Enhancing Data Analysis Efficiency: Leveraging ChatGPT in B2B2C Technology
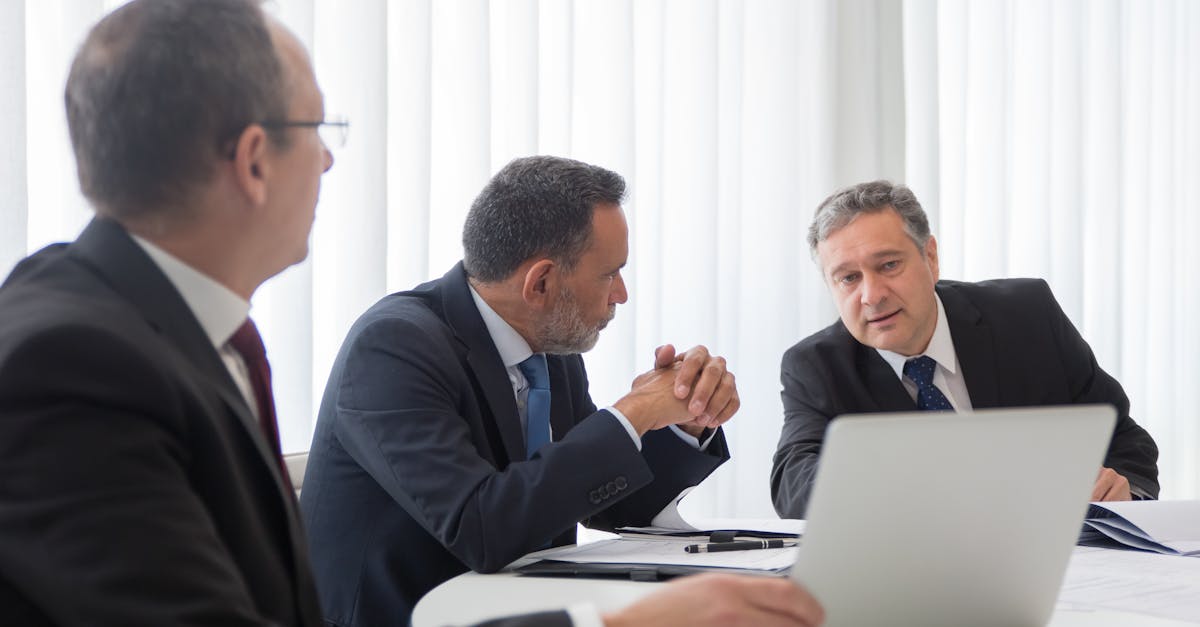
Data analysis plays a crucial role in today's business decision-making processes. With the increasing complexity and volume of data, organizations are constantly searching for innovative technologies to leverage their data insights effectively. The advent of ChatGPT-4, powered by B2B2C technology, is a game-changer in the field of data analysis. This cutting-edge technology promises to translate complex data insights into easily understood narratives, thus revolutionizing the decision-making process.
Understanding B2B2C Technology
B2B2C (Business-to-Business-to-Consumer) is a technology model that facilitates interactions between businesses, service providers, and end-users. In the context of data analysis, B2B2C technology bridges the gap between data analysis software providers and the end-users, enabling them to derive actionable insights from complex data sets. It combines the functionalities of business-to-business (B2B) and business-to-consumer (B2C) technologies, providing a comprehensive data analysis solution.
Enhancing Data Analysis with ChatGPT-4
ChatGPT-4 is an advanced language model that utilizes artificial intelligence and machine learning algorithms to generate human-like responses. By leveraging B2B2C technology, ChatGPT-4 can transform complex data insights into easily understood narratives. This is beneficial for organizations as it enables decision-makers, regardless of their technical background, to access and comprehend the valuable information hidden within their data.
With ChatGPT-4, organizations can simply input their data sets and ask specific questions regarding their business objectives to obtain coherent and contextual responses. The language model analyzes the data and generates narrative descriptions that highlight significant trends, patterns, correlations, and anomalies within the data. These narratives serve as a medium for efficient communication between the data analysis software and the end-users, eliminating the need for extensive technical knowledge to interpret complex statistical outputs.
Improving the Decision-Making Process
The ability of ChatGPT-4 to translate data insights into easily understood narratives has a profound impact on the decision-making process. This technology empowers decision-makers, irrespective of their technical proficiency, to make informed decisions quickly. The narratives provided by ChatGPT-4 allow decision-makers to extract actionable insights, identify potential risks, and develop strategies to optimize their business operations.
Furthermore, ChatGPT-4 facilitates collaboration among team members by serving as a universal communication tool. It bridges the gap between data scientists, business analysts, and decision-makers, enabling them to share and exchange ideas efficiently. The simplified narratives generated by ChatGPT-4 enhance clarity and understanding, promoting productive discussions and aligning the entire organization towards common goals.
Conclusion
ChatGPT-4, powered by B2B2C technology, introduces a revolutionary approach to data analysis. By translating complex data insights into easily understood narratives, it empowers decision-makers to extract valuable information from their data sets and make informed decisions. This technology not only enhances the decision-making process but also promotes collaboration, productivity, and alignment within organizations. As data analysis continues to play an increasingly significant role in business success, ChatGPT-4 proves to be an invaluable tool in leveraging data insights to drive positive outcomes.
Comments:
Thank you all for reading my article on enhancing data analysis efficiency using ChatGPT in B2B2C technology. I'm glad to see your interest in this topic.
Great article, Anthony! I found the concept of leveraging ChatGPT for data analysis quite intriguing. Can you share more insights into how it can benefit B2B2C technology?
Thank you, Samuel! ChatGPT can benefit B2B2C technology by automating data analysis processes, enabling real-time interaction with data, and assisting in generating insights for businesses.
Interesting read! I'm curious about the limitations of ChatGPT in terms of handling complex datasets. Anthony, could you provide some information on that?
Emily, while ChatGPT can handle complex datasets to some extent, it primarily excels in tasks that involve natural language processing and generating text-based insights. Dealing with highly specialized or domain-specific datasets might require additional fine-tuning or domain-specific models.
Impressive article, Anthony! I agree that ChatGPT can really boost data analysis efficiency. I'd love to hear some real-life use cases of its application in B2B2C technology.
Liam, sure! One example is using ChatGPT to analyze customer feedback in a B2B2C context. By feeding the model with text data, it can extract sentiments, identify trends, and provide valuable insights for improving products or services.
Hey Anthony, great write-up! As an analyst myself, I'm wondering about the potential challenges one might face while implementing ChatGPT in a B2B2C context. What do you think?
Sophia, implementing ChatGPT in B2B2C can have challenges related to data security and privacy, ensuring the model understands industry-specific terminology, and considering biases that might affect the analysis. It's crucial to address these challenges to obtain accurate and reliable results.
Anthony, I can picture how ChatGPT can help analyze large datasets efficiently. How scalable is the implementation? Are there any performance concerns or limitations?
Megan, ChatGPT can be scaled by distributing the workload across multiple instances or leveraging cloud-based solutions. However, performance can be impacted by factors like the size of the dataset, the complexity of the queries, and the available computing resources.
Anthony, your article convinced me that ChatGPT can be a game-changer in data analysis for B2B2C. Are there any known limitations or potential pitfalls one should be aware of while using this approach?
Timothy, indeed, there are some limitations to be aware of. ChatGPT might generate plausible but incorrect responses, and it's important to validate its output. It can also be sensitive to input phrasing, so formulating clear and precise queries is crucial to obtain accurate results.
Anthony, I'm concerned about biases in the data that might affect the insights generated by ChatGPT. How can one mitigate this issue?
Olivia, mitigating biases is crucial. It involves carefully curating training data to ensure diversity and fairness. Bias monitoring and running sensitivity tests can also help in identifying and addressing any potential biases. Continuously refining the training data and fine-tuning the model can lead to better representation and reduced biases.
Anthony, what kind of computing resources are typically required to implement ChatGPT on large-scale datasets? Are there any specific hardware or software requirements?
David, the computing resources required depend on the size of the dataset and the complexity of the analysis. For large-scale datasets, powerful GPUs or TPUs are often used to speed up the computations. Additionally, software frameworks like Tensorflow or PyTorch are commonly utilized for implementing ChatGPT.
I enjoyed your article, Anthony! In terms of B2B2C technology, can ChatGPT be applied beyond data analysis? Are there any other potential use cases?
Rachel, absolutely! ChatGPT has the potential to be applied beyond data analysis in B2B2C technology. It can be utilized for tasks like customer support chatbots, virtual assistants, content generation, and more. Its versatility makes it a valuable tool in various domains.
Anthony, how would you compare ChatGPT's performance with traditional data analysis techniques when it comes to B2B2C scenarios?
Daniel, ChatGPT offers a different approach compared to traditional data analysis techniques. While traditional methods excel in structured analysis and statistical inference, ChatGPT shines in unstructured data analysis, natural language processing, and generating human-like responses. It can complement and enhance traditional techniques, providing a more interactive and conversational analysis experience.
Anthony, I'm curious about the training process of ChatGPT. How is the model trained to handle B2B2C domain-specific terminology and context?
Isabella, training ChatGPT involves pre-training it on a massive corpus of publicly available text from the internet. However, to make it domain-specific, additional fine-tuning is performed using proprietary or specific datasets related to B2B2C technology. This fine-tuning helps the model understand the terminology and context specific to the domain.
Anthony, can ChatGPT handle real-time data analysis? What is the typical latency in generating responses?
Nathan, ChatGPT can handle real-time data analysis, but latency depends on factors like the complexity of the queries and the available computing resources. In some cases, responses can be generated within a few seconds, while more complex queries might require additional time for processing and analysis.
Anthony, what measures can be taken to ensure the security and privacy of sensitive data while using ChatGPT for B2B2C analysis?
Grace, ensuring security and privacy is crucial. It's recommended to implement proper data encryption, access controls, and secure communication channels while using ChatGPT. Additionally, sensitive information should be masked or anonymized before feeding it to the model. Compliance with data protection regulations and best practices is essential in maintaining data privacy.
Anthony, can ChatGPT handle different data formats like structured data or only unstructured text data?
Oliver, while ChatGPT primarily excels in analyzing unstructured text data, it can also handle structured data to some extent. However, transforming structured data into a text format that the model can understand might be required. For more advanced structured data analysis tasks, other specialized techniques would be more appropriate.
Anthony, how often does the ChatGPT model need to be retrained to maintain its accuracy and relevance?
Lucas, the retraining frequency depends on factors like the availability of new relevant data and any changes in the B2B2C domain. If the context evolves, regular updates are needed to ensure the model stays accurate and relevant. Continuous monitoring of model performance and feedback from users also helps in identifying when retraining is necessary.
Anthony, what kind of computational costs are associated with retraining ChatGPT periodically?
Evelyn, retraining ChatGPT can involve significant computational costs, as it requires powerful hardware and extensive computing resources. Depending on the size of the model and the dataset, it might also consume a considerable amount of time. Proper resource planning and optimization are necessary to ensure the retraining process is efficient and cost-effective.
Anthony, what are some common approaches to collecting user feedback to improve the performance of ChatGPT in B2B2C applications?
Hannah, a common approach is to collect user feedback through rating systems or by allowing users to provide explicit feedback on the generated responses. This feedback can be used to identify areas where the model performs well or needs improvements. User feedback is invaluable in iteratively refining and enhancing the performance of ChatGPT in B2B2C applications.
Anthony, can you explain the fine-tuning process of ChatGPT using specific B2B2C datasets? How crucial is it to achieve accurate results?
William, fine-tuning ChatGPT with specific B2B2C datasets involves training the model on domain-specific examples to make it understand and respond accurately to the context. It helps ensure accurate results by aligning the model's knowledge with the particular domain. Fine-tuning is crucial in enhancing the performance and relevance of ChatGPT in B2B2C scenarios.
Anthony, how can one assess the performance and accuracy of ChatGPT in a B2B2C context?
Victoria, assessing ChatGPT's performance and accuracy in a B2B2C context can be done through various methods. These include metric-based evaluations by comparing the model's responses with gold-standard answers, user feedback analysis, and real-world testing of the model's outputs against known ground truths. Regular evaluations and continuous monitoring help in understanding and improving its performance.
Anthony, are there any specific evaluation metrics commonly used to measure the performance of ChatGPT in B2B2C applications?
Natalie, in B2B2C applications, commonly used evaluation metrics for ChatGPT include metrics like BLEU score (measuring response similarity to reference answers), perplexity (measuring language model uncertainty), and human evaluation involving subjective assessments of generated responses. A combination of these metrics provides a comprehensive evaluation of performance in different dimensions.
Anthony, I'm curious about the applicability of ChatGPT in different languages. Can it handle language-specific nuances and understand non-English contexts?
Caleb, while ChatGPT's primary training is based on English text data, it can handle other languages to some extent. However, understanding language-specific nuances and non-English contexts might not be as accurate or reliable as in English. Fine-tuning with specific non-English datasets can improve its performance for other languages, but it has its limitations.
Anthony, what are some potential future developments in using ChatGPT for B2B2C data analysis?
Andrew, the future developments in using ChatGPT for B2B2C data analysis are promising. Improvements in model scalability, access to more domain-specific training data, and further advances in fine-tuning techniques can enhance its accuracy and applicability. Additionally, research on addressing biases, reducing response ambiguity, and refining multi-turn conversation handling can contribute to future advancements in this field.
Anthony, how would you compare ChatGPT with other language models like BERT or GPT-3 for data analysis in B2B2C settings?
Chloe, ChatGPT, BERT, and GPT-3 serve different purposes in data analysis. While ChatGPT specializes in conversational analysis and generating text-based insights, BERT focuses more on understanding language context, and GPT-3 offers a broader range of language generation tasks. The choice depends on the specific requirements of the B2B2C data analysis task at hand.
Anthony, what are some potential challenges in deploying ChatGPT for real-world B2B2C data analysis projects?
Jason, deploying ChatGPT in real-world B2B2C data analysis projects can face challenges like integrating it with existing infrastructure, ensuring data security and privacy, handling high volumes of data, and addressing limitations in language understanding. Additionally, transferring insights from ChatGPT into actionable decisions and managing user expectations can also pose challenges.
Anthony, in your opinion, what are the key advantages of using ChatGPT over traditional data analysis methods in a B2B2C context?
Sophie, some key advantages of using ChatGPT over traditional data analysis methods include its ability to interactively explore data, generate human-like responses, handle unstructured text data well, and provide a more conversational and dynamic analysis experience. ChatGPT enables a more intuitive and user-friendly approach to data analysis, especially in domains involving B2B2C interactions.