Enhancing Product Recommendations: Leveraging ChatGPT for Experienced Business Analysts
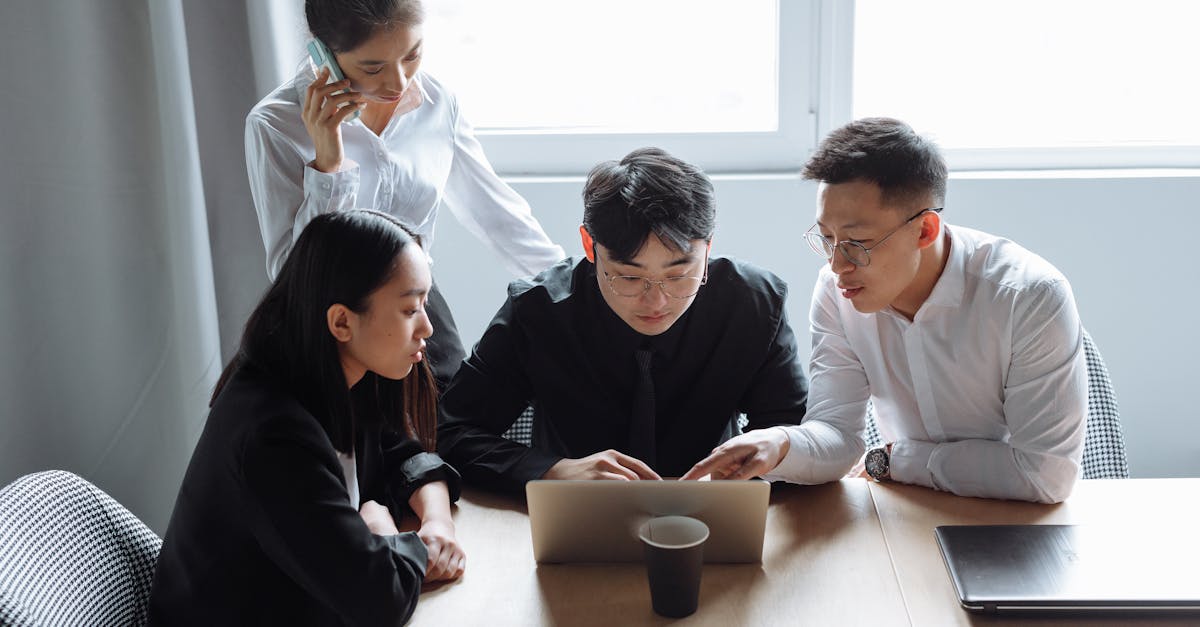
In the digital age, businesses are constantly searching for ways to personalize their customer experiences and drive sales. As a result, the role of a business analyst has become increasingly valuable, especially in the area of product recommendations. By leveraging technology, a business analyst can study customer behavior and preferences to recommend products that customers are likely to purchase. This article will explore how an experienced business analyst can utilize their expertise in product recommendations.
Understanding Customer Behavior
One of the key responsibilities of a business analyst is to understand customer behavior. By analyzing data such as purchase history, browsing patterns, and demographic information, a business analyst can identify patterns and trends. This information can provide valuable insights into customer preferences, enabling the analyst to make accurate product recommendations.
Utilizing Machine Learning
To effectively analyze customer behavior, an experienced business analyst should be well-versed in machine learning algorithms. Machine learning algorithms can process vast amounts of data and identify hidden patterns that are not immediately apparent to humans. By using machine learning algorithms, a business analyst can train models to predict customer preferences and make targeted product recommendations.
Collaborating with Stakeholders
While technology plays a significant role in the process of product recommendations, collaboration with stakeholders is equally important. A business analyst must work closely with stakeholders such as marketing teams, product managers, and developers to gather requirements and align recommendations with business objectives. By collaborating with stakeholders, an analyst can ensure that product recommendations are not only accurate but also aligned with the overall business strategy.
Measuring Effectiveness of Recommendations
An experienced business analyst must also have a strong understanding of metrics and analytics to measure the effectiveness of product recommendations. By tracking key performance indicators such as conversion rates, click-through rates, and average order value, an analyst can evaluate the impact of their recommendations on business outcomes. This data can be used to refine and improve future product recommendations, ultimately driving higher sales and customer satisfaction.
Conclusion
In the realm of product recommendations, an experienced business analyst plays a crucial role in leveraging technology to understand customer behavior and provide personalized recommendations. By utilizing machine learning algorithms, collaborating with stakeholders, and measuring the effectiveness of recommendations, a skilled analyst can drive sales and enhance the overall customer experience. As businesses continue to prioritize personalization, the demand for experienced business analysts in the field of product recommendations is expected to grow.
Comments:
Thank you all for joining the discussion! I'm glad to see the interest in leveraging ChatGPT for experienced business analysts. Please feel free to share your thoughts and questions.
Great article, Steve! The potential of using ChatGPT to enhance product recommendations is intriguing. Can you provide more insights into how it works?
Thank you, Emma! ChatGPT is a language model trained on diverse internet text, allowing it to generate human-like responses. In the context of product recommendations, it can engage in dynamic conversations with users to understand their preferences better and suggest relevant products.
I can see the benefit of ChatGPT in personalizing recommendations, but how reliable is it compared to traditional recommendation algorithms?
That's a valid concern, Mike. ChatGPT does bring a different approach, incorporating more user interaction. It can handle ambiguity better by asking clarifying questions, but it may require additional fine-tuning to ensure optimal reliability.
I wonder if using ChatGPT would add significant complexity to the system. What are your thoughts on the implementation process, Steve?
Good question, Sara. Implementation might involve integrating ChatGPT with existing recommendation systems and training it on relevant data. While it can introduce complexity, it's worth exploring for businesses aiming to provide more interactive and personalized experiences.
ChatGPT sounds promising, but what challenges should analysts expect while working with it?
Indeed, Kevin. Analysts may encounter challenges in handling biased responses, managing user expectations, and ensuring appropriate error handling. Continuous monitoring and training can help mitigate such issues.
I'm curious about the computational resources required for leveraging ChatGPT. Could it be a barrier for small businesses?
Good point, Emily. Initially, the computational resources required can be a challenge. However, with advancements in cloud-based services and pre-trained models, the affordability and accessibility are improving.
As an analyst, I'm concerned about the privacy aspect. How can we ensure user data is protected while leveraging ChatGPT?
Privacy is crucial, Daniel. When implementing ChatGPT, businesses must prioritize user data protection, follow privacy regulations, and ensure appropriate data anonymization. Transparency with users about data usage is essential as well.
I can see the value of ChatGPT in engaging users, but what happens if it provides inaccurate recommendations?
Good question, Hannah. It's important to have fallback mechanisms in place when ChatGPT may not generate accurate recommendations. These fallbacks can include a mix of traditional algorithms, human reviews, or automated checks to ensure the best possible recommendations.
Are there any ethical concerns to consider when implementing ChatGPT for product recommendations?
Absolutely, Mark. Ethical considerations include addressing biases present in the training data, being transparent about algorithmic decision-making, and avoiding manipulation or exploitation of user preferences. Fairness and accountability are key aspects to keep in mind.
I'm impressed by the potential of ChatGPT, but what use case scenarios can benefit the most from this approach?
Good question, Rachel. ChatGPT can be particularly beneficial in scenarios where user preferences are complex or require more detailed exploration. Examples include fashion and style recommendations, personalized services, and domains where interactive conversations enhance the overall experience.
Considering the current limitations of ChatGPT, what are the areas where further research is needed?
Thanks for the question, John. Further research is needed to improve handling of ambiguous queries, reducing bias in recommendations, developing more efficient training techniques, and enabling smoother integration with existing recommendation systems.
How can businesses measure the success of implementing ChatGPT for product recommendations?
Measuring success can involve monitoring metrics like customer satisfaction, conversion rates, average order value, or user engagement. A/B testing and user surveys can provide valuable insights to gauge the impact of ChatGPT on product recommendations.
Would you recommend starting with a small pilot implementation of ChatGPT before scaling it up across all products?
Absolutely, David. Starting with a small pilot allows businesses to assess the real impact, identify challenges, and get user feedback. It provides an opportunity to refine the implementation and address any concerns before scaling across the entire product range.
ChatGPT seems like a powerful tool, but what resources would you recommend for analysts to learn more about its implementation?
Great question, Olivia. OpenAI's documentation and guides are excellent resources to start with. Additionally, exploring relevant research papers, attending industry conferences, and connecting with experts in the field can provide valuable insights and learning opportunities.
How can businesses strike the right balance between automation and human intervention when using ChatGPT for recommendations?
Finding the right balance is crucial, Alex. Businesses need to define clear guidelines for when human intervention is necessary, establishing review processes, and ensuring adequate training for analysts to handle complex cases. This ensures the best of both automated recommendations and human expertise.
Do you see ChatGPT as a supplement to existing recommendation algorithms or a potential replacement?
Great question, Sophia. While ChatGPT adds a powerful interactive element, it can serve as both a supplement and a potential replacement depending on the business objectives and the suitability of the application. A hybrid approach that combines different types of algorithms might be the best choice in many cases.
How can ChatGPT handle user inquiries on out-of-stock items or product discontinuations?
Good question, Andrew. ChatGPT can be trained to handle such scenarios by providing alternative recommendations, suggesting similar items, or referring users to customer support for further assistance. Ensuring a seamless user experience even in such cases is important.
What level of technical expertise is required for business analysts to work effectively with ChatGPT?
Business analysts should have a solid understanding of natural language processing and machine learning concepts. Familiarity with programming, data analysis, and experience with recommendation systems would be beneficial. Collaborating with data scientists and engineers can also support effective utilization of ChatGPT.
What are the potential bottlenecks or limitations when implementing ChatGPT in a production environment?
Good question, Sophie. Some potential bottlenecks include response latency due to model inference time, managing resource-intensive computations during high user loads, and ensuring effective handling of edge cases. Deployment infrastructure and continuous monitoring are essential to address any limitations.
Are there any restrictions or licensing requirements for businesses to use ChatGPT for product recommendations?
While OpenAI has specific usage policies and licensing agreements, it's essential to review and comply with them. For commercial use, OpenAI offers the ChatGPT API, which businesses can integrate following the provided guidelines for licensing and usage restrictions.
What's your take on potential user resistance to interacting with a chatbot for product recommendations?
User resistance can be a challenge, Susan. Ensuring a seamless and conversational user experience without feeling forced or intrusive is important. Transparency about the chatbot nature and the value it brings, combined with an option to switch to a human agent if desired, can help address user resistance.
What is the typical time frame required to implement ChatGPT for product recommendations in an existing system?
The implementation time frame can vary based on various factors such as system complexity, data availability, resource allocation, and the level of integration desired. It can take weeks to months, involving initial setup, training, fine-tuning, and iterative improvements based on performance analysis.
Would integrating ChatGPT for recommendations affect the overall system performance or response times?
Integrating ChatGPT can introduce additional computations that might impact response times, Michael. However, optimizing the deployment infrastructure, employing efficient model architectures, and parallelizing computations can help mitigate any potential performance or latency issues.
Can ChatGPT be adapted to work with multiple languages to cater to a diverse user base?
Absolutely, Lisa! ChatGPT can be adapted to support multiple languages, allowing businesses to cater to a broader user base. Training the model on diverse multilingual data and carefully considering language-specific nuances can enable effective cross-language recommendations.
In an evolving marketplace, how can businesses ensure that ChatGPT-based recommendations remain up-to-date and aligned with current trends?
Staying up-to-date is important, Eric. Continuous monitoring of recommendations, feedback collection from users, and incorporating new data regularly can help ensure alignment with current trends. Regular retraining and model updates become crucial to adapt to evolving market dynamics.
Thank you all for participating in the discussion! Your questions and insights have been valuable. Feel free to reach out if you have any further queries or thoughts. Have a great day!