Exploring the Role of Gemini in Advancing Technological Rheology
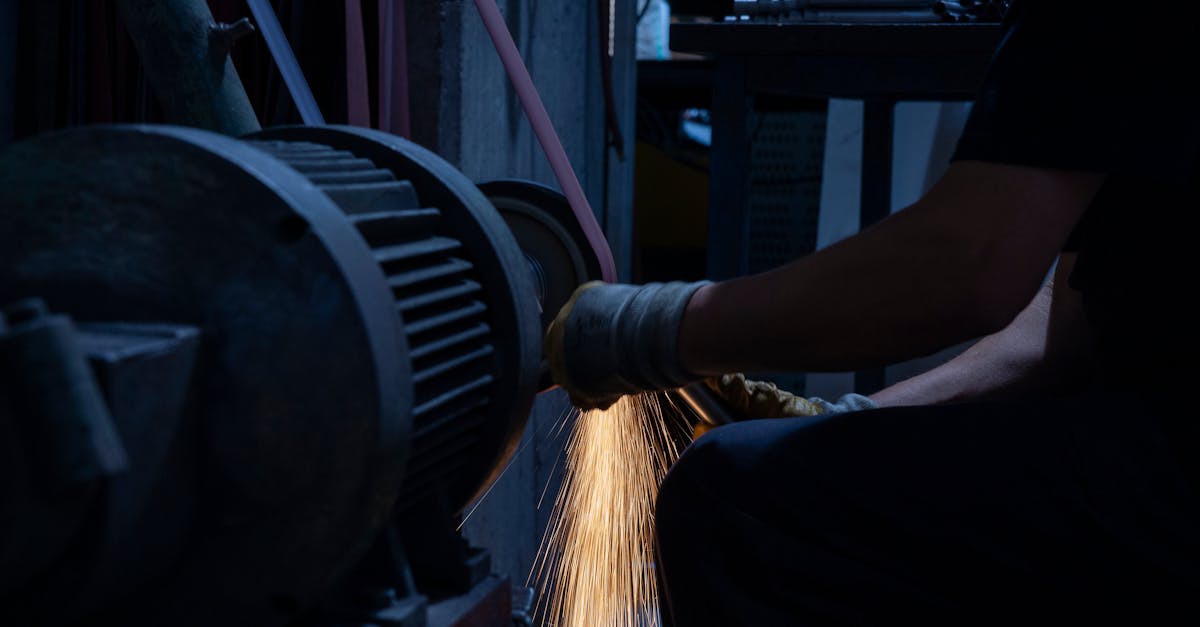
Rheology, as a scientific field, has witnessed significant advancements in recent years, owing to the integration of emerging technologies. Artificial Intelligence (AI) and machine learning have played a crucial role in enhancing various domains, and one such technology, Gemini, has shown promising results in advancing technological rheology.
Understanding Rheology
Rheology is the study of how materials deform and flow under mechanical forces or stresses. It primarily focuses on the relationship between force, deformation, and the material's response. Understanding the rheological properties of various substances is crucial in industries such as manufacturing, cosmetics, food processing, pharmaceuticals, and more.
The Role of Gemini
Gemini, a language model developed by Google, has revolutionized the way we interact with AI. Built upon the successful LLM (Generative Pre-trained Transformer) architecture, Gemini takes AI assistance to a whole new level. Its ability to generate human-like responses makes it an ideal tool for exploring and advancing technological rheology.
1. Data Analysis and Modeling
Gemini can analyze large volumes of rheological data, helping scientists identify patterns, correlations, and anomalies. By understanding the data, experts can gain insights into the behavior of materials under different conditions. With these insights, engineers can develop more effective models for predicting rheological properties and their relationship with external factors.
2. Virtual Experiments and Simulations
Gemini enables researchers to conduct virtual experiments and simulations using machine learning techniques. By inputting relevant parameters, scientists can obtain simulated outputs, such as stress-strain curves, viscosity profiles, and other rheological parameters. This allows for faster and more cost-effective research, reducing the need for physical experiments.
3. Enhanced Quality Control
Rheological tests are crucial in ensuring quality control in industries such as manufacturing and pharmaceuticals. With the assistance of Gemini, real-time analysis of rheological data can be performed, enabling better quality control throughout the production process. Any discrepancies or deviations from desired rheological properties can be identified and rectified promptly, saving time and resources.
4. Expert Knowledge and Assistance
Gemini can also act as a virtual assistant, providing expert knowledge and guidance in navigating the complexities of rheology. Researchers and engineers can interact with Gemini to seek advice, ask questions, and explore new possibilities. This enables better collaboration and learning in the field, further advancing the knowledge and application of rheology.
The Future of Technological Rheology
Gemini's potential in advancing technological rheology is immense. As AI continues to evolve, so will Gemini, further enhancing its analytical capabilities and expanding its scope. With ongoing advancements, we can expect Gemini to become an indispensable tool in the field of rheology.
With the integration of Gemini, researchers, engineers, and industries can unlock new avenues of innovation, improve productivity, and develop more efficient materials and products. The transformative potential of Gemini in technological rheology is just beginning to unfold, and its impact is set to accelerate in the coming years.
Comments:
Thank you all for taking the time to read my article on the role of Gemini in advancing technological rheology! I'm excited to engage in a discussion with you.
Great article, Gabriel! I wasn't familiar with the concept of 'technological rheology' before. Can you explain how Gemini is being used to advance this field?
Thank you, Michael! Technological rheology is the study of how materials flow and deform under applied forces. Gemini is being used to simulate and predict the rheological behavior of various materials. By training the model on data from different processes and experimental results, it can provide valuable insights and help optimize industrial processes.
Interesting application, Gabriel! Do you think Gemini can eventually replace traditional rheological testing methods?
That's a great question, Emily! While Gemini can assist in rheological predictions, it's not meant to replace traditional testing methods entirely. The model's predictions can guide experimental design and provide quick estimates, but real-world testing is still necessary for accurate and reliable results.
I'm impressed by how AI is being applied to such specific fields. Gabriel, do you think Gemini can handle complex rheological behaviors, like viscoelasticity?
Absolutely, Oliver! Gemini has the potential to tackle complex rheological behaviors, including viscoelasticity. These behaviors can be challenging to model, but with the right training data and adjustments to the model architecture, it can learn to capture the intricate characteristics of materials exhibiting viscoelastic properties.
I wonder how domain-specific the training data needs to be for Gemini to provide accurate predictions in rheology.
Good point, Sarah! Domain-specific training data is crucial for accurate predictions in rheology. It's important to train the model on a wide range of materials, manufacturing processes, and experimental data to ensure its predictions are reliable and applicable in various scenarios.
Gabriel, are there any limitations or challenges when using Gemini for rheology predictions?
Definitely, Matthew! Gemini has limitations, including its reliance on the quality and diversity of training data. If the data is biased, the model's predictions can be skewed. Additionally, Gemini might struggle with extrapolation beyond its training data, so it's vital to perform rigorous testing and validation to ensure accurate results.
Impressive article, Gabriel! I can see how Gemini's capabilities can revolutionize the field of rheology. Do you have any recommendations for incorporating Gemini into industrial processes?
Thank you, David! To incorporate Gemini into industrial processes, it's crucial to collaborate with experts in rheology and AI. Developing a robust data collection strategy, ensuring the model's predictions align with real-world experiments, and continuously refining the model based on feedback and new data are key steps for successful integration.
Gabriel, where do you see the future of Gemini in the field of technological rheology?
Great question, Sophia! The future of Gemini in technological rheology looks promising. As the model improves and the training data becomes more extensive, it has the potential to become a valuable tool for material scientists and engineers, aiding in the design of novel materials and optimizing industrial processes to enhance efficiency and reduce costs.
Gemini's potential impact on rheology is fascinating! Gabriel, do you see any ethical concerns or risks associated with using AI in this field?
Absolutely, Jason! The ethical concerns involve data privacy and ensuring the responsible use of AI. It's crucial to handle sensitive data appropriately and address any biases that may exist in the training data. Transparency in AI's decision-making process is vital to build trust and mitigate risks.
Gabriel, can Gemini be used to analyze the impact of different flow rates and temperatures on material behavior?
Yes, Natalie! Gemini can be trained on data that includes different flow rates and temperatures. By analyzing this data, it can make predictions about the impact of these factors on material behavior, helping researchers and engineers understand how different process parameters affect rheological properties.
Thank you for this informative article, Gabriel! Is Gemini widely accessible for researchers and industries working with rheology?
You're welcome, Andrew! Gemini is becoming increasingly accessible to researchers and industries. Google has plans to release more advanced and domain-specific versions of the model, which will offer improved performance and cater to specialized needs. This accessibility will greatly benefit the field of rheology.
Gabriel, how does the accuracy of Gemini's predictions compare to traditional rheological modeling approaches?
Good question, Jennifer! Gemini's accuracy depends on the quality and representativeness of its training data. While it can provide quick estimates, it may not match the accuracy of traditional rheological modeling approaches that are typically built on extensive experimental data and physical models. However, Gemini's predictions can still be valuable in early-stage design and optimization processes.
Fascinating article, Gabriel! Can Gemini be used to predict the behavior of complex multi-component materials?
Thank you, Richard! Gemini can indeed be used to predict the behavior of complex multi-component materials. By training the model on data that includes various components and their interactions, it can learn to simulate the behavior of such materials and aid in their characterization and optimization.
Gabriel, what are the computational requirements for using Gemini effectively in rheology?
Good question, Rebecca! Gemini can be resource-intensive in terms of computational requirements, especially when training the model on large datasets. However, when it comes to predicting rheological behaviors, the computational demands are relatively lower for the application phase, enabling it to be used effectively even on moderate computing resources.
Gabriel, what kind of applications or industries can benefit the most from incorporating Gemini in their rheology research?
Great question, Michelle! Industries such as polymer processing, food and beverage, pharmaceuticals, and materials engineering can benefit significantly from incorporating Gemini in their rheology research. Gemini can aid in process optimization, new material design, and troubleshooting, leading to enhanced efficiency, cost savings, and improved product quality.
Gabriel, how does the accuracy of Gemini's predictions vary across different material types?
Good question, Samuel! Gemini's prediction accuracy can vary across different material types based on the availability and representativeness of training data. For materials that have similar characteristics to the ones in the training data, the predictions are likely to be more accurate. However, for materials with unique properties or limited training data, extra caution and validation are necessary.
Gabriel, how do you see the collaboration between material scientists and AI experts shaping the future of rheology research?
Excellent question, Ethan! The collaboration between material scientists and AI experts holds great potential for advancing rheology research. Material scientists can provide the domain expertise and guidance needed to collect relevant training data, while AI experts can develop and refine AI models like Gemini to analyze and make predictions based on that domain-specific data. Together, they can unlock new insights and push the boundaries of rheology research.
Great work, Gabriel! How can researchers ensure the interpretability of Gemini's predictions, particularly in complex rheological systems?
Thank you, Daniel! Ensuring the interpretability of Gemini's predictions is essential in complex rheological systems. Researchers can work on developing techniques to explain the model's decisions, such as attention mechanisms or saliency analysis. These techniques can provide insights into which factors or features the model is focusing on when making its predictions, adding transparency and aiding interpretation.
Gabriel, what are the key factors to consider when selecting appropriate training data for Gemini in rheology?
That's a crucial question, Sophie! When selecting training data for Gemini in rheology, it's important to consider the diversity of materials, manufacturing processes, and experimental data. The training data should cover a wide range of scenarios, ensuring that the model captures the broad spectrum of rheological behaviors it might encounter in real-world applications.
Gabriel, how can researchers effectively validate the predictions made by Gemini in rheology?
Validating Gemini's predictions in rheology is crucial, Olivia. Researchers can do so by comparing the model's predictions with existing experimental data or conducting new experiments based on valuable insights provided by the model. Continuously iterating and refining the model based on this validation process will contribute to increased reliability and trustworthiness of its predictions.
Gabriel, can Gemini handle time-dependent rheological behaviors, such as non-Newtonian fluids?
Absolutely, Chris! Gemini can handle time-dependent rheological behaviors, including non-Newtonian fluids. By training the model on data that includes these behaviors, it can learn to simulate and predict the viscosity and flow properties of such fluids under various conditions.
Gabriel, in terms of computational costs, how does training Gemini for rheology compare with other AI models?
Sophia, training Gemini for rheology can be computationally expensive due to the model's large size and the need for extensive training data. However, with the right computing resources, it is feasible. In comparison to some other AI models, Gemini offers an advantage by being able to assist in generating human-readable text, which is beneficial for communication with researchers and engineers in the field of rheology.
Gabriel, how can industries ensure proper deployment and integration of Gemini-based systems in their production lines?
A crucial question, Liam! Proper deployment and integration of Gemini-based systems require a well-defined and robust implementation plan. It's important to thoroughly validate the model's predictions against real-world data in a controlled environment. Conducting pilot studies and gradually integrating the system into production lines, while closely monitoring and validating its performance, will help ensure its proper deployment and effectiveness in industrial settings.
Gabriel, as the technology advances, do you envision Gemini being able to actively learn from new experimental data to improve its predictions over time?
Definitely, Emma! As the technology advances, Gemini can be designed to actively learn from new experimental data and user feedback. Continuously updating and retraining the model with new data will help improve its predictions over time, making it more accurate and adaptable to evolving rheological challenges.
Gabriel, what potential enhancements or additions do you see being made to Gemini specifically for rheology research?
An excellent question, Melissa! Potential enhancements to Gemini for rheology research could include incorporating more domain-specific knowledge, expanding the training data to cover a wider range of materials and process conditions, and refining the model architecture to capture even more complex behaviors. Feedback from rheology experts and ongoing research will drive these improvements, making Gemini an even more valuable tool in the field.
Thank you for sharing your insights in this article, Gabriel! It's exciting to see AI playing a role in advancing technological rheology. I look forward to seeing how Gemini continues to develop and benefit the field.
Thank you all for taking the time to read my article on the role of Gemini in advancing technological rheology. I'm excited to hear your thoughts and perspectives on this topic!
Great article, Gabriel! I found it fascinating how Gemini can contribute to technological rheology. It has the potential to revolutionize the field by enhancing material properties and analyzing structural behavior. Looking forward to more research in this area!
Natalie, I agree! Gemini offers a unique way to study rheological properties and predict material responses under different conditions. It could lead to more efficient design processes and improved product performance.
Interesting read, Gabriel. I can see Gemini being particularly valuable in simulating the dynamic behavior of complex fluids. It opens up new avenues for fluid analysis and optimization. Well done!
I think Gemini could also help in understanding the flow characteristics of non-Newtonian fluids. It's great how AI is contributing to advancements in rheology!
Gabriel, your article was enlightening. I'm curious about the limitations of Gemini in modeling complex fluid behavior. Are there any challenges you foresee?
Thanks for your question, Linda! While Gemini offers remarkable capabilities, it may struggle when dealing with highly non-linear fluid models. Further research is needed to enhance its accuracy in such scenarios.
Gabriel, your article was thought-provoking. I wonder how Gemini's abilities can be leveraged in industrial applications. Can you provide some examples?
Certainly, Yusuf! Gemini can assist in optimizing production processes of viscoelastic materials, predict flow patterns in pipes, and even aid in designing efficient mixing techniques. Its versatility makes it valuable across various industries.
This article shed light on an exciting area of research. I'm curious, Gabriel, how accessible is Gemini for researchers and engineers who want to apply it in their work?
Great question, Sophia! Gemini is fairly accessible, but it requires some technical expertise to adapt and train it for specific tasks. Google provides guides and resources to help users get started.
Sophia, while Gemini is accessible, it's important to note that its usage should be combined with domain expertise. Collaborations between AI experts and rheology specialists can yield the best results.
Gabriel, I'm impressed with the potential applications of Gemini in the field of rheology. However, I'm curious about the ethical considerations and implications of using AI in this domain.
Emily, ethics is indeed an important aspect to consider. While AI can greatly benefit rheology, it's crucial to ensure responsible and unbiased use. Regulations and guidelines must be in place to address potential risks and biases associated with AI models.
Congratulations on the article, Gabriel! I'm intrigued by the idea of AI assisting in material design. How do you think Gemini can contribute in this area?
Thank you, Jennifer! Gemini can help researchers explore and identify optimal material compositions based on desired properties. It can accelerate the material design process through simulations and data analysis.
Gabriel, as AI systems like Gemini continue to evolve, do you anticipate them completely automating the rheology field, or will they primarily serve as powerful tools for human professionals?
Excellent question, Oliver! While AI can augment and streamline rheology research, I believe human expertise will remain essential. AI systems like Gemini serve as powerful tools, but human professionals bring critical insights and domain knowledge to the table.
Nice article, Gabriel! I wonder if Gemini has any applications in studying the complex behavior of multiphase flows?
Thanks, David! Gemini can indeed be used to understand and analyze multiphase flows. Its ability to process and interpret large amounts of data makes it valuable in studying the dynamic behavior and interactions between different fluid phases.
Gabriel, your article gave me a new perspective on the role of AI in rheology. Are there any specific challenges or limitations you faced while working with Gemini for this research?
Richard, one challenge was ensuring the accuracy and reliability of predictions made by Gemini. It required extensive training and fine-tuning to optimize its performance. Additionally, interpreting the model's outputs and validating them with experimental data presented some difficulties.
Gabriel, I appreciate the depth of information in your article. How do you envision Gemini influencing future research and innovation in rheology?
Thank you, Michelle! Gemini has the potential to accelerate research and innovation in rheology by providing researchers with powerful insights and enabling faster simulations. It can enhance our understanding of complex fluid behavior and lead to the development of advanced materials with improved properties.
Impressive article, Gabriel! I'm curious how Gemini's performance compares to traditional rheological models. Are there any benchmarks or studies conducted?
Samuel, comparing Gemini's performance to traditional models is an ongoing area of research. Initial results show that it can offer competitive predictions, especially in complex scenarios. However, further benchmarking and validation against established models is necessary for comprehensive evaluation.
Congratulations on the informative article, Gabriel! I'm curious if Gemini's application in rheology can lead to breakthroughs in other scientific fields as well.
Thank you, Peter! Gemini's application in rheology can definitely inspire breakthroughs in related scientific fields. Its ability to understand and model complex systems can contribute to advancements in various domains, including material science and fluid dynamics.
Great article, Gabriel! I'm intrigued by Gemini's potential in predicting the behavior of complex fluid mixtures. Are there any specific areas where it has shown promising results?
Robert, Gemini has shown promising results in predicting the behavior of polymer blends and suspensions. It can help researchers understand the impact of different factors on the final properties of complex fluid mixtures, leading to more efficient formulations.
Gabriel, your article was enlightening. In terms of implementation, what computational resources are typically required to train and deploy Gemini models for rheology?
Sara, training and deploying Gemini models for rheology often require significant computational resources. High-performance GPUs and large datasets are typically used in the training process. Deployment can vary depending on the specific application, but it usually involves running the models on powerful hardware or cloud computing services.
Congratulations on the well-researched article, Gabriel! I'm curious to know if Gemini's predictions in rheology align with experimental observations or if further validation is needed.
Laura, validating Gemini's predictions with experimental data is crucial to ensure accuracy. Although initial validations show promising alignment with observations, further experimental collaboration and fine-tuning are necessary to improve reliability and build more confidence in the model's predictions.
Your article was an engaging read, Gabriel! I'm curious about any potential risks or challenges associated with using AI models like Gemini in the field of rheology. Could you shed some light on this?
Isabella, there are a few risks and challenges to consider when using AI models in rheology. These include the ethical implications of biased or inaccurate predictions, the potential for data privacy concerns, and the need for transparent and explainable AI. Addressing these challenges is crucial to ensure responsible and effective use of AI in the field.
Gabriel, your article was comprehensive and well-written. Do you see any barriers that might hinder the widespread adoption of Gemini or similar AI systems in rheology?
Andrew, while Gemini and similar AI systems have great potential, some barriers to widespread adoption include the need for specialized technical knowledge, computational resources, and the time required for training and fine-tuning. Overcoming these barriers will be essential to facilitate broader use and maximize the impact of AI in rheology.
Congratulations, Gabriel, on an informative article! I'm curious if Gemini can be used to analyze time-dependent material properties. Can it capture the behavior of materials under varying conditions?
Sophie, Gemini can indeed analyze time-dependent material properties. By training the model on relevant datasets and introducing suitable conditioning, it becomes capable of capturing the behavior of materials under varying conditions over time. It opens up possibilities for studying viscoelastic materials and their responses to dynamic forces.
Gabriel, your research is impressive! Can Gemini be used to investigate the effect of temperature and other environmental factors on the rheological behavior of materials?
Thank you, Jason! Gemini can certainly be used to explore the relationship between temperature, environmental factors, and rheological behavior. It can assist in analyzing how these variables affect material properties and predict responses under different conditions, aiding in the development of materials that perform optimally in specific environments.
Excellent article, Gabriel! I'm curious if Gemini can be applied to predict the behavior of materials at micro and nanoscales, where structural effects play a significant role.
Brandon, Gemini can be a valuable tool in predicting the behavior of materials at micro and nanoscales. By considering structural effects and training the model on relevant data, it becomes capable of capturing the intricate behavior of materials at these scales. This opens up possibilities for designing materials with desired properties at the micro and nanoscale level.
Gabriel, your article was incredibly informative! I was wondering if Gemini's predictions in rheology can be integrated with other data-driven technologies to provide a more comprehensive understanding of complex fluid behavior?
Thank you, Julia! Absolutely, integrating Gemini's predictions with other data-driven technologies, such as machine learning and computational simulations, can enhance our understanding of complex fluid behavior. Combining multiple approaches can provide a more comprehensive and accurate representation of rheological phenomena, enabling better designs and optimizations.
Gabriel, your article delves into exciting possibilities. I'm curious if Gemini can assist in predicting long-term material aging and degradation, which play a critical role in various engineering applications.
Alex, Gemini can be utilized in predicting long-term material aging and degradation. By training the model on historical data and incorporating appropriate time-dependent constraints, it becomes capable of capturing the effect of aging and predicting material behavior over extended periods. This has implications in engineering applications, where understanding deterioration is crucial.
Congratulations on an insightful article, Gabriel! I'm curious about the computational efficiency of Gemini in modeling complex rheological phenomena. Does it require substantial computational resources?
Thank you, Lisa! Modeling complex rheological phenomena with Gemini can indeed require substantial computational resources, depending on the complexity of the problem and the size of the dataset. High-performance GPUs and efficient parallel computing techniques are often used to accelerate the processing capabilities.