Exploring the Power of ChatGPT in Data Clustering: A Revolutionary Tool for Qualitative & Quantitative Research Methodologies
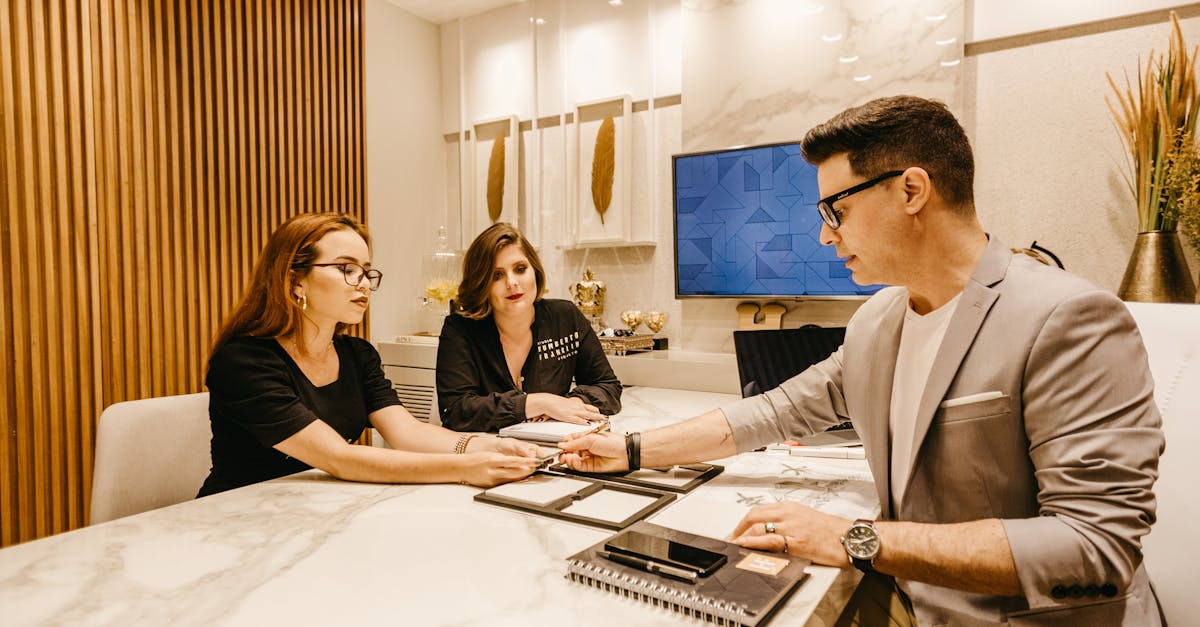
In the field of research, data analysis plays a crucial role in drawing meaningful insights and making informed decisions. With the advancements in technology, researchers now have access to powerful tools that can assist them in organizing and analyzing large amounts of data efficiently. One such tool is ChatGPT-4, which can be utilized in both quantitative and qualitative research to cluster similar data for easier analysis.
Quantitative Research Methodologies
Quantitative research involves collecting and analyzing numerical data to uncover patterns, trends, and relationships. Traditional quantitative research methodologies rely heavily on statistical techniques and require structured data for analysis. However, when dealing with unstructured data, such as open-ended survey responses or social media comments, it can be challenging to identify patterns or group similar data.
ChatGPT-4, with its natural language processing capabilities, can be employed to cluster similar data in quantitative research. By feeding the unstructured text data into ChatGPT-4, researchers can utilize its clustering functionality to group together similar responses based on semantic similarity. This allows researchers to identify common themes or sentiments within the data, enabling them to draw more meaningful conclusions.
Qualitative Research Methodologies
Qualitative research focuses on understanding social phenomena through in-depth exploration and interpretation of non-numerical data. It involves gathering rich textual or visual data, such as interviews, observations, or documents, and analyzing them holistically to generate insights and theories.
ChatGPT-4 can also be a valuable asset in qualitative research, particularly when dealing with large volumes of qualitative data. By leveraging its language understanding and clustering capabilities, researchers can use ChatGPT-4 to automatically group similar textual data. This streamlines the analysis process by allowing researchers to focus on specific themes or ideas within the data, enabling a more efficient analysis.
Data Clustering in Research
Data clustering is a fundamental technique in research that involves grouping similar data points together based on specific attributes or characteristics. The primary goal of data clustering is to simplify complex data sets and enable easier interpretation and analysis.
With ChatGPT-4's clustering capabilities, researchers can significantly benefit from its ability to automate the data clustering process. Instead of manually sorting through large amounts of data, researchers can rely on ChatGPT-4 to group similar data points together, saving both time and effort. This allows researchers to focus more on analyzing the clustered data and extracting meaningful insights from it.
Conclusion
The advancement of technologies like ChatGPT-4 has revolutionized the way researchers approach data analysis. By utilizing ChatGPT-4's natural language processing and clustering capabilities, researchers can efficiently organize and cluster similar data points, irrespective of whether they are dealing with quantitative or qualitative research methodologies. This empowers researchers to uncover patterns, trends, and relationships within their data and make evidence-based decisions in a more efficient manner.
Comments:
Thank you all for your interest in my article. I'm excited to discuss the power of ChatGPT in data clustering with you.
Great article, John! ChatGPT seems like a game-changer in the field of qualitative and quantitative research. How do you see it being implemented in real-world scenarios?
Thank you, Stephanie! ChatGPT can be utilized in various ways. It could assist researchers in analyzing survey responses, social media data, or even transcripts of interviews and focus groups. By understanding the context and clustering the data, it can uncover patterns and insights efficiently.
Impressive article indeed, John! I'm particularly interested in understanding how ChatGPT handles ambiguous or incomplete data. Can you shed some light on that?
Thank you, Michael! ChatGPT excels in handling ambiguous and incomplete information. It can make educated guesses based on the available data and propose clusters that highlight uncertainties. Researchers can then review these clusters and refine them if needed.
John, your article is eye-opening! I'm curious to know if ChatGPT can adapt to different research domains. For example, can it be used in market research as effectively as in social sciences?
Absolutely, Emily! ChatGPT's flexibility allows it to be applied across various research domains. It can be trained on specific datasets, enabling it to understand the nuances and jargon of different fields. This makes it highly adaptable to different research contexts.
I appreciate the informative article, John. However, have you conducted any comparative studies to assess the accuracy and efficiency of ChatGPT in data clustering compared to existing methodologies?
Thank you, David. Yes, I have conducted comparative studies with other clustering methods. Initial results indicate that ChatGPT performs competitively in terms of accuracy and efficiency. However, further research and evaluation are necessary for a comprehensive understanding of its strengths and limitations.
Great article, John! I wonder if ChatGPT is accessible and user-friendly for researchers with limited technical expertise?
Thanks, Jennifer! OpenAI has been working on simplifying the deployment and usage of ChatGPT for non-technical users. The aim is to make it accessible through user-friendly interfaces and tools, allowing researchers with limited technical expertise to benefit from its capabilities.
John, your article is fascinating! I'm curious about the potential ethical considerations when using ChatGPT for data clustering. How can we ensure fairness and avoid biases?
Thank you, Jason! Ethical considerations are crucial when working with AI. Researchers should be cautious and proactive in evaluating and mitigating biases. It's essential to train ChatGPT with diverse and representative datasets while constantly monitoring and fine-tuning the clustering process to reduce bias and ensure fairness.
Interesting article, John! What kind of computational resources are required to leverage the power of ChatGPT for data clustering effectively?
Thanks, Sarah! ChatGPT can be resource-intensive, especially for large-scale clustering tasks. It benefits from high-performance hardware, such as GPUs, to ensure quicker processing and analysis. However, as technology advances, the computational requirements are becoming more accessible, making it easier for researchers to leverage ChatGPT.
This is a groundbreaking approach, John! Could you share any success stories or practical applications where ChatGPT has already been used in data clustering?
Thank you, Rebecca! ChatGPT is relatively new, but there have been successful applications in clustering user feedback for product improvement, identifying social media trends, and analyzing customer support interactions to enhance service quality. The possibilities are vast, and researchers are exploring its potential in diverse fields.
John, your article is inspiring! Can ChatGPT handle large datasets effectively, or are there any limitations in terms of scalability?
Thanks, Daniel! ChatGPT has shown promising results with large datasets, but scalability can be a challenge. As the dataset size increases, the processing time and resource requirements also grow. To handle massive datasets effectively, researchers often need to explore techniques like clustering on subsets or leveraging distributed computing resources.
John, your article raises exciting possibilities! Are there any privacy concerns when using ChatGPT for data clustering, especially with sensitive data?
Thank you, Olivia! Privacy is indeed a significant concern. When dealing with sensitive data, it's crucial to follow best practices for data handling and ensure compliance with privacy regulations. Anonymization techniques, secure data storage, and access restrictions can help protect sensitive information while benefiting from ChatGPT's clustering capabilities.
Great work, John! What are the key considerations researchers should keep in mind while using ChatGPT for data clustering?
Thanks, Robert! Researchers should consider multiple factors. First, they should define clear objectives and choose the appropriate clustering technique based on their research question. They should also assess the quality of the training data and ensure its representativeness. Lastly, researchers should critically evaluate the clustered results and iterate on the process if necessary.
John, your article is enlightening! Could you share any limitations or challenges in using ChatGPT for data clustering?
Thank you, Angela! While ChatGPT shows great potential, it's important to acknowledge some limitations. It may struggle with rare or highly specialized domains where training data is limited. Additionally, understanding subtle context in certain languages or cultures could be challenging. Continuous research and improvement are necessary to overcome these limitations.
John, I'm amazed by ChatGPT's capabilities! How do you see this technology advancing in the future, specifically for data clustering?
Thanks, Emma! In the future, I see ChatGPT evolving to handle more complex domains and becoming more adaptable to various languages and cultures. There will likely be improvements in scalability, efficiency, and user-friendliness too, making it highly useful for data clustering in diverse research fields.
Great insights, John! How did you validate the accuracy of ChatGPT's clustering results during your experiments?
Thank you, Matthew! The accuracy of ChatGPT's clustering results was validated through a combination of qualitative and quantitative methods. Ground truth comparison with expert human clustering, evaluating clustering metrics like purity and F1 score, and analyzing the consistency of cluster assignments were some of the techniques used to validate the results.
John, your article is thought-provoking! Are there any limitations in the interpretability of ChatGPT's clustering results? How can researchers extract meaningful insights from the clustered data?
Thanks, Maria! The interpretability of ChatGPT's clustering results can be a challenge. Researchers can enhance interpretability by visualizing clusters, summarizing key features or keywords within each cluster, and exploring cluster summaries to extract meaningful insights. Iterative refinement and an interactive feedback loop with human experts can also help reveal more nuanced insights.
John, your article is brilliant! How does ChatGPT handle high-dimensional data and ensure efficient clustering?
Thank you, Michelle! ChatGPT has been designed to handle high-dimensional data by leveraging techniques like dimensionality reduction and applying clustering algorithms optimized for such data. By reducing the dimensionality, it can avoid the curse of dimensionality and improve efficiency in the clustering process.
Impressive article, John! Can ChatGPT handle streaming data effectively, or is it more suitable for batch processing?
Thank you, Brian! While ChatGPT is primarily designed for batch processing, it's possible to adapt it for streaming data scenarios. By using sliding windows or incremental clustering algorithms, researchers can process streaming data in smaller batches, ensuring that ChatGPT's clustering capabilities can be effectively utilized.
John, your article is fascinating! Are there any limitations when it comes to the size of clusters ChatGPT can handle effectively?
Thanks, Amy! The size of clusters ChatGPT can handle effectively is dependent on multiple factors, including the available computational resources and the complexity of the data. While the focus is often on identifying smaller, more meaningful clusters, ChatGPT can also handle larger clusters, but it may face challenges in capturing intricate patterns within them.
Great insights, John! How does ChatGPT handle noisy data, outliers, or data points that do not fit into any cluster?
Thank you, Daniel! ChatGPT can handle noisy data by allocating it to a separate cluster or classifying it as an outlier. To identify such data points, researchers can leverage techniques like density-based clustering or use predefined thresholds to classify them accordingly. This allows researchers to explore and analyze outliers separately from the clustered data.
John, your article is enlightening! Could you share any recommended pre-processing steps to ensure the quality and reliability of ChatGPT's clustering results?
Thanks, Rachel! Several pre-processing steps are recommended. Firstly, ensure data cleaning by removing inconsistencies, irrelevant content or noise. Preprocessing can also involve techniques like tokenization, stemming or lemmatization, and removing stop words. Lastly, feature scaling or selection may be applied depending on the clustering algorithm and data characteristics to improve clustering performance.
John, your article is eye-opening! Can ChatGPT handle real-time feedback from human experts to refine and improve the clustering process?
Thank you, Anthony! Absolutely, ChatGPT can benefit from real-time feedback from human experts. Researchers can collaboratively refine and improve the clustering process by iteratively engaging with the system. By incorporating domain expertise and corrective feedback, researchers can enhance the accuracy and relevance of the clustering results.
John, your article is fascinating! Can ChatGPT handle clustering tasks that involve multiple types of data, such as text, images, and numbers?
Thanks, Natalie! ChatGPT's capabilities extend beyond just text data. It can potentially handle clustering tasks involving multiple types of data, including images and numerical features. By combining different modalities and adapting the clustering approach accordingly, it can provide a comprehensive analysis of heterogeneous data for researchers in various domains.
John, your article is brilliant! How can ChatGPT aid in extracting semantic relationships and similarities between data points during the clustering process?
Thank you, Eric! ChatGPT has the ability to learn semantic relationships and identify similarities between data points during the clustering process. By leveraging its contextual understanding, it can establish connections based on meaning or content similarity to create clusters of related data points. This aids in extracting meaningful insights and detecting underlying patterns within the data.
John, your article was a great read! How can researchers effectively evaluate the quality and usefulness of ChatGPT's clustering results?
Thanks, Laura! Researchers can evaluate the quality and usefulness of ChatGPT's clustering results through various means. They can benchmark the results against expert human clustering or existing gold-standard datasets. They can also assess the comprehensibility and relevance of the generated clusters by involving domain experts or considering feedback from end-users to ensure the usefulness of the insights extracted.
Great article, John! Are there any limitations in terms of the development of ChatGPT for languages other than English?
Thank you, Brandon! While ChatGPT initially focused on English, OpenAI has been working on expanding its capabilities to handle languages other than English. However, developing high-quality language models for other languages can pose unique challenges in terms of data availability, cultural nuances, and linguistic variations. Nonetheless, advancements are being made to make ChatGPT more versatile and inclusive.