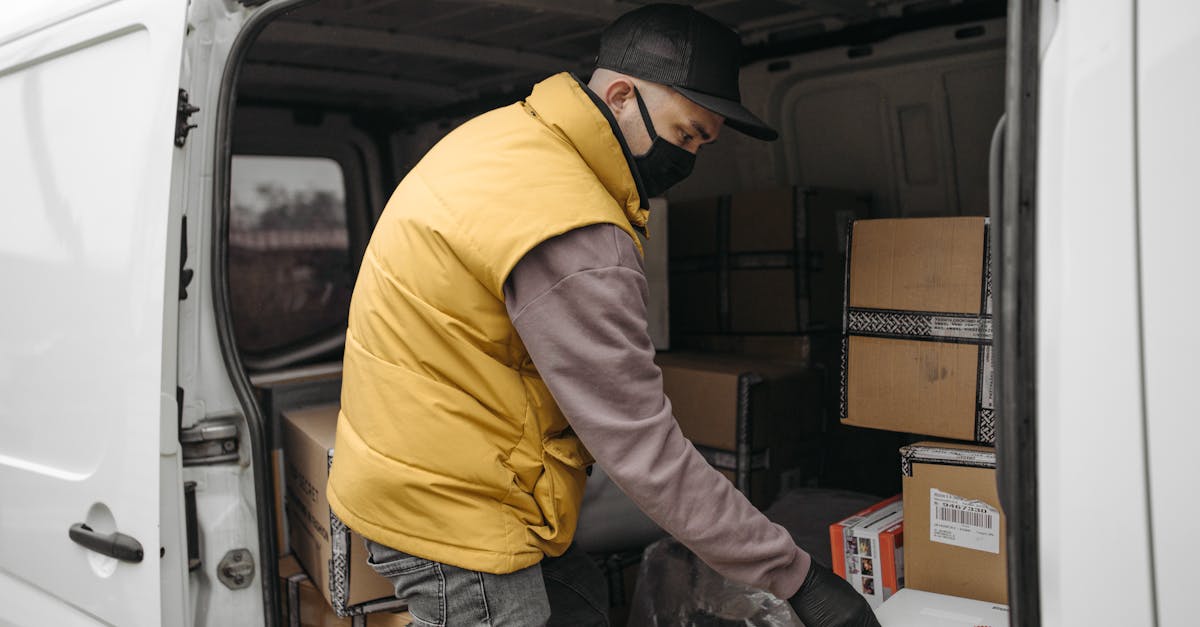
Powder X-ray Diffraction (PXRD) is a technique widely used in scientific research and environmental analysis. It utilizes the interaction of X-rays with crystalline materials to provide information about their atomic and molecular structure. In recent years, PXRD has found extensive application in pollutant analysis, where it plays a crucial role in identifying and measuring pollutants present in environmental samples.
The Principle of Powder X-ray Diffraction
PXRD relies on the scattering of X-rays by the atoms in a crystal lattice. When an X-ray beam interacts with a crystal, it is diffracted in specific directions, resulting in a characteristic diffraction pattern. This pattern provides valuable information about the crystal structure, including interatomic distances, lattice parameters, and orientation of crystal planes.
Pollutant Analysis using PXRD
The identification and quantification of pollutants in environmental samples, such as air, soil, and water, are crucial for assessing their impact on ecosystems and human health. PXRD can aid in this process by analyzing samples for specific pollutant compounds.
One of the key advantages of PXRD in pollutant analysis is its ability to provide a non-destructive, bulk analysis of a sample. This means that the sample can be analyzed as it is, without the need for time-consuming and expensive sample preparation techniques. The non-destructive nature of PXRD ensures that samples can be re-analyzed or preserved for further investigations.
Aiding PXRD with ChatGPT-4
In the field of Artificial Intelligence (AI), OpenAI's ChatGPT-4 model can be utilized to aid in the identification and measurement of pollutants using PXRD. ChatGPT-4 is a state-of-the-art language processing model that can generate text-based responses based on user inputs. By combining the knowledge of pollutant analysis and the capabilities of ChatGPT-4, researchers and analysts can interact with the model to obtain valuable insights in their pollutant analysis workflow.
With ChatGPT-4, researchers can ask questions related to PXRD techniques, specific pollutants, or even seek guidance in interpreting PXRD data. The model can provide helpful explanations, suggest data analysis methods, and assist in troubleshooting any issues encountered during the analysis process. This integration of AI and PXRD technology can streamline and enhance the efficiency of pollutant analysis.
Conclusion
Powder X-ray Diffraction has emerged as a powerful tool in pollutant analysis, enabling scientists and environmental analysts to identify and quantify pollutants in various environmental samples. The combination of PXRD with AI capabilities, such as ChatGPT-4, further enhances the utility of this technique by providing researchers with valuable insights and assistance in their analysis workflow.
As the field of AI continues to evolve, it is likely that a synergy with other scientific disciplines, like PXRD, will lead to significant advancements and breakthroughs in pollutant analysis and environmental research as a whole.
Comments:
Thank you all for taking the time to read my article on utilizing ChatGPT for pollutant analysis in powder X-ray diffraction. I appreciate your feedback and thoughts!
Great article, Stephen. The use of ChatGPT for pollutant analysis seems like a promising approach. Have you considered the possible limitations or challenges that may arise when applying this method in real-world scenarios?
Hi Lisa, thank you for your question. Indeed, there are some limitations when it comes to using ChatGPT for pollutant analysis in real-world scenarios. One challenge is the need for accurate training data to ensure the model's effectiveness in different sample types. Also, the model may require refining and fine-tuning to handle specific edge cases. Continuous improvement and adaptation are necessary to address these challenges.
This article is fascinating! I'm curious about the practicality of implementing ChatGPT for pollutant analysis. Are there any requirements in terms of hardware or computational resources?
Hi David, thanks for your interest. The practicality of implementing ChatGPT for pollutant analysis depends on the scale of the analysis and available computational resources. While the model itself can run on standard hardware, larger datasets and more complex analyses might require more powerful hardware or cloud computing resources to achieve optimal performance.
I really enjoyed reading this article, Stephen. I can see a lot of potential in using ChatGPT for pollutant analysis in powder X-ray diffraction. How do you envision this technology evolving in the future?
Thank you, Emily. It's great to hear that you see the potential in using ChatGPT for pollutant analysis. In the future, I believe this technology will continue to improve in terms of accuracy and flexibility. As more data becomes available and machine learning techniques advance, we can expect better performance and wider applicability of this approach. Additionally, the integration of domain-specific knowledge and expert feedback will enhance the model's capabilities.
Stephen, congratulations on the article! I'm curious about the training process for ChatGPT to perform pollutant analysis. Could you shed some light on the data requirements and labeling process?
Thank you, Robert. The training process for ChatGPT involves providing it with a large dataset that includes examples of pollutant analysis results along with associated annotations or labels. It requires data that covers a broad range of pollutant types and a variety of analysis scenarios to ensure its effectiveness. The labeling process typically involves domain experts marking the pollutants and providing additional information for training the model.
Interesting article, Stephen! How does ChatGPT handle situations where multiple pollutants are present in the sample, or when there are overlapping X-ray diffraction patterns?
Hi Anna, great questions. ChatGPT is designed to handle situations where multiple pollutants are present in the sample. By analyzing the X-ray diffraction patterns collectively, the model can identify different pollutants and their respective concentrations. However, when there are overlapping patterns, the model may face challenges in accurately discerning the individual patterns. This is an area where further research and refinement are necessary.
Thanks for sharing this article, Stephen. I can see the potential benefits of using ChatGPT for pollutant analysis in powder X-ray diffraction. Are there any specific advantages of this approach compared to existing methods?
Hi Mark, thanks for your comment. One of the advantages of using ChatGPT for pollutant analysis is its ability to handle complex natural language queries related to the analysis. It provides a user-friendly interface for researchers and analysts, allowing them to ask specific questions and receive detailed results. Additionally, the model can continuously learn and improve through feedback, enabling it to adapt to new challenges and emerging pollutants.
Impressive work, Stephen! Could you elaborate on the steps involved in training ChatGPT for pollutant analysis? How long does the training process usually take?
Thank you, Grace. The training process for ChatGPT involves several steps. First, a large dataset with pollutant analysis examples and associated annotations is collected. Next, the dataset is preprocessed, including cleaning and formatting the data. The model is then trained on this processed dataset using techniques like transfer learning. The training time can vary depending on factors such as the dataset size, hardware resources, and training configuration, but it typically takes several hours to a few days.
This is a fascinating application of ChatGPT, Stephen. I'm curious about the potential risks or limitations in relying on an AI model like this for pollutant analysis. Can you discuss any ethical considerations?
Hi Samantha, you raise an important point. When relying on an AI model like ChatGPT for pollutant analysis, ethical considerations are crucial. It's essential to ensure transparency and understand the model's limitations. The potential risks include biases in the training data or the model's decisions, which may lead to inaccurate or unfair analyses. Close collaboration with domain experts, validation of results, and careful monitoring are necessary to mitigate these risks and ensure responsible use of the technology.
Stephen, congratulations on this innovative approach to pollutant analysis! How transferable do you think the knowledge and expertise gained from ChatGPT can be to other scientific domains?
Thank you, Jennifer. The knowledge and expertise gained from ChatGPT can indeed be transferable to other scientific domains that involve analysis and interpretation. By adapting the training data and fine-tuning the model according to the specific domain requirements, ChatGPT can serve as a valuable tool for various scientific tasks. However, it's important to carefully consider the nuances and complexities of each domain to ensure accurate and meaningful results.
Interesting article, Stephen. Given the nature of pollutant analysis, how reliable is ChatGPT in terms of providing accurate results?
Hi Michael, thanks for your question. ChatGPT's reliability in providing accurate results for pollutant analysis depends on the quality and diversity of the training data, as well as the fine-tuning process. The model strives to provide accurate and reliable analyses, but it's important to remember that it has limitations. Close collaboration with domain experts and thorough validation of results are crucial to ensure the accuracy and reliability of the outputs.
Great article, Stephen! I'm curious about the potential applications beyond pollutant analysis. Can ChatGPT be adapted to analyze other types of materials or scientific data?
Hi Nathan, glad you found the article interesting. Yes, ChatGPT can be adapted to analyze other types of materials or scientific data. By providing appropriate training data and fine-tuning the model, it can be utilized in various scientific domains, such as material characterization or data analysis. The flexibility of ChatGPT makes it a versatile tool for a wide range of applications beyond pollutant analysis.
Stephen, I enjoyed reading your article on utilizing ChatGPT for pollutant analysis. Can you discuss the potential impact of this technology on the field of X-ray diffraction analysis?
Thank you, Daniel. The potential impact of utilizing ChatGPT for pollutant analysis in X-ray diffraction is significant. This technology can enhance the efficiency and accuracy of analyzing X-ray diffraction patterns to identify and quantify pollutants. By providing a user-friendly interface and leveraging the capabilities of machine learning, ChatGPT can streamline the analysis process, enable deeper insights, and contribute to advancements in the field of X-ray diffraction analysis.
Excellent article, Stephen! I'm wondering if ChatGPT can be integrated into existing software tools or platforms commonly used in the field of X-ray diffraction analysis?
Hi Rachel, thank you! Yes, ChatGPT can be integrated into existing software tools or platforms used in X-ray diffraction analysis. It can serve as an additional module or feature within such tools, providing users with a conversational interface to interact with and obtain pollutant analysis results. Integration with existing platforms can leverage their functionalities while benefiting from the capabilities of ChatGPT, resulting in an improved user experience in the analysis workflow.
Fascinating article, Stephen. In terms of accuracy, have you conducted any comparative studies or benchmarks to evaluate ChatGPT's performance against other existing pollutant analysis methods?
Hi Olivia, great question. Evaluating ChatGPT's performance against other existing pollutant analysis methods is an important aspect. While the specific comparative studies or benchmarks might vary depending on the context and available reference methods, rigorous evaluations and validations need to be conducted to assess the model's accuracy and reliability. These studies can involve comparing the results obtained from ChatGPT with established analysis methods, involving experts in the field, and considering real-world sample sets.
Stephen, your article on ChatGPT for pollutant analysis offers an innovative approach. Are there any plans to develop a user-friendly interface to make this technology more accessible to researchers and analysts?
Hi Oliver, thank you for your comment. Developing a user-friendly interface is indeed an important consideration to make this technology more accessible. While the article focuses on the utilization of ChatGPT for pollutant analysis, the development of an intuitive and interactive user interface to facilitate researchers and analysts' usage is an area of ongoing work. Such an interface will enhance usability, enable efficient interactions, and contribute to broader adoption of this technology.
I found your article on pollutant analysis using ChatGPT very enlightening, Stephen. Have you encountered any limitations or challenges in terms of training the model with diverse pollutant samples?
Thank you, Sophia. Training the model with diverse pollutant samples does present certain challenges. The availability of representative and diverse training data is crucial for the model's effectiveness. Obtaining and annotating diverse pollutant samples can be a time-consuming task, and acquiring such data may have associated costs. It's important to dedicate efforts to ensure an extensive and diverse training set to avoid potential biases or limitations during pollutant analysis.
Great article, Stephen. Given the dynamic nature of pollutant analysis and the evolving understanding of pollutant types, how can ChatGPT continually adapt and learn to keep up with emerging challenges?
Hi Lucas, you raise an important point. ChatGPT's adaptability and continuous learning depend on a feedback loop involving domain experts and users. Regular feedback, refinements, and updates to the model based on emerging challenges and new pollutant types are crucial. Training the model on new data and incorporating domain-specific knowledge allows it to continuously improve and adapt to the evolving understanding of pollutants in X-ray diffraction analysis.
Stephen, this article on utilizing ChatGPT for pollutant analysis in powder X-ray diffraction is quite intriguing. Can you discuss any limitations or considerations when applying this approach to real-world samples?
Hi Ryan, thanks for your interest. Applying the ChatGPT approach to real-world samples does come with certain limitations. While the model strives to provide accurate results, external factors like variations in sample composition, sample quality, or instrumental limitations can affect the analyses. It's essential to consider these factors and validate the results obtained from ChatGPT with other methods, expert knowledge, or additional experiments to ensure reliable pollutant analysis in real-world scenarios.
Great job, Stephen! I'm curious if there are any plans to make the ChatGPT model publicly available for researchers and analysts in the field of powder X-ray diffraction analysis.
Thank you, Lucy. Making the ChatGPT model publicly available is indeed a consideration. However, it requires further evaluation, refinement, and validation to ensure its robustness and reliability across different analysis scenarios. Efforts are underway to make the model accessible to the scientific community, taking into account the necessary precautions, intellectual property considerations, and data privacy concerns.
Stephen, your article on utilizing ChatGPT for pollutant analysis is fascinating. Are there any plans to apply this approach to other analytical techniques or methods?
Hi Samuel, thank you for your comment. While the article focuses on using ChatGPT for pollutant analysis in powder X-ray diffraction, there is potential for applying this approach to other analytical techniques or methods. The underlying principles of natural language processing and machine learning can be extended to other scientific domains to assist researchers and analysts with their specific analysis needs. Exploring broader applicability is an area of ongoing research and development.
Stephen, your article presents an innovative approach to pollutant analysis using ChatGPT. How accessible is the model for researchers who might want to explore its capabilities?
Hi Abigail, accessibility for researchers is an important aspect. While the model's accessibility depends on factors like its availability and deployment options, efforts are being made to explore user-friendly interfaces and create more accessible versions. This includes providing detailed documentation, code examples, and tutorials to assist researchers in understanding and utilizing the model effectively for their pollutant analysis requirements.
This article on utilizing ChatGPT for pollutant analysis opens up exciting possibilities, Stephen. In terms of data security, what measures are being taken to protect sensitive information during the analysis process?
Hi Hannah, data security is indeed crucial when utilizing ChatGPT for pollutant analysis. Measures are being taken to protect sensitive information during the analysis process. These include anonymization and encryption techniques to ensure privacy and confidentiality of the data. Furthermore, adherence to data protection regulations and best practices is a priority to mitigate potential risks and maintain the integrity of the analysis workflow.
Stephen, your article on using ChatGPT for pollutant analysis is intriguing. How does the model handle cases where the X-ray diffraction patterns are of low quality or exhibit noise?
Hi Ethan, thanks for your question. When dealing with low-quality X-ray diffraction patterns or noise, the model's performance may be affected. Noisy or low-quality patterns can introduce uncertainties, making the analysis challenging. Preprocessing steps, such as noise reduction or signal enhancement techniques, can help alleviate the impact of low-quality patterns. Additionally, incorporating domain expertise or adopting methods to manage noise can aid in improving the model's ability to handle such cases.
Stephen, I found your article on utilizing ChatGPT for pollutant analysis to be very insightful. How do you envision the integration of ChatGPT with laboratory instruments and automation systems?
Thank you, Chloe. The integration of ChatGPT with laboratory instruments and automation systems is an exciting prospect. The model can be integrated into software running on the instruments or automation systems, providing real-time analysis capabilities and interactive querying. Researchers and analysts can obtain pollutant analysis results without leaving their accustomed tools or workflow, enhancing the efficiency and productivity of laboratory operations.
This article on utilizing ChatGPT for pollutant analysis presents an intriguing approach. What are the key factors to consider when implementing this technology in a laboratory setting?
Hi Isabella, implementing ChatGPT for pollutant analysis in a laboratory setting involves considering several key factors. These include assessing the model's performance, compatibility with existing laboratory infrastructure, training data availability, integration with analysis workflows, and validation of results against established methods. Additionally, addressing data security, scalability, and ethical considerations are vital aspects when deploying the technology in a laboratory environment.