Utilizing ChatGPT to Mitigate Liquidity Risk in the Digital Age
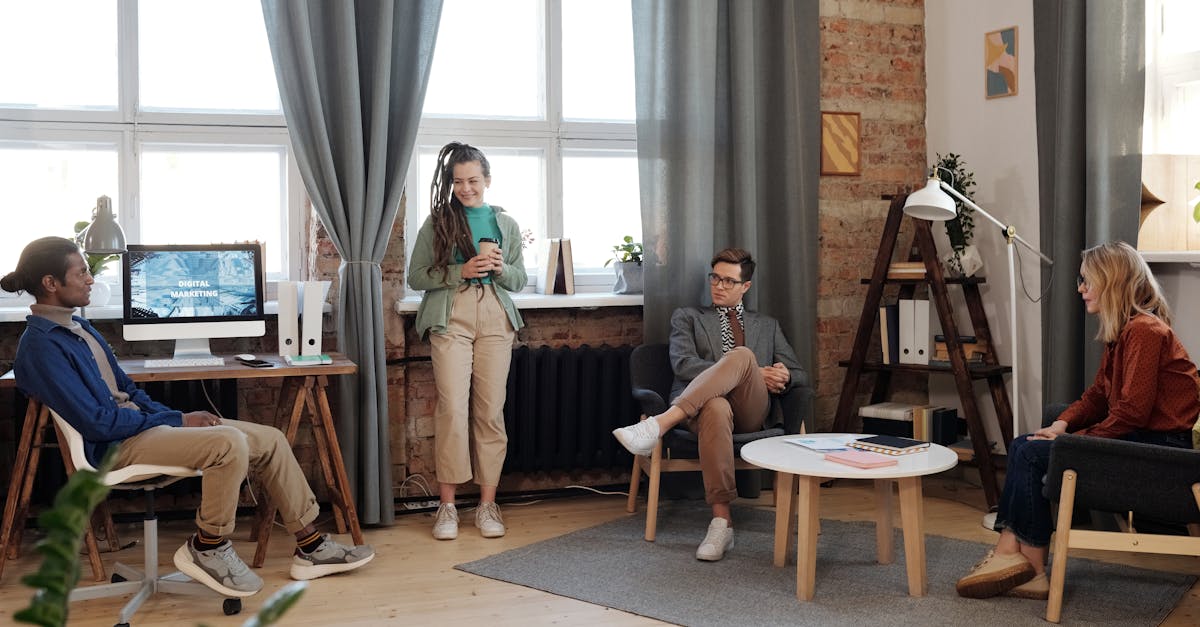
Introduction
Liquidity risk, per se, is the potential vulnerability for an organization, usually a financial institution, to not meet its short-term or long-term obligations due to an inability to convert its assets into cash without incurring significant loss. It might sound less incendiary than credit risk or market risk, but the 2008 financial crisis has manifested that liquidity risk can devastate entire economies. Recently, a novel solution has emerged in the form of OpenAI's latest version of Generative Pre-trained Transformer, known as ChatGPT-4, which can be employed to analyze market data, scrutinize historical trends and predict possible scenarios. Anticipating adverse scenarios before they unfold gives an asset or risk manager a significant advantage in mitigating risks and ensuring financial stability.
The Concept of Liquidity Risk
Before plunging into the usage of ChatGPT-4 for liquidity risk assessment, it is pivotal to understand more about this type of risk. In simplistic terms, liquidity risk is the risk that a company or individual will not be able to meet short-term financial demands. This usually occurs due to the inability to convert assets into cash without facing loss. This risk is intrinsically associated with the solvency of the market participants as well as market stability.
ChatGPT-4: The Breakthrough Technology
ChatGPT-4, an advanced version of its predecessor ChatGPT-3, is an AI-powered language model developed by OpenAI. It is capable of synthesizing human-like text based on input data, making it exceptional for numerous tasks, including translating languages, writing essays, and even generating poetry. In short, it has been trained to predict the probability of a word given its preceding words and can be fed with vast, specific language data, making it astoundingly effective in many use cases.
The Application of ChatGPT-4 in Risk Assessment
A big stride forward in harnessing AI in liquidity risk management, implementing ChatGPT-4 in this domain seems promising. It can handle vast amounts of unstructured data, process it, and generate insightful risk analysis. This involves analysing historical trends, interpreting market data, and synthesizing probable scenarios. In the context of liquidity risk management, such capability could be useful in discerning patterns in market data that could suggest a potential liquidity crisis. Additionally, by analyzing historical trends, ChatGPT-4 can aid risk managers in understanding how the liquidity conditions of their holdings have evolved over time. Moreover, its ability to predict possible scenarios could prepare businesses for various situations, allowing them to devise necessary preemptive strategies.
Analyzing Market Data
Market data proffers a wealth of information about factors which can influence liquidity. Through machine learning algorithms, the model learns to understand how different factors correlate with liquidity risk. The more data it has, the more nuanced its analysis is. This allows for the generation of a more robust and complete analysis than a human analyst might be able to achieve.
Identifying Historical Trends
Understanding historical trends in the market can unveil insights about potential liquidity risks. Given the vast amounts of data, ChatGPT-4 can reveal subtler patterns and trends that might be overlooked by human analysts. By harnessing the computational power of ChatGPT-4, risk managers can considerably enhance their risk detection accuracy.
Predicting Possible Scenarios
By synthetizing historical data and current market conditions, ChatGPT-4 can predict various scenarios, including those that have not occurred but are possible given the available data. These scenarios can help organizations prepare for different situations, thus minimizing potential losses.
Conclusion
The facilitation of AI in risk management, particularly liquidity risk, is rapidly evolving and ChatGPT-4 represents the forefront of this technology. Harnessing its processing power and predictive capabilities will provide a significant edge to organizations, enabling them to ameliorate their risk management strategies and ensure a more secure, stable financial future.
Comments:
Thank you all for taking the time to read my article on utilizing ChatGPT to mitigate liquidity risk in the digital age. I'd love to hear your thoughts and opinions on this topic!
Great article, Marty! I've always been interested in how AI can be leveraged in the financial industry. ChatGPT seems like a promising tool for managing liquidity risks.
Thank you, Sarah! I agree, ChatGPT can definitely help financial institutions navigate liquidity risks more effectively. Have you come across any specific use cases or challenges in this area?
I enjoyed reading your post, Marty. The idea of using AI to tackle liquidity risk is intriguing. It could potentially enhance decision-making processes and provide real-time insights.
Thanks, Michael! You're right, AI-powered tools like ChatGPT can offer valuable real-time insights, which can be crucial in managing and mitigating liquidity risks. In your opinion, what are some of the key benefits of such technologies?
This is an interesting perspective, Marty. It's crucial for financial institutions to adapt and adopt new technologies to better manage liquidity risk in the digital age. However, what are the potential risks and limitations of relying solely on AI?
That's a great question, Emily! While AI technologies like ChatGPT can be valuable, they do have their limitations. One potential risk is overreliance on AI systems without human oversight. It's important to strike a balance and complement AI with human judgment in managing liquidity risks.
Marty, I appreciate your article shedding light on applying AI to liquidity risk. However, what are the potential ethical concerns that arise when implementing AI in the financial industry?
Excellent point, David! The use of AI in finance does raise ethical concerns, such as bias in training data and the impact of algorithmic decisions on individuals. Responsible and transparent AI development and deployment should be a top priority to address these concerns.
Interesting read, Marty. Do you think the widespread adoption of AI in the financial industry will face resistance from industry professionals fearing job displacement?
Thanks, Olivia! The concern of job displacement is valid. However, AI technologies like ChatGPT can assist professionals in making better decisions, rather than replacing them entirely. It's essential for professionals to adapt and upskill to embrace these technological advancements.
Marty, as an AI enthusiast, I'm excited about the potential of ChatGPT in mitigating liquidity risk. Are there any limitations in terms of data availability that might hinder its effectiveness?
Great question, Ethan! Data availability can indeed be a challenge. The effectiveness of ChatGPT relies on the quality and quantity of data it's trained on. Ensuring access to relevant, diverse, and accurate data is crucial for its optimal performance.
Marty, thanks for sharing your insights. One concern I have is regarding the interpretability of AI models like ChatGPT. Can we trust their decisions when it comes to managing liquidity risks?
Valid concern, Sophie! Interpreting the decisions made by AI models can be challenging. Ensuring transparency and interpretability in AI systems is vital, especially in managing liquidity risks where accountability and understanding the decision-making process are crucial.
Really knowledgeable article, Marty. Do you think regulators are prepared to address the implications and risks associated with AI-powered tools used in managing liquidity risks?
Thank you, Liam! Regulators are indeed aware of the implications of AI in finance. As AI adoption increases, regulators need to keep pace with technology to establish appropriate frameworks that address risks while fostering innovation and ensuring the stability of the financial system.
Marty, I found your article thought-provoking. How do you see the future of AI-powered risk management tools evolving in the context of liquidity risks?
I'm glad you found it thought-provoking, Emma! The future of AI-powered risk management tools looks promising. Continued advancements in AI algorithms, increased data availability, and collaboration between humans and AI will shape the evolution of effective liquidity risk management in the digital age.
Thank you all for your valuable insights and engaging in this discussion! It's been a pleasure discussing the potential of ChatGPT in mitigating liquidity risk. If you have any more questions or thoughts, feel free to share.
Thank you all for taking the time to read my article on 'Utilizing ChatGPT to Mitigate Liquidity Risk in the Digital Age'. I look forward to hearing your thoughts and engaging in a meaningful discussion.
Great article, Marty! I agree that using ChatGPT to mitigate liquidity risk is an interesting approach. It can provide real-time insights and analysis, especially when combined with algorithmic trading. However, I do wonder about the potential ethical concerns that may arise from removing human decision-making in such critical situations.
I share similar concerns, Peter. While using AI to mitigate liquidity risk has its advantages, we should also consider the possible downsides. Human intervention and oversight are crucial for maintaining a healthy balance between automation and human decision-making. Without proper safeguards, relying solely on ChatGPT could lead to unintended consequences.
I find the concept fascinating, Marty. However, I would like to better understand how ChatGPT handles complex real-world scenarios. Are there any limitations or challenges we need to be aware of when implementing this technology?
That's an excellent question, Sarah. While ChatGPT shows great promise, it does have limitations. It can sometimes generate plausible but incorrect responses, especially when dealing with complex or ambiguous scenarios. This underscores the importance of having human experts involved in the decision-making process to verify and validate the AI-generated insights.
I appreciate the potential benefits highlighted in the article, Marty. Still, I wonder about the implementation challenges associated with integrating ChatGPT into existing financial systems. How easy or difficult would it be to adopt ChatGPT in practice?
Thank you for your question, David. Integrating ChatGPT into existing financial systems can indeed pose some challenges, particularly with regards to data privacy, security, and compliance. Additionally, developing the necessary infrastructure and expertise to train and deploy ChatGPT effectively requires significant resources. It's a multi-faceted process that needs careful planning and considerations of the unique requirements within each organization.
I agree, Marty. Privacy and security should be top priorities when implementing ChatGPT in financial systems. The potential benefits are great, but we must ensure that sensitive data is appropriately protected from unauthorized access or misuse.
Marty, your article raises an interesting point about using ChatGPT to mitigate liquidity risk. However, I wonder about the potential impact on job roles within the financial industry. Could widespread adoption of AI technologies like ChatGPT lead to significant job losses?
That's a valid concern, Michael. While AI technologies may automate certain tasks, it is important to note that they also create new opportunities. Rather than replacing jobs, they often augment human capabilities, enabling professionals to focus on higher-level decision-making and more strategic aspects of their roles. However, proper education and retraining programs are essential to help individuals adapt to the changing landscape.
Marty, I enjoyed reading your article. However, I wonder about the potential biases that might exist in ChatGPT, especially when it comes to making critical financial decisions. How can we ensure that the AI models are unbiased and fair?
You raise an important point, Robert. Addressing biases in AI models is crucial to ensure fair and ethical decision-making. To mitigate biases, it's essential to carefully design the training process, consider diverse and representative datasets, and actively monitor and assess the AI system's outputs. Transparency and accountability are key in building trustworthy AI models that can help mitigate liquidity risk without introducing unintended biases.
As an AI enthusiast, I find the idea of using ChatGPT to mitigate liquidity risk quite fascinating. Marty, do you have any suggestions for further research or areas where ChatGPT can be utilized in the financial industry?
Absolutely, Julia! There are several exciting areas where ChatGPT can be further explored. For instance, it can be used in real-time fraud detection, algorithmic trading strategies, personalized financial advice, or even streamlining customer support services. The potential is vast, and further research can unlock new ways to leverage ChatGPT's capabilities in the financial industry.
Marty, your article presents an interesting perspective on mitigating liquidity risk. However, I'm curious about the computational requirements and costs associated with deploying ChatGPT at scale. Could it be a barrier for smaller financial institutions?
That's a valid concern, Sophia. Deploying ChatGPT at scale does come with computational requirements and costs. However, with advancements in cloud infrastructure and services, smaller financial institutions can access AI capabilities without needing to invest heavily in their own hardware. Additionally, collaborations with AI service providers can help reduce the barriers to entry and enable more widespread adoption.
Marty, I appreciate your insights on utilizing ChatGPT for liquidity risk mitigation. However, do you think there could be potential regulatory challenges or skepticism from regulatory bodies when it comes to implementing AI technologies in the financial sector?
You bring up an important consideration, Daniel. Regulatory challenges and skepticism are indeed factors to consider when implementing AI technologies in the financial sector. However, as AI technology matures, regulatory bodies are also adapting and working towards frameworks that ensure responsible and trustworthy AI deployment. Engaging in open dialogue and collaboration between industry participants, regulators, and policymakers can help navigate these challenges.
Marty, your article offers an interesting perspective on utilizing AI for liquidity risk mitigation. How do you see the future of AI evolving in the financial industry, particularly in terms of improving risk management processes?
Thank you for your question, Oliver. The future of AI in the financial industry looks promising, especially when it comes to risk management processes. As AI technologies continue to advance, we can expect more accurate risk assessments, enhanced fraud detection capabilities, and real-time insights for better decision-making. However, it's essential to strike the right balance between AI automation and human expertise to ensure responsible and effective risk management.
Marty, I found your article thought-provoking. However, could you discuss any potential limitations or risks associated with relying heavily on AI systems like ChatGPT for critical financial decision-making?
Absolutely, Jennifer. While AI systems like ChatGPT offer significant benefits, there are also potential limitations and risks to consider. Some of these include biases in data, lack of interpretability of AI models, and the need for continuous monitoring and updates. Additionally, over-reliance on AI without human oversight can lead to unintended consequences and errors. These risks highlight the importance of adopting responsible AI practices and maintaining a balance between human judgment and AI-driven insights.
Marty, your article raises interesting points about utilizing AI for liquidity risk management. I'm curious about the timeline for implementing such technologies in the financial sector. How long do you think it will take for widespread adoption of AI systems like ChatGPT?
Thank you for your question, Liam. The timeline for widespread adoption of AI systems like ChatGPT can vary depending on several factors, including industry readiness, regulatory frameworks, and technological advancements. While larger financial institutions may adopt AI technologies sooner, smaller organizations may take more time to navigate the complexities. However, as awareness grows and best practices emerge, we can expect a gradual but progressive adoption of AI systems like ChatGPT within the financial sector.
Marty, your article provides an interesting perspective on leveraging AI for liquidity risk mitigation. However, what are the potential challenges in convincing stakeholders and decision-makers to adopt AI systems like ChatGPT?
That's a great question, Grace. Convincing stakeholders and decision-makers to adopt AI systems like ChatGPT can involve several challenges. These include ensuring transparency and explainability of AI models, showcasing tangible value and return on investment, addressing concerns around job displacement, and building trust through successful pilot projects and industry use cases. Demonstrating the benefits, risks, and responsible implementation of AI systems are key in gaining the confidence and support of decision-makers.
Marty, thank you for sharing your insights on leveraging AI for liquidity risk mitigation. I'm curious to know if you foresee any potential resistance from financial professionals who may be skeptical or reluctant to embrace AI for critical decision-making in the industry?
You raise an important point, Isabella. Resistance or skepticism from financial professionals is indeed a potential challenge when it comes to embracing AI for critical decision-making. To address this, it's crucial to involve professionals early on and provide them with opportunities to understand and contribute to the development and deployment of AI systems. Education, training, and showcasing successful case studies can help alleviate concerns and foster a collaborative environment where AI and human expertise can work hand in hand.
Marty, your article offers valuable insights on leveraging AI systems for liquidity risk mitigation. What would be the key considerations for organizations looking to implement ChatGPT or similar AI technologies?
Thank you for your question, Nathan. Organizations considering the implementation of ChatGPT or similar AI technologies should carefully assess their specific needs, data availability, and infrastructure readiness. They should prioritize data privacy and security, ensure compliance with regulations, and establish governance frameworks for responsible AI usage. Collaborating with AI experts, incorporating feedback loops, and conducting thorough testing and validation are also crucial for successful adoption and integration of ChatGPT.
Marty, I thoroughly enjoyed reading your article. How do you envision the role of AI systems like ChatGPT evolving in the future when it comes to liquidity risk management?
Thank you, Lily. The role of AI systems like ChatGPT in liquidity risk management is likely to evolve significantly. As AI technologies continue to advance, they will provide more accurate and real-time insights, helping organizations proactively identify and address liquidity risks. Furthermore, AI systems can play a vital role in stress testing, scenario analysis, and predictive modeling, enabling financial institutions to make better-informed decisions and enhance their overall risk management practices.
Marty, your article sheds light on an interesting application of AI for liquidity risk mitigation. However, could you elaborate on the potential limitations or challenges of implementing AI systems like ChatGPT in a rapidly changing financial landscape?
Certainly, Chris. Implementing AI systems like ChatGPT in a rapidly changing financial landscape can pose challenges. The evolving regulatory landscape, continuous technological advancements, and dynamic market conditions require AI systems to be adaptable and updatable. Additionally, staying ahead of emerging risks, managing large-scale data, and addressing varying requirements across financial institutions are factors to consider. Successful implementation requires agility, strategic planning, and an ongoing commitment to research and development.
Marty, thank you for sharing your insights on leveraging AI for liquidity risk mitigation. What would be the key considerations for organizations when it comes to data governance and the responsible use of AI systems?
You're welcome, Jake. Data governance and responsible use of AI systems are crucial considerations for organizations. They should prioritize data quality, accuracy, and privacy to ensure the integrity and reliability of AI models. Establishing clear guidelines for data acquisition, storage, and usage is essential. Additionally, organizations must regularly monitor and audit AI systems to detect and address any biases or unintended consequences. Transparent and ethical practices surrounding data governance are fundamental in building trust and fostering responsible AI usage.
Marty, your article provides valuable insights into the potential of AI for liquidity risk mitigation. However, how can financial institutions ensure the explainability and transparency of AI-driven decisions?
An excellent question, Emma. Ensuring the explainability and transparency of AI-driven decisions is crucial for building trust within financial institutions and regulatory frameworks. One approach is to adopt interpretable AI models or develop explainability techniques that provide insights into the decision-making process. Making AI models more understandable and traceable helps stakeholders comprehend and validate the decisions being made. Striking the right balance between AI capabilities and explainability is key in achieving trustworthy and transparent AI-driven processes.
Marty, your article highlights the potential of using AI systems like ChatGPT for liquidity risk mitigation. However, could you discuss any potential legal or regulatory challenges that may arise from implementing such technologies?
Certainly, Aiden. Implementing AI systems like ChatGPT can present legal and regulatory challenges. Financial institutions must ensure compliance with existing regulations, such as data privacy laws, and address potential issues like algorithmic bias or the use of customer data. Collaborating with legal and compliance experts, engaging in ongoing dialogue with regulators, and participating in industry initiatives can help navigate these challenges and establish robust legal frameworks for the responsible and ethical use of AI technologies.
Marty, your article provides valuable insights. However, how can organizations effectively manage the integration of AI technologies without disrupting existing business processes?
Thank you, Camila. Managing the integration of AI technologies while minimizing disruption to existing business processes is a critical consideration. Organizations should adopt a phased approach, starting with pilot projects that focus on specific use cases or departments. This allows for testing, learning, and fine-tuning the AI system's integration with existing processes. Effective change management, user training, and collaboration between IT and business teams are essential to ensure a smooth transition and successful integration of AI technologies into the organization.
Marty, your article brings attention to an innovative approach for mitigating liquidity risk. However, what steps can organizations take to build trust among stakeholders, particularly when implementing AI technologies that can significantly impact financial decision-making?
Building trust among stakeholders is crucial, Christopher. To do so, organizations should prioritize transparency, explainability, and accountability in their AI systems' design and implementation. Communicating the value, benefits, and risks associated with AI technologies, along with showcasing successful implementation and adherence to ethical principles, helps build confidence. Engagement with stakeholders throughout the process, addressing concerns, and actively seeking feedback fosters a collaborative environment and demonstrates the organization's commitment to responsible AI usage.
Marty, your article provides an interesting perspective on leveraging AI for liquidity risk mitigation. However, what steps can be taken to address the potential biases in AI systems and ensure fairness in decision-making?
Addressing biases in AI systems and ensuring fairness is crucial, Sophie. Organizations can take several steps to mitigate biases. These include implementing diverse and unbiased training datasets, conducting regular audits to identify and correct biases, and involving a multidisciplinary team in the AI development process. Additionally, ongoing monitoring and fine-tuning of AI systems, coupled with clear policies and guidelines, can help reduce bias and promote fairness. Continuously evaluating and refining AI models is necessary to ensure responsible and ethical decision-making.
Marty, your article sheds light on an intriguing use case for AI in mitigating liquidity risk. However, what are the potential challenges of securing the necessary resources, both in terms of talent and infrastructure, when it comes to implementing AI systems?
You raise an important point, Connor. Securing the necessary resources for implementing AI systems can be challenging. Talent acquisition and retention for AI expertise are highly competitive. Organizations should invest in attracting and developing AI talent through partnerships, training programs, and collaborations with educational institutions. Additionally, building the required infrastructure, such as high-performance computing systems and robust data storage, requires significant investments. Collaborating with technology providers and leveraging cloud services can help alleviate resource constraints and facilitate AI system implementation.
Marty, I appreciate your insights on leveraging AI for liquidity risk mitigation. However, what would be the potential implications or challenges of AI systems making real-time decisions in highly volatile market conditions?
Great question, Grace. Real-time decision-making by AI systems in highly volatile market conditions poses potential challenges. These include the need for robust risk management frameworks, adaptive models that can account for rapid changes, and continuous monitoring of AI outputs. Combining real-time AI insights with human judgment, maintaining clear risk thresholds, and implementing fail-safe mechanisms are essential to navigate such market conditions. Ensuring the agility and adaptability of AI systems is crucial for effective decision-making in highly volatile environments.
Marty, your article offers valuable insights into leveraging AI for liquidity risk mitigation. However, how can organizations strike the right balance between AI-driven automation and preserving the human touch in financial decision-making processes?
Striking the right balance between AI-driven automation and preserving the human touch is essential, Elijah. Organizations should focus on augmenting human decision-making with AI-driven insights rather than replacing humans entirely. By leveraging AI technologies like ChatGPT, professionals can benefit from real-time analysis and recommendations while retaining their critical-thinking abilities, experience, and ethical judgment. Human intervention in the decision-making process adds a layer of oversight, adaptability, and empathetic understanding, which is valuable in the complex and dynamic financial landscape.
Marty, your article highlights the potential of AI for liquidity risk mitigation. However, how can organizations ensure the security and integrity of AI models, given the potential vulnerabilities to adversarial attacks?
Securing the integrity of AI models is paramount, Stella. Organizations should adopt robust security protocols, including secure data storage, access controls, and encryption to protect AI models from adversarial attacks. Implementing AI architectures that are resistant to tampering and continuously monitoring AI system behavior for any unusual patterns or anomalies can help detect potential threats. Awareness, proactive monitoring, and regular updates to AI models are critical in maintaining their security and integrity in the face of evolving adversarial attack techniques.
Marty, your article discusses an intriguing approach for mitigating liquidity risk. However, what potential social or economic implications can arise from increased adoption of AI systems like ChatGPT in the financial industry?
You bring up an important consideration, Callum. Increased adoption of AI systems like ChatGPT can have social and economic implications. While it can lead to increased efficiency and risk mitigation, it may also impact employment dynamics within the financial industry. Workforce reskilling and upskilling programs become crucial to ensure a smooth transition and help individuals adapt to the changing job landscape. Additionally, organizations and policymakers should address potential equity concerns, ensure fair access to AI-driven services, and promote responsible AI usage to mitigate any negative societal or economic impacts.
Marty, your article provides valuable insights into leveraging AI for liquidity risk mitigation. However, what safeguards should be in place to prevent AI systems from making inaccurate or erroneous decisions?
Ensuring safeguards for accurate decision-making by AI systems is crucial, Henry. Organizations can implement validation processes, including backtesting, scenario analysis, and stress testing, to assess the accuracy and robustness of AI models. Regular monitoring of performance metrics, comparison with benchmark data, and incorporating feedback loops help identify and rectify any inaccuracies. Establishing continuous evaluation mechanisms, involving experts in validation processes, and maintaining a human-in-the-loop approach are fundamental to prevent AI systems from making erroneous decisions.
Marty, your article offers an interesting perspective on leveraging AI for liquidity risk mitigation. However, what potential risks or challenges can arise from over-reliance on AI systems?
Over-reliance on AI systems can pose several risks and challenges, William. It can lead to complacency, reduced critical thinking, and inability to adapt to unprecedented situations. Unintended biases, privacy concerns, and lack of transparency may also arise from excessive reliance on AI-driven decision-making. Organizations must strike the right balance between AI capabilities and human judgment, emphasizing the importance of human oversight, continuous monitoring, and validation of AI outputs. A holistic approach that combines the strengths of AI systems and human expertise is crucial for responsible and effective risk mitigation.
Marty, your article raises important insights on utilizing AI for liquidity risk mitigation. However, could you elaborate on the potential ethical considerations and societal impacts associated with AI systems making critical financial decisions?
Absolutely, Joshua. Ethical considerations and societal impacts are paramount when AI systems make critical financial decisions. Transparency, accountability, and fairness should guide the development and deployment of AI models. Organizations must avoid perpetuating biases, ensure equitable access to AI-driven services, and prioritize responsible AI usage. Additionally, public discourse, interdisciplinary collaborations, and involvement of diverse perspectives are instrumental in shaping policies and regulations that govern AI systems' decision-making, mitigating ethical concerns, and safeguarding societal interests.
Marty, your article offers valuable insights on leveraging AI for liquidity risk mitigation. However, what steps can organizations take to address data quality and reliability challenges associated with training AI systems?
Addressing data quality and reliability challenges is crucial, Alexandra. Organizations should adopt rigorous data management practices, including data cleaning, preprocessing, and validation techniques, to enhance data quality. Collaborating with domain experts to define relevant data variables and identifying potential data biases are essential steps. Establishing robust data governance frameworks and regular auditing of training datasets can help ensure the reliability and integrity of data used for training AI systems. A strong data foundation is fundamental to the accuracy and effectiveness of AI models in mitigating liquidity risk.
Marty, thank you for sharing your insights on leveraging AI for liquidity risk mitigation. However, how can organizations ensure that AI systems align with regulatory requirements, especially given the dynamic nature of financial regulations?
You're welcome, Sophie. Ensuring AI systems align with regulatory requirements is crucial. Organizations should establish strong governance frameworks that encompass regulatory compliance. This includes conducting regular audits to assess compliance, collaborating closely with legal and compliance teams, and actively monitoring regulatory updates. Engaging in industry initiatives and maintaining open channels of communication with regulators can help organizations stay ahead of evolving regulatory landscapes. By proactively aligning their AI systems with regulatory requirements, organizations can reinforce trust, mitigate risks, and foster responsible AI deployment.
Marty, your article provides valuable insights into the potential of leveraging AI for liquidity risk mitigation. However, what role can industry collaborations play in advancing the adoption and development of AI technologies?
Industry collaborations play a vital role, Olivia, in advancing the adoption and development of AI technologies. By sharing expertise, knowledge, and resources, organizations can collectively address challenges and drive innovation. Collaborations can facilitate data sharing, promote best practices, and establish industry standards. Additionally, partnerships between financial institutions, technology providers, and regulatory bodies can foster responsible AI deployment and evolve regulatory frameworks to keep pace with technological advancements. Industry collaborations create an ecosystem that accelerates the adoption and development of AI technologies for liquidity risk mitigation and other financial applications.
Marty, your article offers valuable insights on leveraging AI for liquidity risk mitigation. However, what considerations should organizations keep in mind regarding potential biases in the training data that AI systems rely on?
Considering potential biases in training data is essential, Emma. Organizations should ensure that training datasets are diverse, representative, and free from biases. It is important to include data points from various demographics and socioeconomic backgrounds to avoid skewing AI system outputs towards specific groups. Regularly auditing training data and leveraging techniques like adversarial testing can help identify and rectify biases. Transparency and openness in addressing biases are crucial for building trust and ensuring the responsible and fair deployment of AI systems in liquidity risk mitigation and other financial applications.
Marty, your article sheds light on an intriguing application of AI for liquidity risk mitigation. However, how can organizations effectively communicate the value and benefits of AI systems to stakeholders who may be skeptical or resistant to change?
Effectively communicating the value and benefits of AI systems to stakeholders is vital, Emily. Organizations should focus on tangible outcomes and real-world use cases that demonstrate the potential impact of AI on liquidity risk mitigation. By showcasing the value AI systems bring in terms of enhanced decision-making, risk reduction, and operational efficiency, organizations can address skepticism and build buy-in. Engaging in open dialogues, providing clear information, and offering training and support are also key in helping stakeholders better understand and appreciate the benefits of AI-driven solutions.
Marty, your article offers valuable insights into leveraging AI for liquidity risk mitigation. However, what are the potential risks associated with relying heavily on AI systems, and how can organizations mitigate them?
Risk mitigation is crucial when relying heavily on AI systems, Isaac. Organizations should implement comprehensive risk management frameworks that include regular monitoring, continuous evaluation, and validation processes. Building redundancy and fail-safe mechanisms into the AI system's design can help mitigate the impact of erroneous outputs or system failures. Employing human-in-the-loop practices, incorporating human expertise, and ensuring robust data governance further enhance risk mitigation measures. By adopting a holistic approach that combines human judgment and AI capabilities, organizations can effectively manage and mitigate the potential risks associated with heavily relying on AI systems.
Marty, your article highlights the potential role of AI in liquidity risk mitigation. However, could you discuss any potential limitations or challenges of AI systems when it comes to analyzing complex market dynamics?
Certainly, Elijah. AI systems may face limitations and challenges when analyzing complex market dynamics. Rapidly changing market conditions, unexpected events, and interconnectedness of various factors can make accurate analysis and prediction challenging. While AI technologies like ChatGPT excel in analyzing large volumes of data, they may struggle with interpreting complex patterns and nuance. Human judgment and expertise remain crucial in interpreting AI insights within the context of market dynamics. Complementary collaboration between AI systems and experienced professionals can enable better decision-making in the face of complex market dynamics.
Marty, your article provides valuable insights into leveraging AI for liquidity risk mitigation. However, how can organizations ensure that AI systems like ChatGPT remain up-to-date and adapt to changing market conditions?
Ensuring AI systems remain up-to-date and adaptable is key, Liam. Organizations should establish mechanisms for continuous monitoring and evaluation of AI system performance. This includes regular updates to training data, retraining of AI models, and incorporating feedback loops to capture evolving market conditions. Staying informed about technological advancements and research in the field of AI is also essential to adopt state-of-the-art practices. Organizations that foster a culture of learning, embrace emerging techniques, and engage in ongoing research and development are better equipped to ensure their AI systems remain robust and effective in mitigating liquidity risk.
Marty, your article raises thought-provoking points on leveraging AI for liquidity risk mitigation. However, what role can regulatory bodies play in shaping the responsible adoption and deployment of AI technologies in the financial industry?
Regulatory bodies play a crucial role, Stella, in shaping the responsible adoption and deployment of AI technologies in the financial industry. They can develop and enforce regulations that promote fairness, transparency, and accountability in AI-driven decision-making processes. Regulatory frameworks can address data privacy, security, ethical guidelines, and bias mitigation. Engaging in collaborative discussions and consultations between regulators, industry participants, and policymakers helps establish standards and best practices that strike a balance between innovation and safeguarding public interest. Regulatory bodies are pivotal in creating an environment that fosters responsible AI usage and protects stakeholders in the financial industry.
Marty, your article provides valuable insights on leveraging AI for liquidity risk mitigation. However, how can organizations build and maintain trust in AI systems, especially with increasing concerns around ethical implications?
Building and maintaining trust in AI systems is critical, Connor. Organizations can prioritize transparency by openly communicating about the limitations, benefits, and risks of AI systems. Developing explainability techniques that provide insights into the decision-making process of AI models can enhance trust. Implementing robust governance frameworks, including ethics committees and audit mechanisms, helps ensure responsible AI deployment. Organizations should also actively engage with stakeholders, address concerns, and incorporate feedback to establish trust. By fostering a culture of responsible and ethical AI usage, organizations can build and maintain trust in AI systems for liquidity risk mitigation and beyond.
Marty, your article offers valuable insights on utilizing AI for liquidity risk mitigation. However, what potential societal or economic benefits can arise from wider adoption of AI systems in the financial industry?
Wider adoption of AI systems in the financial industry can lead to several societal and economic benefits, Christopher. Enhanced risk management practices can contribute to greater financial stability. Improved decision-making, based on real-time insights from AI systems, can mitigate liquidity risk and reduce the potential impact of market downturns. Moreover, AI systems' operational efficiency and automation have the potential to reduce costs and enhance customer experiences by streamlining processes. Additionally, the development of AI technologies can drive innovation, create new job opportunities, and foster economic growth. The wider adoption of AI in the financial sector can have positive ripple effects across various aspects of the economy.
Marty, your article raises intriguing points about leveraging AI for liquidity risk mitigation. However, what steps can organizations take to ensure the responsible use of AI systems and mitigate potential unintended consequences?
Ensuring the responsible use of AI systems is crucial, Oliver. Organizations should establish clear guidelines and ethical principles for AI usage, incorporating considerations like fairness, transparency, and accountability. Implementing multidisciplinary teams that include ethicists, domain experts, and technologists can help identify potential unintended consequences and address ethical pitfalls. Regular auditing and monitoring of AI outputs, along with incorporating human oversight and validation, further mitigate unintended consequences. A proactive approach to responsible AI usage, grounded in industry standards and best practices, is key in mitigating risks and ensuring organizations derive maximum value from their AI systems.
Marty, your article sheds light on leveraging AI for liquidity risk mitigation. However, what potential challenges can organizations face in terms of data accessibility and availability when implementing AI systems?
Data accessibility and availability can pose challenges, Joshua, when implementing AI systems. Organizations need to ensure data privacy and adhere to regulatory requirements. Data silos and fragmented systems within organizations can make data integration challenging. Collaborating with data providers or technology partners, exploring public or alternative data sources, and implementing data sharing agreements can help address data accessibility. Additionally, effective data governance practices, including data quality assurance, metadata management, and data cleansing, are crucial to ensure the availability of reliable data for training and deploying AI systems.
Marty, your article presents a compelling perspective on leveraging AI for liquidity risk mitigation. However, what precautions should organizations take to ensure that AI systems can operate in real-time without compromising robust risk assessment?
Ensuring that AI systems can operate in real-time without compromising risk assessment requires careful considerations, William. Organizations should prioritize building scalable and efficient AI architectures that can handle real-time data processing and analysis. This includes leveraging technologies like distributed computing, parallel processing, and cloud infrastructure. Real-time risk assessment also necessitates establishing risk thresholds, real-time monitoring mechanisms, and developing clear protocols for immediate response and action. Organizations should thoroughly test and validate the performance of their AI systems under real-time scenarios to ensure that robust risk assessment is not compromised in the pursuit of real-time operations.
Marty, your article provides valuable insights into leveraging AI for liquidity risk mitigation. However, how can organizations strike a balance between cutting-edge AI technologies and legacy systems prevalent in the financial industry?
Striking a balance between cutting-edge AI technologies and legacy systems poses a challenge, Grace. Organizations can adopt an incremental approach, starting with targeted use cases and gradually expanding AI integration. Developing effective integration strategies that accommodate legacy systems, data formats, and protocols is crucial. Organizations should identify areas where AI technologies can complement existing systems and demonstrate tangible benefits through small-scale pilots. Collaborating with technology providers that offer compatibility solutions and adopting modular design principles can help ensure seamless integration and minimize disruption to legacy systems. Gradual adoption allows organizations to leverage AI capabilities while preserving their investments in legacy infrastructure.
Marty, your article presents an innovative approach for mitigating liquidity risk. However, what steps can organizations take to ensure the privacy and security of sensitive data when using AI systems?
Ensuring the privacy and security of sensitive data when using AI systems is paramount, Liam. Organizations should implement robust data privacy frameworks, including data anonymization, encryption, access controls, and intrusion detection systems. It is critical to adhere to relevant data protection regulations and regularly assess compliance. Prioritizing secure storage and transmission mechanisms and conducting regular security audits can help mitigate risks. Additionally, employee training on data privacy and security best practices, coupled with ongoing monitoring and governance of AI system usage, further ensures data privacy and security in the context of AI-driven liquidity risk mitigation.
Marty, your article raises intriguing points about leveraging AI for liquidity risk mitigation. However, what are the potential ethical considerations and societal impacts associated with AI systems influencing financial decision-making?
Ethical considerations and societal impacts are integral to address when AI systems influence financial decision-making, Emily. Transparency and explainability are crucial in ensuring fair outcomes and avoiding unintended biases. Organizations should actively mitigate biases, address equity concerns, and promote inclusiveness and diversity in the development and deployment of AI systems. Furthermore, engaging in public discourse, collaborating with regulatory bodies, and embracing interdisciplinary research and development help shape ethical frameworks. By fostering responsible AI usage, organizations can minimize potential societal impacts and ensure that AI systems contribute to fair, transparent, and accountable financial decision-making processes.
Marty, your article highlights the potential benefits of leveraging AI for liquidity risk mitigation. However, what role can AI play in enhancing audit and compliance processes within financial institutions?
AI can play a significant role, Connor, in enhancing audit and compliance processes within financial institutions. AI technologies can help automate compliance monitoring, identify potential anomalies or suspicious activities in real-time, and streamline auditing procedures. Natural Language Processing (NLP) algorithms can assist in analyzing large volumes of regulatory documents and identifying key compliance requirements. Machine Learning models can learn from past audit findings and assist in risk assessment. By leveraging AI in audit and compliance processes, financial institutions can enhance accuracy, efficiency, and timeliness while ensuring adherence to regulatory guidelines.
Marty, your article provides valuable insights into leveraging AI for liquidity risk mitigation. However, what potential challenges or resistance can organizations face in terms of cultural adoption and embracing AI systems?
Cultural adoption and embracing AI systems can present challenges, Alexandra. Resistance to change, fear of job displacement, and lack of familiarity or understanding about AI can hinder adoption. Organizations should prioritize change management practices, fostering a culture of learning and openness to new technologies. Providing training and upskilling opportunities to professionals equips them to navigate the evolving landscape. Transparent communication, addressing concerns, and actively involving employees in the AI deployment process help facilitate cultural adoption. By showcasing the benefits, successes, and collaborative potential of AI systems, organizations can overcome resistance and foster an environment that embraces responsible AI usage for liquidity risk mitigation and beyond.
Thank you all for taking the time to read my article on utilizing ChatGPT to mitigate liquidity risk in the digital age. I look forward to hearing your thoughts and engaging in a fruitful discussion.
Great article, Marty! I especially liked how you highlighted the potential of AI tools like ChatGPT in managing liquidity risk. It's fascinating how technology continues to revolutionize the finance industry.
Sarah, I completely agree with your observation. The potential applications of AI in the finance industry are immense, and when it comes to liquidity risk management, tools like ChatGPT can provide valuable insights and assist decision-makers.
I agree, Sarah. AI can definitely make a huge impact on managing liquidity risk. However, I wonder about the potential limitations and challenges when using ChatGPT specifically. Marty, what are your thoughts on that?
That's a valid concern, Peter. While ChatGPT is a powerful tool, it does have certain limitations. For example, it may generate responses that sound plausible but might not always be accurate. It's crucial to have proper validation and oversight in place.
Peter, you brought up an important point. While ChatGPT has its limitations, proper validation, and oversight, along with ongoing improvements in AI technology, can help address those challenges effectively.
I found the article very informative, Marty. It's interesting to see how AI can enhance decision-making in finance. Do you think there will be resistance from traditional financial institutions in adopting these technologies?
Thank you, Emily. Yes, there might be some resistance initially, especially from traditional institutions that are accustomed to more traditional approaches. However, as AI tools like ChatGPT continue to prove their effectiveness, I believe the resistance will diminish.
I have concerns regarding the potential biases in AI systems. It's crucial to ensure that the data used to train ChatGPT and similar tools is diverse and representative. Marty, could you shed some light on how this concern is being addressed?
Absolutely, Alex. Bias mitigation is a significant concern in AI systems. OpenAI is actively working towards reducing biases during the training of ChatGPT. They are investing in research and engineering to create more transparent and controllable AI.
Alex, you're absolutely right. Bias mitigation is a critical aspect of AI development. OpenAI and other organizations are actively researching ways to reduce biases and ensure fair and accountable AI systems.
Marty, your article touched on the benefits of using ChatGPT for liquidity risk management. Can you provide some real-life examples of how this AI tool has been successfully utilized?
Certainly, Ethan. One example is in the banking sector, where ChatGPT has been utilized to assess the impact of liquidity events on institutions. It has helped identify potential risks and develop strategies to mitigate them. Another example is in market surveillance, where ChatGPT aids in detecting anomalies and suspicious trading patterns.
I enjoyed reading your article, Marty. It got me thinking about the ethical implications of relying heavily on AI tools for decision-making. How do you suggest balancing the use of technology with human judgment to ensure responsible risk management?
Thank you, Sophia. Balancing technology with human judgment is crucial. AI tools like ChatGPT should be seen as aids to assist human decision-makers, rather than replace them entirely. Human oversight and critical thinking are essential for responsible risk management.
Interesting article, Marty. However, what are your thoughts on the potential risks of overreliance on AI tools? Can they create new vulnerabilities in the financial system?
Good question, Liam. Overreliance on AI tools can indeed create new vulnerabilities. It's important to have robust risk management frameworks in place and regularly evaluate the performance and limitations of AI tools. Striking the right balance between automation and human expertise is crucial.
Valid concern, Liam. Overreliance on AI tools without proper human oversight can create systemic risks. That's why it's crucial to implement comprehensive risk management frameworks that consider the limitations of AI and maintain a balance with human expertise.
Marty, I appreciate your article shedding light on the role of AI in liquidity risk management. Are there any legal or regulatory challenges that need to be addressed in implementing AI tools like ChatGPT?
Thank you, Olivia. Yes, there are legal and regulatory challenges to consider. AI technologies often raise concerns around privacy, data protection, and algorithmic transparency. Policymakers and regulators are actively working on frameworks to address these challenges.
Olivia, implementing AI tools like ChatGPT requires organizations to navigate regulatory and legal challenges related to privacy, data protection, and algorithmic transparency. Collaboration between industry and regulators can help establish frameworks to address these concerns.
I found your article thought-provoking, Marty. With the rapid advancement of AI, do you believe that traditional risk management practices will become obsolete in the future?
Thanks, Andrew. While AI can enhance risk management practices, I don't think traditional practices will become obsolete. Rather, they will evolve to incorporate AI tools and insights. The human element will always remain important in decision-making.
Andrew, while AI tools can enhance risk management practices, I don't believe traditional practices will become obsolete. Instead, they will evolve, integrating AI insights to make better-informed decisions and navigate the complexities of liquidity risk.
Marty, I appreciated your article on ChatGPT and liquidity risk. However, I'm curious about the computational resources required to implement such AI tools. Could you provide some insights into the scalability and feasibility of using ChatGPT in real-world scenarios?
Certainly, Jessica. Implementing ChatGPT does require computational resources, especially with large-scale applications. However, as technology advances, computational power becomes more accessible, and cloud computing services can make it more feasible for organizations to leverage AI tools.
Jessica, the scalability and feasibility of implementing ChatGPT depend on various factors such as data availability, computational resources, and the specific needs of the organization. However, advancements in technology are making AI tools more accessible and scalable.
Great article, Marty! It's interesting to see how AI is transforming risk management. Do you think AI tools like ChatGPT will eventually lead to fully automated decision-making processes?
Thank you, Nathan. While AI tools can automate certain aspects of decision-making, I believe that fully automated processes without human oversight would be risky. Combining AI with human judgment is crucial for more reliable and responsible decision-making.
Nathan, fully automated decision-making processes without human oversight might lead to unaccountable and potentially risky outcomes. Combining AI tools with human judgment allows for a comprehensive and reliable approach to liquidity risk management.
Marty, as AI tools like ChatGPT become more advanced, do you foresee any potential ethical dilemmas arising in liquidity risk management?
That's a valid concern, Sophie. As AI tools advance, potential ethical dilemmas can arise, such as issues around fairness, transparency, and accountability. It's essential to actively address and mitigate these dilemmas as AI technologies are adopted.
The article was a great read, Marty. It made me wonder about the potential impact of AI tools like ChatGPT on employment in the finance industry. Will it lead to job losses or more job creation?
Thank you, Daniel. While AI can automate certain tasks, it also has the potential to create new job roles and opportunities in the finance industry. Rather than replacing jobs, AI tools like ChatGPT can augment human capabilities and free up time for more strategic work.
Marty, your article offered valuable insights into liquidity risk management. Are there any specific industries or sectors that can benefit the most from implementing AI tools like ChatGPT?
I'm glad you found it valuable, Lily. The financial industry, including banking, insurance, and investment firms, stands to benefit significantly from AI tools like ChatGPT in managing liquidity risk. However, the potential applications extend to other industries where liquidity management is crucial.
Marty, I appreciate your perspective on AI and liquidity risk. Do you believe that AI tools can provide faster and more accurate liquidity risk assessments compared to traditional methods?
Absolutely, Ethan. AI tools like ChatGPT can handle large volumes of data quickly and provide more accurate risk assessments in real-time compared to traditional methods. This speed and accuracy can be a significant advantage in managing liquidity risk.
Ethan, certainly! In one case, a financial institution used ChatGPT to analyze historical liquidity data and predict potential liquidity shortfalls accurately. It allowed them to take preventive measures and ensure they had sufficient liquidity to meet obligations.
Marty, I found your article very insightful. Apart from liquidity risk, do you think AI tools like ChatGPT can be applied to other areas of risk management in the finance industry?
Thank you, Sophia. Absolutely, AI tools like ChatGPT can be applied to various areas of risk management, including credit risk, operational risk, and market risk. The ability to analyze large datasets and identify patterns can enhance risk management practices across the board.
Sophia, you're right about the ethical implications. Transparency, explainability, and responsibility should always be at the forefront when leveraging AI tools to make critical decisions involving liquidity risk.
Great article, Marty! However, I'm curious about the potential costs associated with implementing AI tools like ChatGPT. Are they financially feasible for smaller financial institutions?
Thank you, Gabriel. The costs of implementing AI tools can vary depending on the scale of application and infrastructure requirements. While there may be initial investment costs, cloud-based AI services and advancements in technology are making it more feasible for smaller institutions to adopt such tools.
Marty, your article highlighted the benefits of AI in liquidity risk management. However, what are the potential risks and challenges in adopting AI tools like ChatGPT in this context?
Good question, Emily. One of the risks is overreliance on AI without human oversight. The interpretability and explainability of AI-generated decisions can also be challenging. Additionally, ensuring data privacy and security when utilizing AI tools is of utmost importance.
Marty, I enjoyed reading your article. It's exciting to see how AI is transforming risk management. Do you believe that the adoption of AI tools will become a competitive advantage for organizations in the finance industry?
Thank you, William. Absolutely, I believe that organizations that effectively adopt AI tools like ChatGPT will have a competitive edge. The ability to make data-driven decisions quickly and accurately can provide a significant advantage in managing liquidity risk.
Marty, your article raised my curiosity about the future possibilities of AI in liquidity risk management. Do you think we will see even more advanced AI systems replacing ChatGPT in the future?
Thank you, Victoria. It's certainly possible that more advanced AI systems will emerge in the future. As AI technology evolves, we might see even more sophisticated tools complementing or surpassing the capabilities of ChatGPT. Continuous innovation is key.