Applying ChatGPT for Enhanced Anomaly Detection in C-TPAT Technology
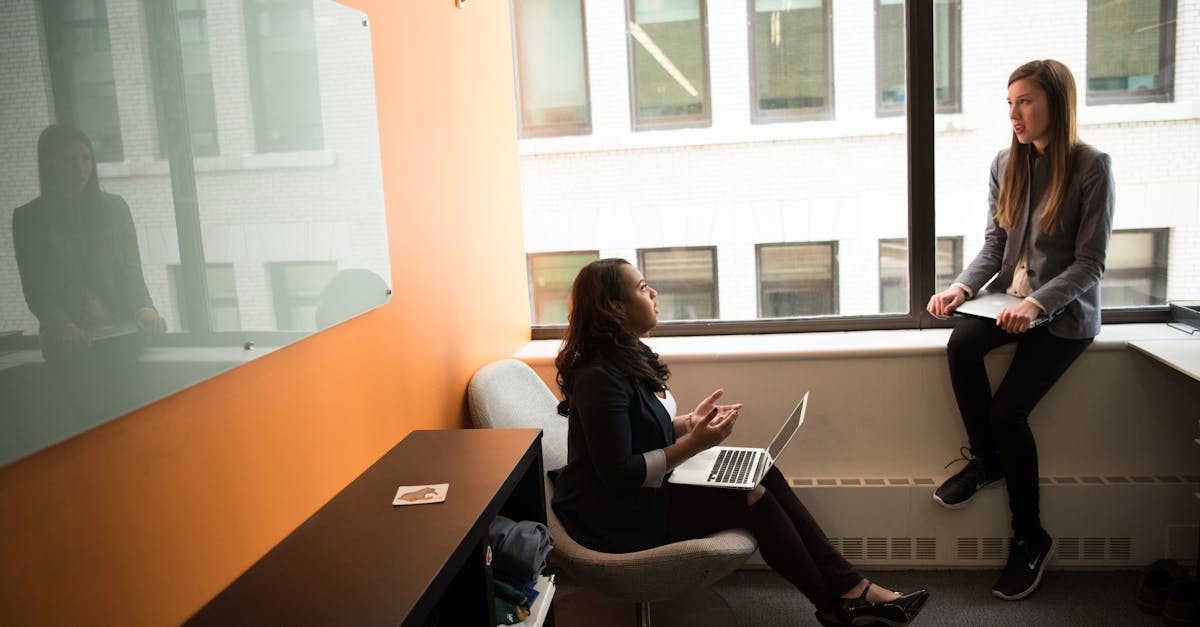
In the age of highly advanced technology, ensuring security is of utmost importance. With the emergence of artificial intelligence and natural language processing, ChatGPT-4 has proven to be a powerful tool in various domains. One such domain where ChatGPT-4 can play a significant role is in enhancing security through anomaly detection in data transactions. Combining the potential of ChatGPT-4 with the technology of C-TPAT (Customs-Trade Partnership Against Terrorism) can provide robust security measures for identifying suspicious activities and potential threats.
Introduction to C-TPAT
C-TPAT is a voluntary program led by the U.S. Customs and Border Protection (CBP) that aims to strengthen the security of international supply chains. Its primary objective is to prevent terrorism and improve overall supply chain efficiency. The program focuses on various aspects, including physical security, personnel security, procedural security, and more. By collaborating with businesses involved in global trade, C-TPAT helps implement effective security measures throughout the supply chain.
Anomaly Detection with ChatGPT-4
ChatGPT-4, developed by OpenAI, is a cutting-edge language model that can understand and generate human-like text. By leveraging its capabilities, ChatGPT-4 can be trained to identify anomalies or suspicious patterns in data transactions within the supply chain. This can be particularly valuable in detecting potential security breaches and illegal activities.
Benefits of ChatGPT-4 and C-TPAT Integration
The integration of ChatGPT-4 with the C-TPAT program offers numerous benefits in terms of security enhancement:
- Improved Risk Assessment: ChatGPT-4 can analyze vast amounts of data, including shipping manifests, customs documents, and other relevant information, to identify potential anomalies and assess associated risks. By leveraging its natural language processing capabilities, ChatGPT-4 can quickly understand complex relationships and cross-check data against known patterns of suspicious activities.
- Real-time Anomaly Detection: With its ability to process information in real-time, ChatGPT-4 can provide instant alerts when unusual or suspicious transactions occur. By flagging potential anomalies promptly, security personnel can take immediate action, preventing potential threats from materializing.
- Efficient Fraud Detection: By training ChatGPT-4 on historical data and known fraud patterns, the model can learn to recognize fraudulent activities that may otherwise go unnoticed. This can help in combating smuggling, counterfeiting, and other illicit activities, ensuring compliance with both legal and ethical standards.
- Adaptable Security Measures: As new threat vectors emerge, the integration of ChatGPT-4 with C-TPAT allows for adapting security measures quickly. By continuously training the model with updated data, it can evolve alongside emerging threats and provide effective security solutions.
Conclusion
Combining the power of ChatGPT-4 and C-TPAT can significantly enhance security in data transactions within the supply chain. By leveraging ChatGPT-4's anomaly detection capabilities, businesses can mitigate risks, prevent potential security breaches, and ensure overall compliance with security standards.
In this era of rapid technological advancements, it is crucial to utilize intelligent tools to safeguard global trade and maintain the integrity of supply chains. The integration of ChatGPT-4 and C-TPAT exemplifies how the synergy between AI and established programs can drive innovation and reinforce security measures for a safer future.
Comments:
Thank you all for reading my article on ChatGPT for Enhanced Anomaly Detection in C-TPAT Technology. I'm excited to hear your thoughts and discuss further!
Great article, Joe! I found the concept of applying ChatGPT for anomaly detection very interesting. Can you share any specific real-world examples where this approach has been successfully implemented?
Lisa, thanks for your question! One real-world example is in network security, where ChatGPT has been used to detect unusual network traffic patterns indicative of cyber attacks.
That's fascinating, Joe! Cybersecurity is indeed an area where detecting anomalies is critical. How would you handle false positives and negatives in anomaly detection using ChatGPT?
Thanks for addressing my concern, Joe. How do you fine-tune ChatGPT to minimize false positives and negatives, especially in critical applications like cybersecurity?
Lisa, to minimize false positives and negatives, fine-tuning is crucial. By training ChatGPT with carefully curated data that includes both normal and anomalous patterns, we can improve its accuracy and reduce false alarms in critical applications like cybersecurity.
Thank you for the explanation, Joe. It's reassuring to know that fine-tuning can significantly enhance ChatGPT's accuracy in critical applications like cybersecurity. Exciting possibilities!
I agree, Joe. The potential to significantly enhance accuracy in critical cybersecurity applications is promising. This could be a significant step forward in ensuring the security of digital systems.
Hi Joe, thanks for the informative article! I have a question regarding the accuracy of ChatGPT in anomaly detection. How does it compare to traditional methods like statistical analysis or rule-based systems?
Robert, great question! ChatGPT shows promise in anomaly detection, especially in cases where regular patterns might not be easily predefined. However, it's important to note that traditional methods still excel in certain scenarios where well-defined rules and statistical analysis are applicable.
Joe, thanks for the clarification! It's good to know that ChatGPT can work in tandem with traditional methods. This way, we can leverage the best of both approaches for more robust anomaly detection.
I completely agree, Joe. A hybrid approach can combine the strengths of both ChatGPT and traditional methods, leading to more reliable anomaly detection solutions.
Hey Robert, interesting question! While ChatGPT offers a more flexible approach, traditional methods still excel in situations where historical data and statistical analysis hold significant weight. ChatGPT might be more suitable for dynamic environments with evolving anomalies.
Chris, I appreciate your insights! You're right, dynamic environments may benefit from ChatGPT's flexibility. Traditional methods can establish a solid foundation, while ChatGPT can learn from new patterns and adapt to changing anomalies.
Chris, you've made a great point. Having a combination of traditional methods and ChatGPT's flexibility could be a powerful way to tackle anomaly detection challenges.
Emily, I couldn't agree more. Traditional methods provide a solid foundation, while ChatGPT's flexibility allows us to adapt to evolving anomalies and detect emerging patterns in complex environments.
Joe Halpin, kudos for writing such an insightful piece! I'm curious about the potential limitations of using ChatGPT for anomaly detection. Are there any specific scenarios in which it may not perform well?
Sara, thank you! While ChatGPT is a powerful tool, it may face challenges when dealing with complex outliers that deviate from usual patterns. Additionally, the model's performance heavily relies on the quality and diversity of training data, as well as ongoing fine-tuning.
Hi Joe, I loved your article! I can imagine how ChatGPT could be useful in detecting anomalies in financial transactions. With the increasing sophistication of fraud techniques, would ChatGPT be able to adapt and stay effective over time?
Emily, thanks for your kind words! ChatGPT has the potential to adapt by fine-tuning the model with recent data. This can help overcome evolving fraud techniques and ensure its continued effectiveness over time.
Joe, I also think that a hybrid approach could provide a comprehensive solution. We could use ChatGPT's flexibility to identify novel anomalies and then leverage traditional methods to further validate and fine-tune the results. What do you think?
Hi Joe, excellent article! I'm curious about the scalability of ChatGPT for large-scale anomaly detection tasks. Are the computational requirements manageable in real-time systems?
Daniel, scalability is an important concern. While ChatGPT can handle large-scale anomaly detection tasks, the computational requirements can be demanding. Efficient infrastructure and optimization strategies are crucial for managing real-time systems effectively.
Joe, excellent work on the article! Could you shed some light on the training process for ChatGPT when it comes to anomaly detection? How do we ensure a diverse and representative dataset?
Mark, thank you! Ensuring a diverse and representative dataset is crucial for training ChatGPT effectively. By including a variety of normal and anomalous patterns across different contexts, industries, and scenarios, we can improve its ability to detect anomalies accurately.
Joe, regarding natural language processing tasks, can ChatGPT also detect anomalies in sentiment analysis or identify unusual language patterns in customer reviews or social media?
Sarah, definitely! ChatGPT can be adapted for sentiment analysis and anomaly detection within natural language processing tasks. By identifying unusual language patterns or sentiment deviations from the norm, it can help uncover anomalies in customer reviews or social media conversations.
Joe, fascinating article! I'm curious if ChatGPT can handle dynamic anomalies that evolve over time, such as behavioral changes in user activities in an online platform?
Thank you, Joe! I can imagine that a diverse dataset would mitigate bias and improve ChatGPT's understanding of different anomalies across applications.
Joe, given the computational requirements, what are some key considerations for implementing ChatGPT in real-time systems for anomaly detection? Are there any trade-offs to be aware of?
Emily, one important consideration is optimizing the model's architecture and fine-tuning techniques to achieve a good balance between accuracy and computational efficiency. Another trade-off is the need for continuous monitoring and maintenance to keep the system performing at its best.
Joe, thanks for acknowledging the limitations. Would you recommend a hybrid approach that combines ChatGPT with traditional methods for improved anomaly detection?
Hi Joe, great article! I was wondering if ChatGPT has any advantages over traditional methods when it comes to detecting anomalies in natural language processing tasks?
William, thank you! ChatGPT can have advantages over traditional methods in natural language processing tasks due to its ability to understand context, generate human-like responses, and adapt to evolving language patterns.
Joe, agreed! ChatGPT's contextual understanding and adaptability make it an exciting tool for natural language processing tasks, especially in detecting anomalies that traditional methods might miss.
Joe, fantastic article! I'm curious if ChatGPT can handle scenarios where anomalies exhibit temporal patterns, like seasonality or periodic behaviors?
Mary, great question! ChatGPT can handle anomalies with temporal patterns by considering historical data and learning from seasonality or periodic behaviors. However, its performance will depend on the availability and quality of training data for such scenarios.
Exactly, Joe! By leveraging the strengths of both approaches, we can improve accuracy, reduce false positives, and enhance overall anomaly detection capabilities. A hybrid approach seems very promising.
Hi Joe, great article! I was wondering if ChatGPT requires a large amount of labeled data for training, or can it learn effectively with limited labeled examples?
Laura, ChatGPT benefits from a large amount of labeled data during training. However, recent research has shown that it can also learn effectively with limited labeled examples by leveraging techniques like unsupervised or semi-supervised learning.
Joe, great article! Considering the dynamic nature of anomalies, how frequently would ChatGPT need to be retrained to ensure optimum performance?
John, the frequency of retraining depends on the nature of anomalies and evolving patterns. It's a best practice to periodically retrain ChatGPT with updated data to adapt to changes and ensure optimum performance, especially in dynamic environments.
Thank you for the insight, Joe! Periodic retraining sounds reasonable to adapt to evolving anomalies. It's essential to strike a balance between keeping the model up-to-date and avoiding excessive retraining that may lead to overfitting.
John, you're absolutely right! Striking the right balance is key to ensuring the model remains effective without becoming overly sensitive to noise or losing its ability to generalize anomalies based on past patterns.
Hey Joe, interesting read! How does the detection speed of ChatGPT compare to traditional anomaly detection methods, especially in real-time systems where timely response is crucial?
David, great question! The detection speed of ChatGPT depends on various factors, including the computational resources allocated and the complexity of the anomaly detection task. Real-time systems require efficient infrastructure and optimizations to ensure timely responses.
Joe, thanks for the clarification. Ensuring timely responses is crucial in anomaly detection. ChatGPT's speed and efficiency in real-time systems will play a significant role in its practical implementation.
Thank you for the insight, Joe! It's impressive that ChatGPT can handle temporal patterns. I can see the potential for applications in various industries like finance or manufacturing.
Mary, absolutely! The applications of ChatGPT in industries like finance and manufacturing can range from detecting financial fraud to identifying deviations in manufacturing processes, leading to improved efficiency and security.
Joe, that sounds promising! Detecting anomalies in finance and manufacturing can greatly impact security and productivity. This approach can provide proactive measures to prevent financial losses and enhance product quality.