Exploring the Role of ChatGPT in Sentiment Analysis for Language Services
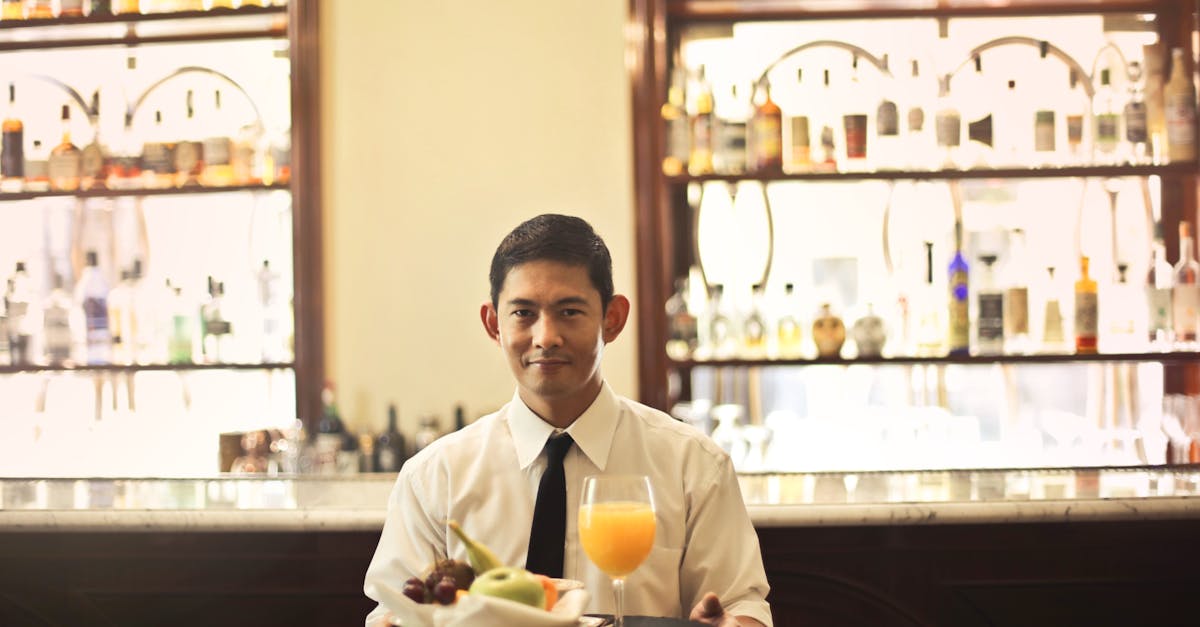
In today's digital age, there is an enormous amount of textual data available through various online channels, including social media, customer reviews, forums, and blogs. This abundance of information presents businesses with a significant opportunity to gain insights into public opinion and sentiment. This is where language services, specifically sentiment analysis, come into play.
Technology: Language Services
Language services encompass a wide range of technologies that enable computers to understand, interpret, and generate human language. Sentiment analysis, also known as opinion mining, is a form of natural language processing (NLP) that focuses on identifying and categorizing opinions expressed in a piece of text, such as positive, negative, or neutral sentiments.
Area: Sentiment Analysis
Sentiment analysis can be applied to various areas, including market research, customer feedback analysis, brand monitoring, reputation management, and social media analytics. By mining sentiments from textual data, businesses can gain valuable insights into the public perception of their products, services, or brand reputation.
Usage: Gather, Identify, and Categorize Opinions
The primary goal of sentiment analysis is to gather, identify, and categorize opinions expressed in a piece of text. This can be achieved through various techniques, such as rule-based methods, machine learning algorithms, or deep learning models.
Firstly, sentiment analysis technology gathers large volumes of textual data from different sources, such as customer reviews, social media posts, or online forums. This data is then processed to remove noise, such as stop words or punctuation, and normalize the text for analysis.
Next, the identified opinions in the extracted text are classified into sentiment categories, such as positive, negative, or neutral. Advanced sentiment analysis tools can even detect nuanced sentiments, such as sarcasm or irony.
Finally, the categorized opinions can be aggregated and analyzed to generate insights and actionable recommendations for businesses. For example, sentiment analysis can reveal customer satisfaction levels, identify potential issues or concerns, or gauge the success of marketing campaigns.
Conclusion
Sentiment analysis, as a language service technology, plays a crucial role in helping businesses make informed decisions based on public opinions expressed in textual data. By leveraging sentiment analysis, organizations can gain valuable insights into customer sentiment, enhance their brand reputation, improve products or services, and drive overall business success.
Overall, sentiment analysis is a powerful tool that allows businesses to tap into the abundance of opinions available in today's digital landscape, enabling them to make data-driven decisions and stay ahead in their respective industries.
Comments:
Thank you for reading my article on 'Exploring the Role of ChatGPT in Sentiment Analysis for Language Services'. I'm excited to hear your thoughts and opinions!
Great article, Je'quan! I found it really interesting how ChatGPT can be utilized for sentiment analysis. It seems like a promising tool for language services.
Hi Je'quan! Thanks for sharing your insights. I have a question: How does the accuracy of sentiment analysis with ChatGPT compare to other existing methods?
Hi Emma! Good question. The accuracy of sentiment analysis with ChatGPT has shown promising results, although it's important to note that it may not be as accurate as specialized sentiment analysis models. However, ChatGPT offers the advantage of versatility and can be more easily fine-tuned for specific domains or languages.
Interesting article, Je'quan! I'm curious to know if ChatGPT can handle sentiment analysis in languages other than English. Can you enlighten me on that?
Hey Liam! Yes, ChatGPT can handle sentiment analysis in languages other than English. While it was primarily trained on English text, it can be fine-tuned to support multiple languages. However, the accuracy might vary depending on the language and the available training data.
Thanks, Je'quan, for the informative article! I'm wondering if there are any limitations or challenges when using ChatGPT for sentiment analysis?
Hi Sophia! Absolutely, there are a few limitations. Since ChatGPT is trained on large amounts of internet data, it could potentially generate incorrect or biased sentiments. Fine-tuning can help alleviate this issue, but it's an important consideration. Additionally, it might not perform well on complex or nuanced sentiment analysis tasks.
I enjoyed reading your article, Je'quan! As someone in the language services field, I'm excited about the potential ChatGPT has for sentiment analysis. Do you think it could eventually replace human evaluators in certain scenarios?
Hello Elijah! Thank you for your comment. While ChatGPT shows promise in sentiment analysis, I don't think it can entirely replace human evaluators. Human judgment and context comprehension are essential in certain scenarios, especially when dealing with complex emotions or specific cultural contexts.
Great article, Je'quan! Could you share some use cases where ChatGPT can be effectively applied for sentiment analysis in language services?
Hi Ava! Absolutely, ChatGPT can be effectively applied for sentiment analysis in various language services. Some use cases include analyzing customer feedback, sentiment monitoring on social media platforms, and assessing sentiment in product or service reviews.
Fascinating article, Je'quan! I'm curious about the ethical aspects of using ChatGPT for sentiment analysis. Are there any concerns regarding bias or privacy when implementing it in language services?
Hi Oliver! Excellent question. Bias and privacy are indeed prime concerns. Large language models like ChatGPT have the potential to amplify or introduce biases present in the training data. Careful consideration and mitigation strategies are necessary to address these issues and ensure privacy compliance when using ChatGPT for sentiment analysis in language services.
Really engaging article, Je'quan! I'm wondering if you have any recommended resources or tools for someone looking to integrate ChatGPT into their language services for sentiment analysis.
Hey Emily! Thank you for your kind words. As for resources, OpenAI provides documentation and guidelines for fine-tuning GPT models like ChatGPT, which can be helpful for integrating it into language services. Additionally, various open-source libraries and frameworks exist that assist in working with GPT models.
Thanks for sharing your insights, Je'quan! I'm curious about the computational resources needed to perform sentiment analysis with ChatGPT. Can it be run on a regular machine, or does it require specialized hardware?
Hi Benjamin! ChatGPT can be run on a regular machine, but it is resource-intensive, especially for large-scale sentiment analysis tasks. For optimal performance, using specialized hardware like GPUs or TPUs can significantly speed up the analysis process.
Great article, Je'quan! I'm curious if ChatGPT can handle sentiment analysis in real-time. Is it suitable for processing a constant stream of data or better suited for static analysis?
Hello Isabella! ChatGPT is better suited for static analysis rather than real-time sentiment analysis. While it can process a constant stream of data, its response time might not be ideal for real-time applications where immediate sentiment analysis is required.
Thanks for the informative article, Je'quan! I'm curious to know if ChatGPT can differentiate between different levels of sentiment intensity, such as mild, moderate, or strong. Can it provide nuanced sentiment analysis?
Hi Lucas! While ChatGPT can capture sentiment intensity to some extent, it might not provide the level of nuanced sentiment analysis that specialized sentiment analysis models offer. It is more adept at identifying overall sentiment rather than finer-grained levels.
Wonderful article, Je'quan! I'm wondering if ChatGPT can handle sentiment analysis on short texts or if it is more suitable for longer documents?
Hey Grace! ChatGPT can handle sentiment analysis on both short texts and longer documents. However, due to its nature as a language model, it might perform better on longer texts where there is more contextual information available.
Nice work, Je'quan! Considering the advancements in AI and sentiment analysis, do you think ChatGPT will further improve in the future, addressing some of the limitations it currently has?
Hi Daniel! Indeed, the field of AI and sentiment analysis is continuously evolving. With ongoing research, fine-tuning techniques, and access to more diverse and high-quality training data, I believe ChatGPT will improve further, addressing some of the current limitations.
Thanks for sharing this, Je'quan! I'm curious if ChatGPT can also identify sarcasm and understand its sentiment correctly. Does it have that capability?
Hi Zoe! ChatGPT has demonstrated some capability to understand sarcasm, but it may not always accurately interpret the sentiment behind sarcastic remarks. Detecting and interpreting sarcasm remains a challenge even for advanced language models.
Really enjoyed your article, Je'quan! I'm wondering how long it typically takes to fine-tune ChatGPT for sentiment analysis tasks. Is it a time-consuming process?
Hello Connor! The time required to fine-tune ChatGPT for sentiment analysis tasks depends on various factors, including the size of the training dataset, computing resources, and the desired level of accuracy. It can range from a few hours to several days, and in some cases even longer.
Thank you for this informative article, Je'quan! I'm curious if ChatGPT can account for sentiment variations across different demographics or cultural backgrounds. Is it able to adapt to diverse contexts?
Hi Madison! ChatGPT can adapt to some extent based on the training data it has been fine-tuned on, which can include diverse contexts. However, it's important to closely monitor and account for any biases that may arise when analyzing sentiment across different demographics or cultural backgrounds.
Great insights, Je'quan! I'm interested to know if ChatGPT can handle sentiment analysis for different industries. Does it require domain-specific training to be effective in various fields?
Hi Samuel! ChatGPT can be fine-tuned for specific industries or domains, which improves its effectiveness in various fields. Domain-specific training helps the model grasp the particular nuances and jargon of a given industry, leading to better sentiment analysis results.
Very insightful article, Je'quan! I'm wondering if there are any notable applications of sentiment analysis with ChatGPT that you can share.
Hey Ella! Sentiment analysis with ChatGPT has various applications. Some notable ones include analyzing customer feedback to improve products and services, tracking public sentiment towards brands or events on social media, and assisting in market research to gauge consumer opinions and preferences.
Thanks for sharing this, Je'quan! I'm wondering if ChatGPT can handle sentiment analysis for informal texts or online conversations where the language is less formal. Does it perform well in such scenarios?
Hi Leo! ChatGPT can perform reasonably well in sentiment analysis for informal texts or online conversations. Being trained on internet data, it has exposure to informal language usage. However, it's important to consider the potential impact of noise and inaccuracies in informal texts on sentiment analysis results.
Very well-written article, Je'quan! I'm curious if ChatGPT can handle sentiment analysis for individual sentences within a larger text or if it primarily focuses on the overall sentiment of the entire text?
Hello Hailey! ChatGPT can handle sentiment analysis for individual sentences within a larger text. While it captures the overall sentiment of the entire text, it can also provide sentiment analysis at a more granular sentence level.
Thank you for sharing your knowledge, Je'quan! I'm wondering if there are any specific challenges when fine-tuning ChatGPT for sentiment analysis in languages other than English.
Hi Owen! Fine-tuning ChatGPT for sentiment analysis in languages other than English can pose challenges such as the availability of labeled training data, ensuring the quality of translations while creating labeled datasets, and adapting the model to the different nuances and linguistic features of the target language.
Great article, Je'quan! Can ChatGPT handle sentiment analysis in noisy text with typos or abbreviations commonly found in social media conversations?
Hi Sophie! ChatGPT can handle sentiment analysis in noisy text to some extent, including typos and abbreviations commonly found in social media conversations. However, its performance might be affected by unusual spellings, abbreviations, or slang words that it may not have encountered during training.
Very insightful article, Je'quan! I'm curious about the data requirements for fine-tuning ChatGPT for sentiment analysis. How much labeled data is typically needed?
Hey Harper! The labeled data requirements for fine-tuning ChatGPT depend on various factors, including the desired accuracy, domain specificity, and the complexity of the sentiment analysis task. While thousands of labeled examples can be ideal, even a few hundred labeled examples can yield reasonably decent results.
Thank you for sharing your insights, Je'quan! I'm interested in knowing the potential impact of bias in training data on sentiment analysis results with ChatGPT. How is it mitigated?
Hi Mason! Bias in training data can indeed impact sentiment analysis results with ChatGPT. To mitigate it, careful preprocessing of training data is required to reduce biases, and developers need to incorporate diverse and representative datasets. Regular evaluation, fine-tuning, and manual bias checking are essential steps in ensuring that the model provides fair and unbiased sentiment analysis.
This was a fascinating read, Je'quan! Could you highlight any potential future advancements or directions in sentiment analysis for language services?
Hello Scarlett! The future of sentiment analysis for language services holds exciting possibilities. Advancements may include better models for sarcasm detection, improved handling of nuanced sentiments, enhanced support for different languages, and increased efforts to address bias and privacy concerns. Additionally, incorporating multimodal approaches by combining text, images, and audio data could further enhance sentiment analysis capabilities.