Enhancing Data Analysis with ChatGPT: The Power of Glassfish Technology
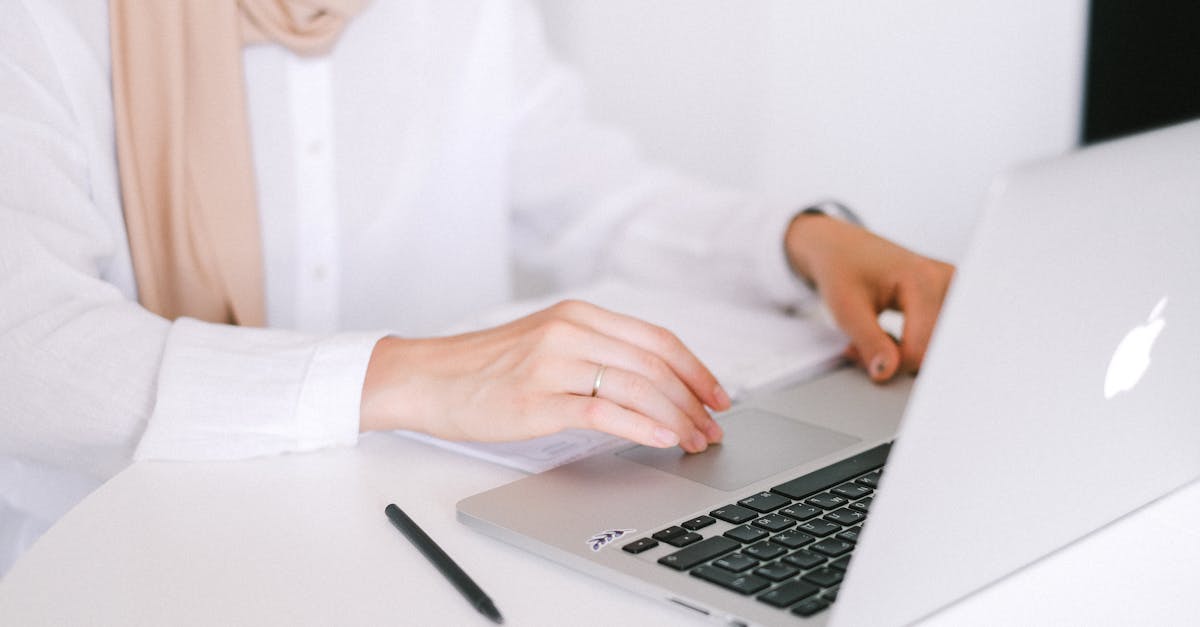
As technology continues to advance, the need for efficient data analysis has become increasingly important. With the introduction of ChatGPT-4, an advanced language model designed to understand and generate human-like text, the potential for data analysis has reached new heights. However, the ability to process massive amounts of data in a quick and user-friendly manner is crucial. This is where Glassfish, a powerful technology, comes into play.
The Technology: Glassfish
Glassfish is an open-source application server that is highly sought after in the world of Java-based enterprise applications. Developed by the community, Glassfish provides a reliable and scalable environment for hosting applications. Its support for Java EE standards and extensive set of features make Glassfish a popular choice among developers and organizations.
The Area: Data Analysis
Data analysis is the process of inspecting, cleaning, transforming, and modeling data in order to discover useful information, draw meaningful conclusions, and support decision-making. It plays a critical role in various industries, including finance, healthcare, marketing, and more. However, the analysis of large datasets can be a complex task, requiring sophisticated tools and techniques.
The Usage: ChatGPT-4's Data Analysis Capabilities
ChatGPT-4, powered by Glassfish, revolutionizes data analysis by providing an effective and user-friendly way to process massive amounts of data. Its advanced language comprehension capabilities combined with Glassfish's reliability and scalability make it an ideal tool for such tasks.
With ChatGPT-4, users can provide input in the form of raw data or queries, and the model can analyze the information to generate actionable insights and user-friendly reports. By leveraging Glassfish, ChatGPT-4 can handle significant data sets efficiently, ensuring quick analysis and faster report generation.
The ability to process data at scale is crucial in today's data-driven world. ChatGPT-4, powered by Glassfish, excels in this area by providing efficient data analysis capabilities. Its integration with Glassfish enables seamless data processing, allowing users to explore and make sense of large data sets in a timely manner.
Conclusion
Glassfish empowers ChatGPT-4 to perform data analysis tasks with unparalleled efficiency and effectiveness. The combination of Glassfish's powerful capabilities as an application server and ChatGPT-4's advanced language comprehension abilities make it a dynamic duo in the field of data analysis. Whether it is drawing insights from massive datasets or generating user-friendly reports, ChatGPT-4, powered by Glassfish, sets a new standard in the realm of efficient data processing.
Comments:
Thank you all for reading my article on enhancing data analysis with ChatGPT! I'm glad you found it interesting. If you have any questions or comments, feel free to let me know!
I really enjoyed your article, Jed! It's fascinating to see how ChatGPT can enhance data analysis. Do you have any recommendations on how to get started with this technology?
Emma, to get started with ChatGPT, I recommend exploring OpenAI's documentation and examples. They provide a good starting point for understanding its capabilities and usage.
Great article, Jed! ChatGPT seems like a powerful tool for data analysis. What are the limitations or challenges that users might face when using this technology?
Robert, while ChatGPT is a powerful tool, it has its limitations. It might provide incorrect answers or generate plausible-sounding but incorrect responses. It's crucial to review and verify the outputs.
Thanks for sharing your insights, Jed! I'm curious, how does ChatGPT compare to other data analysis tools available in the market?
Sarah, compared to other data analysis tools, ChatGPT offers a more interactive and conversational approach. It can help users make sense of their data by providing detailed explanations and insights.
I found your article very informative, Jed! ChatGPT seems like a great addition to the data analysis toolkit. Have you tried using it for real-world applications?
Thank you, Oliver! Yes, I've personally used ChatGPT for various real-world applications, such as customer support and market research. Its ability to understand and respond to user queries makes it versatile.
Jed, this technology sounds promising, but what steps can users take to mitigate the risks of incorrect answers or misleading responses from ChatGPT?
Good question, Sophia! To mitigate risks, users can employ techniques like prompt engineering, where carefully crafted questions and instructions help guide ChatGPT towards more accurate responses.
Additionally, users can consider using rule-based filters to identify and remove any potentially incorrect responses generated by ChatGPT. Regularly reviewing and validating the outputs is essential.
Jed, it's intriguing how ChatGPT offers a conversational approach to data analysis. How does it handle large datasets or complex queries?
Great question, Michael! ChatGPT can handle large datasets by breaking them down into smaller queries or by focusing on specific aspects of the data. Complex queries can be handled through iterative conversations.
Jed, what measures can users take to ensure they verify and validate the outputs of ChatGPT in their data analysis process?
Karen, it's crucial to have human reviewers involved in the data analysis process. They can review the outputs, perform follow-up checks, and compare against established benchmarks or ground truth.
Jed, how does ChatGPT handle data privacy and security concerns when working with sensitive datasets?
Data privacy and security are of utmost importance. When working with sensitive datasets, it's recommended to follow industry-standard security practices, like anonymization and encryption, to protect the data.
Jed, I'm curious about the performance and scalability of ChatGPT. How well does it handle high volumes of queries?
Liam, ChatGPT can handle high volumes of queries, but its response time might increase with increased load. Proper infrastructure scaling and optimization can help maintain its performance.
Jed, what are the specific use cases where ChatGPT has shown the most value in data analysis?
Emily, apart from customer support and market research, ChatGPT has been valuable in areas like content generation, query handling, exploratory data analysis, and assisting data scientists in their workflows.
Jed, how can prompt engineering be effectively used to improve the accuracy of ChatGPT's responses?
Daniel, prompt engineering involves providing explicit instructions and examples within the prompt to guide ChatGPT. By carefully shaping the input, users can influence the quality and accuracy of the responses.
It's an iterative process where users experiment with different prompts, iteratively refine them, and assess the generated outputs to achieve the desired results.
Jed, are there any existing rule-based filters available for users to remove potentially incorrect ChatGPT responses?
Sophie, OpenAI provides guidance on how to apply rule-based filters to mitigate risks. Users can define a set of rules to detect certain types of incorrect responses and prevent them from being shown to users.
The OpenAI Cookbook also offers examples and techniques for building rule-based systems on top of ChatGPT.
Jed, are there any limitations or challenges when using ChatGPT for handling complex queries?
Ava, handling complex queries with ChatGPT can sometimes be challenging as it might need clarifications or additional prompts to better understand the user's intent. Iterative conversations can help overcome this challenge.
Jed, in the context of data analysis, how can human reviewers effectively validate and verify the outputs?
Henry, human reviewers play a vital role in the validation process. They can compare the outputs generated by ChatGPT against known ground truth or established benchmarks, perform spot checks, and verify the accuracy of the analysis.
Human reviewers help ensure the generated insights are reliable and align with the desired results or objectives of the data analysis.
Jed, are there any additional precautions or best practices users should consider when handling sensitive datasets?
Grace, in addition to standard security practices, it's advisable to enforce strict access controls and conduct regular security audits to identify and address any vulnerabilities in the system.
Users should also stay updated with the latest security patches and follow compliance regulations relevant to the data they are handling.
Jed, from a user's perspective, how can one ensure the performance of ChatGPT doesn't degrade in high-load scenarios?
Noah, to ensure optimal performance under high-load scenarios, users can leverage cloud-based infrastructure and auto-scaling mechanisms. This allows the system to dynamically allocate resources based on demand, preventing degradation.
Efficient resource allocation, load balancing, and optimizing the system architecture can help maintain ChatGPT's performance even during peak usage times.
Jed, in what ways does ChatGPT assist data scientists in their workflows?
Olivia, ChatGPT assists data scientists by helping them explore datasets, answer ad-hoc analytical queries, gain quick insights, and provide explanations for model predictions or analysis results.
It can be a valuable tool during the initial stages of data analysis and model development, where data scientists often require interactive feedback and explanations.
Jed, what factors should users consider when shaping the prompt during prompt engineering?
Nathan, users should consider the desired level of detail in the response, the specific aspects or dimensions of the data to focus on, and any potential ambiguities that need clarification within the prompt.
Experimenting with prompt variations and evaluating the generated outputs can help users iteratively refine their prompts to achieve more accurate and insightful responses.
Jed, how does ChatGPT handle queries that are outside the scope of its training data?
Lucy, ChatGPT might struggle with queries outside the scope of its training data. It's important to set clear expectations with users about the domain and limits of ChatGPT to manage queries that fall outside its capabilities.
Jed, what are the recommended practices for selecting human reviewers to validate the outputs?
Benjamin, human reviewers should have a good understanding of the domain, contextual knowledge, and expertise in the data analysis area. They should be familiar with the organization's data analysis goals and objectives. It's also important to train and provide guidelines to reviewers.
Having a diverse set of reviewers can help ensure a well-rounded validation process, bringing in different perspectives and reducing potential biases.