Utilizing ChatGPT in Machine Vision: Enhancing Food Recognition for Dietary Apps
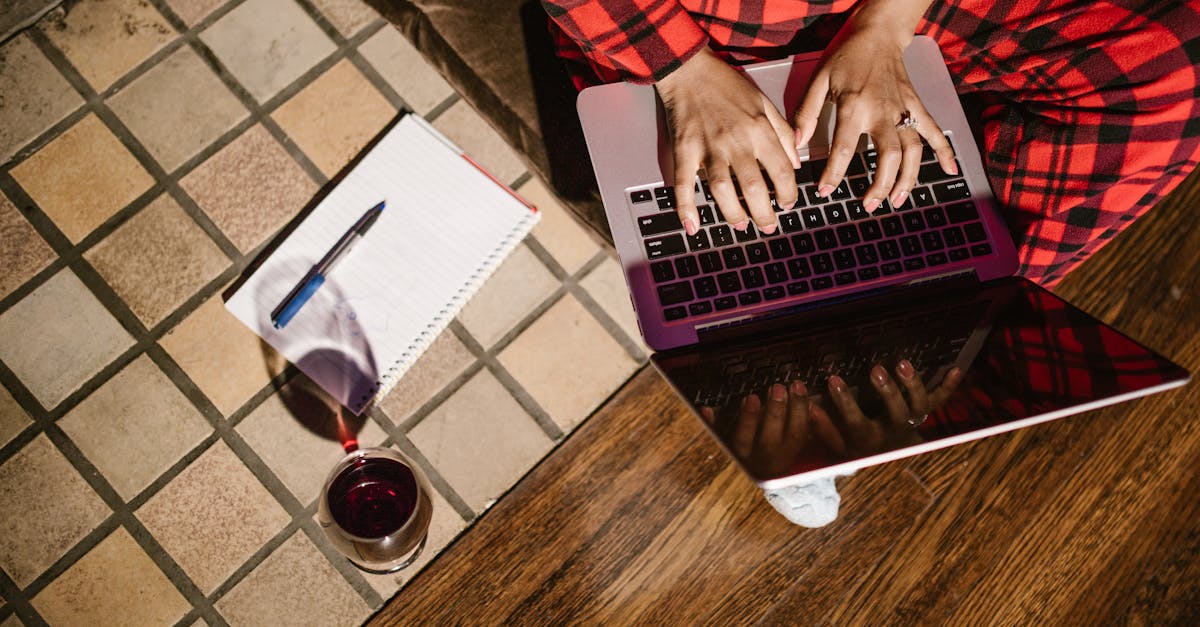
Machine vision, a subset of artificial intelligence, is revolutionizing the way we interact with technology. One area where machine vision is making a significant impact is in food recognition for dietary apps. With the advancements in machine learning algorithms and neural networks, it is now possible to accurately classify and calculate the nutrition of various foods using pictures captured through dietary tracking apps.
ChatGPT-4, powered by machine vision technology, plays a crucial role in helping individuals effortlessly track their nutritional intake. This innovative integration allows users to take a picture of their meal and receive detailed information about its nutritional content.
How Does Machine Vision Work for Food Recognition?
Machine vision algorithms follow a series of steps to recognize and classify food items accurately:
- Image Acquisition: The user captures an image of their meal using their device's camera through the dietary tracking app.
- Preprocessing: The acquired image is processed to remove noise, adjust lighting, and enhance the overall quality.
- Feature Extraction: Machine learning algorithms identify distinctive features in the image, such as color, texture, shape, and size.
- Classification: The extracted features are analyzed and compared against a pre-trained model that has been trained on a vast database of food images. This step determines the most likely food category to which the image belongs.
- Nutrition Calculation: Once the food item is identified, the machine vision system references a comprehensive nutritional database to determine its nutritional information, including calories, macronutrients, vitamins, and minerals.
Benefits of Machine Vision in Dietary Apps
The integration of machine vision in dietary apps offers several benefits:
- Efficiency: Manual input of food items can be time-consuming and prone to errors. Machine vision simplifies the process by eliminating the need for manual data entry.
- Accuracy: Machine vision algorithms have been trained on vast datasets, making them highly accurate in identifying food items and calculating their nutritional content.
- Convenience: Users can effortlessly track their nutritional intake by simply taking a picture of their meals using their smartphones, reducing the barriers to effective dietary tracking.
- Personalization: Machine vision technology can be customized to cater to individual dietary needs, making it an invaluable tool for those with specific dietary restrictions or preferences.
Limitations and Future Developments
While machine vision technology for food recognition in dietary apps has made tremendous progress, it does have certain limitations:
- Food Variations: Recognizing regional or cultural variations of the same food item can be challenging for machine vision algorithms.
- Ingredient Blends: Identifying individual ingredients in complex dishes can be difficult, particularly when they are mixed together.
- Portion Estimation: Accurately estimating portion sizes based on images alone is a complex task that still requires refinement.
Despite these challenges, ongoing research and advancements in machine vision show promising developments. Future iterations, such as ChatGPT-4, will continue to improve both accuracy and versatility, making food recognition in dietary apps even more reliable and user-friendly.
Conclusion
Machine vision is transforming the way we track and manage our nutrition through food recognition in dietary apps. With ChatGPT-4, users can effortlessly classify and calculate the nutritional content of their meals by simply taking a picture. While there are limitations to overcome, the continued progress in machine vision brings us closer to a future where accurate and convenient dietary tracking is accessible to all.
Comments:
Great article! I'm excited to see how ChatGPT can improve food recognition for dietary apps.
I agree, Sarah. The potential for more accurate food recognition is very promising.
As a dietitian, I think this innovation could greatly benefit dietary apps. Looking forward to trying it!
Thank you, Sarah, Tom, and Linda, for your positive feedback! I'm glad you see the potential in utilizing ChatGPT for food recognition.
This could be a game-changer for people looking to track their calorie intake. Exciting stuff!
I'm curious how accurate ChatGPT can be when it comes to recognizing different types of food.
That's a great point, Emily. ChatGPT has shown promising results, but it's important to continue testing and refining its accuracy for different food categories.
Do you think ChatGPT can overcome the challenge of accurate portion size estimation? That seems crucial for dietary apps.
Portion size estimation is indeed a challenge, Lucas. While ChatGPT can assist in recognizing food items, it may require additional algorithms or user input for accurate portion estimation.
I hope using ChatGPT won't compromise user privacy. How will the data be handled?
Privacy is a valid concern, Sophie. When developing systems using ChatGPT, it's essential to prioritize data protection and ensure user consent for data usage.
I'm wondering if ChatGPT can handle dietary restrictions and allergies while recognizing food.
Adapting ChatGPT to consider dietary restrictions and allergies is a crucial aspect. This can be achieved by training the model on labeled data specific to these restrictions.
This technology could be a great tool for people with conditions like diabetes who need to track their carbohydrate intake.
Absolutely, Olivia. Improving food recognition can greatly assist individuals with conditions requiring precise macronutrient tracking.
I wonder how long it will take for dietary apps to integrate ChatGPT and make it widely available to users.
Adoption timelines may vary, Ethan. It depends on factors like research advancements, industry collaboration, and user adoption rates.
Are there any limitations to using ChatGPT in food recognition? It sounds almost too good to be true.
There are limitations, Mia. ChatGPT may struggle with low-quality images, unusual food items, or complex dishes. It's an ongoing challenge to improve its robustness.
I'm concerned about potential biases in food recognition. Can ChatGPT handle diverse cuisines and cultural food variations?
Bias is an important issue, Peter. Ensuring diversity in training data and ongoing evaluation will be pivotal to address biases and make food recognition more inclusive.
How much training does ChatGPT require to achieve good accuracy in food recognition?
ChatGPT requires training on large datasets to achieve good accuracy. Continued research and iterative improvements are necessary for better results.
I hope dietary apps will maintain their user-friendly interfaces while integrating ChatGPT. Sometimes too much technical complexity can be off-putting.
That's a valid concern, Jason. Striking a balance between technical advancements and user-friendly interfaces is crucial for successful integration.
I'm excited to see how ChatGPT can enhance the effectiveness of meal planning apps. It could save a lot of time and effort!
Indeed, Isabella. By streamlining food recognition, ChatGPT has the potential to significantly improve the accuracy and convenience of meal planning apps.
How does ChatGPT handle real-time recognition for food items when capturing images?
Real-time recognition is a challenge, Kevin. It requires efficient image processing and model optimization to ensure quick and accurate results.
I'm concerned about potential misidentification of food items. How can ChatGPT be reliable enough?
Minimizing misidentification is crucial, Jennifer. Continual improvement of the model's training data and design can help enhance the reliability of ChatGPT in food recognition.
Will ChatGPT be accessible to small dietary app developers or only the big players?
Integration possibilities will depend on factors like model availability and affordability, Robert. Efforts are being made to democratize access to such technology.
I'm fascinated by how machine vision is revolutionizing various fields. Food recognition can be a game-changer for dietary tracking!
It's incredible to witness the impact of machine vision, Karen. The potential improvements in food recognition can indeed revolutionize dietary tracking and overall health management.
Has ChatGPT been tested extensively on real-world food images? Results from lab tests can sometimes differ from real-world scenarios.
You're right, Adam. Real-world testing is crucial to understand and address the challenges faced by ChatGPT in food recognition. Extensive testing is an ongoing process.
I'd love to see ChatGPT integrated with recipe apps to provide accurate nutritional information for homemade meals.
That's an intriguing idea, Catherine. Integrating ChatGPT into recipe apps could indeed provide valuable nutritional information for homemade meals and aid in dietary planning.
I wonder if ChatGPT can handle food recognition in different lighting conditions. That could be a challenge.
Food recognition in varying lighting conditions is indeed a challenge, Samuel. It requires robust image processing techniques and training on diverse data to handle such scenarios.
Will there be an option for users to correct misidentified food items if they encounter any? User feedback could help improve the system.
User feedback is invaluable, Julia. Integrating a user-friendly feedback mechanism can help improve food recognition accuracy over time.
Can ChatGPT recognize multiple food items in a single image? That could be useful for tracking complex meals.
Recognizing multiple food items in a single image is an interesting challenge, Daniel. It can offer enhanced meal tracking capabilities, but it requires advanced model architectures and training data.
What about modifications or customized recipes? Can ChatGPT adjust its recognition to fit individual preferences?
Customization is important for user satisfaction, Melissa. Creating personalized recognition capabilities within ChatGPT can improve its usefulness for customized recipes and individual preferences.
I hope dietary apps using ChatGPT will have clear disclaimers about the limitations and potential inaccuracies of the system.
Transparency is key, Wayne. Clear communication about ChatGPT's limitations and the need for user validation will be essential for responsible use in dietary apps.
Overall, this article highlights exciting possibilities. I'm looking forward to seeing how ChatGPT evolves in food recognition applications!
Thank you, Susan, and everyone who participated in this discussion. The potential of ChatGPT in food recognition is indeed exciting. Let's anticipate its evolution and impact in dietary apps!