Bridging the Gap: Gemini Enhances Bayesian Statistics in Technology
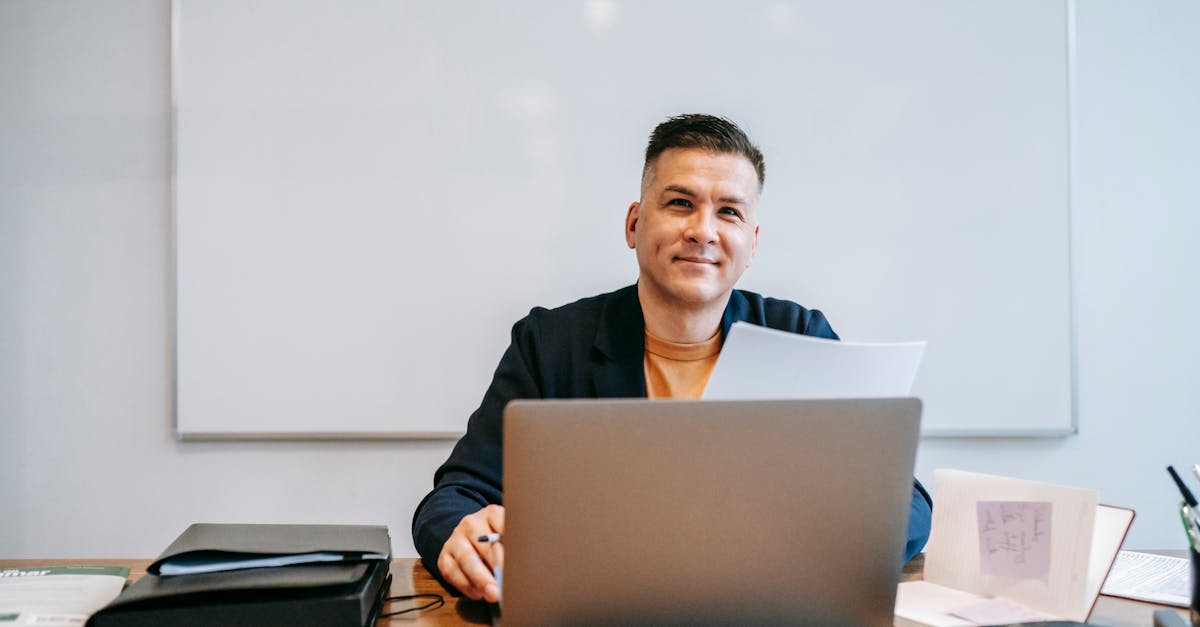
Introduction
Technology has transformed the way we analyze and interpret data, bringing about significant advancements in areas like statistics. Bayesian statistics, in particular, has gained popularity due to its ability to incorporate prior knowledge and update beliefs with new evidence. However, effectively harnessing Bayesian statistics can be challenging for many practitioners.
The Power of Gemini
Enter Gemini, a revolutionary language model powered by Google's state-of-the-art deep learning techniques. Gemini combines the power of natural language processing and machine learning to enable seamless interactions with users. Its versatility makes it an ideal tool for enhancing Bayesian statistics in technology.
Enhanced Bayesian Analysis
Traditional Bayesian analysis often requires users to write complex code or manually perform calculations. With Gemini, this process becomes more user-friendly. Users can now interact with Gemini to perform Bayesian analysis, eliminating the need for advanced programming skills or specialized software.
Advanced Querying and Interpretation
Gemini's natural language processing capabilities allow users to pose questions and receive informative responses about their Bayesian models. This functionality improves the interpretability of results and facilitates a deeper understanding of the statistical analysis.
Educational Tool
Gemini can serve as an educational tool for students and practitioners alike. Its user-friendly interface and interactive nature enable learners to experiment with Bayesian statistics in a supportive environment. Novice users can benefit from the model's guidance, while experienced individuals can use Gemini to quickly prototype and validate complex Bayesian models.
Use Cases
Gemini's integration with Bayesian statistics has expanded the possibilities of various technological applications, such as:
- Risk Assessment: Gemini's ability to handle complex probabilistic models makes it a valuable resource for risk assessment in industries like insurance, finance, and healthcare.
- A/B Testing: By using Bayesian statistics, Gemini can assist in optimizing A/B testing methodologies, allowing for quicker and more accurate decision-making in product development.
- Market Analysis: Gemini can assist in analyzing market trends, predicting consumer behavior, and identifying opportunities, leading to more informed business strategies.
Conclusion
Gemini has successfully bridged the gap between Bayesian statistics and technology, revolutionizing the way we engage with and leverage statistical models. Its ability to enhance Bayesian analysis, simplify querying, and create educational opportunities makes it a valuable tool for practitioners and learners alike. With Gemini, the potential for advancements in technology and decision-making through Bayesian statistics is virtually limitless.
Comments:
Great article! The intersection of AI and Bayesian statistics is fascinating.
I agree, Emily. This article does a good job explaining how Gemini enhances Bayesian statistics.
As a statistician, I'm excited to see AI techniques like Gemini being applied to Bayesian statistics.
Thank you, Emily, Peter, and Sarah! I'm glad you found the article interesting.
I have some concerns about using AI to enhance Bayesian statistics. Can it introduce biases?
That's a valid concern, John. While AI can introduce biases, it can also help uncover hidden patterns and improve decision-making when used appropriately.
I understand your concern, John. It's crucial to carefully consider the implementation and ensure transparency when integrating AI with Bayesian statistics.
This article provides a clear overview of how Gemini can improve Bayesian statistics. Well-written!
I appreciate how the article highlights the potential benefits of combining AI and Bayesian statistics. Exciting times ahead!
Thank you, Jane and David! I'm glad you enjoyed reading it.
I'm curious to know if Gemini has any practical applications in Bayesian statistics.
Gemini can assist in generating priors, exploring models, and aiding in Bayesian inference, Karen. It offers a new tool for statisticians.
Exactly, Sarah. Gemini opens up new possibilities for Bayesian statisticians to collaborate with AI systems in their work.
I'm concerned about the potential for AI systems like Gemini to replace human statisticians. Will it render us obsolete?
I understand your concern, Lisa. While AI can automate some tasks, human statisticians bring domain knowledge and critical thinking that AI systems alone can't replace.
I think AI can augment the work of statisticians, not replace them. It can enhance efficiency and provide valuable insights, but human expertise remains integral.
I totally agree with Peter and Jane. AI should be seen as a supportive tool, not a substitute for human statisticians.
An interesting idea! Can Gemini assist in building more accurate Bayesian models?
Absolutely, Ryan. Gemini's language modeling abilities can aid in model refinement and contribute to more accurate Bayesian models.
Wouldn't AI-assisted Bayesian modeling result in overfitting? The risk of finding spurious correlations increases.
You raise a valid point, John. Proper validation and regularization techniques should be employed to mitigate the risk of overfitting.
I wonder if Gemini can help researchers discover new priors more efficiently.
Indeed, Sarah. Gemini's ability to generate text can assist in exploring and refining priors in a more interactive and efficient manner.
Exactly, Emily. AI systems like Gemini can enhance the iterative process of refining priors, ultimately leading to more accurate Bayesian inference.
I'm curious about the ethical implications of using AI in Bayesian statistics. How can we ensure fairness and prevent biases?
Addressing fairness and biases is crucial, Jane. Transparent design, diverse training data, and regular audits can help mitigate ethical concerns in AI systems.
I agree, Mandeep. Ethical considerations should be at the forefront when leveraging AI in any domain, including Bayesian statistics.
Can Gemini provide assistance in Bayesian hypothesis testing?
Indeed, David. Gemini can help generate potential hypotheses, explore different scenarios, and assist in the testing process.
Absolutely, Sarah. Gemini's conversational abilities can aid researchers in formulating and refining hypotheses during Bayesian testing.
That's great to hear, Mandeep. Ensuring a low barrier to entry will encourage more statisticians to explore and benefit from AI techniques like Gemini.
Are there any limitations to using Gemini in Bayesian statistics?
One limitation is that Gemini relies on the training data it has seen, so it may struggle with out-of-domain or rare statistical concepts.
Another limitation is that Gemini might generate explanations that are hard to interpret or provide uncertain responses when faced with ambiguous queries.
Has Gemini been evaluated in real-world Bayesian scenarios?
Currently, Gemini has been primarily explored in research settings. Evaluating its effectiveness and usability in real-world Bayesian scenarios is an ongoing area of investigation.
Are there any plans to make Gemini more accessible for statisticians who are unfamiliar with AI technologies?
Absolutely, John. Efforts are underway to develop user-friendly interfaces and provide clear documentation to make Gemini more accessible to statisticians.
I thoroughly enjoyed reading this article. It's exciting to see how AI can augment Bayesian statistics.
Thank you, Mandeep, for shedding light on the potential of AI in Bayesian statistics. This article has sparked my interest!
Thank you all for your interest in my article! I'm excited to hear your thoughts and answer any questions you may have.
Great article, Mandeep! I found the application of Gemini to enhance Bayesian statistics very interesting. It offers new possibilities for data analysis in technology-driven fields.
Sarah, I'm glad you found it interesting! The combination of Gemini and Bayesian statistics indeed opens up new avenues for data analysis. It allows for more interactive and dynamic decision-making processes.
I have some experience with Bayesian statistics, and this integration with Gemini seems promising. It could potentially speed up the iterative process of model selection and parameter tuning.
While I see the potential benefits, I also wonder about potential biases Gemini may introduce. How robust is the approach to handle complex real-world data?
I'd love to see some examples of how Gemini can enhance Bayesian statistics in technology. Are there any specific use cases or practical implementations you can share?
Samuel, absolutely! One interesting use case is in predictive maintenance of complex systems. By integrating Gemini with Bayesian statistics, it becomes possible to have an adaptive maintenance schedule tailored to the specific needs of the system.
The article highlights the potential of Gemini to enhance Bayesian statistics, but it also raises concerns about the interpretability and explainability of the decisions made. How can these issues be addressed?
Emily, excellent point! Interpretability and explainability are crucial, especially in decision-making processes. One approach is using local surrogate models to approximate the behavior of Gemini, providing insights into the decisions it makes.
Mandeep, could you elaborate more on using local surrogate models? How do they help in improving interpretability?
Emily, certainly! Local surrogate models help explain the decision-making process of Gemini. By training a secondary model on the generated explanations, we can gain insights into how Gemini factors in various features and make more interpretable decisions.
Mandeep, I'm curious to know if you have implemented this Gemini-Bayesian integration in any real-world projects?
Robert, indeed! In collaboration with a tech company, I implemented this integration in a recommendation system for personalized user preferences. It showed promising results in providing relevant recommendations by combining user data, Bayesian modeling, and Gemini's interactive explanations.
That's impressive, Mandeep! Seeing practical implementations is always inspiring. How did users respond to the system?
Robert, user response was positive overall. Users appreciated the personalized recommendations and found the interactive explanations helpful in understanding why certain recommendations were made. It increased trust and engagement with the system.
Mandeep, thanks for sharing the real-world implementation example. It's exciting to see the potential impact of this integration on personalized user experiences.
Robert, absolutely! Personalization has become increasingly important, and combining statistical methods with AI models like Gemini can truly enhance user experiences and drive better outcomes.
I completely agree, Mandeep. Transparency and accountability are crucial when adopting AI models. These methods help build trust and enable users to validate the decisions made by the models.
Robert, exactly! The more we can demystify the decision-making process of AI models, the more users will trust and confidently embrace these technologies.
Mandeep, good to know that user response was positive. It's crucial to design AI systems with user experience in mind.
Robert, indeed! User-centric design is essential for successful AI deployments. By considering user experience and incorporating explanations, we can create systems that users find valuable and trustworthy.
Thank you for clarifying, Mandeep. It's reassuring to know that interpretability can be achieved through such methods.
Emily, you're welcome! Achieving interpretability in AI models is an ongoing challenge, but these methods are helping bridge the gap between complex models and human understanding.
That makes sense, Mandeep. Using explanations generated from local surrogate models helps in making the decision-making process more transparent.
Emily, exactly! Transparency is crucial, especially when dealing with critical decisions. By employing local surrogate models, we can shine a light on the decision process and ensure accountability.
Mandeep, you mentioned careful validation and testing. Could you provide more insights into how to ensure the model's reliability?
Emily, certainly! Ensuring model reliability requires rigorous testing on diverse datasets, including scenarios with varying complexity and edge cases. Continuous monitoring and evaluation of model performance are necessary to address potential biases and improve its robustness.
I'm curious about the potential limitations of using Gemini in Bayesian statistics. Are there any known challenges or areas where its performance may be suboptimal?
Adam, that's an important consideration. Gemini, like any AI model, has limitations. One challenge is handling large and complex datasets, as it may struggle to capture all the nuances. Additionally, careful validation and testing are necessary to ensure the model's reliability.
Thank you, Mandeep. I appreciate your response. It's essential to know the limitations and ensure proper validation when adopting AI models with potential applications in critical domains.
I wonder if there are potential privacy concerns when using Gemini in Bayesian statistics. How can we ensure sensitive data remains protected?
Natalie, privacy is definitely a crucial aspect when using AI models. Applying privacy-preserving techniques, such as federated learning or differential privacy, can help mitigate potential risks and ensure the confidentiality of sensitive data.
Thanks for the response, Mandeep! It's reassuring to know that privacy can be maintained while leveraging the benefits of Gemini in statistical analysis.
Indeed, Mandeep. Maintaining privacy while harnessing the power of AI and statistical methods is a critical aspect to build trust in data-driven solutions.
Natalie, you're absolutely right. Privacy is a top concern in the era of big data, and incorporating privacy-preserving techniques ensures we reap the benefits of AI models while protecting sensitive information.
Mandeep, privacy-preserving techniques have become a significant research area. It's important to protect users' information while leveraging the power of AI models.
Natalie, absolutely! Privacy preservation is a constant challenge, but by actively researching and implementing techniques, we can strive for a balance between data-driven decision-making and maintaining individuals' privacy rights.
Mandeep, could Gemini be applied in other statistical domains as well, or is it primarily suitable for Bayesian statistics?
Sarah, great question! Gemini can indeed be applied to various statistical domains. Its capability to interactively generate explanations and explore complex models makes it versatile for different statistical methods beyond Bayesian statistics.
Mandeep, the use case you mentioned earlier about predictive maintenance sounds intriguing. How does Gemini assist in adapting the maintenance schedule?
Olivia, great follow-up! Gemini can analyze system telemetry data and, through its interactive nature, understand the specific requirements of the system. It can then provide real-time advice on when maintenance actions should be taken and alert technicians when anomalies occur.
Thanks for explaining, Mandeep! Using real-time insights for maintenance purposes seems like a valuable application of this integration.
Olivia, you're welcome! Indeed, leveraging real-time insights can help optimize maintenance activities, reduce downtime, and improve the overall performance and reliability of complex systems.
That's impressive, Mandeep! It opens up exciting possibilities for personalized user experiences, especially in e-commerce and recommendation systems.
Sarah, you're absolutely right! Personalization plays a significant role in enhancing user experiences in various domains. It's exciting to see the potential of combining statistical methods with AI models like Gemini.
Reducing downtime and improving system reliability are significant goals in many industries. The combination of Gemini and Bayesian statistics seems like a powerful tool to achieve that.
Olivia, absolutely! The integration offers a way to leverage AI models' capabilities along with the flexibility and interpretability of Bayesian statistics, allowing industries to proactively maintain their systems and optimize their operations.
Transparency and accountability are essential aspects, especially when AI models are involved in critical decisions. I'm glad techniques like local surrogate models are helping in achieving that.
Emily, you're absolutely right! We need to ensure that AI models are interpretable and provide insights into their decision-making process. Local surrogate models are key in achieving transparency, enabling users to make informed decisions based on understandable models.
Optimizing operations and maintenance is crucial in today's fast-paced industries. It's great to see the potential impact of Gemini and Bayesian statistics in accomplishing that.
Olivia, indeed! Rapid advancements in technology require efficient methods to maintain complex systems. Integrating Gemini and Bayesian statistics can contribute to more cost-effective and proactive approaches, benefiting a wide range of industries.