Enhancing Cluster Monitoring with ChatGPT: Revolutionizing Nagios Technology
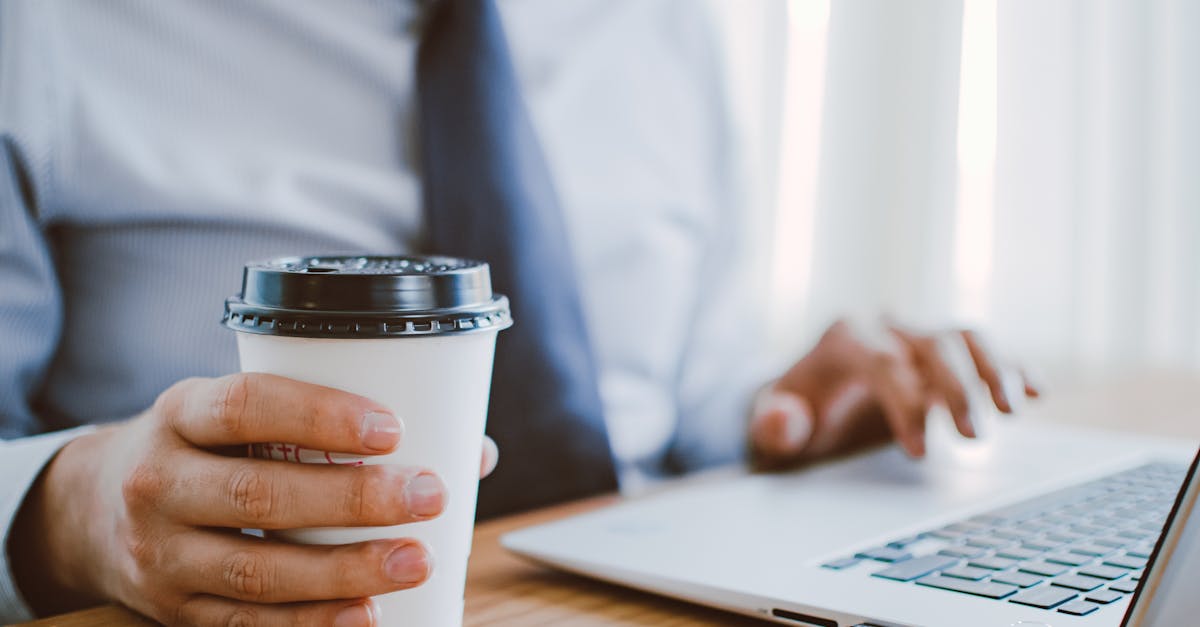
Nagios, an open-source monitoring solution, provides a comprehensive set of tools for monitoring and managing various aspects of an IT infrastructure. One of its key functionalities is cluster monitoring, which allows administrators to monitor the health and performance of clusters in a network environment. In this article, we will explore how to set up and manage cluster monitoring using Nagios.
Getting Started
Before diving into cluster monitoring with Nagios, it is important to have a basic understanding of Nagios itself. Nagios is designed to monitor hosts, services, and network devices, enabling administrators to quickly identify and resolve any issues that may arise. It uses a combination of active and passive checks to collect data and alerts administrators if any predefined thresholds are exceeded.
Setting up Cluster Monitoring
To begin setting up cluster monitoring with Nagios, the first step is to install and configure Nagios on a dedicated monitoring server. This server will act as the central hub for collecting and processing monitoring data. Once Nagios is up and running, the next step is to define the clusters that need to be monitored.
To define a cluster in Nagios, you need to create a host definition for each member of the cluster. This will include the necessary configuration parameters such as the host name, IP address, and monitoring checks to be performed. Additionally, you need to create a service group to group the cluster members together and define the checks that should be executed on the cluster as a whole.
Managing Cluster Monitoring
Once cluster monitoring is set up, managing it becomes crucial for ensuring the stability and performance of the cluster. Nagios provides various features and tools to aid in managing cluster monitoring effectively.
One important aspect of cluster monitoring is defining thresholds and notifications. Nagios allows administrators to set up threshold values for various metrics, such as CPU usage, memory utilization, and network latency. When these thresholds are exceeded, Nagios can send out notifications to the appropriate individuals or groups, alerting them of the issue.
Another useful feature in Nagios is the ability to visualize monitoring data in the form of reports and dashboards. With Nagios Core, you can generate reports that provide insights into the health and performance of the clusters over time. These reports can be used for trending analysis, capacity planning, and troubleshooting.
To further enhance the management of cluster monitoring, Nagios offers integration with other tools and services. For example, you can integrate Nagios with a ticketing system to automatically generate tickets when an issue is detected. This streamlines the incident management process and ensures that issues are addressed promptly.
Additionally, Nagios provides extensive support for plugins, allowing administrators to extend its functionality. There are numerous community-developed plugins available that can be used to monitor specific aspects of cluster performance, such as load balancing, failover, and resource allocation.
Conclusion
Cluster monitoring is a critical aspect of maintaining the stability and performance of cluster environments. Nagios offers a powerful and flexible solution for setting up and managing cluster monitoring. By following the steps outlined in this article, administrators can leverage Nagios to effectively monitor their clusters, set thresholds, and receive timely notifications, thereby ensuring the smooth operation of the cluster environment.
Comments:
Thank you all for reading my article on enhancing cluster monitoring with ChatGPT! I'm thrilled to be here to answer any questions or discuss further insights with you.
Great article, Cornelia! The idea of leveraging ChatGPT to enhance Nagios technology is quite fascinating. Are there any limitations to using ChatGPT that we need to be aware of?
Thank you, Thomas! While ChatGPT has revolutionized the way we interact with monitoring tools like Nagios, it does have some limitations. The model can sometimes generate incorrect or nonsensical responses, especially when faced with ambiguous input. It's important to provide clear instructions and validate responses when using ChatGPT in critical monitoring scenarios.
I agree, Thomas. The potential of ChatGPT in the monitoring field seems promising. Cornelia, can you share some use cases where ChatGPT has shown significant improvements in cluster monitoring?
Sure, Emily! ChatGPT has shown significant improvements in cluster monitoring in various use cases. For example, it can help automate the analysis of monitoring alerts, provide real-time insights into cluster health, and even assist in troubleshooting by suggesting potential solutions based on historical patterns. It takes monitoring to a whole new level by incorporating natural language understanding and contextual recommendations.
Excellent article, Cornelia! I've been using Nagios for a while now, and I'm curious to know how ChatGPT can help in detecting anomalies or predicting potential cluster issues.
Thank you, Michael! ChatGPT can indeed enhance anomaly detection and prediction in cluster monitoring. By analyzing historical data, it can identify patterns that may indicate abnormalities or potential issues. Additionally, by leveraging its language capabilities, ChatGPT can help interpret complex log files or error messages, reducing the time required for issue resolution. It's a powerful tool when combined with Nagios for proactive monitoring.
I'm also interested in the limitations, Cornelia. How does ChatGPT handle scenarios where the input is incomplete or lacks context?
Good question, Julia! When the input is incomplete or lacks context, ChatGPT may generate vague or incorrect responses. It's crucial to provide relevant context and clarify any ambiguity to improve the accuracy of its outputs. However, in cases where the context is missing or insufficient, it's always recommended to validate the model's suggestions with other monitoring techniques or domain experts.
Cornelia, can you elaborate on how ChatGPT assists in troubleshooting by suggesting potential solutions? That sounds incredibly useful!
Absolutely, Oliver! In troubleshooting, ChatGPT can leverage its understanding of historical patterns and knowledge of potential solutions to suggest actions or steps that might resolve the issue at hand. It can analyze the current problem, cross-reference it with similar past incidents, and offer recommendations based on successful resolutions. It saves time for administrators and provides valuable insights to prevent recurring issues.
Hi Cornelia, as someone who works with Nagios daily, I'm curious about the implementation of ChatGPT. Do we need to integrate it directly into Nagios, or can it work as a standalone system?
Good question, Sophia! ChatGPT can work both ways. It can be integrated directly into Nagios, providing an interactive chat-based interface to monitor and manage the cluster. Alternatively, it can also be deployed as a standalone system that interacts with Nagios via APIs or other communication methods. The choice depends on specific requirements, infrastructure, and the desired level of integration with Nagios.
Cornelia, can ChatGPT handle unstructured log files effectively? In complex environments, log analysis is critical for cluster monitoring.
Indeed, Lucas! ChatGPT can effectively handle unstructured log files. It can understand and parse log data, making it easier to identify anomalies, errors, or patterns of concern. Its natural language processing capabilities enable it to extract relevant information from log files, correlate events, and provide insights into potential cluster issues. It's a valuable addition to the toolkit for comprehensive log analysis and monitoring.
Cornelia, how does ChatGPT handle the dynamic nature of cluster environments, where new issues or configuration changes are frequent?
Good question, Daniel! ChatGPT is adaptable to the dynamic nature of cluster environments. By continuously training and fine-tuning the model with up-to-date data, it learns from new issues and configuration changes. This agility helps ChatGPT stay relevant and effective in providing insights and recommendations, even as the cluster evolves. It's essential to have a continuous training pipeline to ensure optimal performance in such environments.
Hi Cornelia, can you provide some examples of how ChatGPT enhances the user experience compared to traditional Nagios interfaces?
Certainly, Aria! ChatGPT enhances the user experience in various ways compared to traditional Nagios interfaces. It offers a more conversational interaction, eliminating the need for complex commands or searches. Users can describe their requirements in natural language and receive contextual recommendations or solutions. It also provides a more intuitive approach by reducing the learning curve and making monitoring more accessible to users with different levels of expertise.
Cornelia, does ChatGPT support multilingual capabilities? Monitoring systems often span across diverse regions.
Great point, Blake! ChatGPT does support multilingual capabilities. It can be trained on data in multiple languages, enabling it to communicate and understand monitoring needs in diverse regions. This flexibility helps ensure effective monitoring across language barriers and enhances collaboration, especially in distributed environments. Multilingual support is a valuable feature when dealing with global deployments and diverse teams.
That's impressive! Can ChatGPT handle technical jargon and industry-specific terminology effectively?
Absolutely, Isabella! ChatGPT can handle technical jargon and industry-specific terminology effectively. It can be trained on domain-specific data to understand and interpret specialized terms used in monitoring. This capability ensures accurate communication and reduces the chance of misinterpretation when dealing with technical concepts. Training the model with relevant data helps align it with the specific language and jargon used in the industry.
Cornelia, how does ChatGPT handle the security aspect while interacting with Nagios?
Good question, Steven! Security is of utmost importance when interacting with Nagios through ChatGPT. It's crucial to follow best practices and implement secure communication channels, such as encrypted APIs or secure protocols, to ensure the confidentiality and integrity of monitoring data and system interactions. Implementing proper authentication and access controls is also vital to prevent unauthorized access to sensitive information.
How often does ChatGPT require retraining or fine-tuning to maintain its accuracy?
Hi Emma! The frequency of retraining or fine-tuning ChatGPT depends on several factors, such as the rate of change in the cluster environment, the amount of new data available, and the model's ongoing performance. It's good practice to have regular training cycles, ensuring that the model adapts to the evolving nature of the cluster and maintains its accuracy. Continuous evaluation and retraining help optimize its performance over time.
Cornelia, how can we ensure data privacy when training ChatGPT for cluster monitoring?
Ensuring data privacy when training ChatGPT is crucial, Emma! It's advised to anonymize or sanitize any sensitive or personally identifiable information (PII) from the training dataset. Additionally, employing privacy-preserving techniques such as differential privacy or federated learning can help protect the data used during training. By following privacy best practices, you can maintain compliance with data privacy regulations and ensure the confidentiality of the monitored cluster information.
Cornelia, what potential challenges might arise when managing the training data for ChatGPT in a constantly evolving cluster environment?
Good question, David! Managing the training data for ChatGPT in a constantly evolving cluster environment can be challenging. One potential challenge is maintaining a diverse and representative dataset that captures the evolving nature of the cluster, ensuring that the model doesn't become biased or outdated. Another challenge is efficiently managing the storage and retrieval of large-scale training data. Implementing proper data versioning and backup mechanisms is essential to tackle these challenges.
Are there any specific challenges when scaling ChatGPT for larger clusters with a high volume of monitoring data?
Great question, Nathan! Scaling ChatGPT for larger clusters with high monitoring data volumes can pose a challenge. As the data increases, the model's training needs grow, requiring more computational resources. To deal with this, distributed training frameworks and infrastructure can be employed. These enable parallel training on multiple machines, allowing faster and efficient training on large datasets. Proper infrastructure planning ensures the scalability of ChatGPT in such scenarios.
Cornelia, are there any best practices to follow when integrating ChatGPT with Nagios?
Certainly, Lily! When integrating ChatGPT with Nagios, it's essential to ensure a seamless experience and optimal outcomes. Some best practices include thorough testing of the integration before deployment, validating ChatGPT's responses with monitoring experts, implementing fail-safe mechanisms if ChatGPT encounters any critical errors, and providing clear instructions to users on how to interact effectively with the system. Regular maintenance and updates are crucial too.
Regarding security, how does ChatGPT handle authentication and access controls to prevent unauthorized actions?
Good question, Sarah! To handle authentication and access controls, ChatGPT can integrate with existing authentication systems or implement its own authentication mechanism. Users interacting with ChatGPT for Nagios should have appropriate credentials and access permissions assigned to their accounts. Access control lists, role-based access control, or any relevant security framework can be employed to enforce authorized actions and prevent unauthorized access or modifications.
Cornelia, can you shed some light on the potential risks associated with utilizing ChatGPT for sensitive monitoring tasks?
Certainly, Ethan! When utilizing ChatGPT for sensitive monitoring tasks, there are some potential risks to consider. As with any AI model, there's a chance of generating inaccurate or misleading responses that could impact critical decision-making. Unauthorized access to the system or its components can also lead to security breaches. It's essential to have proper validation mechanisms and backup monitoring processes in place to mitigate these risks and maintain system resilience.
Hi Cornelia, can you provide insights on the performance impact of introducing ChatGPT to the monitoring ecosystem?
Of course, Gabriel! Introducing ChatGPT to the monitoring ecosystem can have a performance impact, especially during the initial integration phase. The computational resources required for training, fine-tuning, and inference can affect the monitoring system's overall response time. However, with proper resource allocation, optimization, and parallelization techniques, this impact can be minimized. It's crucial to analyze and benchmark the system's performance before and after introducing ChatGPT to identify any bottlenecks or required optimizations.
Would you recommend any specific monitoring or logging tools to complement the implementation of ChatGPT for Nagios?
Certainly, Sophie! There are several monitoring and logging tools that can complement the implementation of ChatGPT for Nagios. Tools like Elasticsearch, Logstash, and Kibana (ELK stack) can provide comprehensive log analysis and visualization capabilities. Prometheus and Grafana offer powerful monitoring and alerting solutions. It's important to choose tools that align with your monitoring needs, infrastructure, and the level of data analysis required to gain actionable insights.
Cornelia, what are your thoughts on incorporating machine learning techniques in an MLops pipeline for monitoring?
Great question, Matthew! Incorporating machine learning techniques in an MLops (Machine Learning Operations) pipeline for monitoring can be highly beneficial. By employing MLops practices, you can automate the model training and deployment process, monitor the model's performance, and ensure continuous improvement. This enables you to adapt the monitoring system to evolving cluster dynamics, effectively leverage ChatGPT, and enhance overall cluster management and stability.
Do you have any recommendations on how to measure the effectiveness and accuracy of ChatGPT in the context of cluster monitoring?
Certainly, James! Measuring the effectiveness and accuracy of ChatGPT in cluster monitoring involves multiple steps. It's advisable to define evaluation metrics, such as response accuracy, resolution success rate, or user satisfaction. Conducting user studies and gathering feedback can provide valuable insights. Comparing ChatGPT's recommendations with expert opinions or using historical incident data for validation can also help measure its effectiveness. Regular monitoring and benchmarking assist in identifying areas for improvement.
What kind of training data is required to achieve high-performance results with ChatGPT?
Good question, Mia! To achieve high-performance results with ChatGPT, you need a diverse and high-quality training dataset. It should ideally contain examples of various monitoring scenarios, alerts, issues, and resolutions. Incorporating both positive and negative examples helps train the model to distinguish correct recommendations from incorrect ones. Iterative improvement based on user feedback and continuous data collection is also important to fine-tune the model and maintain its accuracy over time.
Cornelia, can you elaborate on the potential bias or limitations that might arise in ChatGPT's recommendations?
Certainly, Olivia! ChatGPT's recommendations can be subject to biases and limitations. The model's responses are based on its training data, and if the training dataset is not diverse, balanced, or representative of all possible scenarios, the recommendations may be biased or incomplete. Additionally, ChatGPT can sometimes provide overly confident but incorrect responses. Continuous monitoring, user feedback, and addressing biases in the training dataset help mitigate these limitations and enhance reliability.