Bridging the Gap: Leveraging Gemini in Technology's FPLC
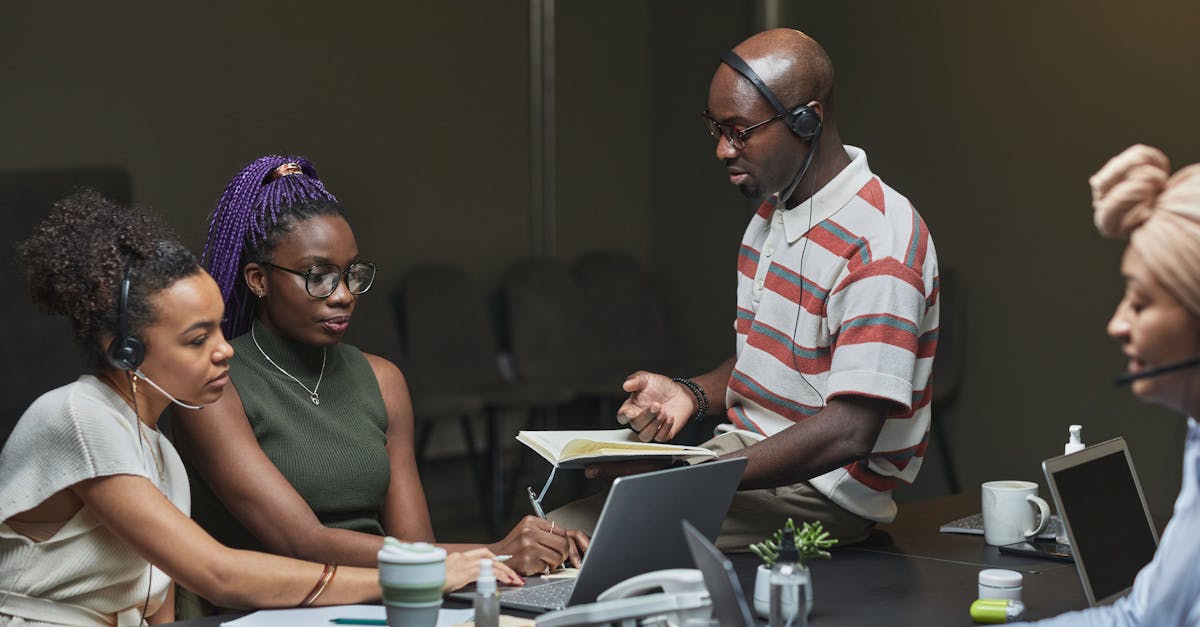
In the world of technology, advancements have always aimed at bridging the gap between humans and machines. The introduction of Gemini, an AI-powered conversational agent developed by Google, has revolutionized the field of human-computer interaction and opened up new possibilities in various areas, including the domain of FPLC (Fluid Phase Liquid Chromatography).
Technology
Gemini is powered by Google’s LLM (Large Language Model) model, which is trained on vast amounts of text data to generate human-like responses to user inputs. This cutting-edge technology leverages natural language processing and machine learning techniques to understand and generate human-like conversations.
Area of Application
The application of Gemini in FPLC can significantly enhance the efficiency and accuracy of laboratory processes. FPLC is a widely used technique for separating and purifying molecules in research and industry settings. By integrating Gemini into FPLC workflows, scientists and technicians can leverage its conversational capabilities to optimize experimental design, troubleshoot issues, and obtain valuable insights in real-time.
Usage
The versatility of Gemini in FPLC is immense. Users can interact with Gemini through various means, such as a web interface or custom-built software that leverages Google's API. With the ability to comprehend and respond to natural language commands, Gemini can assist users in setting up chromatography runs, suggesting appropriate column conditions, and even predicting separation outcomes based on specific parameters. It can also provide guidance on column selection, buffer compositions, and optimal flow rates to achieve the desired separations.
Furthermore, Gemini can aid in real-time troubleshooting by analyzing sensor data from the FPLC system. By presenting the data to Gemini, users can receive detailed explanations, recommendations, and potential solutions to rectify any issues that arise during the experiment. This eliminates the need for extensive manual analysis and provides quick insights for timely decision-making.
Conclusion
The integration of Gemini in FPLC heralds a new era in the field of liquid chromatography. By leveraging its conversational abilities, scientists and technicians can streamline their experimental processes, enhance decision-making, and gain valuable insights. As Gemini technology continues to evolve, its impact in FPLC and other scientific domains is poised to grow exponentially.
Comments:
Thank you for taking the time to read and comment on this article. I'm happy to answer any questions or provide further clarification on the topic.
Great article, Kyle! I've been using Gemini in my technology company, and it has been a game-changer for our FPLC processes. The ability to leverage AI in this way has saved us a significant amount of time and improved accuracy. Looking forward to exploring more use cases!
Emily, I'm glad to hear about the positive impact of Gemini in your company. Can you share any specific examples of how it improved your FPLC processes?
Sarah, one example is in our customer support. Gemini assists our support team by generating accurate responses to common inquiries, freeing up their time to focus on more complex issues. This has significantly reduced response times and improved customer satisfaction.
Emily, how did you initially implement Gemini in your company? Did you face any resistance from employees who may have been skeptical about utilizing AI?
Jessica, initially, we started with a pilot program where a small team of customer support representatives used Gemini alongside their usual workflow. This helped us collect real-world feedback and refine the implementation. There was some skepticism, but with proper training and support, employees embraced the technology.
Emily, integrating new technology can often pose challenges. Did you face any issues related to data compatibility or ensuring the accuracy of the responses generated by Gemini?
Emily, it's great to hear that employees eventually accepted Gemini. Did you encounter any difficulties in training the support team to effectively utilize the system?
Jessica, training the support team was indeed a process. We provided comprehensive training sessions on utilizing Gemini effectively and emphasized the importance of reviewing and validating responses generated by the system. This approach helped them gain confidence in using the technology.
Jessica, training the support team was indeed a process. We provided comprehensive training sessions on utilizing Gemini effectively and emphasized the importance of reviewing and validating responses generated by the system. This approach helped them gain confidence in using the technology.
Emily, I'm curious to know how you ensured that the responses generated by Gemini aligned with your company's brand voice and tone. Did it require extensive customization?
Sarah, ensuring alignment with our brand voice was indeed a priority. We fine-tuned the system to generate responses that matched our company's established tone and had the support team review and make any necessary adjustments. It required some customization, but the end result was worth it.
Emily, I imagine validating the generated responses plays a crucial role in maintaining accuracy. Did you establish any specific guidelines for the support team to follow during the validation process?
Sarah, indeed! Validating responses is crucial, especially in the early stages of implementation. We provided clear guidelines for the support team, such as checking for factual accuracy, ensuring the response aligns with the inquiry, and addressing any potential biases present in the generated answer.
Emily, those guidelines sound comprehensive. It's essential to address biases and ensure factual accuracy. Did you also collect user feedback to continuously improve the system's responses?
Emily, you mentioned the importance of reviewing and validating responses. Did you encounter any instances where the system generated inaccurate or inappropriate answers? How did you address such situations?
Jessica, during the pilot phase, we did come across a few instances where the system generated inaccurate or inappropriate responses. We had a feedback loop in place where the support team reported such cases, and we used that data to retrain the model and make necessary improvements. It was important to iterate and continually enhance the system's performance.
Emily, it's great to know that you had a feedback mechanism to address inaccuracies. How frequently did you retrain the model, and how did you evaluate the improvements made?
Sarah, we retrained the model every quarter to incorporate new data and address any issues identified during the feedback process. Evaluating the improvements involved conducting internal assessments, comparing system-generated responses with manually curated responses, and collecting feedback from the support team and customers.
Emily, aligning responses with the established brand voice is crucial. Can you share any tips on how to effectively fine-tune the system to reflect the desired tone?
Jennifer, one tip is to provide the model with a diverse range of training examples that match the desired tone and style. This helps it learn the nuances of the brand voice. Additionally, incorporating a validation step during the training process, where outputs are manually reviewed and refined, can also significantly contribute to aligning the system's responses with the brand's tone.
Emily, the guidelines you provided for the support team seem comprehensive. How did you handle cases where the response generated by Gemini was accurate but conveyed the information in a complex manner?
Kyle, in cases where the response was accurate yet complex, the support team was responsible for simplifying and clarifying the response without altering the meaning. The goal was to ensure that customers could easily understand the information provided.
Emily, simplifying complex responses is crucial for effective communication. Did you provide specific training or guidelines to the support team to help them handle such cases?
Sarah, absolutely. During the training sessions, we focused on providing the support team with techniques and examples to simplify complex responses while maintaining accuracy. Additionally, we had regular knowledge-sharing sessions where team members could discuss and learn from each other's experiences.
Emily, aligning responses with the established brand voice is essential. How did you ensure that the generated answers remained consistent across different support team members?
Oliver, to ensure consistency, we developed a shared document that compiled approved responses for different types of inquiries. This document served as a reference for the support team, enabling them to align their answers with the established brand voice. Continuous communication and regular updates helped maintain a consistent tone across different team members.
Kyle, great article! Could you shed some light on the cost implications of implementing Gemini in FPLC processes? Are there any significant expenses associated with this technology?
Susan, implementing Gemini does come with some expenses. While Google offers free access to the Gemini API, there may still be costs associated with training data, cloud infrastructure, and ongoing model maintenance. However, the benefits it brings in terms of time-saving and improved efficiency usually outweigh the expenses for many organizations.
Jennifer, in addition to the challenges mentioned by Kyle, another important consideration when implementing AI systems like Gemini is user acceptance. How did you manage this aspect within your organization?
Oliver, managing user acceptance was vital. We focused on transparently communicating the purpose and benefits of Gemini to employees, highlighting how it would assist rather than replace human expertise. Providing training and support to ensure employees were comfortable using the technology helped overcome any initial resistance.
Jennifer, involving employees in the pilot phase seems like a great strategy. Did you experience any specific challenges during the pilot, and how did you address them?
Jessica, during the pilot phase, one challenge we encountered was fine-tuning the system to generate responses specific to our industry. This required significant data curation efforts. We addressed it by closely collaborating with the Gemini development team, who provided guidance and support throughout the process. Their expertise helped us overcome the challenges effectively.
Oliver, user acceptance is indeed crucial. To manage this, we conducted informative sessions to educate employees about Gemini and its benefits. We also encouraged open feedback and addressed any concerns or skepticism they had. The involvement of employees in the pilot phase and their early positive experiences played a significant role in driving acceptance.
Emily, that's an excellent use case. It's impressive how Gemini can enhance customer support functions. Have there been any challenges in integrating the technology into your existing systems?
I found this article to be very informative. Gemini indeed has the potential to bridge the gap in technology's FPLC. It's impressive how AI applications continue to evolve and provide practical solutions!
David, I agree with you. AI applications like Gemini have a promising future in various industries. It's fascinating to witness the advancements in this field!
Oliver, the potential of AI applications like Gemini is immense. As long as we embrace the technology responsibly and address any concerns related to privacy and security, it can revolutionize how we operate in numerous sectors.
David, I completely agree. The responsible adoption of AI must be accompanied by thorough privacy and security measures. The potential benefits are substantial, but we must also be mindful of potential risks.
Oliver, absolutely. Responsible development and use of AI are essential to address any potential risks. As the technology progresses, it's crucial to maintain a balance between innovation and safeguards.
David, agreed. Innovations like Gemini should be accompanied by regulatory frameworks to protect user privacy and prevent misuse. Responsible development, along with constant evaluation, is crucial.
Kyle, I appreciate your insights on leveraging Gemini in FPLC. I'm curious to know if there are any limitations or challenges you have encountered while implementing this technology?
Jennifer, while Gemini has proven to be a valuable tool, there are a few challenges to consider. One of them is the need for large amounts of training data to fine-tune the model for specific use cases. Additionally, ensuring the AI system accounts for ethical considerations and biases is crucial.
Kyle, I can see how training data volume and biases are important considerations. Would you recommend any best practices for organizations looking to implement Gemini effectively?
Kyle, besides the challenges you mentioned, are there any specific industries or use cases where the integration of Gemini has shown remarkable success?
Jennifer, while Gemini has shown promise in various industries, one notable success is in the e-commerce sector. Retail companies have used it to improve customer assistance, provide personalized recommendations, and even generate product descriptions.
Kyle, the success in the e-commerce sector is fascinating! It's great to see how Gemini can enhance customer experiences and provide valuable support. Are there any other sectors you think would benefit greatly from this technology?
Jennifer, while the e-commerce sector has seen notable benefits, I believe healthcare is another industry that can greatly benefit from leveraging Gemini. It could assist in providing quick medical advice, aiding in triage, and helping patients find relevant information.
David, the potential use cases in healthcare are indeed intriguing. With precise training, Gemini could revolutionize patient interactions and contribute to more accessible healthcare services. However, what steps should be taken to address potential biases in medical advice?
Jessica, addressing biases in medical advice generated by AI systems is crucial. It requires a multi-step approach that involves thorough training data curation, diversity in data sources, and continuous monitoring of outputs. Collaboration with medical professionals and regulatory bodies helps ensure the accuracy and safety of the advice provided.
Thank you all for joining the discussion on my article, 'Bridging the Gap: Leveraging Gemini in Technology's FPLC'. I'm excited to hear your thoughts and answer any questions you may have.
Great article, Kyle! I've always been interested in the application of AI in technology. Can you share more about the specific benefits of leveraging Gemini in FPLC?
Thanks, Erica! Gemini offers several benefits in FPLC. Firstly, it enables more efficient and accurate analysis of complex data sets, optimizing performance. Additionally, Gemini enhances automation capabilities in FPLC processes, reducing manual effort and increasing overall productivity.
Kyle, I enjoyed reading your article. AI advancements like Gemini can definitely make a difference. However, do you have any concerns about potential biases in the language model?
Thank you, David! Valid point. Bias is a significant concern in AI. Google is actively working to reduce both glaring and subtle biases in Gemini. They are taking user feedback seriously to address these issues and improve the system's fairness.
Interesting article, Kyle! How do you see Gemini impacting the future of technology beyond FPLC?
Thank you, Sophie! I believe Gemini has vast potential. Apart from FPLC, it can benefit customer support, content creation, virtual assistants, and more. As AI continues to evolve, Gemini's applications may expand to various domains.
Kyle, great job on the article! Could you elaborate on the implementation challenges one might face while leveraging Gemini in FPLC?
Thank you, Mark! Implementing Gemini in FPLC can have challenges related to data privacy, training requirements, and the need for ongoing model fine-tuning. Ensuring data security and reducing dependency on vast training data are some aspects to consider.
Gemini is undoubtedly a powerful tool. However, can it completely replace human experts in FPLC?
Great question, Laura! While Gemini offers exceptional capabilities, it's important to note that it works best in collaboration with human experts. Combining AI's efficiency with human expertise leads to better decision-making in FPLC.
Kyle, excellent article! As Gemini learns from text data, how do you ensure the accuracy and reliability of information it provides in the FPLC context?
Thank you, Samuel! Ensuring accuracy is crucial. Gemini's training involves large-scale datasets, but it may still produce incorrect or nonsensical responses. Iterative feedback and continuous improvement are essential to minimize inaccuracies and enhance reliability.
Kyle, fascinating article! How can organizations start integrating Gemini into their FPLC systems?
Thank you, Rachel! Organizations can begin by identifying suitable FPLC processes where Gemini can be leveraged. They need to ensure data compatibility, establish a feedback loop, and gradually deploy the system while monitoring and refining its performance.
Kyle, insightful article! What are the potential limitations of Gemini when applied to FPLC?
Thank you, Nathan! Gemini's limitations include occasional incorrect responses, sensitivity to input phrasing, and over-reliance on training data. Generalization to unseen scenarios and fine-grained control over outputs are areas that require further development.
Kyle, do you think the use of Gemini in FPLC could lead to job displacement for human analysts?
Good question, Emily! While Gemini automates certain tasks, it also augments human analysts' abilities. It can enable analysts to focus on more complex and strategic work, reducing mundane activities—not necessarily displacing jobs.
Kyle, enjoyed reading your article! Are there any ethical considerations associated with using Gemini in FPLC?
Thank you, Mike! Ethical considerations exist. Organizations must ensure data privacy, avoid bias, and address transparency concerns. Employing safeguards and guidelines is crucial to mitigate ethical risks when adopting Gemini.
Kyle, great article! How do you see the future of AI in FPLC evolving beyond Gemini?
Thank you, Sophia! The future of AI in FPLC will likely involve more advanced models, improved domain-specific training, and increased integration with other AI technologies like computer vision and natural language understanding for a comprehensive analytical approach.
Kyle, informative article! What are the potential cost implications of integrating Gemini in FPLC systems?
Thank you, Peter! Cost implications include the computation expenses of training the models and maintaining infrastructure. Organizations must carefully consider the overall value proposition and potential efficiency gains when assessing the cost of integration.
Kyle, great insights! How do you see the adoption of Gemini in FPLC varying across industries?
Thank you, Grace! The adoption of Gemini in FPLC may vary based on industry needs, data availability, and regulatory factors. Industries that heavily rely on data-driven decision-making are more likely to embrace Gemini's potential.
Kyle, excellent article! How can Gemini improve decision-making in FPLC compared to traditional methods?
Thank you, Oliver! Gemini can improve decision-making in FPLC by quickly analyzing vast amounts of data, providing insights for accurate risk assessment, and enabling prompt identification of anomalies or patterns that human analysts might overlook.
Kyle, well-written article! Are there any challenges related to user interface design when implementing Gemini in FPLC systems?
Thank you, Emma! User interface design can be a challenge. It's crucial to create an intuitive and user-friendly interface that helps users effectively interact with Gemini, ensuring smooth integration into FPLC workflows.
Kyle, great work! Is it possible for organizations to deploy customized versions of Gemini for their FPLC systems?
Thank you, Daniel! Absolutely! Organizations have the flexibility to fine-tune and customize Gemini based on their specific FPLC requirements, domain, and data. This allows tailoring the system to deliver optimal results.
Kyle, fascinating insights! Are there any legal considerations when adopting Gemini in FPLC?
Thank you, Liam! Legal considerations include compliance with data protection and privacy regulations. Organizations must ensure Gemini's usage aligns with legal frameworks and address potential liabilities to maintain regulatory adherence.
Kyle, excellent article! Do you think Gemini can successfully handle non-English languages in FPLC?
Thank you, Gabriella! While Gemini primarily operates in English, adapting it to non-English languages is an area of active research. Expanding Gemini's language capabilities can unlock its potential for broader global FPLC adoption.
Kyle, great insights! Could you share any real-world examples where Gemini has been successfully implemented in FPLC?
Thank you, Maxwell! While I don't have concrete examples to share at the moment, organizations in finance, healthcare, and various other sectors are exploring and implementing Gemini in their FPLC systems for enhanced decision-making and automation.
Kyle, fascinating article! How can organizations ensure the ethical usage of AI models like Gemini?
Thank you, Samantha! To ensure ethical usage, organizations must establish clear guidelines, regularly evaluate the system for biases, maintain transparency with users, and encourage accountability. Ethical considerations should be an integral part of AI governance frameworks.
Kyle, thought-provoking article! How can Gemini contribute to operational efficiencies in FPLC?
Thank you, Isabella! Gemini contributes to operational efficiencies by automating repetitive tasks, enabling faster data analysis, augmenting experts' capabilities with AI assistance, and enhancing decision-making speed in FPLC workflows.
Kyle, great insights! Are there any limitations concerning the interpretability of decisions made by Gemini in FPLC?
Thank you, Jason! Interpretability is a challenge with AI models like Gemini. Understanding the reasoning behind the system's decisions can be difficult due to its complexity. Ensuring interpretability and explainability in high-stakes FPLC scenarios is an area for further exploration.
Kyle, well-researched article! How can organizations handle potential security risks associated with Gemini implementation in FPLC?
Thank you, Chloe! Organizations must enforce rigorous security measures to mitigate potential risks. Implementing secure data handling, access controls, and periodic vulnerability assessments can help safeguard systems against security threats.
Kyle, excellent job with the article! Could you share some best practices to ensure successful integration of Gemini in FPLC systems?
Thank you, Ethan! Best practices for integration include starting with well-defined objectives, piloting the system in specific FPLC use cases, establishing data quality processes, monitoring model performance, and continuously iterating to refine system behavior.
Kyle, intriguing article! How do you think the FPLC landscape will evolve with the widespread adoption of AI technologies like Gemini?
Thank you, Lily! With wider AI adoption, the FPLC landscape will evolve towards more automated, data-driven decision-making, driving efficiency, accuracy, and innovation in risk management. Organizations will likely redefine their FPLC strategies to leverage AI technologies effectively.