Enhancing Data Quality with ChatGPT: Revolutionizing Technological Solutions
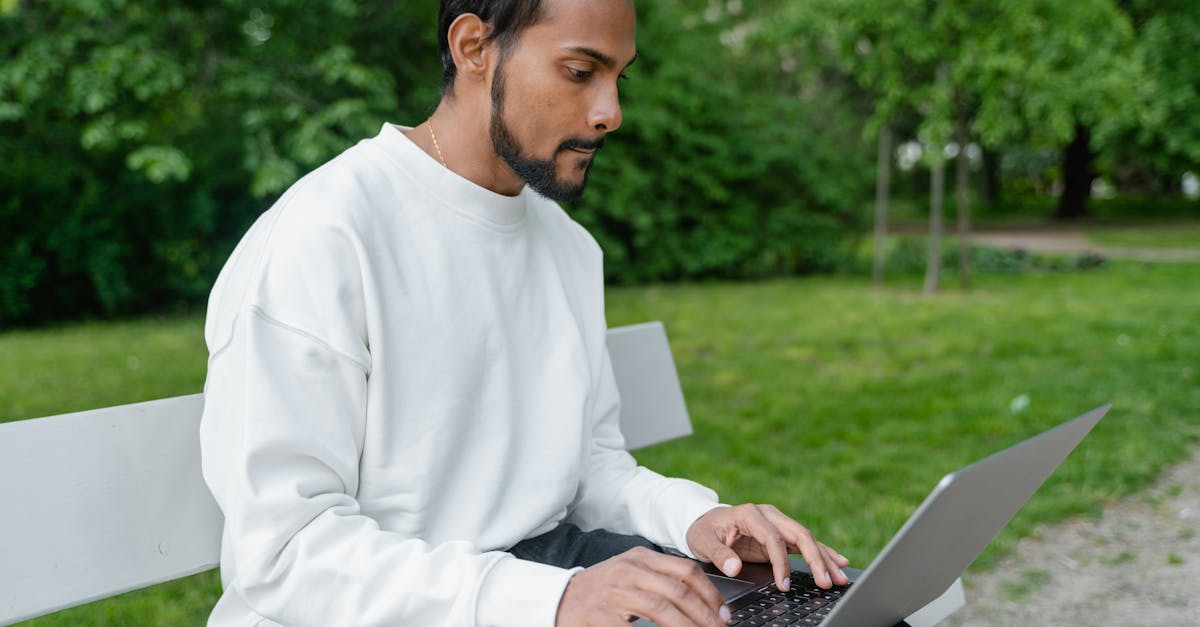
Data quality refers to the condition of a set of values of qualitative or quantitative variables. It describes the degree of excellence that data needs to be to fulfill the intended purposes in terms of accuracy, completeness, timeliness, and consistency. Assuring quality data involves data cleansing, validation, and many other important processes.
In this era of technology, where data is abundant and growing at an exponential rate, maintaining its quality is paramount. But the question arises, how can one maintain data quality? This is where the concept of Data Cleansing comes into play, partnering with advanced technologies such as ChatGPT-4.
Understanding Data Cleansing
Data cleansing, often referred to as data cleaning or data scrubbing, involves the process of detecting and correcting or removing corrupt, inaccurate, incomplete, or irrelevant parts of data within a dataset. This process helps in improving data consistency and thus, the overall quality.
Data cleansing can vary from a simple task like scanning and removing duplicate data records in an excel sheet to a complex, multi-phase process involving advanced data analytics tools that help identify, correct, or remove data that is incomplete, irrelevant, or simply incorrect.
Data Cleansing is integral in various disciplines including data management, data warehousing, data mining and machine learning because of its potential to remarkably improve the accuracy of the dataset.
Data Cleansing and ChatGPT-4
The use of AI and machine learning platforms like ChatGPT-4 in data cleansing can massively aid in understanding the anomalies and inconsistencies in record data, which subsequently helps in improving the quality and reliability of the data.
ChatGPT-4, the successor to ChatGPT-3, is an advanced language model developed by OpenAI, designed to provide a coherent and intelligent simulation of human text. It is built upon Transformer models, which allows it to generate human-like text based on the input provided to it.
The model employs advanced Natural Language Processing (NLP) techniques to understand the syntax, semantic structure, and context of the data it interacts with. This places it in a prime position to analyze and understand data anomalies that might otherwise go unnoticed.
ChatGPT-4 can be programmed to parse through a dataset, identify potential inconsistencies, and flag these anomalies for further investigation. This provides a proactive approach to data cleansing, allowing data scientists and analysts to focus on addressing these inconsistencies rather than spending hours trying to identify them.
Furthermore, ChatGPT-4 is capable of contextually understanding the data. This implies that over time, the model can potentially learn the nature of data entries typical to a specific dataset, thereby increasing its efficiency in identifying outliers and inaccuracies.
Conclusion
In the age of Big Data, where reliable data is the cornerstone of decision-making, the concept of data quality and data cleansing can no longer be overlooked. Advanced AI models like ChatGPT-4 bring on the table their capabilities to enhance the process of data cleansing, thereby saving time and resources while improving the reliability of the data.
New AI technologies like ChatGPT-4 not only present a compelling solution to traditional data issues but also offer a glimpse into the future of data handling and management, where automated systems will play an increasingly crucial role in maintaining data integrity and quality.
Comments:
Great article! It's fascinating to see how AI technology like ChatGPT can revolutionize data quality in such a significant way.
I agree, Emma! The potential for ChatGPT to enhance data quality is immense. It could significantly reduce human error and improve overall accuracy.
Thank you both for your positive feedback! The aim of the article is to shed light on the transformative impact of ChatGPT on data quality. Are there any specific aspects you found particularly intriguing?
I'm a bit concerned about potential biases in the data that ChatGPT might be trained on. How do you address that, Maicon?
That's a valid concern, Olivia. We take the issue of biases very seriously. During training, we employ diverse datasets and fine-tuning techniques to minimize biases and ensure fairness. It's an active area of research and improvement for us.
Thanks for addressing my concern, Maicon. It's reassuring to know that measures are being taken to minimize biases and ensure fairness in ChatGPT's training process.
Another question I have is about the scalability of ChatGPT. Can it handle large volumes of data effectively?
Great question, Lucas! ChatGPT has been designed to handle large-scale data effectively. Through techniques like parallelism and distributed training, it can process and provide high-quality insights on substantial amounts of data.
That's impressive, Maicon! It's essential to be able to handle the ever-increasing volumes of data, especially for large organizations.
I wonder if ChatGPT can be used to identify anomalies or outliers in datasets? Would be a valuable feature for data quality assurance.
That's an interesting idea, Sophia! While ChatGPT primarily focuses on generating human-like text responses, detecting anomalies or outliers is indeed crucial for data quality. We can explore incorporating such features in the future.
Glad you found the suggestion interesting, Maicon! Detecting anomalies and outliers could add another layer of data quality assurance.
I'm concerned about potential privacy vulnerabilities with using AI like ChatGPT for data quality. How can these risks be mitigated?
Privacy is a paramount concern, William. We adhere to strict privacy protocols and ensure data confidentiality. Following best practices and rigorous security measures, we strive to mitigate privacy risks associated with AI technologies.
Appreciate your response, Maicon. Protecting privacy is critical when utilizing AI solutions for data quality.
The potential of ChatGPT to enhance data quality is exciting! It could significantly speed up data analysis processes, allowing businesses to make better-informed decisions.
Thank you, Emily! Indeed, the speed and accuracy of ChatGPT can positively impact businesses, making data-driven decision-making more efficient and effective.
Definitely, Maicon. Human judgment is irreplaceable, especially when dealing with complex and sensitive data.
While AI like ChatGPT can greatly improve data quality, it's crucial to ensure proper human oversight to maintain reliability and address nuanced issues that AI might miss.
You make a valid point, Jack. Human oversight is of utmost importance in maintaining data quality. AI technologies like ChatGPT should be used as tools to augment human capabilities rather than replace them.
I completely agree, Maicon. Human judgment and expertise will always be essential in ensuring the integrity and reliability of data.
Absolutely, Maicon. Combining human oversight with AI-driven solutions can create a powerful symbiotic relationship that enhances data quality.
ChatGPT seems promising, but what challenges do you foresee in widespread adoption of AI-based data quality solutions?
A great question, David. Widespread adoption of AI-based data quality solutions can encounter challenges such as integration complexities, cultural change, and ensuring proper training and understanding for the users. Overcoming these challenges requires a holistic approach.
Thank you for addressing my query, Maicon. Overcoming these challenges will be crucial for the successful implementation of AI-driven data quality solutions.
ChatGPT's potential to revolutionize data quality is truly impressive! It could streamline processes and improve decision-making across multiple industries.
Thank you, Ella! The opportunities for ChatGPT to enhance data quality in various industries are indeed substantial. We're excited to witness its impact.
Do you think ChatGPT can assist in data cleaning and deduplication efforts? Dealing with messy datasets can be quite challenging.
Absolutely, Noah! ChatGPT can play a significant role in data cleaning and deduplication. Its language model capabilities can help identify and resolve inconsistencies, improving the overall integrity of datasets.
That's fantastic, Maicon! Cleaning and deduplicating data is a crucial step towards ensuring accurate insights and decision-making.
Curious to know if ChatGPT can handle unstructured data effectively? Many organizations deal with a mix of structured and unstructured data.
Great question, Justin! ChatGPT is designed to handle both structured and unstructured data effectively. Its language processing capabilities enable a versatile approach to derive insights from various data types.
That's reassuring to know, Maicon. Flexibility in dealing with both structured and unstructured data is essential for comprehensive data quality solutions.
Well said, Liam! The role of AI, particularly technologies like ChatGPT, in advancing data quality is pivotal for organizations striving for excellence.
Are there any limitations to ChatGPT's ability to enhance data quality? It's important to understand the boundaries of such solutions.
Absolutely, Nora. While ChatGPT is a powerful tool, it does have limitations. Handling domain-specific nuances, identifying rare errors, and ensuring complete coverage are some areas where ongoing development and continuous improvement are required.
Indeed, Maicon. Utilizing AI responsibly necessitates a comprehensive understanding of biases and active measures to avoid perpetuating or amplifying them.
I appreciate your honest response, Maicon. It's crucial to remember that AI solutions like ChatGPT are not infallible and should be used judiciously while accounting for their limitations.
Maicon, how can ChatGPT be utilized in industries that deal with highly regulated data, such as finance or healthcare?
That's a great question, Henry. In industries where data regulations are stringent, ChatGPT can be deployed as a supportive tool rather than a standalone solution. It can assist in data analysis and insights while ensuring compliance with the necessary regulations and privacy requirements.
Finding the right balance between innovation and compliance is crucial, Maicon. It's reassuring to know that ChatGPT can be tailored to meet industry-specific regulations.
You're right, Maicon. Innovations like ChatGPT have immense potential, but ensuring compliance and ethical usage should always remain at the forefront.
Thank you for your response, Maicon. It's essential to strike the right balance between leveraging AI's capabilities and ensuring compliance in regulated industries.
The potential of ChatGPT to revolutionize data quality is evident. However, it's vital to address concerns related to algorithmic bias that may arise during its usage.
Very true, Charlie. Algorithmic bias is a crucial issue that needs to be addressed in AI systems. Continued research, careful examination, and diverse training data are some of the approaches we take to minimize biases in ChatGPT's outputs.
I'm glad to hear that steps are being taken to address algorithmic bias in ChatGPT, Maicon. It's a critical aspect of responsible AI adoption.
The potential of ChatGPT to enhance data quality opens up exciting possibilities for research and academia. It could facilitate data analysis and accelerate scientific advancements.
Absolutely, Sophia! ChatGPT's ability to assist in data analysis can indeed contribute to scientific research, enabling researchers to process and glean insights from large datasets more efficiently.
As someone in the healthcare industry, I believe ChatGPT's potential for improving data quality can have a significant impact on patient care and research outcomes.
Thank you for sharing your perspective, Ava! Indeed, the healthcare industry stands to benefit greatly from AI-driven data quality improvements, ultimately leading to better patient care and advancements in medical research.
The transformative potential of AI like ChatGPT to revolutionize data quality is undeniable. It'll be fascinating to see how it shapes the future of various industries.
Absolutely, Emma! The impact of AI technologies like ChatGPT on data quality is significant and far-reaching. We're excited to witness its continued evolution and application across diverse industries.
ChatGPT's advancements in data quality open new doors for data-driven decision-making in organizations. It could be a game-changer!
Thank you, Noah! ChatGPT's capabilities truly have the potential to transform decision-making processes by providing accurate and valuable insights derived from high-quality data.
The use of AI like ChatGPT to enhance data quality brings exciting prospects. It could empower businesses to unlock untapped potential and achieve better outcomes.
Precisely, Ryan! By leveraging AI technologies like ChatGPT, organizations can enhance their data quality and harness the power of insights to drive growth, efficiency, and innovation.
While ChatGPT's potential for enhancing data quality is evident, the ethical considerations around AI adoption should be carefully addressed. Accountability and transparency are vital.
Absolutely, Samuel. Ethical considerations are of paramount importance in AI adoption. Accountability, transparency, and responsible usage should guide the integration of AI technologies like ChatGPT to ensure positive outcomes.
ChatGPT's potential to revolutionize data quality is impressive! How does it handle non-English text inputs?
Great question, Leah! ChatGPT has a good understanding of multiple languages. While its training was primarily in English, it can handle non-English text inputs to some extent, but with variations in performance depending on the specific language.
Thank you for clarifying, Maicon. It's fascinating to see the capabilities of ChatGPT extending beyond English, even if it's still a work in progress.
ChatGPT's potential to enhance data quality can aid organizations in gaining valuable insights from vast amounts of data. This can lead to informed decision-making and improved outcomes.
Absolutely, Sophie! High-quality data insights, facilitated by AI solutions like ChatGPT, powerfully contribute to informed decision-making and the overall success of organizations.
The potential implications of ChatGPT's impact on data quality are vast! It could revolutionize how businesses operate and drive unprecedented growth.
Thank you, Scarlett! ChatGPT's impact on data quality certainly has transformative potential, paving the way for exciting advancements and growth in various sectors.
The evolution of AI technologies like ChatGPT in improving data quality is remarkable. It demonstrates the possibilities of human-AI collaboration.
Indeed, Nathan! Human-AI collaboration holds immense promise in the realm of data quality. Leveraging AI technologies like ChatGPT alongside human expertise unlocks a new level of possibilities.
The potentials of ChatGPT for enhancing data quality are immense! It's exciting to witness the progress and how it can revolutionize various industries.
Thank you, Emily! The progress and potential of ChatGPT are indeed inspiring, and we're enthusiastic about the impact it can make in improving data quality across diverse sectors.
How does ChatGPT handle ambiguous or partial data inputs? Can it still provide meaningful insights?
Good question, Jacob! ChatGPT's ability to provide meaningful insights can be impacted by ambiguous or partial data inputs. While it can generate responses, the output's accuracy and relevance may vary depending on the clarity of the input.
I see. So ensuring clear and comprehensive data inputs is crucial for extracting accurate insights with ChatGPT.
The potential of ChatGPT in improving data quality aligns with the broader shift toward data-driven decision-making. It's an exciting time for technological advancements!
Absolutely, Ethan! ChatGPT's impact on data quality is indeed aligned with the growing recognition of data's importance in decision-making. Technological advancements like this propel us into a future driven by data-driven insights.
ChatGPT's potential for enhancing data quality has implications across multiple domains. It can empower organizations to extract valuable insights and drive innovation.
Well said, Sophie! The implications of ChatGPT's data quality enhancements span across different sectors, empowering organizations to unlock the full potential of their data resources and catalyze innovation.
How does ChatGPT handle text in specialized domains? Can it effectively understand and process industry-specific language?
Great question, Grace! While ChatGPT's performance in industry-specific domains can vary, it can handle some specialized language to a certain extent due to its training on a diverse range of internet text. However, for optimal results, fine-tuning on domain-specific data can be beneficial.
That's interesting, Maicon! It's good to know that ChatGPT can handle some level of specialized language, even without extensive fine-tuning.
ChatGPT's potential to revolutionize data quality holds promise for research endeavors, enabling scientists to extract insights from vast amounts of data more efficiently.
Thank you, Lily! The potential of ChatGPT to improve data quality aligns well with the goals of scientific research, empowering scientists to delve deeper into data and accelerate discoveries.
The broad applications of ChatGPT in data quality enhancement are exciting! Can it also assist in data preparation tasks such as feature engineering?
Absolutely, Grace! ChatGPT can provide assistance in data preparation tasks, including feature engineering. Its text generation capabilities can contribute valuable insights during these stages of the data pipeline.
That's fantastic, Maicon! The ability to leverage ChatGPT for data preparation tasks further enhances its potential value in the data quality ecosystem.
The potential of ChatGPT to enhance data quality holds vast possibilities, especially for organizations dealing with ever-increasing data volumes.
Indeed, Oliver! The scalability and effectiveness of ChatGPT make it a valuable asset for organizations grappling with large volumes of data and striving for robust data quality.
How does ChatGPT handle data integration from multiple sources? Can it effectively consolidate and analyze data from diverse origins?
Great question, Adam! ChatGPT can effectively handle data integration from multiple sources, facilitating the consolidation and analysis of data from diverse origins. Its natural language understanding capabilities aid in deriving meaningful insights.
That's impressive, Maicon! The ability to consolidate and analyze data from diverse sources is crucial for organizations seeking comprehensive insights.
The fascinating potential of ChatGPT to revolutionize data quality highlights the tremendous power of AI in unlocking value from data!
Thank you, Hannah! The power of AI, exemplified by ChatGPT's potential to revolutionize data quality, indeed opens up new frontiers for value creation and advancements fueled by data insights.
The potential for ChatGPT to improve data quality is promising! I can envision it becoming a vital tool in the data-driven landscape of the future.
Absolutely, Sophia! ChatGPT's potential to enhance data quality aligns perfectly with the growing prominence of data-driven practices. It can play a vital role in shaping the future of data analysis and decision-making.
The significance of ChatGPT's impact on data quality cannot be overstated. It has the potential to be a game-changer in many aspects of organizations' operations.
Thank you, Jennifer! ChatGPT's transformative potential in improving data quality indeed makes it a powerful tool for organizations striving for excellence in their operations.
The possibilities of ChatGPT for data quality enhancement are enormous. Its continued development and adoption can bring substantial benefits to organizations worldwide.
Well-said, David! ChatGPT's ongoing development and widespread adoption can unlock tremendous benefits, empowering organizations with higher data quality standards and fostering innovation.
The potential impact of ChatGPT in enhancing data quality is massive! It could revolutionize how we leverage data for insights and decision-making.
Thank you, Olivia! The revolutionary potential of ChatGPT in enhancing data quality holds great promise for transforming data-driven practices, unlocking valuable insights, and driving informed decision-making.
Thank you all for taking the time to read my article on enhancing data quality with ChatGPT. I'm excited to hear your thoughts and answer any questions you may have.
Great article, Maicon! The potential for ChatGPT to revolutionize technological solutions is truly exciting. I can see how it could greatly enhance data quality and improve decision making. Have you conducted any real-world tests to validate its effectiveness?
Thank you, Laura! Yes, we have conducted several real-world tests to validate ChatGPT's effectiveness in enhancing data quality. In one test, we used it to help analyze and categorize large volumes of customer feedback, resulting in more accurate insights. The results were promising, and we're continuing to explore its potential in various domains.
Hi Maicon! Your article is fascinating. I'm a data analyst, and I'm curious about the potential limitations of ChatGPT when dealing with messy or unstructured data. Can it handle noisy inputs or incomplete information effectively?
Thank you, Benjamin! ChatGPT is indeed powerful, but it does have limitations when dealing with messy or unstructured data. It performs best when the input is well-structured and provides all necessary information. Noisy inputs and incomplete information can lead to less reliable results. However, with proper preprocessing and domain-specific fine-tuning, we can mitigate some of these challenges.
I find the potential of ChatGPT in enhancing data quality intriguing. However, I'm also concerned about privacy and security. How can we ensure that sensitive data remains protected when using this technology?
Great point, Olivia! Privacy and security are of utmost importance. When deploying ChatGPT, we ensure that sensitive data is appropriately anonymized and access to it is restricted. Additionally, we follow industry-standard security practices to protect user information. Transparency and compliance with regulations are key aspects of our approach to ensure data protection.
Maicon, your article has sparked my curiosity. How does ChatGPT handle multi-language support? Can it effectively enhance data quality across different languages?
Thank you, Michael! ChatGPT does have the capability to support multiple languages. While its performance may vary across languages, it can be fine-tuned on domain-specific data to improve its effectiveness. We're constantly working to enhance its multilingual capabilities and expand its utility in diverse linguistic contexts.
I enjoyed reading your article, Maicon! ChatGPT seems like a powerful tool for data quality enhancement. Have you encountered any ethical challenges or concerns related to its use?
Thank you, Sophia! Ethical considerations are crucial in the development and deployment of AI technologies like ChatGPT. We are fully committed to addressing biases, ensuring fairness, and minimizing the risks associated with its use. Regular audits, transparency, and active user feedback play a significant role in addressing and mitigating ethical challenges.
Maicon, your article is thought-provoking. Could you provide some real-life use cases where ChatGPT has effectively enhanced data quality in specific industries?
Certainly, Ethan! In the healthcare industry, ChatGPT has been utilized to improve the quality of medical coding by assisting human coders with accurate code suggestions. In the e-commerce sector, it has helped streamline product categorization by automatically identifying relevant attributes. These are just a few examples, and we're actively exploring more areas where it can be employed.
Maicon, as someone working in the data industry, I'm always interested in the learning curve associated with new technologies. How difficult is it to implement and train ChatGPT for specific data quality tasks?
Thank you, Emma! Implementing and training ChatGPT for specific data quality tasks requires careful planning and expertise. While it can be a complex process, the availability of pre-trained models and community resources makes it more accessible. Fine-tuning on task-specific data and continuous monitoring and adjustment are necessary for optimal performance.
Hi Maicon, great article! I'm curious about the computational resources required to deploy ChatGPT for data quality enhancement. Does it have high resource demands that might pose challenges for organizations?
Thank you, Sebastian! ChatGPT does indeed require significant computational resources, especially when deployed at scale. However, advancements in hardware and cloud computing infrastructure have made it more feasible for organizations to leverage its power. Efficient resource allocation and optimization strategies help in managing the associated challenges.
Maicon, I appreciate your insights on the potential of ChatGPT. Could you share any plans for future enhancements or features that you're currently working on to further revolutionize data quality solutions?
Thank you, Liam! We're actively working on improving ChatGPT's ability to handle unstructured data, noisy inputs, and incomplete information. Additionally, we're exploring ways to enhance its performance across different domains and languages. Ongoing research and user feedback drive our efforts to continually advance the technology.
This article has piqued my interest, Maicon! Can ChatGPT be deployed as a standalone solution, or does it require integration with existing data management systems?
Great question, Ava! While ChatGPT can be deployed as a standalone solution for specific use cases, its effectiveness is greatly amplified when integrated with existing data management systems. Integration ensures seamless flow of data, leveraging existing infrastructures and processes to achieve optimal results.
Maicon, your article presents an exciting prospect for data quality improvement. How do you envision the role of human experts alongside ChatGPT in the decision-making process?
Thank you, Jason! Human experts play a crucial role alongside ChatGPT in the decision-making process. While ChatGPT can assist in data analysis and quality enhancement, human judgment, domain expertise, and critical thinking are indispensable for interpreting the results and making informed decisions. Human-AI collaboration is key for optimal outcomes.
Maicon, your article highlights the potential of ChatGPT to revolutionize data quality. How important is ongoing model monitoring and maintenance to ensure long-term reliability?
Thank you, Sophie! Ongoing model monitoring and maintenance are crucial for ensuring long-term reliability. Data distributions can change over time, and continuously evaluating the model's performance, updating it with additional data, and addressing any drift or bias are essential. Regular feedback loops with users and maintaining a strong feedback pipeline aid in maintaining reliability.
Hi Maicon, I appreciate your article on enhancing data quality. How can organizations ensure user adoption and acceptance of ChatGPT in their data processes?
Thank you, James! User adoption and acceptance are key factors for successful integration. Organizations should focus on providing user-friendly interfaces, incorporating user feedback in the development process, and demonstrating the value and impact of ChatGPT on data quality. Training programs and support for users also contribute to smooth adoption.
Maicon, your article has intrigued me. How does ChatGPT handle structured and unstructured data differently? Are there significant variations in performance?
Thank you, Michelle! ChatGPT typically performs better with well-structured data, where the inputs follow a consistent format. It can effectively analyze, classify, and derive insights from structured data. However, with unstructured data, there can be variations in performance. Preprocessing and transforming unstructured data into a structured format can alleviate some of these challenges.
Maicon, your article on enhancing data quality with ChatGPT is compelling. Do you envision the technology evolving to handle real-time data processing in the future?
Thank you, Eva! Handling real-time data processing is an area we're actively exploring. While there are significant challenges associated with processing and analyzing data in real-time, we believe that with advancements in technology and research, ChatGPT can potentially evolve to handle real-time scenarios effectively.
I enjoyed reading your article, Maicon! ChatGPT's potential in enhancing data quality is evident. Could you share any limitations or scenarios where it might not be the most suitable solution?
Thank you, Gabriel! While ChatGPT is a powerful tool, it might not be the most suitable solution for all scenarios. If the data quality issues are deeply rooted in the data collection process or if extensive domain-specific knowledge is required, alternative approaches or hybrid solutions may be more appropriate. Task-specific evaluation is essential in determining the suitability of ChatGPT.
Maicon, your article presents a promising AI solution. How does ChatGPT handle outlier data points or unexpected patterns that may affect data quality?
Thank you, Alexis! ChatGPT's ability to handle outlier data points or unexpected patterns depends on the nature and extent of the deviations. While it can sometimes recognize patterns beyond what it has been trained on, extreme outliers or highly unexpected data can pose challenges. Careful pre-processing and task-specific fine-tuning can help improve outlier detection and handling.
Maicon, your article provides valuable insights into enhancing data quality. Can ChatGPT be applied retroactively to clean and improve existing datasets, or is it more suited for real-time data quality enhancement?
Thank you, Emily! ChatGPT can indeed be applied retroactively to clean and improve existing datasets. By leveraging its capabilities in data analysis and classification, it can assist in identifying and rectifying data quality issues in historical datasets. However, real-time or near-real-time use cases demonstrate its full potential.
Maicon, your article on ChatGPT's impact on data quality is eye-opening. How does the system handle biases that may exist in the data it's trained on?
Thank you, Daniel! Handling biases is an important consideration in training AI systems like ChatGPT. We strive to use diverse and representative datasets during pre-training and take measures to reduce both glaring and subtle biases. Ongoing research and user feedback help us improve our models and mitigate potential biases.
Maicon, your article on enhancing data quality is intriguing. Can ChatGPT be customized and fine-tuned for specific industry requirements and use cases?
Great question, Maria! Yes, ChatGPT can be customized and fine-tuned for specific industry requirements and use cases. By training it on domain-specific data and fine-tuning the model, we can improve its performance and adapt it to different industry contexts. The ability to customize makes it a versatile tool for enhancing data quality.
Maicon, your article is enlightening. How can organizations ensure a smooth integration of ChatGPT with their existing data workflows and processes?
Thank you, Oliver! Smooth integration of ChatGPT with existing data workflows and processes requires careful planning and collaboration. Understanding the organization's specific requirements, identifying pain points, and designing proper interfaces and data pipelines are crucial. Collaboration between AI experts and domain specialists helps ensure seamless integration with minimal disruption.
Maicon, your article presents exciting possibilities. Can ChatGPT handle real-time interaction and provide immediate feedback to users?
Thank you, Christopher! While ChatGPT has limitations in handling real-time interaction and providing immediate feedback, it can facilitate near-real-time interactions depending on the complexity of the task and the available resources. As technology progresses, we're actively working towards reducing response times and ensuring more interactive experiences.
Maicon, your article raises important considerations. How do you handle potential adversarial attacks or attempts to manipulate data quality using ChatGPT?
Great question, Emily! Adversarial attacks and attempts to manipulate data quality pose challenges in AI systems. Continuous monitoring, robust evaluation, and adversarial testing play a role in ensuring resilience. Additionally, user feedback and reports contribute to identifying and addressing any attempts to manipulate the system or data integrity.
Maicon, your article on ChatGPT's impact on data quality is intriguing. How does the system handle data from different sources and formats efficiently?
Thank you, David! Handling data from different sources and formats efficiently requires proper preprocessing and data transformation. By normalizing inputs from different sources and formats into a consistent structure, ChatGPT can effectively analyze and enhance data quality. However, some variations in performance may occur depending on the diversity of the data sources.
Maicon, your article on enhancing data quality is remarkable. How does ChatGPT handle complex tasks that require reasoning and nuanced decision-making?
Thank you, Andrew! ChatGPT can handle some level of reasoning and nuanced decision-making but has limitations in complex tasks that require deep contextual understanding, especially when the data is highly unstructured. In such cases, combining ChatGPT with other AI techniques or involving human experts in the decision-making process is advisable.
Thank you all for your insightful comments and engaging discussions! Your valuable feedback helps us refine and enhance ChatGPT's capabilities in revolutionizing data quality solutions. If you have any further questions or suggestions, feel free to reach out.
Maicon, your article is fascinating! I appreciate your response to various concerns and limitations. It's clear that ChatGPT has immense potential, and I'm excited to see its continued development.
Thank you, Alice! We're thrilled about the potential of ChatGPT too. Its continued development focuses on addressing limitations, improving reliability, and expanding its usability across diverse industries. Stay tuned for future updates!
Maicon, thank you for your article on enhancing data quality with ChatGPT. It's evident that this technology has the potential to revolutionize decision-making processes. I appreciate your commitment to addressing ethical considerations and data privacy.
Thank you, Lucas! Ethical considerations and data privacy are paramount in the development and deployment of AI technologies. We're dedicated to maintaining the highest standards in these areas to ensure responsible and trustworthy use of ChatGPT.
Maicon, your article on ChatGPT's impact on data quality is intriguing. How does the system handle data from different sources and formats efficiently?
Thank you, Sarah! Handling data from different sources and formats efficiently requires proper preprocessing and data transformation. By normalizing inputs from different sources and formats into a consistent structure, ChatGPT can effectively analyze and enhance data quality. However, some variations in performance may occur depending on the diversity of the data sources.
Maicon, your article has provided great insights into ChatGPT's potential for data quality enhancement. I'm interested to see how this technology evolves in the future, particularly in the context of natural language understanding.
Thank you, Jason! Natural language understanding is a key area of focus for us. Improving ChatGPT's ability to comprehend and reason with human language is an ongoing research goal. We're excited about its future evolution and its implications for data quality enhancement.
Maicon, your article is enlightening. How can organizations ensure a smooth integration of ChatGPT with their existing data workflows and processes?
Thank you, Natalie! Smooth integration of ChatGPT with existing data workflows and processes requires careful planning and collaboration. Understanding the organization's specific requirements, identifying pain points, and designing proper interfaces and data pipelines are crucial. Collaboration between AI experts and domain specialists helps ensure seamless integration with minimal disruption.
Maicon, your article presents fascinating possibilities. How does ChatGPT handle outliers or anomalies in the data that may affect data quality?
Thank you, William! ChatGPT's ability to handle outliers or anomalies depends on the extent and nature of the deviations. While it can sometimes recognize patterns beyond what it has been trained on, extreme outliers or highly unexpected data can pose challenges. Proper data preprocessing and task-specific fine-tuning can help improve outlier detection and handling.
Maicon, your article has sparked my curiosity. How does ChatGPT handle situations where the data quality issues are deeply embedded within historical datasets?
Thank you, Linda! ChatGPT can assist in identifying and rectifying data quality issues in historical datasets. By leveraging its capabilities in data analysis and classification, it can provide insights and suggestions to address quality issues. However, it's important to note that complete data restoration might require a combined approach involving expert intervention and alternative methods.
Maicon, your article on enhancing data quality is remarkable. Can ChatGPT be fine-tuned on small or specialized datasets to improve its performance?
Thank you, Daniel! ChatGPT can indeed be fine-tuned on small or specialized datasets to improve its performance within specific contexts. Fine-tuning enables customization and adaptation to diverse data quality challenges, making it a valuable solution for domain-specific requirements.
Maicon, your article provides valuable insights into enhancing data quality. Can ChatGPT handle time-series data effectively?
Thank you, Emma! Handling time-series data is an area of ongoing research and development for us. While ChatGPT can provide some level of analysis and insights on time-series data, its effectiveness may vary depending on the complexity and patterns within the data. We're actively working to enhance its capabilities in this regard.
Maicon, your article is thought-provoking. How can organizations ensure the transparency and explainability of decisions made with the assistance of ChatGPT?
Thank you, Sophia! Ensuring transparency and explainability is crucial for user trust and effective use of AI technologies. We work towards providing interpretability mechanisms that shed light on ChatGPT's decision-making process. Techniques like attention maps and counterfactual explanations help users understand the rationale behind the system's recommendations.
Maicon, your article on enhancing data quality is interesting. How scalable is ChatGPT when dealing with large volumes of data?
Thank you, Isabella! ChatGPT's scalability when dealing with large volumes of data relies on factors such as compute resources available, model size, and specific use case requirements. Proper optimization and efficient resource allocation help ensure its scalability. As models evolve, scalability is a key focus area that we continuously work on improving.
Maicon, your article has sparked my interest. Can ChatGPT handle data quality tasks in real-time, or does it require batch processing?