Enhancing Wireless Networking with ChatGPT: Revolutionizing RF Technology.
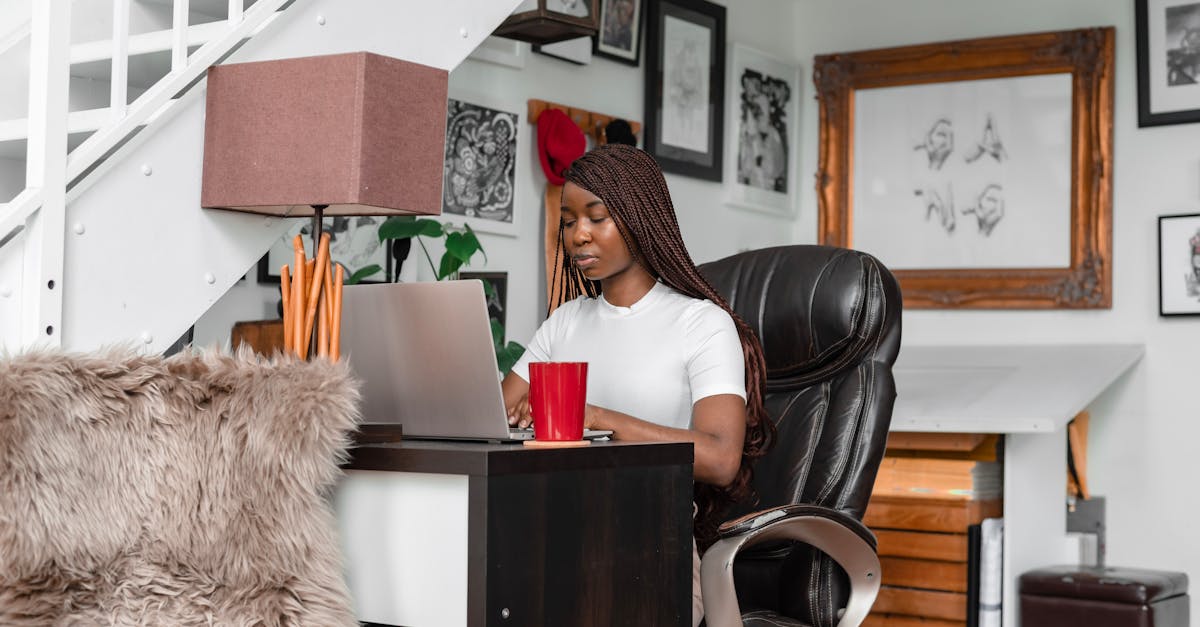
Wireless networking has become an integral part of our daily lives, enabling us to stay connected and access information on the go. One of the key technologies that powers wireless networks is RF (Radio Frequency). RF technology is used to transmit and receive signals wirelessly, allowing devices to communicate with each other without the need for physical connections.
Setting up and optimizing RF-based wireless networks can be a complex task. Factors such as interference, signal strength, and channel allocation need to be carefully considered to ensure optimal performance. This is where Artificial Intelligence (AI) can play a significant role.
1. AI-assisted Network Planning and Design
AI can aid in the network planning and design phase by analyzing various parameters such as building layout, signal propagation characteristics, and user density. By considering these factors, AI algorithms can determine the optimal placement of access points and antennas for maximum coverage and minimized interference.
Furthermore, AI can utilize machine learning techniques to predict the performance of the network under different scenarios. This allows network administrators to make informed decisions regarding hardware selection, channel allocation, and power settings to optimize network performance.
2. AI-based Spectrum Management
In RF-based wireless networks, the radio spectrum is a valuable and limited resource. Efficient spectrum management is crucial to avoid interference and ensure reliable communication. AI algorithms can analyze the spectrum usage patterns in real-time and dynamically allocate channels to different devices and applications based on their bandwidth requirements and interference levels.
Moreover, AI can adaptively adjust the transmission power levels of devices to minimize interference and optimize power consumption. By continuously monitoring the network conditions, AI can intelligently allocate spectrum resources to ensure seamless and reliable connectivity.
3. AI-driven Network Optimization and Self-healing
A wireless network's performance can degrade over time due to changes in the environment, user behavior, or interference sources. AI-driven network optimization can dynamically adapt to these changes and self-optimize the network parameters to maintain optimal performance.
AI algorithms can continuously monitor network performance metrics, such as signal strength, throughput, and latency. They can adjust settings like channel allocation, transmission power, and access point configurations to optimize network performance in real-time.
In addition, AI can identify and mitigate sources of interference or network congestion by analyzing the RF environment. This self-healing capability minimizes manual intervention and reduces network downtime, ensuring a seamless and reliable wireless experience.
4. AI-powered Predictive Maintenance
Maintaining the operational health of a wireless network is crucial to ensure uninterrupted connectivity. AI can utilize predictive analytics to detect potential network issues and proactively perform maintenance tasks.
By analyzing historical network performance data and applying machine learning algorithms, AI can identify patterns that may lead to future network failures. It can then notify network administrators in advance, enabling them to take corrective actions to prevent service disruptions.
Furthermore, AI can analyze network logs and perform automated troubleshooting to quickly identify and resolve network issues. This proactive approach to maintenance minimizes network downtime and improves overall network reliability.
Conclusion
The role of AI in setting up and optimizing RF-based wireless networks is becoming increasingly important. By leveraging AI-driven network planning, spectrum management, optimization, and predictive maintenance techniques, network administrators can ensure maximum network performance, reliability, and user satisfaction.
As wireless networks continue to evolve and become more complex, AI will play a pivotal role in simplifying network management and driving advancements in RF technology.
Comments:
This article on enhancing wireless networking with ChatGPT is fascinating! The potential to revolutionize RF technology is incredible.
I agree, Sam! The advancements in AI technology are truly exciting. I can't wait to see how it transforms wireless networking.
Thank you, Sam and Emily! I'm glad you find the article fascinating. The potential for ChatGPT to enhance RF technology is indeed quite promising.
As a professional in the wireless networking industry, I'm always interested in new technologies. ChatGPT seems very promising, but I wonder about its practical implementation. Thoughts?
Mark, you bring up an important point. The practical implementation of ChatGPT in RF technology requires extensive testing and validation to address potential challenges. We are actively working on ensuring its real-world effectiveness.
I understand your concern, Mark. While ChatGPT shows great potential, practical implementation could face challenges. It's important to ensure it works flawlessly in real-world scenarios.
The concept sounds exciting, but how does ChatGPT actually enhance RF technology? Can you provide some examples, Fred?
Absolutely, Ben! ChatGPT can assist in optimizing wireless networks, such as automatically adjusting signal frequencies, enabling smart routing decisions, and providing intelligent troubleshooting guidance. Its ability to understand complex RF challenges is where it excels.
This article raises concerns about job displacement. Will ChatGPT replace the need for human RF engineers in the future?
Sophia, while ChatGPT can assist and automate certain aspects of RF engineering tasks, it's important to note that human expertise will still be crucial. The technology aims to enhance the capabilities of engineers rather than replace them.
I'm curious how ChatGPT's performance compares to traditional methods in RF technology. Are there any studies or research articles that showcase its accuracy and effectiveness?
Daniel, excellent question! We have conducted extensive internal tests, and preliminary results show promising performance of ChatGPT in dealing with various RF challenges. We are also collaborating with industry experts for third-party validation.
Would ChatGPT be accessible to small-scale networking setups, or is it primarily targeted at large-scale infrastructure?
Great question, Alex! ChatGPT can be scaled to different networking setups and is not limited to large-scale infrastructure. Its flexibility allows it to adapt to various RF environments, serving both small and large deployments.
I'm concerned about the security implications of integrating AI like ChatGPT into wireless networks. Is there a risk of vulnerabilities or potential misuse?
Nadia, security is a valid concern. We are taking utmost care to ensure the safety and integrity of systems that incorporate ChatGPT. Implementing robust security practices and conducting thorough vulnerability assessments are among our primary focuses.
ChatGPT sounds promising, but how will it handle highly dynamic RF environments where conditions change rapidly and unpredictably?
Peter, addressing dynamic RF environments is a priority. ChatGPT is being designed to quickly adapt to changing conditions, leveraging real-time data and predictive modeling. It will have the ability to make rapid and informed decisions.
Are there any plans to make ChatGPT open-source? It could pave the way for collaborative development and improvements.
Olivia, we recognize the benefits of open-source collaboration. While we don't have immediate plans for complete open sourcing, we are exploring ways to engage with the community for valuable feedback and iterative improvements.
The article mentions revolutionizing RF technology, but is ChatGPT the only AI solution being explored in this domain, or are there other competing technologies as well?
Lucas, ChatGPT is not the only AI solution being explored. We are aware of other AI technologies, and we actively explore their potential. The emphasis is on leveraging the most effective tools available to enhance wireless networking.
I'm excited about the possibilities ChatGPT brings! How soon do you think we'll see its tangible benefits in the field of RF technology?
Grace, I'm glad you share the excitement! While I can't provide a specific timeline, we are diligently working on bringing ChatGPT's benefits to the field as soon as possible. Realizing tangible benefits will depend on successful testing, integration, and deployment.
The integration of AI raises ethical questions. How will ChatGPT ensure fairness and avoid bias in its decision-making processes?
Sarah, fairness and bias mitigation are key considerations. We are committed to rigorous assessment of ChatGPT's decision-making processes and implementing appropriate measures to ensure fairness, transparency, and accountability.
The possibilities of ChatGPT are exciting, but what kind of computational resources will be required to support its implementation in wireless networks?
Eric, computational resources are indeed a concern. While ChatGPT may have resource requirements, we are optimizing its implementation to be efficient and scalable. The aim is to minimize computational demands without compromising performance.
ChatGPT's potential to assist in intelligent troubleshooting sounds intriguing! Can you provide more insights into how it would work in practice, Fred?
Certainly, Amy! When it comes to intelligent troubleshooting, ChatGPT can analyze network logs and real-time data, identify issues, and suggest possible solutions. It can even guide engineers step-by-step through complex debugging procedures.
I'm curious if ChatGPT's recommendations could lead to novel RF strategies and approaches that human engineers might not have considered before.
Liam, that's an exciting possibility! ChatGPT has the potential to explore a vast search space and discover innovative RF strategies. It can augment human expertise by suggesting out-of-the-box approaches that could lead to groundbreaking advancements.
Will the use of ChatGPT in wireless networks require extensive retraining and constant updates to ensure its knowledge is up to date?
Eva, continuous learning is crucial for AI models. While ChatGPT will require updates to ensure it remains up to date with evolving technology, we are working on streamlining the retraining process and minimizing disruptions during deployment.
I'm concerned about potential vulnerabilities that malicious actors could exploit within ChatGPT's implementation. How will you address security threats, Fred?
James, security is paramount in any AI implementation. We are investing significant effort in identifying and mitigating potential vulnerabilities. Continuous security assessments and proactive measures against malicious exploitation will be put in place.
When ChatGPT makes recommendations in RF networks, will engineers have the final say, or will the system have autonomous control over network management?
Grace, excellent question! ChatGPT will provide recommendations, but the final decisions and control over network management will rest with engineers. It serves as a powerful tool and an intelligent collaborator, empowering human experts.
I'm glad to hear that human expertise will still be valued in conjunction with ChatGPT's capabilities. Collaboration between AI and humans is crucial for responsible development.
Sophia, you're absolutely right. Responsible and ethical development necessitates collaboration between AI and human experts. Together, we can leverage the transformative potential of technologies like ChatGPT while prioritizing human judgment and values.
Fred, I appreciate the transparent and informative responses you've provided to all our questions. It's great to have this discussion and gain deeper insights.
Emily, thank you for your kind words. This discussion with the community has been insightful, and I'm grateful for your engagement and curiosity. Let's continue exploring the exciting possibilities together!
Indeed, thank you, Fred. This conversation has shed light on the potentials and considerations surrounding ChatGPT in RF technology. I'm excited to witness its future impact.
Sam, I'm glad you found the conversation valuable. Thank you for your enthusiasm and support. I share your excitement for the future of ChatGPT in RF technology.