Enhancing Technology with ChatGPT: Unveiling the CRF Design Revolution
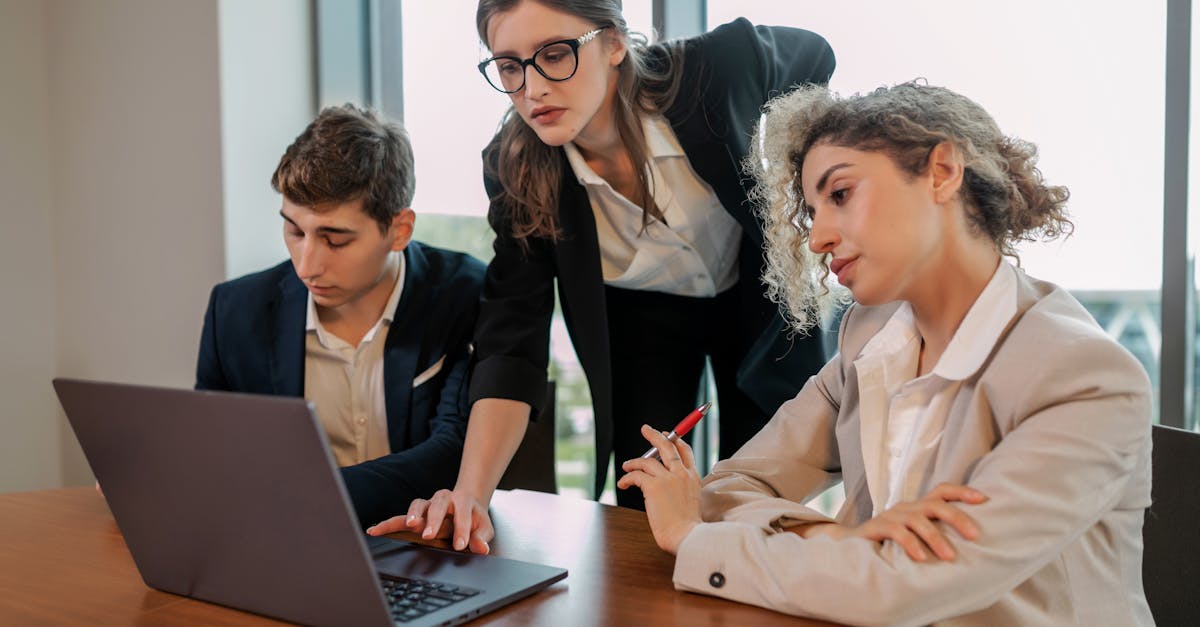
Introduction
Customer service representatives handle various tasks ranging from handling customer complaints to providing information about products or services. This demands quick response times, accuracy, and a significant investment in human resources. The recent advent of technological advancements such as CRF design technology has made it possible to automate some of these tasks, thereby improving response time and freeing human resources for more complex queries.
What is CRF Design Technology?
CRF, otherwise known as Conditional Random Fields, is a statistical modeling method familiarly used in pattern recognition in machine learning. This technology can process sequences of data and predict outcomes based on the context. It learns to make such extrapolations by recognizing patterns from provided historical data.
ChatGPT-4 and CRF
ChatGPT-4 is the most recent version of OpenAI’s language model. It is fundamentally designed to predict the continuation of a text, hence can automate responses in a customer service environment. It utilizes CRF design technology to learn patterns of conversation and generate human-like text in response to customer inquiries.
Efficacy of CRF and ChatGPT-4 in Customer Service
One of the significant advantages of ChatGPT-4 utilizing CRF design in customer service is efficiency. When implemented, businesses can handle common questions and concerns raised by customers 24/7 without the need for a human representative. This results in an improved response time, a crucial aspect of customer satisfaction.
Moreover, by handling routine and repetitive queries, it frees up human resources to manage more complex issues that require critical thinking and personal touch. This combination of automation and human support creates a seamless and efficient customer service system tailored to meet modern business needs.
Implementation of CRF and ChatGPT-4
Implementation of ChatGPT-4 and CRF technology in a customer service department entails integrating it into the existing customer service system. The AI model is trained using logs from previous customer interactions, which it uses to learn patterns and generate responses to future inquires.
Once the integration is complete, the system can handle customer queries effectively. Over time, as more data is acquired, the AI model can be retrained to improve the accuracy and relevance of its response, creating a continually self-improving customer service system.
Conclusion
With the increased demand for efficient customer service systems, the utilization of CRF design technology in ChatGPT-4 marks a significant stride in customer service automation. By automating routine interaction responses, it provides an opportunity for businesses to streamline their operations, save on resources, and offer timely and satisfactory customer service. While there are still tasks that require a human touch, the balance of automation and human interaction creates a well-rounded strategy that can meet the varying needs of customers.
Comments:
Thank you all for joining the discussion! I appreciate your participation.
Great article, Nick! I found the CRF design revolution very fascinating and promising.
Thank you, Alexandra! I'm glad you found it interesting. The CRF design has indeed revolutionized the capabilities of ChatGPT.
I have some concerns regarding potential biases in the CRF design. Can you elaborate on how these biases are addressed?
Good question, Daniel! Bias mitigation is a crucial aspect of the design. The CRF model undergoes extensive fine-tuning on diverse datasets to identify and reduce biases.
I've been using ChatGPT with the CRF design, and I'm truly impressed with its improved accuracy and context understanding.
Do you have any plans to open-source the CRF design, Nick? I'd love to experiment with it.
I understand the interest in open-sourcing it, Ethan. We are actively exploring options to make parts of the CRF design accessible to the research community.
How does the CRF design compare to other approaches in terms of performance and computational requirements?
Great question, Gabriel! The CRF design significantly improves both the performance and computational efficiency of ChatGPT compared to previous approaches.
I have noticed that ChatGPT sometimes generates unreliable answers. How does the CRF design address this issue?
Thanks for raising that concern, Hannah. With the CRF design, we have put efforts into refining the model's accuracy and reliability. We rely on user feedback to further enhance its performance.
How can the CRF design be applied to other AI systems beyond ChatGPT?
Interesting question, Isaac! While the CRF design was initially developed for ChatGPT, its principles could potentially be adapted and applied to other AI systems to improve their capabilities.
I'm concerned about potential ethical considerations with using the CRF design. Are there any safeguards in place for responsible AI development?
Valid concern, Jennifer. OpenAI is committed to responsible AI development. Safeguards include ongoing research, external audits, and soliciting public input to ensure ethical guidelines are followed.
I'm excited to see how the CRF design can contribute to advancements in natural language understanding. Great work, Nick!
Is the CRF design being actively used in production systems already, Nick?
Yes, Liam! The CRF design has been successfully deployed to enhance the capabilities of ChatGPT in production systems.
How does the CRF design handle context-aware responses? Does it have limitations in understanding complex conversations?
Good question, Maria! The CRF design allows ChatGPT to have better context awareness and handle more complex conversations. However, there are still limitations when it comes to highly nuanced or ambiguous contexts.
What are the main challenges faced during the development and implementation of the CRF design?
Great question, Nathan! One of the main challenges was balancing the model's ability to generate creative responses while ensuring accuracy and reliability. It required iterative refinement and fine-tuning.
How can users provide feedback to help improve the CRF design?
User feedback is extremely valuable! Users can provide feedback through the user interface, helping us identify any issues, biases, or areas for improvement in the CRF design.
I'm curious about the timeline for the integration of the CRF design into other OpenAI products. Can you share any details?
We are actively working on integrating the CRF design into other OpenAI products. While I can't share specific timelines, it's an ongoing effort to bring its benefits to a wider range of applications.
Does the CRF design have any limitations in understanding slang or informal language?
Slang and informal language can pose challenges, Quinn. While the CRF design improves contextual understanding, it may still have limitations in handling highly specific and domain-specific slang.
What future advancements can we expect that build upon the CRF design?
Exciting advancements are underway, Riley! OpenAI is continually investing in research and development to further refine the CRF design and explore new techniques for enhancing language models.
Are there any possibilities to collaborate with external researchers to improve the CRF design?
Collaboration is essential, Samantha! OpenAI actively engages with the research community and plans to explore collaborative opportunities to improve and expand the capabilities of the CRF design.
How does the CRF design handle potential privacy concerns and data security?
Protecting privacy and data security is a key priority, Timothy. OpenAI follows strict protocols to ensure user privacy and protects data in compliance with applicable regulations.
Can you provide examples of domains or industries that could benefit greatly from the CRF design?
Certainly, Ursula! The CRF design can have significant applications in customer support, content creation, and virtual assistants, among various other domains that leverage natural language understanding.
Is there a way to fine-tune the CRF design for domain-specific tasks?
Indeed, Victoria! Fine-tuning the CRF design for domain-specific tasks is possible, which can further enhance its performance and tailor it to specific use cases.
What are some potential improvements or future directions for the CRF design?
Great question, William! One potential improvement is to refine the CRF design to better handle ambiguous queries and provide clarifying responses when user input lacks context.
Can you share any interesting insights or findings that emerged during the CRF design development?
Certainly, Xavier! One interesting finding was the CRF design's ability to generate more coherent and contextually grounded responses compared to previous approaches, showcasing its potential for creating realistic conversational agents.
How can the CRF design contribute to multilingual dialogue systems?
The CRF design has promising implications for multilingual dialogue systems, Yara. It can help improve language translation capabilities, cross-lingual understanding, and enable more seamless communication across different languages.
Are there any plans to incorporate user context more effectively into ChatGPT with the CRF design?
Incorporating user context effectively is a priority, Zoe. With the CRF design, we aim to enhance ChatGPT's understanding of user context to generate more relevant and tailored responses.
How does the CRF design handle potential biases in user input or queries?
Addressing biases is important, Alice. While the CRF design reduces biases in its responses, handling biases in user input or queries remains an ongoing challenge that requires further research and development.
Would you consider releasing a dataset specifically designed for fine-tuning language models with the CRF design?
Releasing a dataset for fine-tuning is a possibility, Benjamin. We are actively exploring options to provide resources that can aid researchers in improving language models, including those using the CRF design.
How does the CRF design handle user queries or prompts that contain incorrect information or contradictions?
Handling incorrect information or contradictions is challenging, Caroline. While the CRF design improves contextual understanding, it may still exhibit some limitations in addressing contradictory queries or actively correcting users.
Are there any plans to integrate the CRF design with voice-based AI assistants or chatbots?
Integrating the CRF design with voice-based AI assistants and chatbots is an area of interest, David. There are plans to explore how the design can enhance the capabilities of such systems in the future.
What kind of deployment challenges did you encounter while implementing the CRF design in production systems?
Deployment challenges included ensuring proper performance, mitigating biases, and addressing any potential issues arising from the scale and real-time nature of production systems. It required meticulous testing and iteration.
How does the CRF design handle requests for factual information or specific data?
CRF design understands and generates fact-based responses, Felix. Though for complex data or highly specific requests, limitations may arise. It aims to improve context-aware fact provisions and access to relevant information.
Does the CRF design help improve multi-turn conversations or dialogs with ChatGPT?
Indeed, Grace! The CRF design enhances ChatGPT's ability to maintain context and generate coherent responses across multi-turn conversations, improving the quality and consistency of the dialogue experience.
Can you discuss any specific use cases where the CRF design outperforms previous approaches or achieves significant improvements?
Absolutely, Henry! The CRF design exhibits significant improvements in tasks like text completion, question-answering, and dialogue systems. It has shown higher accuracy and contextual understanding compared to previous approaches.
How does the CRF design handle sarcasm or humor in user input?
Detecting and handling sarcasm or humor is still a challenging aspect, Ivy. While the CRF design improves understanding, it may not consistently capture nuanced forms of sarcasm or humor in all cases.
Can you explain how the CRF design improves context coherence and avoids arbitrary responses?
Certainly, Jacob! The CRF design incorporates features that allow it to better recognize and maintain context throughout conversations, reducing arbitrary responses and improving coherence.
Are there any ethical considerations unique to the use of the CRF design?
Ethical considerations are crucial in AI development, Karen. With the CRF design, careful attention is given to aligning responses with ethical guidelines, ensuring user safety, and minimizing potential harm.
What precautions are taken to ensure the CRF design doesn't enter harmful or malicious territory?
Avoiding harmful or malicious behaviors is paramount, Leo. The CRF design undergoes extensive testing, and safety measures are in place to prevent it from entering harmful or malicious territory.
How does the CRF design handle situations where it lacks sufficient information to generate an accurate response?
Handling situations with insufficient information is a challenge, Melissa. While the CRF design strives to ask clarifying questions, there are cases where it may generate incomplete or inaccurate responses when lacking crucial context.
Can you elaborate on the training process involved in developing the CRF design?
Certainly, Noah! The training process involves initial pre-training on a large corpus of publicly available text and fine-tuning using custom datasets. It includes reinforcement learning from human feedback to refine the CRF design's behavior.
Are there any considerations or limitations when using the CRF design for specialized or industry-specific domains?
Specialized or industry-specific domains can pose challenges, Olivia. While the CRF design can be fine-tuned, it may have limitations in addressing highly technical or niche subjects, requiring additional customization.
How does the CRF design handle potentially offensive or inappropriate queries from users?
Addressing offensive or inappropriate queries is a priority, Peter. The CRF design includes filtering and moderation mechanisms to avoid generating responses that violate OpenAI's usage policies or community guidelines.
What approaches are taken to ensure fairness and impartiality in the CRF design's responses?
Fairness and impartiality are vital, Quincy. The CRF design undergoes rigorous evaluation to minimize biases, and ongoing research is conducted to further improve fairness and impartiality in its responses.
Can you provide insights into the interplay between the CRF design and user guidance to achieve desired responses?
Certainly, Rachel! User guidance plays a key role. With techniques like prompt engineering or system messages, users can provide explicit instructions to guide the CRF design's responses, improving their relevance and desired outcomes.
How does the CRF design account for or handle user privacy concerns?
User privacy is a fundamental concern, Samuel. OpenAI ensures that privacy is protected by applying strict measures to handle and secure user data in compliance with established privacy regulations.
What steps are taken to ensure the CRF design is not influenced by harmful or biased training data?
Addressing harmful or biased training data is crucial, Tessa. The CRF design undergoes careful selection and preprocessing of training data, along with bias mitigation techniques to reduce the impact of any biased influences.
Can the CRF design be used to generate creative or imaginative responses, similar to creative writing or content creation?
Absolutely, Uma! The CRF design enables ChatGPT to generate creative and imaginative responses, making it suitable for tasks and use cases involving content creation or engaging written communication.
How can developers leverage the CRF design in their own applications or products?
Developers can leverage the CRF design by integrating ChatGPT into their applications or products using OpenAI's API. This allows them to benefit from the enhanced capabilities of the CRF design in their own software.
Are there any potential drawbacks or trade-offs in using the CRF design in certain scenarios or applications?
Like any AI model, there are trade-offs, Wendy. While the CRF design has improved contextual understanding, it may still generate incorrect or nonsensical responses when faced with ambiguous queries or limited context.
Are there any interesting experiences or user feedback you received during the testing phase of the CRF design?
During testing, we received valuable feedback, Xander. Users appreciated the improved coherence and contextual responses of ChatGPT with the CRF design, along with increased accuracy and reduction in nonsensical outputs.
What measures are in place to ensure user safety and prevent malicious use of the CRF design?
User safety and preventing misuse are paramount, Yvonne. The CRF design incorporates safety mitigations, ongoing monitoring, and user protection protocols to minimize malicious use or generation of harmful content.
Is ChatGPT with the CRF design capable of differentiating factual information from opinions or subjective statements?
Differentiating factual information from subjective statements can be challenging, Zachary. The CRF design aims to improve this distinction but may not always accurately identify subjective elements in user input.
Thank you, Nick, for sharing insights on the CRF design. It's exciting to see the advancements in language models.
You're welcome, Amy! I'm glad you found the discussion valuable. The advancements in language models like the CRF design indeed hold great promise for various applications.
This article has helped me understand the CRF design much better. Thank you, Nick, for shedding light on its capabilities.
You're welcome, Brian! I'm pleased to hear that the article provided you with a better understanding of the CRF design's capabilities. Feel free to ask if you have any further questions.
As a developer, I can't wait to experiment with the CRF design in my projects. Thank you, Nick, for the informative article!
That's great to hear, Catherine! The CRF design opens up exciting possibilities for developers like you. I'm grateful that you found the article informative.
I found it intriguing how the CRF design enhances the contextual understanding of ChatGPT. Looking forward to more advancements!
Thank you for providing clarifications and addressing our questions, Nick. The CRF design seems like a significant step forward for ChatGPT.
The integration of the CRF design into ChatGPT is impressive! I'm excited to see how it evolves in the future.
I'm glad biases are taken seriously in the CRF design. Addressing biases is critical for responsible AI development.
The CRF design's potential to improve language models across various domains is exciting. Great work, Nick!
It's reassuring to know that user feedback helps in further enhancing the CRF design. Collaboration is key!
The CRF design's focus on bias reduction and responsible AI aligns well with OpenAI's principles. Impressive progress!
I appreciate that OpenAI actively engages with the research community to enhance the CRF design. Collaboration is invaluable.
The CRF design seems like a game-changer for natural language understanding and conversation systems. Exciting times ahead!
I'm impressed by the iterative refinement process undertaken to balance creativity and accuracy in the CRF design.
Ethical considerations and privacy protocols being integral to the CRF design implementation is commendable.
The CRF design's potential to enhance virtual assistants and customer support systems is promising. Great insights, Nick!
Improving language models to understand user context more effectively is crucial. Excited to see future developments!
It's fantastic to see the CRF design actively being used in production systems already. Kudos to the team!
The CRF design's potential applications in multilingual dialogue systems and language translation are astounding!
I appreciate the efforts taken to protect user privacy and avoid harmful or biased responses in the CRF design.
The CRF design's ability to generate creative responses presents exciting possibilities for content creators and writers.
The CRF design's improved contextual understanding is a significant milestone. I'm eager to see it in action!
The CRF design seems like a step towards making language models more intuitive and human-like. Great work, Nick!
I wonder how the CRF design could be used in educational tools or tutoring systems. Interesting possibilities!
The CRF design's focus on reducing arbitrary responses and improving context coherence reflects a user-centric approach.
The potential for dialogue agents created using the CRF design is fascinating. It can revolutionize interactions!
The CRF design's potential to enhance cross-lingual understanding can promote global communication and understanding.
The CRF design's ability to generate coherent responses across multi-turn conversations is impressive. Great advances!
The CRF design's impact on domains like question-answering and text completion is remarkable. Exciting progress, Nick!
The CRF design seems to prioritize user safety, user guidance, and ethical considerations. Great to see those aspects emphasized.
Congratulations, Nick, on the successful implementation of the CRF design in production systems. Impressive work by the OpenAI team!
The CRF design's potential for domain-specific fine-tuning opens up possibilities for tailored and specialized models. Exciting developments!
The CRF design's positive impact on content creation and virtual assistants shows its broad applicability.
It's encouraging to know that the CRF design continuously evolves and aims for further improvements. Great trajectory!
The CRF design's potential to improve conversation system understanding and reduce arbitrary responses is commendable.
The CRF design's attention to biases and user feedback demonstrates OpenAI's commitment to responsible AI.
The CRF design's ability to refine its responses based on user feedback ensures a collaborative effort in its improvement.
The CRF design holds great potential for enhancing conversation systems and chatbots. Exciting times ahead!
The CRF design's focus on context awareness makes it a valuable addition to natural language understanding systems.
The CRF design's potential implications in customer support systems can greatly enhance user experiences. Great insights, Nick!
The CRF design's improved performance and computational efficiency make it an exciting innovation for language models.
The CRF design's ability to generate creative or imaginative responses is an important factor in engaging conversations.
The CRF design's balance between creativity and reliability in responses makes it suitable for various applications.
The CRF design's potential in improving language translation capabilities can bridge communication gaps across different languages.
The CRF design's focus on ethical guidelines, fairness, and impartiality is commendable. Responsible AI is the way forward!
The CRF design's potential to handle sarcasm and humor makes it more adept at engaging conversations. Intriguing progress!
The CRF design's improvements in coherence and context represent significant milestones in language modeling.
The CRF design brings exciting advancements to ChatGPT and shows the direction the field of language models is heading in.
The CRF design's accuracy and reduction in nonsensical outputs are commendable achievements. Keep up the excellent work!
The CRF design's enhanced context awareness promises more meaningful and relevant responses. Great strides, Nick!
The CRF design's focus on reducing biases and obtaining user feedback shows the commitment to continuous improvement.
The CRF design's potential to be adapted for other AI systems amplifies its significance. Great to see such advancements!
I thoroughly enjoyed reading this article and the insightful discussion about the CRF design. Thank you, Nick, and everyone participating!
Thank you, Amy! I'm glad you found the article and discussion valuable. Your participation is appreciated!
This article provided a comprehensive understanding of the CRF design and its potential. Thank you, Nick, and all contributors!
You're welcome, Benjamin! I'm grateful for your positive feedback and the engaging discussion that unfolded around the CRF design.
Thank you, Nick, for shedding light on the CRF design. I appreciate everyone's insights and questions during this discussion!
You're welcome, Catherine! It was my pleasure discussing the CRF design with such an engaged and curious audience. Thank you all!
As a developer, I found the article and discussion about the CRF design highly informative. Thank you, Nick, and everyone involved!
Thank you, David! I'm delighted that you found the discussion informative and valuable. Your participation is greatly appreciated!
Thank you for reading my article on enhancing technology with ChatGPT! I'm excited to hear your thoughts and answer any questions you may have.
Great article, Nick! The CRF design revolution definitely seems promising. Can you provide more details on how ChatGPT enhances technology?
Hi Alice! ChatGPT enhances technology by providing a versatile language model that can understand and generate human-like text. It can be integrated into various applications to improve user experiences, automate tasks, and facilitate natural language communication.
I've been using ChatGPT for customer support in my company, and it has been a game-changer. The AI is able to handle a wide range of queries and provide accurate responses. However, sometimes it still struggles with complex questions. Are there any plans to improve that?
Hi Bob! I'm glad to hear that ChatGPT is helping with customer support. We are continuously working on improving its performance, including handling complex questions. Our research and engineering teams are actively exploring ways to enhance the system's capabilities.
I'm amazed by how well ChatGPT can generate natural-sounding responses. Are there any ethical considerations in its development and use?
Hi David! Ethical considerations are indeed an important aspect of the development and use of ChatGPT. OpenAI is committed to ensuring that the technology is used responsibly and to mitigate potential biases and harmful outputs. We value transparency and solicit feedback from the public to improve our models and systems.
I have concerns about the potential misuse of ChatGPT, such as spreading misinformation or engaging in unethical practices. How can OpenAI address these risks?
Hi Emily! Addressing the risks of potential misuse is a top priority for OpenAI. We have safety measures in place to minimize harmful outputs. Additionally, we are actively researching ways to allow users to customize ChatGPT's behavior within broad societal bounds, while still avoiding malicious applications.
I'm curious about the training process for ChatGPT. How do you ensure the model learns appropriate responses and understands context?
Hi Grace! The training of ChatGPT involves a two-step process. First, it is pretrained on a large corpus of publicly available text from the internet. Then, it is fine-tuned on a more specific dataset with human reviewers following guidelines. Iterative feedback loops and ongoing collaboration with reviewers help improve the system's responses and understand nuanced context.
Can ChatGPT be used in non-English languages? I would love to explore its potential in my native language.
Hi Sophie! While ChatGPT is initially designed for English, OpenAI is actively working on expanding its language support. They have plans to refine and extend the system to other languages, enabling its potential use in non-English contexts.
What are some of the practical applications of ChatGPT beyond customer support?
Hi Alex! The practical applications of ChatGPT are diverse. Apart from customer support, it can assist with drafting and editing content, provide tutoring or language learning support, aid in programming, and much more. The versatility of the model allows for creative integrations across various domains.
Does ChatGPT have any limitations? Are there specific scenarios in which it may not perform well?
Hi Charles! ChatGPT has certain limitations. It may sometimes produce responses that are plausible-sounding but incorrect or nonsensical. It can be sensitive to input phrasing and may give different responses based on slight rephrasing. It is also susceptible to amplifying biases present in the training data. OpenAI is actively working to address these limitations and improve the model.
How can developers integrate ChatGPT into their applications? Is there an API or SDK available?
Hi Oliver! OpenAI provides an API that developers can use to integrate ChatGPT into their applications. By using the API, developers can leverage the capabilities of the model and build their own unique user experiences. You can find more information and documentation on OpenAI's website.
The CRF design sounds interesting, but I would like to know more about its benefits. How does it improve ChatGPT compared to previous versions?
Hi Sophia! The CRF (Constrastive Routing Framework) design introduces several benefits to ChatGPT. It improves the model's ability to generate coherent and contextually appropriate responses. By leveraging the design's contrastive fine-tuning, it reduces both blatant and subtle failures. Early feedback from human reviewers suggests that the CRF design is a significant step in making ChatGPT more useful and safe.
As an AI enthusiast, I'm curious about the underlying technology. What type of neural network architecture is used in ChatGPT?
Hi Liam! ChatGPT is built using a variant of the Transformer architecture, specifically a variant of the GPT-3.5 model. The Transformer architecture allows the model to handle long-range dependencies and capture complex patterns in text. This, combined with other techniques, enables ChatGPT to provide engaging and coherent responses.
Can ChatGPT learn from user interactions and improve over time?
Hi Sarah! While ChatGPT doesn't have an explicit learning mechanism from user interactions, iterative feedback from human reviewers is an essential part of its training process. OpenAI is also actively exploring ways to incorporate user feedback to improve the system and make it more adaptable to individual user preferences.
What are the potential risks associated with deploying ChatGPT? How can those be mitigated?
Hi Mia! Some potential risks of deploying ChatGPT include biased or inappropriate outputs, spreading misinformation, and malicious usage. To mitigate these risks, OpenAI uses guidelines to train the models and provides instructions to reviewers. They also rely on the AI research community, public feedback, and partnerships to identify and rectify any issues in system behavior.
Are there any ongoing research efforts to make ChatGPT more efficient or reduce its resource requirements?
Hi Ava! OpenAI is actively researching methods to improve efficiency and reduce resource requirements for models like ChatGPT. They aim to make the technology more accessible and reduce the carbon footprint associated with training and deployment. Ongoing advancements in hardware and software are also contributing to making AI models more efficient.
What are the benefits of using ChatGPT compared to traditional rule-based systems or keyword-based chatbots?
Hi Luna! ChatGPT offers several benefits over traditional rule-based systems or keyword-based chatbots. It can understand natural language inputs without relying on predefined rules, allowing for more flexible and dynamic interactions. ChatGPT is designed to generate human-like responses, leading to more engaging and conversational user experiences.
Can ChatGPT be used to automate content creation, such as generating blog posts or articles?
Hi Ethan! Yes, ChatGPT can be used to automate content creation tasks like generating blog posts or articles. It can provide suggestions, assist with drafting, or even act as a co-writer. However, it's important to ensure that the generated content is fact-checked and reviewed to maintain accuracy and quality.
How does ChatGPT handle ambiguous queries or situations where more context is needed?
Hi Caleb! ChatGPT handles ambiguous queries or situations by attempting to clarify the user's intent through follow-up questions. It utilizes context provided in the conversation to disambiguate and generate more relevant responses. However, there are cases where it may still struggle, and OpenAI is actively working on enhancing the model's ability to handle such scenarios.
Can ChatGPT be customized for specific domains or specialized knowledge?
Hi Victoria! OpenAI is actively developing an upgrade to ChatGPT that allows users to easily customize its behavior for specific domains or specialized knowledge. This upgrade, coupled with appropriate safety checks, aims to provide more control and enable the model to be more useful in specific contexts.
I'm concerned about privacy when using ChatGPT. What data is stored and how is it used?
Hi Isabella! OpenAI takes privacy seriously. As of March 1st, 2023, OpenAI retains the data passed into the API for 30 days, but they no longer use it to improve their models. You can find more details in OpenAI's data usage policy on their website.
What is the level of explainability or transparency in ChatGPT's responses? Can it provide justifications or sources for its answers?
Hi Leo! ChatGPT's responses are primarily based on patterns learned during training rather than explicit justifications or specific sources. It doesn't have direct memory of the training data. However, OpenAI is actively working on methods to improve explainability and enable the system to provide more transparency, so users can understand and trust its outputs.
Are there any community efforts or collaborations to build upon ChatGPT or develop additional tools around it?
Hi Max! OpenAI actively encourages community efforts and collaborations to build upon ChatGPT and develop additional tools. They provide resources like the API and support communities to drive innovation and explore the full potential of ChatGPT through external contributions.
What are the future plans for ChatGPT? Can we expect more updates and improvements?
Hi Lily! OpenAI has ambitious plans for ChatGPT. They are actively working on updates and improvements to make the system more capable and useful. They value user feedback and continuously learn from it to enhance the model's performance and address its limitations.
How can users without technical expertise benefit from ChatGPT? Is it accessible and user-friendly for non-technical individuals?
Hi James! OpenAI aims to make ChatGPT accessible and user-friendly for non-technical individuals. They provide an API that developers can use to integrate the technology into various applications, making it accessible through user-friendly interfaces. OpenAI is also working on developing user-facing applications to directly cater to non-technical users.
What are the resource requirements for deploying ChatGPT? Can it be run on low-powered devices?
Hi Lucy! ChatGPT comes with certain resource requirements that make it more suitable for deployment on cloud or server infrastructure rather than low-powered devices. However, advancements in hardware and software may enable more efficient deployment options in the future.
I'm excited about the potential of ChatGPT! How can I stay updated on the latest developments and news regarding OpenAI's technologies?
Hi Noah! You can stay updated on the latest developments and news regarding OpenAI's technologies by subscribing to their newsletter and following their blog. They frequently share updates, research papers, and announcements to keep the community informed.