Utilizing ChatGPT for Streamlining Resume Screening in Neural Networks Technology
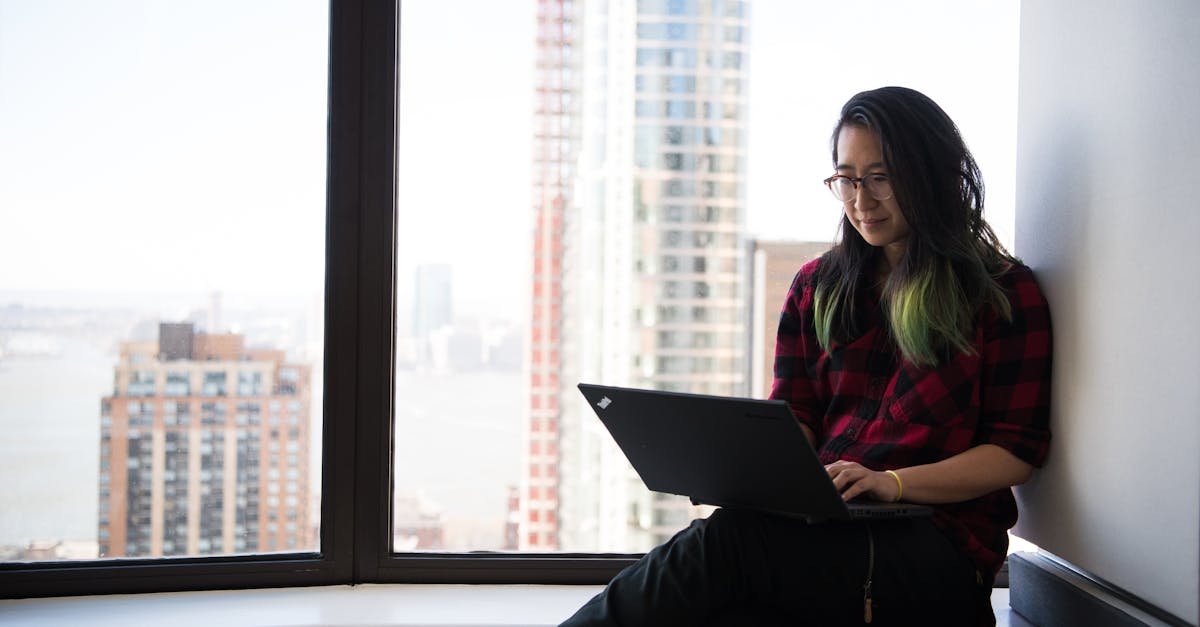
With the advancements in technology, the field of human resources has also seen significant changes. One such change is the use of neural networks, a type of artificial intelligence, in the process of resume screening.
Neural networks have become increasingly popular in various applications due to their ability to learn and make decisions based on patterns and data. In the context of resume screening, neural networks can be used for automating the initial screening process by reading and shortlisting resumes based on job descriptions.
Understanding Neural Networks
Neural networks are computational models inspired by the human brain. They consist of interconnected nodes, known as neurons, that work together to process and interpret information. Each neuron takes inputs, performs mathematical operations on them, and produces an output.
Neural networks are trained by providing them with a large dataset of examples. In the case of resume screening, the dataset would include a collection of resumes along with the corresponding job descriptions and shortlisting decisions made by human recruiters.
Application in Resume Screening
Using neural networks in resume screening can significantly improve the efficiency of the recruitment process. GPT-4, a highly advanced neural network model, has the capability to read and understand job descriptions, enabling it to match relevant skills and qualifications from resumes with the requirements of a particular job.
The utilization of GPT-4 in resume screening involves the following steps:
- Data Preparation: The dataset consisting of job descriptions, resumes, and shortlisting decisions is collected and prepared for training the neural network model.
- Training the Model: The neural network model, GPT-4, is trained using the dataset. During the training process, the model learns to recognize patterns and make decisions based on them.
- Application: After training, GPT-4 is ready to be used in the resume screening process. When a new resume is received, the model analyzes the content and compares it with the job description. It then generates a shortlisting decision based on the matched skills and qualifications.
- Quality Assurance: To ensure the accuracy of the shortlisting decisions made by GPT-4, human recruiters review a sample of the resumes selected by the model.
Benefits and Challenges
The use of GPT-4 in resume screening offers several benefits. It saves time and resources by automating the initial screening process and shortlisting the most suitable candidates. It also eliminates human biases that may arise during the manual screening process.
However, there are challenges associated with using neural networks in resume screening. The accuracy of the shortlisting decisions depends on the quality and diversity of the training dataset. The model may also struggle with less structured or unconventional resumes, causing potential false positives or negatives.
Conclusion
Neural networks, particularly GPT-4, have revolutionized the way resumes are screened in the recruitment process. By leveraging their ability to understand job descriptions and match them with relevant skills and qualifications, neural networks improve the efficiency and objectivity of the resume screening process.
As technology continues to advance, we can expect further enhancements in the capabilities of neural networks, making them an indispensable tool in the field of human resources.
Comments:
Great article, Breaux Peters! I found it very interesting to read about utilizing ChatGPT for resume screening in neural networks technology.
I agree with Lisa, the article is quite informative. It's amazing how artificial intelligence can streamline the resume screening process!
I wonder how effective ChatGPT is in identifying relevant skills and qualifications from resumes. Has it been extensively tested?
Thank you, Lisa and David! Olivia, ChatGPT has undergone rigorous testing and has shown promising results in identifying relevant skills and qualifications from resumes.
That's great to know, Breaux Peters! Having an efficient automated screening process can save a lot of time and effort for HR teams.
Indeed, Olivia. With the increasing number of applicants, automation can significantly speed up the hiring process.
I have some concerns about potential biases in the resume screening process. Has that been addressed in ChatGPT?
I was about to ask the same question, Sarah. It's crucial to ensure fairness and avoid biases in AI-based systems.
That's an important point, Sarah and Rachel. I believe Breaux Peters can shed some light on how bias mitigation is considered in ChatGPT's resume screening.
Sarah and Rachel, addressing biases is a priority. Measures have been taken during the training phase to minimize bias and ensure fair evaluation of applicants.
It's reassuring to know, Breaux Peters. Artificial intelligence systems should be designed with fairness and transparency in mind.
This technology sounds promising. I'm curious if companies have started using ChatGPT for resume screening and if it has proven effective.
Adam, several companies have started utilizing ChatGPT for resume screening, and initial feedback has been positive. It has shown effectiveness in identifying qualified candidates efficiently.
That's great news, Breaux Peters! It seems like ChatGPT has the potential to revolutionize the hiring process.
Absolutely, Adam. AI-powered innovations like ChatGPT can streamline various aspects of business operations.
I'm glad to see organizations adopting advanced technologies like ChatGPT. It can bring efficiency and consistency to the resume screening process.
However, relying solely on AI for resume screening may have its limitations. Human judgment and intuition still play a significant role in evaluating candidates.
I agree with you, Mary. AI can assist, but it can't entirely replace the human element in hiring decisions.
That's a valid point, Mary and Robert. AI should augment human decision-making, not take it over completely.
Mary, Robert, and David, you're absolutely right. ChatGPT is designed to assist HR professionals and augment the screening process, not replace human judgment entirely.
It's fascinating how technology is evolving. What other applications could ChatGPT potentially have in the future?
I believe ChatGPT can be useful in various customer service scenarios, Jack. It has the potential to automate responses and provide accurate information in real-time.
I agree with Samantha. ChatGPT's natural language processing capabilities can be leveraged to improve customer support experiences.
Definitely, David. It could significantly enhance the efficiency and quality of customer interactions.
While the potential of ChatGPT is impressive, I hope companies remain cautious about privacy and data security when implementing such technologies.
I share the same concern, Michael. The responsible and ethical use of AI should always be a priority for organizations.
Absolutely, Emily. There should be strict protocols in place to protect sensitive information during the resume screening process.
I completely agree, Sophia. Safeguarding data and ensuring privacy is crucial to maintaining trust with applicants.
Agreed, Michael. Prioritizing privacy and being transparent about the use of AI technologies will help organizations gain applicants' trust.
With advancements in AI, it's essential to keep upskilling HR professionals to effectively integrate AI-based tools like ChatGPT.
That's a valid point, Robert. HR teams need to adapt and develop new skills to leverage AI in the hiring process efficiently.
Exactly, Laura. Continuous learning and training will enable HR professionals to maximize the benefits of emerging technologies.
I'm glad to see advancements in AI that can streamline hiring processes. It benefits both employers and job seekers.
Indeed, Sophie. A streamlined process ensures that qualified candidates are identified promptly, facilitating overall efficiency.
While AI can accelerate resume screening, I hope it doesn't overlook potential hidden gems amid the flood of applicants.
That's a valid concern, Mark. It's crucial to strike a balance between efficiency and not missing out on potential talent.
Absolutely, Sophia. Human review should still play a role in ensuring valuable candidates aren't inadvertently overlooked.
I appreciate all the comments and concerns shared. The aim of ChatGPT is to assist HR professionals by automating mundane tasks while ensuring fairness, privacy, and human oversight.
Thank you, Breaux Peters, for providing this insight. It's encouraging to see advancements focused on enhancing HR processes.
Agreed, Samantha. Continued innovation in AI will undoubtedly shape the future of HR and recruitment.