Enhancing Automatic Speech Recognition in Computational Linguistics with ChatGPT
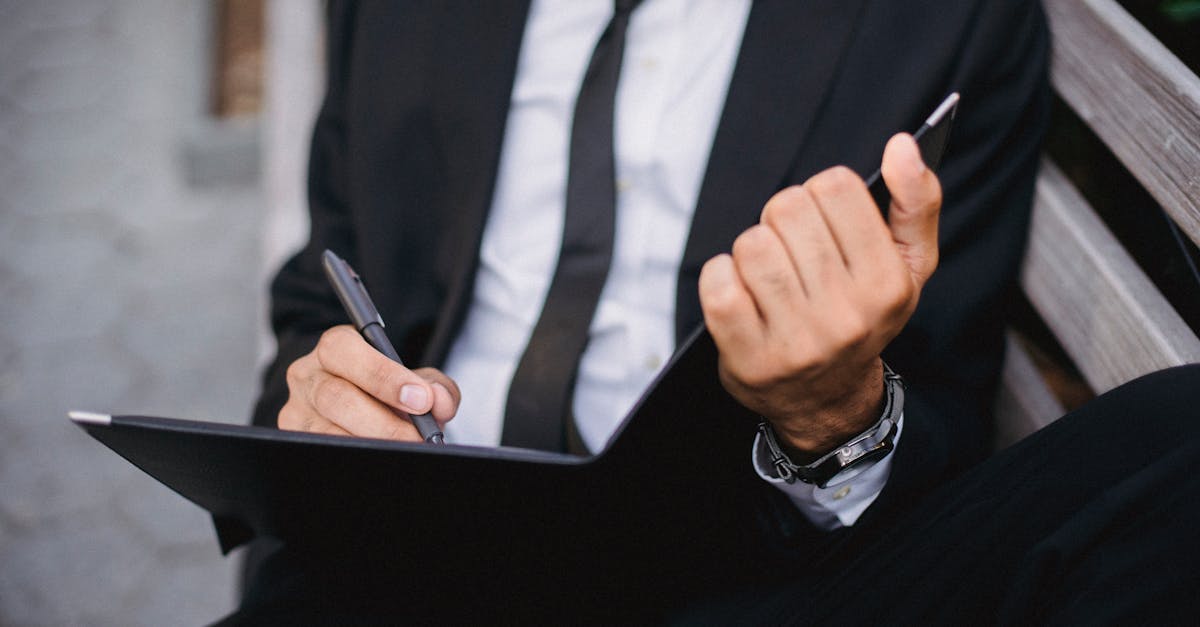
Introduction
Computational linguistics, a subfield of artificial intelligence and linguistics, focuses on developing technologies that enable machines to understand and process human language. Automatic Speech Recognition (ASR) is one of the key areas within computational linguistics that deals with converting spoken language into written text.
What is Automatic Speech Recognition?
Automatic Speech Recognition (ASR) refers to the technology that allows machines to transcribe spoken words into written text. ASR systems use algorithms and models trained on large amounts of speech data to recognize and convert spoken language into textual form. This technology has applications in various domains, including transcription services, voice assistants, voice control systems, and more.
Usage - ChatGPT-4 and Transcription Services
ChatGPT-4, an advanced language model developed by OpenAI, can be utilized for transcription services using Automatic Speech Recognition. By leveraging its capabilities in understanding and generating human-like text, ChatGPT-4 can convert speech into text with impressive accuracy. This opens up possibilities for a wide range of applications in areas such as customer support, content creation, and data analysis.
With ChatGPT-4's ASR functionality, organizations and individuals can easily transcribe audio recordings, conference calls, interviews, webinars, and other spoken content into written documents. This significantly reduces manual effort and time required for transcription, making it an invaluable tool for researchers, content creators, journalists, and many others who deal with audio content regularly.
Using ChatGPT-4 for transcription services also brings the advantage of context-awareness and language understanding. Unlike traditional ASR systems that focus solely on recognizing words, ChatGPT-4 can comprehend meaning and infer contextual information from the spoken input. This leads to more accurate and coherent transcriptions, improving overall usability and eliminating the need for extensive post-processing.
Benefits of ASR in Transcription Services
Incorporating Automatic Speech Recognition in transcription services offers several benefits:
- Increased efficiency: ASR technology accelerates the transcription process, converting speech into text at a significantly faster rate compared to manual transcription.
- Cost-effectiveness: By minimizing manual effort, ASR reduces transcription costs and enables quicker turnaround times.
- Accessibility: ASR-powered transcription services make spoken content accessible to individuals with hearing impairments or those who prefer reading over listening.
- Scalability: ASR systems can handle large volumes of audio content without compromising on accuracy, ensuring scalability for transcription services.
- Improved accuracy: Advanced ASR models like ChatGPT-4 leverage powerful language models, leading to higher transcription accuracy and fewer errors.
Conclusion
Automatic Speech Recognition is a vital technology within the field of computational linguistics. With its ability to convert spoken language into written text, ASR has revolutionized transcription services. The integration of technologies like ChatGPT-4 further enhances the accuracy and usability of ASR in various applications. Whether it's transcribing interviews, lectures, podcasts, or any other audio content, ASR-powered solutions make the process faster, more efficient, and accessible to a wider audience.
Comments:
Thank you all for your comments on my article! I appreciate your insights.
Great article, Carine! I found your discussion on enhancing ASR with ChatGPT very interesting. Have you tested its performance in noisy environments?
Thank you, Alex! Yes, we have conducted experiments in noisy conditions. ChatGPT, combined with certain noise reduction techniques, showed promising results.
This is an exciting application of ChatGPT in computational linguistics. How does it compare to traditional ASR systems in terms of accuracy?
Hi Emily! ChatGPT has shown competitive accuracy compared to traditional ASR systems. However, there is still ongoing research to further improve its performance.
I enjoyed reading your article, Carine! In your opinion, what are the main advantages of using ChatGPT for ASR?
Thank you, David! One of the main advantages is ChatGPT's ability to handle conversational context, which can be beneficial in ASR tasks involving speech recognition in dialogue scenarios.
Interesting article, Carine! Do you think ChatGPT could be employed in other areas of computational linguistics beyond ASR?
Absolutely, Sophia! ChatGPT has a wide range of potential applications in computational linguistics, including natural language understanding, sentiment analysis, and machine translation.
Great work, Carine! Have there been any challenges in fine-tuning ChatGPT for ASR purposes?
Thank you, Jacob! Fine-tuning ChatGPT for ASR has its challenges, particularly in capturing acoustic features effectively. However, by leveraging techniques such as transfer learning, we can overcome some of these challenges.
Very informative article, Carine! How do you see the future of ASR with the integration of models like ChatGPT?
Thanks, Ella! The integration of models like ChatGPT holds great potential for the future of ASR. It can lead to improved accuracy, better contextual understanding, and more seamless interaction in various speech recognition applications.
Carine, have you considered the potential ethical implications of using ChatGPT in ASR?
Hi Nathan! Ethical implications are indeed crucial. While ChatGPT shows promise, it's important to address biases, maintain privacy, and ensure accountability when deploying it in ASR and other applications.
Fascinating research, Carine! How would you recommend incorporating ChatGPT into existing ASR systems?
Thank you, Oliver! Incorporating ChatGPT into existing ASR systems can involve leveraging its capabilities for enhanced contextual understanding or using it as a post-processing module to improve transcription quality.
Impressive work, Carine! What are the potential limitations of using ChatGPT in ASR tasks?
Thank you, Lily! Some potential limitations include the model's sensitivity to input variations, computational complexity, and the need for substantial computational resources during training and inference.
Great article, Carine! How do you foresee the integration of ChatGPT with ASR impacting the accessibility of speech recognition technologies?
Thanks, Emma! Integrating ChatGPT with ASR has the potential to improve accessibility by enabling more accurate transcription services, voice assistants, and inclusive communication tools for individuals with different speech patterns or disabilities.
Carine, what are the primary datasets used for training ChatGPT for ASR?
Hi Samuel! The training of ChatGPT for ASR involves a combination of publicly available ASR datasets, large-scale conversational data, and domain-specific corpora to ensure it performs well across different contexts.
Informative article, Carine! Could you shed some light on the potential applications of ASR systems enhanced with ChatGPT outside of computational linguistics?
Certainly, William! ASR systems enhanced with ChatGPT can find applications in transcription services, voice-controlled devices, virtual assistants, language tutoring, and many other areas where accurate and contextual speech recognition is essential.
This is groundbreaking research, Carine! Are there plans to make ChatGPT for ASR publicly available?
Thank you, Ethan! While the specific availability plans are currently being discussed, the goal is to make ChatGPT for ASR accessible to the research community and promote further development and innovation.
Interesting insights, Carine! Have you evaluated the impact of using ChatGPT on the latency of ASR systems?
Thanks, Mia! Indeed, the impact on latency is a concern. While ChatGPT introduces additional computational requirements, efforts are being made to optimize it for faster processing without compromising its accuracy.
Fascinating work, Carine! How would you suggest mitigating the potential bias in ChatGPT when used in ASR applications?
Thank you, Sebastian! Mitigating bias requires careful dataset curation, diverse training sources, and ongoing monitoring of the model's performance. It's a crucial aspect to address to ensure fairness and inclusivity in ASR applications.
Impressive research, Carine! How do you handle out-of-vocabulary (OOV) words or rare language phenomena in ChatGPT for ASR?
Thanks, Grace! Handling OOV words and rare phenomena involves leveraging techniques like subword tokenization, incorporating pronunciation dictionaries, and incorporating external resources to capture a broader vocabulary and improve speech recognition performance.
Carine, what were some unexpected challenges you encountered while developing ChatGPT for ASR?
Hi Julian! One of the unexpected challenges was balancing context-awareness and speed during inference. Efficiently capturing context while maintaining real-time performance required careful optimization and architectural considerations.
Intriguing work, Carine! How do you handle disfluencies, hesitations, and other non-ideal speech characteristics in ChatGPT for ASR?
Thank you, Daniel! Addressing disfluencies and non-ideal speech characteristics involves incorporating techniques like language modeling, joint pronunciation and language modeling, and leveraging large-scale conversational data to capture and process such variations.
Well-written article, Carine! Are there plans to integrate ChatGPT with popular ASR frameworks like Kaldi or DeepSpeech?
Thanks, Sophie! While not currently integrated, efforts are being made to enable interoperability between ChatGPT and popular ASR frameworks. This would allow researchers and practitioners to benefit from the combined advancements.
Carine, what are some potential use cases where ChatGPT for ASR could outperform traditional ASR systems?
Hi Robert! ChatGPT for ASR has the potential to excel in complex dialogue scenarios, mixed language conversations, or instances where contextual understanding is critical for accurate speech recognition.
Great job, Carine! Do you anticipate any challenges in deploying ChatGPT for ASR in resource-constrained environments?
Thank you, Hannah! Deploying ChatGPT for ASR in resource-constrained environments may pose challenges due to computational demands. However, ongoing research aims to optimize the model and explore lightweight architectures to address such limitations.
Very insightful article, Carine! How does ChatGPT handle multiple speakers and speaker diarization in ASR tasks?
Thanks, Max! Speaker diarization in ChatGPT for ASR involves pre-processing techniques for speaker segmentation, speaker embeddings, or utilizing external speaker labels. Incorporating these methods enables the model to better understand and differentiate multiple speakers in transcription.
Congratulations on your research, Carine! Have you conducted user studies to evaluate the perceived quality of transcriptions using ChatGPT?
Thank you, Harper! User studies evaluating perceived quality are an important aspect of the research. These studies help assess readability, contextual understanding, and overall user satisfaction when using transcriptions generated by ChatGPT for ASR.
Informative article, Carine! Could you shed some light on the training process of ChatGPT for ASR?
Certainly, Isaac! Training ChatGPT for ASR involves a combination of pre-training on large-scale text data, followed by fine-tuning on ASR-specific datasets using techniques like teacher forcing and masked language modeling to build the model's speech recognition capabilities.
This is a significant advancement, Carine! How do you ensure the reliability of ChatGPT when used in ASR applications?
Thank you, Aileen! Ensuring reliability involves continuous evaluation, debugging, and validation processes. By actively monitoring model performance, handling edge cases, and incorporating user feedback, we strive to improve the robustness and reliability of ChatGPT in ASR applications.