Utilizing ChatGPT in Big Data Analytics: Revolutionizing Technology
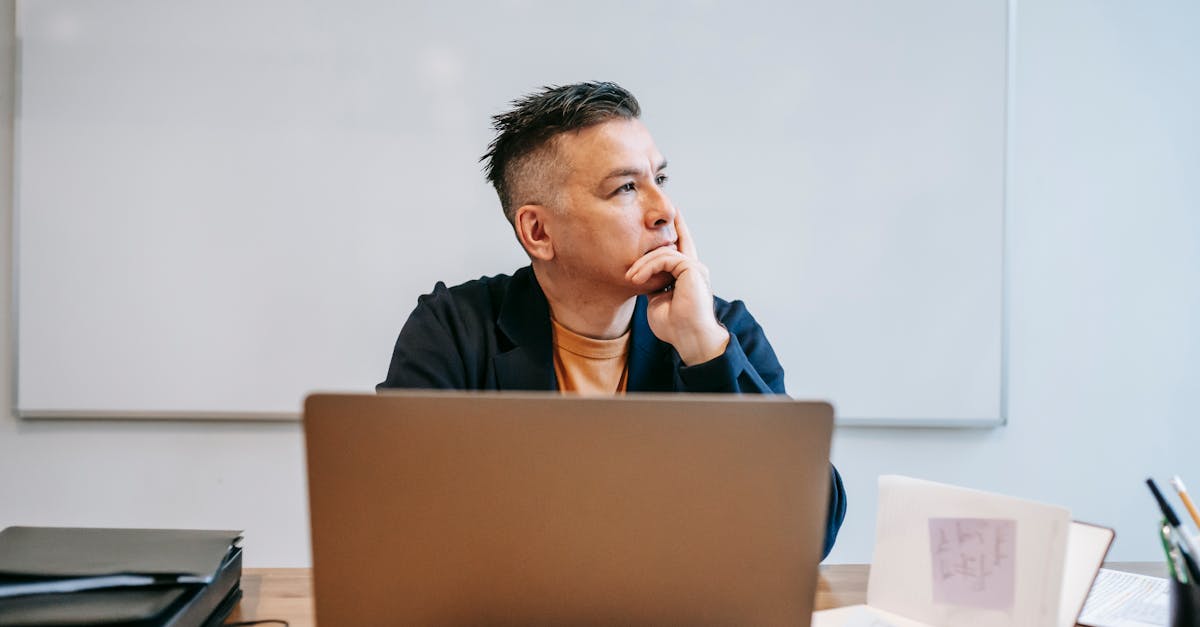
With the rise of artificial intelligence and its integration into everyday tasks, the demand for accurate and reliable data has never been more crucial. Big data analytics is a technology that deals with analyzing large and complex datasets to extract meaningful insights. However, before analysis can take place, the data must go through a process known as data cleaning.
Data cleaning refers to the process of identifying and rectifying errors, inconsistencies, and redundancies in a dataset. This step ensures that the data is accurate, complete, and ready for analysis. In the context of ChatGPT-4, an advanced language processing model, data cleaning plays a significant role in enhancing its performance and accuracy.
How ChatGPT-4 Utilizes Data Cleaning for Accurate Insights
ChatGPT-4 is a powerful language processing model developed by OpenAI that aims to generate human-like responses in conversational contexts. By utilizing big data analytics techniques, ChatGPT-4 can process vast amounts of text data to understand context, generate appropriate responses, and engage in meaningful conversations.
However, the accuracy of ChatGPT-4's responses heavily relies on the quality of the data it is trained on. Inaccurate or inconsistent data can lead to unreliable responses and hinder the model's performance. This is where data cleaning comes into play.
With its advanced big data analytics capabilities, ChatGPT-4 can detect errors, inconsistent data, and redundancies in the training dataset. By identifying and rectifying these issues, the model ensures that it is working with accurate and reliable data, leading to more precise and contextually appropriate responses.
The Significance of Data Cleaning in ChatGPT-4
Data cleaning is crucial in ChatGPT-4 for several reasons:
- Improved Accuracy: By removing errors and inconsistencies, data cleaning significantly improves the accuracy of ChatGPT-4's responses. This allows the model to provide more reliable and trustworthy information to its users.
- Better Context Understanding: Inconsistent or redundant data can confuse ChatGPT-4, leading to irrelevant or incorrect responses. Data cleaning ensures that the model understands the context accurately, enhancing the quality of its generated responses.
- Enhanced Performance: Accurate and clean data allows ChatGPT-4 to perform more efficiently. By eliminating unnecessary noise and inconsistencies, the model can focus on generating high-quality responses, enhancing its overall performance.
- Data Quality Assurance: Data cleaning acts as a quality assurance measure for ChatGPT-4. It ensures that the model is trained on reliable and accurate data, reducing the risk of misinformation and enhancing user satisfaction.
Conclusion
In the world of big data analytics, data cleaning is an essential step in ensuring the accuracy and reliability of analysis. In the case of ChatGPT-4, data cleaning plays a critical role in improving the model's accuracy, context understanding, performance, and data quality assurance.
By utilizing advanced big data analytics techniques, ChatGPT-4 can detect errors, inconsistencies, and redundancies in its training data and rectify them to provide accurate and reliable responses. This ultimately enhances user experience and establishes ChatGPT-4 as a trustworthy conversational AI assistant.
Comments:
Thank you all for reading my article on utilizing ChatGPT in Big Data Analytics. I'm excited to hear your thoughts and answer any questions you may have!
Great article, Sethuraman! ChatGPT has indeed revolutionized the field of big data analytics. Its ability to process vast amounts of information and provide valuable insights is truly remarkable.
I couldn't agree more, Alice. ChatGPT's natural language understanding capability and the ability to generate coherent responses make it a game-changer in analyzing massive datasets.
While ChatGPT is undoubtedly powerful, I think it's important to consider the potential ethical concerns. How do we ensure data privacy and prevent biases in the analysis?
Good point, Charlie. Ethical considerations are crucial in any technological advancement. In the case of ChatGPT, data privacy measures like anonymization and encryption can be employed. Bias detection and mitigation techniques are also needed to address biases.
I have been using ChatGPT in my big data analytics projects, and it has significantly sped up the analysis process. The model's ability to understand complex queries and provide accurate results is impressive.
ChatGPT is undoubtedly a groundbreaking technology. However, have you encountered any limitations or challenges in implementing it for big data analytics?
Absolutely, Eve. While ChatGPT showcases remarkable capabilities, it can sometimes generate responses that appear plausible but are incorrect. This can mislead the analysis if not handled carefully. Validation and verification techniques help address this challenge.
I'm interested in knowing more about the computational requirements of using ChatGPT for big data analytics. Does it demand substantial computational resources?
Good question, Frank. The computational requirements for ChatGPT can be substantial, particularly for processing large-scale datasets. High-performance hardware and distributed computing frameworks can help handle the computational demand efficiently.
I enjoyed your article, Sethuraman! I'm just wondering, how does ChatGPT handle unstructured or noisy data? Can it still provide meaningful analytics in those cases?
Sethuraman, when it comes to unstructured or noisy data, how does ChatGPT handle outliers or anomalies? Can it distinguish reliable patterns from the noise?
Sethuraman, how can ChatGPT handle high-dimensional, multi-modal data? Can it effectively analyze and extract insights from such complex datasets?
In terms of scalability, how well does ChatGPT cope with increasing data volume? Can it handle massive datasets?
Scalability is a key consideration, Grace. ChatGPT can handle massive datasets, but as the size of the data increases, computational requirements can also rise. Distributed computing and parallel processing techniques can be employed to scale the system effectively.
Sethuraman, in your opinion, how can organizations strike a balance between utilizing the power of ChatGPT and ensuring ethical considerations are properly addressed in their analytics?
Grace, accessibility is indeed important. Sethuraman, is it possible for organizations to integrate ChatGPT into existing analytics tools and platforms without requiring significant technical expertise?
Eva, optimizing model size and inference time is crucial for scalability. Sethuraman, what techniques or strategies can organizations employ to achieve efficient performance in large-scale data analysis?
Sethuraman, considering the impact of incorrect or biased outputs generated by ChatGPT, how can organizations implement robust error handling and validation mechanisms in their analytics pipelines?
Grace, I'm also eager to know how effectively ChatGPT can deal with unstructured or noisy data and extract actionable insights from it. Sethuraman, any thoughts on this?
Sethuraman, ensuring accessibility for organizations with varying technical expertise is crucial. What approaches or tools could simplify the adoption of ChatGPT?
What are the potential applications of ChatGPT in big data analytics beyond traditional business intelligence use cases?
Great question, Hank. ChatGPT's flexibility opens up numerous possibilities. It can be used in sentiment analysis, customer support, market research, and even real-time analysis for various industries like healthcare, finance, and social media monitoring.
I'm curious about the learning curve and training requirements for using ChatGPT in big data analytics. Do analysts need specific training to utilize it effectively?
Excellent question, Isabella. While a deep understanding of the underlying algorithms can be beneficial, using ChatGPT for big data analytics doesn't necessarily require analysts to undergo extensive training. It is designed to be user-friendly and accessible to analysts with basic programming and data analysis skills.
As with any technology, security is a primary concern. How can we ensure the security of the data processed by ChatGPT in big data analytics pipelines?
You're right, Jack. Data security is paramount. Secure data transmission protocols, encryption techniques, and access controls can be implemented to protect the confidentiality and integrity of the data processed by ChatGPT in big data analytics pipelines.
Sethuraman, can you provide some insights into how ChatGPT can handle streaming data and deliver near real-time analytics insights? Any performance considerations?
What about the potential biases in the training data used for ChatGPT? How can we ensure equal representation and avoid biased outcomes in big data analytics?
Addressing biases is crucial, Karen. When training ChatGPT for big data analytics, using diverse and representative datasets can help mitigate biases to some extent. Additionally, proactive monitoring and regular audits of the system can help identify and rectify any biases that may arise during analysis.
Sethuraman, comparing ChatGPT to other AI models in big data analytics is intriguing. Are there any specific advantages or unique features that set it apart?
ChatGPT has tremendous potential, but what are the potential risks associated with relying too much on AI models like these in the field of big data analytics?
That's a valid concern, Laura. Over-reliance on AI models like ChatGPT can lead to complacency and the overlooking of potential errors or biases in the analysis. It's crucial to always validate and verify the results generated by such models using multiple techniques and human expertise.
What advancements or future developments do you foresee for ChatGPT in the realm of big data analytics?
A fascinating question, Michael. Further advancements in ChatGPT could involve improved contextual understanding, better handling of domain-specific knowledge, and increased interpretability of the generated responses. These developments would contribute to even more effective and reliable big data analytics using ChatGPT.
How does ChatGPT compare to other existing AI models in the context of big data analytics? Are there any notable differences or advantages?
Good question, Nancy. ChatGPT offers a more conversational and interactive approach compared to traditional AI models. Its ability to understand and respond to queries in a contextual manner makes it highly advantageous for big data analytics, where analysts often need to explore and refine their questions iteratively.
Do you think ChatGPT can eventually replace human analysts in the field of big data analytics?
While ChatGPT and similar AI models are powerful tools for big data analytics, I don't believe they can fully replace human analysts. Human expertise, critical thinking, and domain knowledge are still essential for proper interpretation, validation, and decision-making based on the analysis performed by ChatGPT.
ChatGPT is undoubtedly impressive. Can you share any notable success stories or real-world applications where it has been deployed in big data analytics?
Certainly, Peter. ChatGPT has been successfully deployed in various domains. For instance, it has improved customer support systems by providing automated responses to inquiries based on vast amounts of customer data. It has also been used for sentiment analysis in social media data, assisting in identifying trends and patterns for brands and organizations.
How do you see the future of big data analytics evolving with advancements in language models like ChatGPT?
The future of big data analytics is highly promising with advancements in language models. ChatGPT and similar models can enhance analysts' capabilities to make sense of massive datasets more efficiently. By providing interactive and conversational analysis, these models enable analysts to gain insights quickly and explore data in more intuitive ways.
Are there any specific industries or domains where ChatGPT can have a significant impact on big data analytics?
Absolutely, Rachel. ChatGPT can have a significant impact across various industries. For example, in healthcare, it can assist in analyzing medical records and clinical data. In finance, it can aid in fraud detection and risk analysis. In marketing and advertising, it can provide insights from customer data for targeted campaigns.
What are the key factors organizations should consider before implementing ChatGPT for big data analytics?
Excellent question, Sam. Organizations should consider factors like the scale of their data, available computational resources, potential integration challenges with existing analytics pipelines, data privacy and security requirements, as well as the expertise and training needs of their analysts. A thorough evaluation of these factors will help ensure a successful implementation of ChatGPT for big data analytics.
What are the main advantages and drawbacks of using ChatGPT compared to other AI models in big data analytics?
Good question, Tom. The main advantage of ChatGPT lies in its conversational and interactive nature, allowing analysts to refine their queries iteratively. However, a drawback is that ChatGPT's responses can sometimes be plausible yet incorrect, requiring careful validation. Other AI models may have different trade-offs in terms of performance and features, making it important to choose the most suitable approach based on the specific use case and requirements.
In terms of real-time analytics, how does ChatGPT perform? Can it handle near-instantaneous analysis of streaming data?
Real-time analysis is an important aspect, Uma. While ChatGPT's performance can vary depending on the complexity of the analysis and the computational resources available, it can handle near-instantaneous analysis of streaming data by leveraging distributed computing frameworks and parallel processing techniques.
How challenging is it to integrate ChatGPT with existing big data analytics platforms or pipelines?
Integrating ChatGPT with existing analytics platforms can present some challenges, Vera. However, using standardized protocols and APIs can facilitate the integration process. Additionally, leveraging frameworks like Apache Spark or Apache Flink can help in processing and analyzing data at scale while integrating ChatGPT into the analytics pipeline.
What are the primary considerations for selecting or fine-tuning a ChatGPT model for big data analytics?
When selecting or fine-tuning a ChatGPT model, William, factors like the size and diversity of the training data, the computational resources available, and the specific requirements of the analytics task should be considered. Evaluating the model's performance on relevant benchmark datasets and conducting iterative experiments can help determine the most suitable configuration.
What role does data preprocessing play in utilizing ChatGPT for big data analytics? Are there any specific considerations or challenges?
Data preprocessing is crucial, Xavier. It involves tasks like cleaning, filtering, and transforming the data to make it suitable for analysis by ChatGPT. Challenges can arise in ensuring data quality, handling missing values, and dealing with noisy or unstructured data. Robust preprocessing techniques tailored to the specific characteristics of the data are important for successful utilization of ChatGPT in big data analytics.
What are the potential limitations of ChatGPT in terms of scalability? Can it handle extremely large datasets?
Scalability can be a challenge with very large datasets, Yara. While ChatGPT can handle substantial amounts of data, extremely large datasets may require additional computational resources and efficient distributed processing techniques to ensure timely analysis. Slicing the data into manageable chunks can also be beneficial.
Are there any cost implications associated with utilizing ChatGPT for big data analytics? How does it compare to other approaches in terms of cost?
Cost implications depend on various factors, Zoe. The computational resources required, the scale of the data, and the specific implementation setup can influence the cost of utilizing ChatGPT for big data analytics. It's essential to consider factors like cloud computing costs, hardware requirements, and potential licensing fees when comparing the cost-effectiveness of different approaches.
What level of interpretability does ChatGPT offer in the context of big data analytics? Can analysts understand how it arrives at its conclusions?
Interpretability is an important aspect, Andrea. While ChatGPT's responses can provide insights, it may not offer a detailed breakdown of its decision-making process. Techniques like model introspection, explanation generation, or leveraging augmented intelligence approaches alongside ChatGPT can help analysts gain a better understanding of how conclusions are reached.
What are the potential future challenges that could hinder the widespread adoption of ChatGPT in big data analytics?
Excellent question, Benjamin. Some potential challenges include addressing biases and ethical concerns, improving model accuracy and reliability, reducing computation cost, and enhancing interpretability. Overcoming these challenges will be critical for the widespread adoption of ChatGPT and similar models in big data analytics.
How can organizations ensure that the insights generated by ChatGPT in big data analytics are reliable and trustworthy?
Ensuring the reliability and trustworthiness of the insights is crucial, Catherine. Validation and verification techniques, such as comparing results with existing approaches, conducting A/B testing, or involving domain experts in the analysis process, can help verify the accuracy and reliability of the insights generated by ChatGPT in big data analytics.
What are the potential risks associated with relying solely on ChatGPT for decision-making in big data analytics?
Relying solely on ChatGPT for decision-making can carry risks, Daniel. Despite its capabilities, ChatGPT is an AI model that has limitations and can generate incorrect responses in certain scenarios. It's essential to have human oversight, critical thinking, and a comprehensive evaluation process to avoid making decisions solely based on ChatGPT's output.
What level of technical expertise or programming skills is required to effectively use ChatGPT for big data analytics?
To effectively use ChatGPT for big data analytics, Emilia, basic programming and data analysis skills are necessary. Knowledge of Python and familiarity with libraries for data manipulation and analysis, like Pandas and NumPy, can be beneficial. Additionally, understanding the concepts behind machine learning and natural language processing can enhance the analyst's ability to utilize ChatGPT effectively.
How can analysts ensure that the input queries provided to ChatGPT are phrased in a way that yields accurate and relevant results in big data analytics?
Phrasing queries appropriately is crucial, Fiona. Analysts should ensure clarity and precision in their questions while providing sufficient context. Iterative refinement of queries based on the responses received is also beneficial. Analysts can leverage the capabilities of ChatGPT to engage in conversations, which often helps in eliciting accurate and relevant results in big data analytics.
Is domain-specific training necessary for ChatGPT to be effective in big data analytics, or does it have a broad understanding across domains?
Domain-specific training can enhance ChatGPT's effectiveness in big data analytics, Gary. While it has a broad understanding across domains, fine-tuning the model on domain-specific data can improve its performance. By training ChatGPT with data from the target domain, analysts can leverage its contextual understanding and improve the relevance and accuracy of the generated insights.
Are there any legal or compliance considerations associated with using ChatGPT in big data analytics? How can organizations address them?
Legal and compliance considerations are significant, Hannah. Organizations must ensure that the use of ChatGPT adheres to relevant data protection and privacy regulations. This includes obtaining appropriate consents, anonymizing and encrypting sensitive data, and implementing access controls. Additionally, regular audits and proactive monitoring can help identify and rectify any potential compliance issues that may arise.
Can organizations deploy ChatGPT for real-time big data analytics, or is it more suitable for batch processing?
ChatGPT can be deployed for both real-time and batch processing, Isaac. Its suitability depends on the specific use case and the availability of computational resources. For near-instantaneous analysis of streaming data, appropriate infrastructure and parallel processing techniques should be employed to ensure real-time capabilities.
Can ChatGPT handle structured, semi-structured, and unstructured data equally well in big data analytics?
ChatGPT can handle structured, semi-structured, and unstructured data in big data analytics, Jacob. However, it may exhibit varying degrees of effectiveness depending on the nature of the data. For structured data, where the format and organization are well-defined, other analysis approaches like SQL-based queries may be more suitable. For unstructured or text-based data, ChatGPT can excel in understanding and generating insights.
What are the limitations of ChatGPT in terms of understanding context and nuance in big data analytics?
While ChatGPT possesses impressive contextual understanding abilities, Kelly, it may encounter challenges with subtle nuances and specific domain expertise. Fine-tuning the model on domain-specific data and involving human experts in the analysis process can help overcome these limitations and improve the accuracy and relevance of the generated insights.
Can ChatGPT be used to address real-time anomaly detection or anomaly-based intrusion detection in big data analytics?
Absolutely, Lucas. ChatGPT can be used in conjunction with other anomaly detection techniques to enhance real-time anomaly detection in big data analytics. By analyzing patterns and generating contextual responses based on the data, ChatGPT can contribute to identifying anomalies and potential intrusion attempts.
How can organizations ensure the reproducibility and transparency of the analysis performed using ChatGPT in big data analytics?
Reproducibility and transparency are important, Mia. Organizations can maintain detailed records of the data preprocessing steps, the model configuration, and the specific queries or interactions with ChatGPT. Sharing these records, documenting the analysis pipeline, and open-sourcing the code used can contribute to the reproducibility and transparency of the analysis performed using ChatGPT in big data analytics.
Are there any notable constraints or limitations in terms of the input data size that ChatGPT can handle efficiently in big data analytics?
ChatGPT can handle substantial input data sizes, Noah. However, excessively large datasets may require additional computing resources and efficient processing techniques to maintain efficiency. Breaking down the data into manageable chunks or utilizing distributed computing frameworks can help tackle the constraints associated with extremely large datasets.
What are the primary differences between the free and paid versions of ChatGPT in the context of big data analytics?
The free version of ChatGPT has some limitations, Olivia, such as usage quotas and potential delays during peak periods. The paid versions offer increased access, faster response times, and priority access to new features and improvements. For professional usage in big data analytics, organizations may find the paid versions more suitable due to additional benefits and enhanced performance.
How can organizations ensure data quality and prevent biases when using ChatGPT for big data analytics?
Ensuring data quality and mitigating biases is important, Penelope. Organizations should employ established data cleaning techniques, validate and verify the results generated by ChatGPT using independent approaches, and address any biases that may arise during the analysis process. By promoting diversity and inclusiveness in the training data and involving domain experts, organizations can enhance data quality and minimize biases in big data analytics.
Can ChatGPT be used for predictive analytics in big data analytics? If so, what are the common use cases?
ChatGPT can indeed be used for predictive analytics in big data analytics, Quentin. Common use cases include forecasting future trends based on historical data, predicting customer behavior and preferences, anticipating demand and supply patterns, and identifying potential risks or anomalies. ChatGPT's ability to understand and generate insights from complex data makes it a valuable tool for predictive analytics.
What are the fundamental components or steps involved in utilizing ChatGPT for big data analytics?
The fundamental components in utilizing ChatGPT for big data analytics include data preprocessing, defining queries or interactions with ChatGPT, analyzing the generated responses, and validating the insights. Data cleaning, feature engineering, and model fine-tuning may also be part of the process. Iterative experimentation, debugging, and result interpretation are vital steps to ensure meaningful and accurate analysis.
Can ChatGPT be used for real-time anomaly detection or fraud prevention in big data analytics?
Certainly, Sophia. ChatGPT can be utilized alongside other anomaly detection techniques to enhance real-time anomaly detection. By analyzing patterns, identifying potential anomalies, and generating responses based on the data, ChatGPT can contribute to fraud prevention in big data analytics.
What kind of computational infrastructure or cloud resources are typically required to run ChatGPT for big data analytics?
The computational infrastructure and cloud resources necessary for running ChatGPT in big data analytics depend on the scale of the data and the desired performance, Thomas. High-performance hardware, like GPUs or TPUs, is often beneficial for efficient processing. Cloud providers like AWS, Google Cloud, or Azure offer flexible and scalable infrastructure options that can meet the computational requirements of running ChatGPT for big data analytics.
What are the key factors to consider when evaluating the performance and effectiveness of ChatGPT in big data analytics?
When evaluating ChatGPT's performance in big data analytics, factors like the relevance and accuracy of the generated insights, the response time, and the system's ability to handle the desired workload should be considered, Ursula. Measuring the model's performance against specific metrics and comparing it with other approaches or benchmark datasets can help assess its effectiveness in addressing the analytical requirements.
Can ChatGPT be used for real-time sentiment analysis of social media data in big data analytics pipelines?
Indeed, Victor. ChatGPT can be utilized for real-time sentiment analysis of social media data in big data analytics pipelines. By processing and understanding the textual content of social media posts, ChatGPT can provide valuable insights into the sentiment and opinions expressed by users, helping organizations monitor brand perception, identify emerging trends, or perform targeted social media campaigns.
How can organizations address potential bias issues that may arise when using ChatGPT for sentiment analysis in big data analytics?
Addressing bias issues is crucial, Wendy. Organizations can employ techniques like bias detection and removal algorithms, diversify the training data to include various demographics, and involve human reviewers to validate the sentiment analysis outputs. Regular review and monitoring of the analysis results can help identify and rectify any potential biases that may arise when using ChatGPT for sentiment analysis in big data analytics.
Can ChatGPT be deployed in edge computing or IoT environments for big data analytics?
While edge computing or IoT environments may have resource constraints, Xander, deploying ChatGPT can be challenging. However, by leveraging edge computing capabilities and optimizing the model for resource-constrained environments, it's possible to explore the utilization of ChatGPT in edge computing or IoT setups for big data analytics where real-time analysis is required.
What is the scope of Cloud-Native architectures or serverless computing in utilizing ChatGPT for big data analytics?
Cloud-Native architectures and serverless computing can offer several advantages in utilizing ChatGPT for big data analytics, Yvette. By leveraging the scalability, agility, and cost-efficiency of the cloud, organizations can easily provision resources, handle varying workloads, and scale their analytical pipelines. Serverless computing provides a pay-per-use model and automatic scaling, making it suitable for running ChatGPT in a cost-effective and efficient manner.
Thank you all for reading my article on 'Utilizing ChatGPT in Big Data Analytics: Revolutionizing Technology'. I'm excited to discuss your thoughts and answer any questions you may have!
Sethuraman, in terms of scalability, does ChatGPT rely on distributed computing for handling large volumes of data efficiently? Or can it leverage other methods?
Sethuraman, any advice on optimizing the model size and inference time? These factors can be critical, especially when dealing with immense amounts of data in real-time scenarios.
Sethuraman, apart from distributed computing, are there any other methods or architectures that ChatGPT can utilize for scaling up and handling large volumes of data efficiently?
Sethuraman, efficient performance at large scale is crucial. Are there any advancements or ongoing research in the area of optimizing model size and inference time?
Sethuraman, while the benefits of ChatGPT are evident, can you shed some light on how it makes decisions and if those decisions are interpretable in a transparent manner?
Wow, Sethuraman, your article was mind-blowing! The potential of ChatGPT in big data analytics is really revolutionary. I loved the examples and use cases you mentioned. Great work!
I agree with you, Alice. ChatGPT can definitely transform the way we analyze and make decisions based on big data. Do you think there are any limitations or challenges we should consider though?
Bob, great question! ChatGPT indeed has some limitations. For example, it may generate plausible-sounding but incorrect responses. It's essential to carefully validate the generated outputs.
That's an important point, Alice. It's crucial to establish a feedback loop with domain experts and continuously refine the model to minimize such errors.
Catherine, I haven't personally used ChatGPT in big data analytics yet. However, I've been following some case studies where it showed promising results. It'd be interesting to hear more from Sethuraman.
Alice, good point about validating the generated outputs. Sethuraman, how can organizations ensure the trustworthiness of the analytics derived from ChatGPT?
Alice, thanks for your response. I'm eager to try using ChatGPT in big data analytics, but understanding its real-world performance is crucial. Sethuraman, any insights on this?
Catherine, absolutely! Continuous feedback and refinement are key in dealing with language models like ChatGPT, especially when analyzing big data.
Alice, thanks for sharing your thoughts. Understanding the practical implementation aspects of ChatGPT is crucial, especially for organizations looking to adopt it.
Catherine, the trustworthiness of analytics derived from ChatGPT is vital. Sethuraman, how can organizations validate and ensure the accuracy of ChatGPT outputs?
Alice, thanks for bringing up the trustworthiness aspect. Sethuraman, do you think continuous monitoring of ChatGPT's performance is necessary? How can organizations ensure accountability?
Alice, I completely agree. Collaboration between experts and language models like ChatGPT is vital to minimize errors and ensure reliable analytics.
Catherine, absolutely! Feedback from domain experts can help uncover the limitations and biases of ChatGPT over time, ultimately improving its analytics capabilities.
Alice, understanding the practical aspects before implementation is key. Sethuraman, any advice on how organizations can prepare their existing data and infrastructure to be ChatGPT-friendly?
Alice, practical implementation details are indeed important. Sethuraman, can you share any use cases where organizations with limited technical expertise successfully adopted ChatGPT for big data analytics?
Alice, continuous monitoring and accountability are definitely necessary for reliable analytics. Sethuraman, how can organizations establish such monitoring processes effectively?
Alice, I'm curious to know if you've personally used ChatGPT in big data analytics. It sounds promising, but I wonder how it performs in real-world scenarios.
Sethuraman, great article! I'm particularly interested in the scalability aspect of using ChatGPT in big data analytics. How does it handle large volumes of data efficiently?
David, I think scalability is an important point. I remember reading about potential issues with model size and inference time. Sethuraman, could you share some insights on this?
Eva, you bring up a valid concern. The size of the model can indeed be an issue when dealing with big data. Sethuraman, any insights on optimizing model size and inference time?
Sethuraman, your article was fascinating! I can see ChatGPT revolutionizing big data analytics, but what are some potential ethical considerations we need to keep in mind?
Grace, I have similar concerns about unstructured data. Sethuraman, how does ChatGPT handle unstructured or noisy data? Can it extract insights effectively?
I found the article informative, Sethuraman! However, I wonder if ChatGPT is accessible and usable for organizations without advanced technical expertise or resources. What are your thoughts?
Henry, that's an important consideration. Organizations with varying levels of technical expertise should have access to these advanced analytics tools. Sethuraman, what are your thoughts on this accessibility issue?
Sethuraman, your article was a great read! I'd love to hear your opinion on the security and privacy concerns associated with utilizing ChatGPT in big data analytics.
Isabel, great point about security and privacy. Data is crucial, so it's essential to ensure that ChatGPT is employed within strong security and privacy frameworks. Sethuraman, any insights on this?
Isabel, security and privacy are essential in this domain. Sethuraman, what measures can organizations take to protect sensitive data when applying ChatGPT in big data analytics?
This article excited me about the potential of ChatGPT in big data analytics. Sethuraman, how can organizations get started with implementing ChatGPT in their existing analytics pipelines?
Jason, that's a practical question. Sethuraman, can you suggest any best practices or resources for organizations to start implementing ChatGPT in their analytics pipelines?
Jason, Sethuraman's insights on best practices and resources for ChatGPT implementation would be really valuable. I'm also keen to learn more about this.
Sethuraman, your article was enlightening! I'd like to know if ChatGPT can handle streaming data and provide real-time analytics insights.
Great job, Sethuraman! I wonder how ChatGPT compares to other AI models when it comes to big data analytics. What are its unique advantages?
Sethuraman, you explained the benefits of ChatGPT well! However, I'm concerned about the interpretability of its decision-making process. Can you shed some light on this?