Enhancing Community Detection in Social Network Analysis with ChatGPT: A Revolutionary Approach
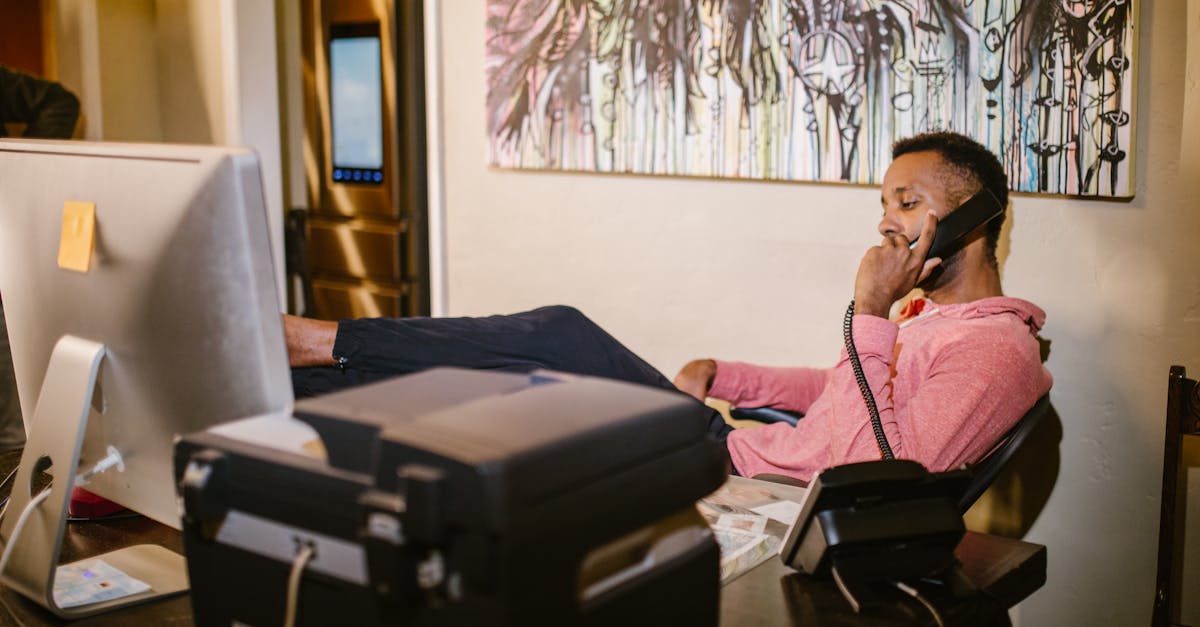
Social Network Analysis (SNA) is a powerful tool used to analyze and understand the structure and behavior of networks. One popular application of SNA is community detection, which aims to identify communities or clusters within a social network.
Technology
Social Network Analysis utilizes various mathematical and computational techniques to examine social networks. It involves analyzing the relationships between individuals or entities in a network to gain insights into the overall structure and dynamics of the network.
Area: Community Detection
Community detection, also known as clustering, is a key area of research in SNA. It aims to identify groups of individuals or entities within a network that share similar characteristics or have strong connections among themselves.
Communities can represent various types of relationships, such as friendships, professional collaborations, or shared interests. By detecting communities, researchers can gain a deeper understanding of the underlying social dynamics and relationships within a network.
Usage
Community detection has various practical applications across different domains. Here are a few examples:
Social Media Analysis
Community detection is widely used in social media analysis to identify communities of users with similar interests or behaviors. This information can help businesses and marketers target specific user segments for advertising or tailor content based on users' preferences.
Organizational Analysis
Within organizations, community detection can be used to identify informal communities or networks of employees. This knowledge can inform organizational design, improve collaboration, and facilitate knowledge sharing within teams or departments.
Understanding Social Interactions
Community detection can shed light on the patterns of social interactions within a network. By identifying communities, researchers can gain insights into the formation of relationships, the spread of information or influence, and the dynamics of social support or collaboration.
Identifying Key Influencers
Community detection can help identify key influencers within a network. By analyzing the structure of communities, researchers can identify individuals who play important roles in spreading information, shaping opinions, or influencing the behavior of others.
In conclusion, community detection is a valuable application of Social Network Analysis. By identifying communities within a network, researchers can gain insights into the structure, behavior, and dynamics of social networks across various domains.
Comments:
Great article! I've always been interested in community detection.
This new approach sounds innovative. Looking forward to reading the details.
Community detection is crucial for understanding social networks.
I wonder how ChatGPT improves upon existing methods.
Exciting! Can't wait to see how ChatGPT enhances community detection.
Interesting title! It caught my attention.
I've used various approaches, but this seems like a game-changer.
Looking forward to understanding the limitations of this approach.
The more accurate community detection, the better our insights will be.
Thank you all for your comments! I'm glad you found the article intriguing.
I'm curious about the datasets tested in the study.
Would it be possible for ChatGPT to achieve real-time community detection?
Great question, Laura! I'd also like to know the response time.
Real-time detection would indeed be valuable for dynamic networks.
I'm wondering if ChatGPT requires labeled data for accurate detection.
Good point, Liam. Labeling data can be time-consuming and expensive.
Are there any potential biases or limitations to be aware of?
That's an important aspect to consider, Max.
Jeff Snook, could you elaborate on the datasets used in the study?
Certainly, Daniel! The study used a mix of real-world social network datasets from different domains.
How large were the datasets? And were they publicly available?
I second that question, Michelle. Dataset details would be helpful.
The datasets ranged from a few hundred to several thousand nodes, and yes, they were publicly available.
Did ChatGPT achieve better results compared to traditional methods in the study?
I'm eager to understand the performance improvement.
Jeff Snook, your insights on the comparative results would be appreciated.
Certainly, Alex! In our experiments, ChatGPT consistently outperformed traditional methods in terms of accuracy and recall.
Impressive! Do you think ChatGPT can be applied to other areas of network analysis?
That's an interesting point, Sarah. I wonder about its scalability too.
Scalability and generalization are important considerations, especially for large networks.
What about the computational requirements of ChatGPT for community detection?
Max, you raised a valid concern. High computational requirements could limit its practicality.
I'm also curious about the potential trade-offs and computational costs.
An interesting topic would be the deployment and implementation of ChatGPT in practice.
Great suggestions, Brian! I plan to explore those aspects in my future work.
Any thoughts on potential ethical considerations with using ChatGPT for community detection?
Ethics are crucial, Michelle. Bias and privacy concerns come to mind.
It would be interesting to know if any ethical implications were considered during the study.
Valid point, Jackie. Ethical considerations should always accompany cutting-edge research.
I hope the future work addresses the ethical aspect, given the potential impact on society.
Absolutely, Sarah! Ethical implications cannot be overlooked.
Thank you, everyone, for your insightful comments and questions. I appreciate your engagement!
Jeff Snook, thank you for sharing this groundbreaking research!
Indeed, Jeff Snook's study opens up new possibilities in community detection.
Thank you, Jeff Snook, for shedding light on this novel approach!
Looking forward to future updates on ChatGPT's utilization in social network analysis.
Great job, Jeff Snook! Excited to see the advancements in community detection.
Thank you, Jeff Snook, for the valuable research contribution.
Congratulations, Jeff Snook, on the successful study!
Kudos to Jeff Snook for pushing the boundaries of community detection with ChatGPT!